Abstract
Maximum Difference Scaling (MaxDiff) is an essential method in marketing concerning forecasting consumer purchase decisions and general product demand. However, the usefulness of traditional MaxDiff studies suffers from two limitations. First, it measures relative preferences, which prevents predicting how many consumers would actually buy a product and impedes comparing results across respondents. Second, market researchers apply MaxDiff in hypothetical settings that might not reveal valid preferences due to hypothetical bias. The first limitation has been addressed by implementing anchored MaxDiff variants. In contrast, the latter limitation has only been targeted in other preference measurement procedures such as conjoint analysis by applying incentive alignment. By integrating anchored MaxDiff (i.e., direct vs. indirect anchoring) with incentive alignment (present vs. absent) in a 2 × 2 between-subjects preregistered online experiment (n = 448), the current study is the first to address both threats. The results show that incentive-aligning MaxDiff increases the predictive validity regarding consequential product choices—importantly—independently of the anchoring method. In contrast, hypothetical MaxDiff variants overestimate general product demand. The article concludes by showcasing how the managerial implications drawn from anchored MaxDiff differ depending on the four tested variants. In addition, we provide the first incentive-aligned MaxDiff benchmark dataset in the field.
Similar content being viewed by others
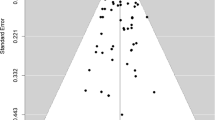
Avoid common mistakes on your manuscript.
1 Introduction
Companies continuously try to introduce successful products that fit consumers’ needs. Maximum Difference Scaling (hereafter MaxDiff, see Louviere et al., 2013) is a preference elicitation technique often applied in market research to assess consumers’ needs and design future products accordingly. Study participants hereby answer multiple MaxDiff tasks (typically comprising three or four alternatives) and indicate the best and the worst alternatives (Louviere et al., 2013). Typical MaxDiff use cases are testing different product flavors (e.g., Chrzan & Orme, 2019, p. 4), prioritizing product attributes (e.g., Rausch et al., 2021), or testing advertising claims (e.g., Chapman & Rodden, 2023, p. 195).
MaxDiff was initially introduced as an alternative to ranking and rating scales (Finn & Louviere, 1992) to overcome issues such as that participants are not trading off between items when answering rating batteries or the cognitive burdens of ranking a high number of items (Louviere et al., 2013). Nowadays, however, MaxDiff is also mentioned in the same breath as conjoint analysis, which is often applied in business forecasting. In contrast to conjoint methods such as choice-based conjoint (hereafter CBC), MaxDiff is not used to predict the success of holistic product concepts, including product prices in market simulations. Instead, product attribute levels or consumer needs (e.g., toppings on a pizza, see Chapman & Rodden, 2023) constitute the alternatives.
In the literature, MaxDiff is also known as best-worst scaling (hereafter BWS) case 1. Besides BWS case 1 (MaxDiff), there are two more BWS cases, namely case 2 (profile case) and case 3 (multi-profile case). In case 2, participants choose the best and worst attribute level from a profile that consists of multiple attributes (e.g., Flynn & Marley, 2014, p. 183). Case 3 resembles the traditional CBC; however, participants must also choose the worst alternative besides the best alternative (e.g., Mühlbacher et al., 2016). The present research focuses exclusively on case 1 (i.e., MaxDiff), which has gained importance in market research practice in recent years (Sawtooth Software Inc. 2022b). However, this trend is not yet reflected in academic marketing research.Footnote 1
One of the present article’s two goals is to initiate rethinking the common MaxDiff practices toward using holistic product concepts with corresponding prices as alternatives in MaxDiff (see Fig. 1A, which illustrates the MaxDiff tasks of the reported study). The stimuli in a MaxDiff are list items (Flynn & Marley, 2014, p. 181) consisting of only one holistic product/characteristic (e.g., different soft drink flavors). In each task, participants see different items and choose both the best and the worst-liked alternative (Louviere et al., 2013), therefore providing more information per task than in, for example, CBC. Importantly, MaxDiff does not permute combinations of levels of different attributes across these items, as is usually seen in conjoint studies (Flynn & Marley, 2014, p. 181). This has two advantages compared to CBC: First, it allows participants to evaluate all products of interest in a MaxDiff since a complete design (vs. fractional design) can be implemented. Second, the participants do not have to assess unrealistic product combinations (e.g., cheap products at premium prices).
