Abstract
This work presents supervised machine learning techniques for the problem of virtualization design with protection over elastic optical networks (EONs) for predicting the total number of used spectrum slots to support all traffic demands. It considers virtual optical networks (VONs) subject to protection and proposes learning techniques to solve the link capacity problem of EONs with virtualization faster than traditional integer linear programming (ILP) formulations, but keeping the finds near to the optimal ones. The performance of the models were evaluated using statistical metrics, along with the time for training and performing inferences, both using quantitative and qualitative analysis. They showed that the proposed method is effective for predicting the number of required slots (bandwidth) on physical substrate subject to several VONs.














Similar content being viewed by others
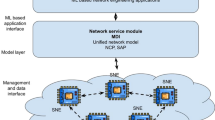
References
Assis, K., et al.: Network virtualization over elastic optical networks with different protection schemes. IEEE/OSA J. Opt. Commun. Netw. 8(4), 272–281 (2016)
Assis, K.D.R., Almeida, R.C.D.A., Dos Santos, A.F., Dias, L.P., Giozza, W.F., De Sousa, R.T., Jaumard, B., Nejabati, R., Simeonidou, D.: Channel-based rsa approaches for qos protection of slices over elastic optical networks. IEEE Access 10, 20714–20726 (2022)
Assis, K.D.R., Almeida, R.C., Dias, L.P., Waldman, H.: Squeezed protection in elastic optical networks subject to multiple link failures. IEEE Trans. Netw. Serv. Manage. 18(3), 2612–2626 (2021)
Assis, K., Almeida, R., Jr., Reed, M., Santos, A., Dinarte, H., Chaves, D., Li, H., Yan, S., Nejabati, R., Simeonidou, D.: Protection by diversity in elastic optical networks subject to single link failure. Opt. Fiber Technol. 75, 103208 (2023)
Bui, M., Jaumard, B., Cavdar, C., Mukherjee, B.: Design of a survivable vpn topology over a service provider network. In: 2013 9th International Conference on the Design of Reliable Communication Networks (DRCN), pp. 71–78. IEEE (2013)
Chatterjee, B., Oki, E.: Elastic Optical Networks: Fundamentals. Control, and Management. CRC Press, Design (2020)
Davalos, E.J., Baran, B.: A survey on algorithmic aspects of virtual optical network embedding for cloud networks. IEEE 6, 20893–20906 (2018)
Dias, L., et al.: Channel-based rsa approach for virtualization and qos-aware protection in optical networks. In: IEEE International Conference on Communications (ICC 2021), pp. 1–6. IEEE (2021)
Hai, D.T.: On the spectrum-efficiency of qos-aware protection in elastic optical networks. Optik 202, 163563 (2020)
Hammad, A., et al.: Novel approaches for composition of online virtual optical networks utilizing o-ofdm technology. In: 39th European Conference and Exhibition on Optical Communication (ECOC 2013), pp. 1–3. IET (2013)
Jinno, M., et al.: Spectrum-efficient and scalable elastic optical path network: Architecture, benefits, and enabling technologies. IEEE Commun. Mag. 47(11), 66–73 (2009)
Kingma, D.P., Ba, J.: Adam: A method for stochastic optimization. arXiv preprint arXiv:1412.6980 (2014)
Klinkowski, M.: A genetic algorithm for solving rsa problem in elastic optical networks with dedicated path protection. In: International joint conference CISIS’12-ICEUTE ‘12-SOCO’ 12 Special Sessions, pp. 167–176. Springer (2013)
Klinkowski, M., Walkowiak, K.: Routing and spectrum assignment in spectrum sliced elastic optical path network. IEEE Commun. Lett. 15(8), 884–886 (2011)
Moura, T., Vieira, R., Almeida, R., Giozza, W., Rodrigues, J., Assis, K.D.R.: Planejamento de redes Ópticas elásticas por meio da solução do max-rmsa. In: Anais do XXXVIII Simpósio Brasileiro de Redes de Computadores e Sistemas Distribuídos, pp. 868–881. SBC (2020)
Pióro, M., Medhi, D.: Routing, flow, and capacity design in communication and computer networks. Elsevier (2004)
Renjith, P., Sujatha, G., Vinoth, M., Vignesh, G., Prabhu, M.R., Mouleswararao, B.: Deep reinforcement learning for comprehensive route optimization in elastic optical networks using generative strategies. Opt. Quant. Electron. 55(13), 1197 (2023)
Roeder, L.: Netron - a viewer for neural network, deep learning and machine learning models (2017). https://github.com/lutzroeder/netron
Shahriar, N.: Survivable virtual network embedding in transport networks (2020). http://hdl.handle.net/10012/16085
Sone, Y., et al.: Bandwidth squeezed restoration in spectrum-sliced elastic optical path networks (slice). IEEE/OSA J. Opt. Commun. Netw. 3(3), 223–233 (2011)
Velasco, L., et al.: Modeling the routing and spectrum allocation problem for flexgrid optical networks. Photon Netw. Commun. 24(3), 177–186 (2012)
Wang, L.: Elastic optical network routing analysis using intelligent machine learning architecture with security analysis. Opt. Quant. Electron. 55(14), 1238 (2023)
Wang, Y., Cao, X., Pan, Y.: A study of the routing and spectrum allocation in spectrum-sliced elastic optical path networks. In: IEEE International Conference on Computer Communications (INFOCOM), pp. 1503–1511 (2011)
Zhang, Y., Xin, J., Li, X., Huang, S.: Overview on routing and resource allocation based machine learning in optical networks. Opt. Fiber Technol. 60, 102355 (2020)
Zhang, L., Li, X., Tang, Y., Xin, J., Huang, S.: A survey on qot prediction using machine learning in optical networks. Opt. Fiber Technol. 68, 102804 (2022)
Acknowledgements
This study was financed in part by the Coordenação de Aperfeiçoamento de Pessoal de Nível Superior – Brasil (CAPES) – Finance Code 001. The authors thank to CNPq for scholarships and grants, and to UFBA for its educational support.
Funding
The authors have not disclosed any funding.
Author information
Authors and Affiliations
Corresponding author
Additional information
Publisher's Note
Springer Nature remains neutral with regard to jurisdictional claims in published maps and institutional affiliations.
Rights and permissions
Springer Nature or its licensor (e.g. a society or other partner) holds exclusive rights to this article under a publishing agreement with the author(s) or other rightsholder(s); author self-archiving of the accepted manuscript version of this article is solely governed by the terms of such publishing agreement and applicable law.
About this article
Cite this article
de Melo, T.A.C., dos Santos, A.F., de Andrade Almeida, R.C. et al. Capacity, resilience and virtual embedding in elastic optical networks planning with adopted machine learning. Opt Quant Electron 56, 1075 (2024). https://doi.org/10.1007/s11082-024-07016-z
Received:
Accepted:
Published:
DOI: https://doi.org/10.1007/s11082-024-07016-z