Abstract
This article studies the problem of fractional exponential stability and passivity analysis for Hopfield conformable fractional-order neural networks (CFONNs) subject to uncertainties. First, we derive some less conservative conditions to ensure the fractional exponential stability of Hopfield CFONNs by using the Lyapunov functional method combined with the linear matrix inequality (LMI) approach. Then, by introducing a new definition of passivity analysis for Hopfield CFONNs, an LMI condition is proposed to ensure the passivity analysis of the considered system. Numerical examples are carried out to verify the correctness of the obtained results.















Similar content being viewed by others
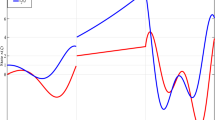
Data Availability Statement
All data generated or analyzed during this study are included in this article.
References
Boroomand A, Menhaj MB (2009) Fractional-order Hopfield neural networks, In: advances in neuro-information processing ICONIP 2008. Lecture Notes in Computer Science 5506: 883–890
Zhang S, Yu Y, Yu J (2017) LMI conditions for g lobal stability of fractional-order neural networks. IEEE Trans Neural Netw Learn Syst 28(10):2423–2433
Zhang S, Yu Y, Geng L (2017) Stability analysis of fractional-order Hopfield neural networks with time-varying external inputs. Neural Process Lett 45(1):223–241
Yang Y, He Y, Wang Y, Wu M (2018) Stability analysis of fractional-order neural networks: an LMI approach. Neurocomputing 285:82–93
Khalil R, Al Horani M, Yousef A, Sababheh M (2014) A new definition of fractional derivative. J Comput Appl Math 264:65–70
Abdeljawad T (2015) On conformable fractional calculus. J Comput Appl Math 279:57–66
Yang S, Wang L, Zhang S (2018) Conformable derivative: application to non-Darcian flow in lowpermeability porous media. Appl Math Lett 79:105–110
Thuan MV, Thu NTH, Sau NH, Huyen NTT (2021) New results on \(H_{\infty }\) control for nonlinear conformable fractional order systems. J Syst Sci Complex 34(1):140–156
Naifar O, Jmal A, Makhlouf AB (2021) Non-fragile \(H_{\infty }\) observer for Lipschitz conformable fractional-order systems. Asian J Cont. https://doi.org/10.1002/asjc.2626
Kütahyalioglu A, Karakoç F (2021) Exponential stability of Hopfield neural networks with conformable fractional derivative. Neurocomputing 456:263–267
Vidhya C, Dharani S, Balasubramaniam P (2020) Stability of impulsive stochastic reaction diffusion recurrent neural network. Neural Process Lett 51:1049–1060
Gunasekaran N, Syed Ali M (2021) Design of stochastic passivity and passification for delayed BAM neural networks with Markov jump parameters via non-uniform sampled-data control. Neural Proces Lett 53:391–404
Rajchakit G, Sriraman R (2021) Robust passivity and stability analysis of uncertain complex-valued impulsive neural networks with time-varying delays. Neural Proces Lett 53:581–606
Ding Z, Zeng Z, Zhang H, Wang L, Wang L (2019) New results on passivity of fractional-order uncertain neural networks. Neurocomputing 351:51–59
Thuan MV, Huong DC, Hong DT (2019) New results on robust finite-time passivity for fractional-order neural networks with uncertainties. Neural Proces Lett 50:1065–1078
Rajchakit G, Chanthorn P, Niezabitowski M, Raja R, Baleanu D, Pratap A (2020) Impulsive effects on stability and passivity analysis of memristor-based fractional-order competitive neural networks. Neurocomputing 417:290–301
Sau NH, Thuan MV, Huyen NTT (2020) Passivity analysis of fractional-order neural networks with time-varying delay based on LMI approach. Circuits Syst Signal Process 39:5906–5925