MaxDiff’s ultimate purpose is forecasting future purchases, making predictive validity a central tenet. Therefore, researchers constantly seek ways to improve the predictions drawn from MaxDiff studies (e.g., Chrzan & Peitz, 2019; Lagerkvist et al., 2012) since reducing prediction errors lowers the company’s cost (Hauser et al., 2014).
Two drawbacks limit the usefulness of the traditional MaxDiff studies, and the second goal of the present article is to evaluate solutions for these issues. First, traditional MaxDiff studies measure relative instead of absolute preferences because participants cannot indicate that none of the items presented is an actual purchase option (Louviere et al., 2013). This is acceptable if managers are only interested in ranking the item list. However, if they want to know whether, for example, products are actually considered as purchase option, traditional MaxDiff is not applicable for this type of question (Chrzan & Orme, 2019, p. 86). Moreover, if the goal is to estimate purchase likelihood or to simulate a realistic market situation, not including a no-buy alternative lacks realism (Lichters et al., 2015) and ultimately hurts predictive validity. Second, researchers and practitioners have conducted MaxDiff studies exclusively in hypothetical settings where participants might be less motivated to reveal their true preferences since their choices do not bear economic consequences (Ding et al., 2005).
To address the first issue, researchers developed, among others, the direct anchored (Lattery, 2010; Orme, 2009b) and the indirect anchored (Orme, 2009a; developed by Jordan Louviere) MaxDiff. Both anchoring approaches enable estimating an anchor in the utility space of list items, which brings the results of a MaxDiff to an absolute scale. This anchor can serve as a consumer’s no-buy threshold when the MaxDiff and anchor questions are adequately framed (see Fig. 1B and C). To overcome the second issue, researchers introduced incentive alignment to preference elicitation methods other than MaxDiff. Extant studies in this field have mainly focused on CBC studies and have proved incentive alignment’s effectiveness in increasing predictive validity for consequential product-choice tasks (e.g., Ding et al., 2005). Although the implementation of anchored MaxDiff enables the estimation of the no-buy utility (i.e., the outside good’s utility) and, therefore, also makes consequential product choices a valid alternative in MaxDiff studies, an equivalent proposal for an incentive-aligned (anchored) MaxDiff study is still lacking (see Table A1 in the Web Appendix, hereafter WA).
The present research addresses this gap and guides market researchers in deciding whether applying incentive alignment when conducting MaxDiff is worth considering and whether they should prefer a specific anchoring approach. Such an endeavor is necessary for multiple reasons. On the one hand, one can argue that in MaxDiff, any measures that seek to enhance participant motivation (i.e., incentive alignment) might have only a limited effect since MaxDiff tasks (compared to CBC tasks) are relatively simple per se (e.g., Lagerkvist et al., 2012), which could potentially weaken the incentive alignment’s overall effect. On the other hand, although anchored MaxDiff is already applied in commercial software (i.e., Sawtooth Software; Lattery, 2010; Orme, 2009a), research on this topic is lacking in the top marketing journals. In the academic literature, traditional (unanchored) MaxDiff still dominates, which, as discussed above, does not enable the extraction of much information relevant to marketing questions and realistic market simulations. This paper, therefore, aims to increase awareness of the method’s advancements. Finally, this paper is the first to provide MaxDiff datasets with consequential product choices to evaluate predictive validity (the Open Science Framework, hereafter OSF, provides the complete data and R analysis scripts: https://osf.io/5h4rk/). This unique data can be the basis for researchers to evaluate further questions, for example, the ability of different modeling approaches to foster predictive validity.
Our results highlight that incentive-aligned MaxDiff bears superior predictive validity, while hypothetical MaxDiff studies overestimate the general product demand, which may have devastating downstream consequences for companies. With our research, we aim to initiate a rethink in market research toward a stronger emphasis on incentive-aligned preference measurement techniques. In particular, incentive-aligned anchored MaxDiff variants (when framed as product decisions) might constitute a fruitful alternative to more complex CBC studies.
2 Conceptual background
2.1 Anchored MaxDiff
In MaxDiff studies, participants indicate the best and worst alternatives in multiple MaxDiff tasks (Fig. 1A presents a MaxDiff task from our study; Finn & Louviere, 1992). This helps establish a ranking among all items under research in participants’ utility space, a relative preference measure (Lagerkvist et al., 2012). Thus, the resulting individual-level utilities can neither be compared across participants (Lagerkvist et al., 2012) nor be used to predict choice shares in markets that include a no-buy alternative. To measure absolute preferences instead, researchers developed anchored MaxDiff. Two approaches are commonly referred to in the literature: the direct anchored (Lattery, 2010; Orme, 2009b) and the indirect anchored (Orme, 2009a) MaxDiff. What is lacking thus far is a rigorous assessment of the predictive validity of the two approaches, which would help researchers choose between them. We posit that the two approaches benefit market researchers most when the MaxDiff tasks are framed as purchase likelihood questions and when holistic products, including prices, are to be evaluated (see above). In this case, adhering to the direct approach, participants first answer all MaxDiff tasks, followed by an additional task indicating whether each product (or a subset) represents a purchase option (Fig. 1B). In contrast, in the indirect anchored MaxDiff (Fig. 1C), also known as the dual-response approach, participants answer whether all, none, or some of the items shown in each MaxDiff task represent a purchase option (Lagerkvist et al., 2012).
2.2 Incentive alignment
Preference measurement techniques usually involve hypothetical decisions and do not consider that participants in such settings tend to overestimate their purchase likelihood and show less price sensitivity (e.g., Miller et al., 2011). To address this adequately, researchers in the domain of conjoint analysis have utilized incentive alignment (e.g., Ding et al., 2005; Sablotny-Wackershauser et al., 2024). By making each product choice in the survey potentially payoff-relevant, participants are sufficiently motivated to reveal their true preferences (Dong et al., 2010).
In the CBC domain, researchers have introduced several mechanisms to incentive-align studies (for an overview, see Dong et al., 2010). Most frequently, participants receive one of their randomly drawn choice task decisions as a reward and pay the corresponding product price (Ding et al., 2005). This is possible because, in CBC, participants select the product with the highest purchase likelihood in each choice task, whereas, alternatively, they always have the option to indicate that none of the shown products is worth buying (i.e., the no-buy alternative).
An equivalent mechanism for traditional MaxDiff would not have been feasible since participants cannot indicate that they do not want to receive a product they have marked as the best alternative for study disbursement. Here, it becomes clear that introducing anchored MaxDiff and reframing MaxDiff tasks as purchase likelihood questions about holistic products have paved the way for implementing incentive-aligned MaxDiff variants to increase predictive validity. This advancement ultimately enables a rigorous assessment of both described anchoring approaches with regard to incentive-aligned versions of anchored MaxDiff and consequential product choices as validation procedure.
2.3 Research goals
Prior research suggests that the difference between hypothetical and incentive-aligned preference measurement methods and the difference between direct and indirect anchored MaxDiff lead to diverging forecasts of product choice as well as demand in market simulations. The question for market researchers remains: Which combination of the two principles provides the most realistic predictions? This study sets out to give an answer; first, by introducing incentive alignment to anchored MaxDiff and, second, by using a consequential validation procedure that allows assessing the relative merits of incentive alignment and anchoring in MaxDiff studies on product choices.
3 Empirical study
3.1 Method and material
In a preregistered online experiment, 16 Sony PlayStation 5 (hereafter PS5) video games served as the focal products (see WA B for stimuli), as video games fulfill the precondition of being holistic products (with fixed prices).Footnote 2 Moreover, Sony also offers PS5 bundles. Determining the best games for bundles is essential and makes MaxDiff a valid and interesting method for these research questions.