Song Q, Chen S, Zhao Z, Liu Y, Alsaadi FE (2021) Passive filter design for fractional-order quaternion-valued neural networks with neutral delays and external disturbance. Neural Netw 137:18–30
Padmaja N, Balasubramaniam P (2021) New delay and order-dependent passivity criteria for impulsive fractional-order neural networks with switching parameters and proportional delays. Neurocomputing 454:113–123
Liu CG, Wang JL (2021) Passivity of fractional-order coupled neural networks with multiple state/ derivative couplings. Neurocomputing 455:379–389
Huang H, Feng G, Cao J (2008) Robust state estimation for uncertain neural networks with time-varying delay. IEEE Transac Neural Netw 19(8):1329–1339
Chen B, Li H, Lin C, Zhou Q (2009) Passivity analysis for uncertain neural networks with discrete and distributed time-varying delays. Phys Lett A 373(14):1242–1248
Song Q, Cao J (2012) Passivity of uncertain neural networks with both leakage delay and time-varying delay. Nonlinear Dyn 67(2):1695–1707
Park MJ, Kwon OM, Ryu JH (2018) Passivity and stability analysis of neural networks with time-varying delays via extended free-weighting matrices integral inequality. Neural Netw 106:67–78
Yang B, Wang J, Hao M, Zeng H (2018) Further results on passivity analysis for uncertain neural networks with discrete and distributed delays. Inf Sci 430:77–86
Ge C, Park JH, Hua C, Shi C (2019) Robust passivity analysis for uncertain neural networks with discrete and distributed time-varying delays. Neurocomputing 364:330–337
Souahia A, Makhlouf AB, Ali Hammami M (2017) Stability analysis of conformable fractional-order nonlinear systems. Indag Math 28:1265–1274
Zeng HB, He Y, Wu M, Xiao SP (2011) Passivity analysis for neural networks with a time-varying delay. Neurocomputing 74(5):730–734
Thuan MV, Trinh H, Hien LV (2016) New inequality-based approach to passivity analysis of neural networks with interval time-varying delay. Neurocomputing 194:301–307
Peng X, Wu H (2018) Robust Mittag-Leffler synchronization for uncertain fractional-order discontinuous neural networks via non-fragile control strategy. Neural Process Lett 48(3):1521–1542
Huang W, Song Q, Zhao Z, Liu Y, Alsaadi FE (2021) Robust stability for a class of fractional-order complex-valued projective neural networks with neutral-type delays and uncertain parameters. Neurocomputing 450:399–410
He Y, Wang QG, Zheng WX (2005) Global robust stability for delayed neural networks with polytopic type uncertainties. Chaos Solitons Fractals 26(5):1349–1354
Gahinet P, Nemirovskii A, Laub AJ, Chilali M (1995) LMI control toolbox-for use with MATLAB. The MathWorks Inc
Kaslik E, Sivasundaram S (2012) Nonlinear dynamics and chaos in fractional-order neural networks. Neural Netw 32:245–256
Acknowledgements
The authors sincerely thank the Associate Editor and anonymous reviewers for their constructive comments that helped improve the quality and presentation of this paper. The authors also thank Dr. N.T. Thanh for helpful comments and discussion in improving Sect.4. The research of Mai Viet Thuan is funded by the Ministry of Education and Training of Vietnam under grant number B2022-MDA-02
Author information
Authors and Affiliations
Corresponding author
Additional information
Publisher's Note
Springer Nature remains neutral with regard to jurisdictional claims in published maps and institutional affiliations.
Rights and permissions
About this article
Cite this article
Huyen, N.T.T., Sau, N.H. & Thuan, M.V. LMI Conditions for Fractional Exponential Stability and Passivity Analysis of Uncertain Hopfield Conformable Fractional-Order Neural Networks. Neural Process Lett 54, 1333–1350 (2022). https://doi.org/10.1007/s11063-021-10683-8
Accepted:
Published:
Issue Date:
DOI: https://doi.org/10.1007/s11063-021-10683-8