We chose the 16 PS5 games based on sales in Germany (e.g., GamesWirtschaft) and download numbers in the PS5 store for 2021. Furthermore, we included games released in 2022 (e.g., Gran Turismo) and genres that were underrepresented in our sample (e.g., simulation games such as Overcooked!). We determined prices based on market prices minus 5% to offer attractive products within the study.
We randomly allocated participants to one of four MaxDiff conditions in a 2 (incentive-aligned: yes vs. no) × 2 (type of anchoring: direct vs. indirect) between-subjects design. Each MaxDiff variant comprised 16 tasks with four alternatives, and each participant saw each video game four times. In each MaxDiff task, participants indicated which video game they were most likely to purchase and which they were least likely to purchase (see Fig. 1A). We implemented both anchoring approaches in the same way as described in Section 2.1.
To assess predictive validity, each participant responded to the same four consequential validation tasks (see WA B; excluded from utilities’ estimation). The first two tasks offered 7 and 11 games, respectively, plus a no-buy alternative. The third validation task was a dual-response choice (a forced decision with subsequent no-buy question) offering eight games. Finally, the fourth task was an incentive-aligned ranking task comprising six games (Lusk et al., 2008), followed by asking up to which rank participants would opt for a buy or if they would buy none of the games.
We incorporated a payout mechanism as follows: Besides receiving a fixed payment of €3.50, each participant had a 1-in-40 chance of winning a PS5 game and cash (the difference between the video game’s price and €55). More precisely, participants in the incentive-aligned groups were instructed that a randomly drawn MaxDiff or validation task could become payoff-relevant if a participant was drawn as a winner. In the hypothetical conditions, a randomly drawn validation task served as study disbursement. To ensure an understanding of the payoff mechanism, participants needed to answer one of a maximum of three consecutive probing questions correctly.
Participants received their chosen game plus an amount of cash (see above) if validation task one, two, or three was randomly drawn. In the ranking task, the probability depended on the assigned rank. It was calculated following the formula \(\frac{J+1-r_{j}}{{\sum }_{j=1}^{J}j}\times 100\), where J represents the number of alternatives and rj represents the assigned rank of the alternative j (Lusk et al., 2008, p. 488).
3.2 Participants
An independent German market research institute helped with recruiting participants for the online experiment. All participants needed to fulfill the following criteria: (I) interest in both video games and the PS5, (II) at least 18 years old, and (III) playing video games at least occasionally. We also included participants who already own some of the games (regardless of the video console platform). We screened out 41 participants due to their response behavior (e.g., attention checks, see preregistration). In the net sample of n = 448 participants, (I) 118 replied to the direct anchored hypothetical, (II) 112 to direct anchored incentive-aligned, and 109 to the indirect anchored hypothetical or indirect anchored incentive-aligned MaxDiff (III and IV) respectively. The sample’s characteristics are 42% females, 57% males, and one diverse participant; Mage = 39.25, SDage = 14.25, and 67% with a monthly income above €1,000. No significant differences emerged between the groups.Footnote 3
3.3 Results
3.3.1 Predictive validity
We applied hierarchical Bayes multinomial logit analysis, making use of a single multivariate normal distribution (Allenby & Ginter, 1995) to estimate individual part-worth utilities (see Table C2 in WA) in Sawtooth Software Lighthouse Studio (Sawtooth Software Inc. 2022a).Footnote 4
In Sawtooth Software, the best and worst choices are stacked together for an estimation in a single run (Chrzan & Orme, 2019, p. 22). Therefore, the worst choice’s design matrix is negated (Chrzan & Orme, 2019, p. 22).
We applied the multinomial logit rule to predict product choice probabilities for the validation tasks. Based on these results, we calculated the hit rate (i.e., correctly predicted choices) and the mean hit probability (MHP, i.e., the predicted probability of the actual choice). We also applied a fourfold out-of-sample cross-validation within each MaxDiff condition for the first three validation tasks. In this cross-validation procedure, we calculated the difference between the actual and the predicted choice share (i.e., mean absolute error). Lastly, for the product ranking task (validation task 4), we calculated the mean rank of the predicted choice and the Spearman correlation between the assigned and predicted ranks.
Table 1 presents the main results (the OSF presents results for each validation task separately, as well as a comparison between unanchored and anchored MaxDiff for validation tasks 3 and 4). Each condition predicts better than chance for all validation tasks (binomial test p’s < 0.001). Incentive-aligned (vs. hypothetical) MaxDiff predicts participants’ product choices better, which is also true when examining out-of-sample prediction.
We ran a generalized logistic mixed-effects model to test for significant differences in the product choice tasks (validation tasks 1–3; Sablotny-Wackershauser et al., 2024). Correctly predicted product choice (0 = no hit, 1 = hit) served as the dependent variable, while incentive alignment (0 = hypothetical, 1 = incentive-aligned) and type of anchoring (0 = direct, 1 = indirect) served as predictors. To account for repeated measurement within subjects, we added random intercepts for both the validation tasks and the subjects. While the main effect of the anchoring method (β = 0.18, z = 1.12, p = 0.262) is not significant, the main effect of incentive alignment is (β = 0.88, z = 5.31, p < 0.001).Footnote 5
To test differences in the MHP, we ran a mixed-effects linear model (Sablotny-Wackershauser et al., 2024). First, we applied ranked-based inverse normal transformation to account for missing linearity in the MHP (Gelman et al., 2013, p. 97). Again, the anchoring method’s main effect (β = 0.06, t(445) = 0.86, p = 0.390) is insignificant, while incentive alignment’s effect is significantly positive (β = 0.33, t(445) = 5.00, p < 0.001).
Second, we ran an ordered logistic regression to analyze the ranking validation task. We find a significant main effect of incentive alignment on the predicted rank of the seven alternatives (no-buy alternative included; β = 0.40, z = 2.23, p = 0.025) but not of the anchoring method (β = 0.14, z = 0.80, p = 0.423). Furthermore, we investigated the rank-based inverse normal transformed rank correlation. A significant main effect of incentive alignment emerged (β = 0.20, t(445) = 2.13, p = 0.034), but no effect of the anchoring method emerged (β = 0.12, t(445) = 1.33, p = 0.185).
3.3.2 General product demand
We additionally evaluated how the two experimental factors influence predictions of general product demand and whether one of the MaxDiff variants leads to an overestimation/underestimation. When looking at the consideration set’s predicted size (see Figure C1 in WA), results highlight that both hypothetical (vs. incentive-aligned) as well as direct (vs. indirect) anchored MaxDiff increase the set size. To assess the accuracy of predicted demand, we examined how often each variant overestimated (predicted buy but observed no-buy) or underestimated (predicted no-buy but observed buy), respectively, in the product choices (i.e., validation tasks 1–3). Figure 2 presents the aggregated differences for both overestimation and underestimation. A generalized logistic mixed-effects model (1 = predicted buy but observed no-buy, 0 = others) shows a significant overestimation of the hypothetical conditions (vs. incentive-aligned, β = 1.19, z = 5.54, p < 0.001) but no differences in the anchoring method (β = 0.01, z = 0.06, p = 0.952). However, we find no differences when it comes to the underestimation of product demand (1 = predicted no-buy but observed buy, 0 = others) between incentive-aligned (vs. hypothetical) anchored MaxDiff variants, (β = 0.44, z = 0.43, p = 0.668). Again, no differences among the anchoring methods emerged (β = 0.95, z = 0.87, p = 0.385). We conclude that the incentive-aligned conditions predict demand quite accurately.
3.3.3 Direct vs. indirect anchoring approach
Finally, we examine the differences between the direct and the indirect anchoring approach in more detail. First, we compare the choice probabilities for all alternatives across the four conditions. To do so, we first transformed the raw individual utilities to choice probabilities for easier interpretation (Chrzan & Orme, 2019, p. 52; see Table B2 in the WA), making use of the following formula: \({P}_{j} = \frac{{exp}^{{u}_{j}}}{{(exp}^{{u}_{j}} + a-1)}\) (Chrzan & Orme, 2019, p. 59), which is one alternative for rescaling MaxDiff scores besides, for example, multinomial logit. Here, u is the raw logit score of product j, and a is the number of products shown per MaxDiff task (four in our case). Then, we normalized the scores to sum up to 100% and aggregated them within groups. Surprisingly, the choice probability of the no-buy alternative (anchor) is exceptionally high for the indirect anchored conditions (highest choice probability in the incentive-aligned condition and second highest in the hypothetical condition). For the directly anchored variants, however, the no-buy alternative has the fifth highest (incentive-aligned condition) and 13th highest probability (hypothetical condition). Thus, when reporting the estimated choice probabilities, the analyst might conclude that the tested products are not attractive to consumers when indirect anchoring is used.
4 Discussion
4.1 Summary of findings
This research evaluated whether incentive alignment in anchored MaxDiff can boost predictive validity. It is the first work that compares direct versus indirect anchored MaxDiff’s predictive validity regarding consequential product choices. A question that arose was whether one of the two approaches systematically overestimates/underestimates general product demand in market simulations.
We contribute to the literature on incentive-aligned preference measurement by adding a unique dataset with both incentive-aligned MaxDiff tasks and validation tasks. Our main findings are as follows: Incentive alignment effectively increases predictive validity in anchored MaxDiff studies regardless of the anchoring method (direct or indirect). Furthermore, independently of incentive alignment (or hypothetical), both anchoring methods provide a comparable level of predictive validity. Our results are also in line with findings in the field of incentive-aligned CBC studies (Ding et al., 2005), in that incentive-aligned (vs. hypothetical) MaxDiff is significantly more accurate in predicting purchase likelihood and can help better assess consumers’ product demand as well as the size of the consideration set.
Interestingly, there are quite substantial differences in choice probabilities for the no-buy alternative between the two anchoring approaches (higher for indirect vs. for direct). The following three explanations seem plausible: (1) the high share of the middle option in the indirect anchor questions (65.7% in the incentive condition, 71.9% in the hypothetical); (2) the number of games for which participants did not indicate whether it represents a purchase option, by neither choosing the game as a best nor worst alternative and choosing the middle option in the corresponding anchor question (4.07% incentive-aligned vs. 4.24% hypothetical); and (3) the share of participants who contradict themselves (state in one anchor question that a particular game is a purchase option and in another the opposite; only 28%, incentive-aligned, and 33%, hypothetical, respectively, of the participants did not state any contradictions).
4.2 Managerial implications
Companies usually conduct MaxDiff studies to rank items according to importance or purchase likelihood to forecast future market outcomes (Chrzan & Orme, 2019). Our research shows that not adopting incentive-aligned study designs deteriorates product assortment decisions due to poorer predictions. The following case study illustrates the managerial consequences of applying a suboptimal method: A retail chain wants to add PS5 games to its assortment. Owing to shelf-space capacity, they decided to add just three games. They chose to use a Total Unduplicated Reach & Frequency (TURF; a “product line extension model” Miaoulis et al., 1990, p. 29) analysis, a method that searches for the perfect product combination (i.e., the combination that reaches the largest share of consumers) out of the \(\left(\genfrac{}{}{0pt}{}{16}{3}\right) = 560\) possible combinations (Chrzan & Orme, 2019, p. 108). The retail chain implements the threshold approach in which a customer is considered reached when the retailer offers at least one product with a utility exceeding the predicted buy/no-buy threshold.
For each of the tested MaxDiff variants, the product assortments with the highest reach differ enormously in their composition (see Fig. 3): incentive-aligned direct anchoring (Call of Duty, KENA, GranTurismo; reach 88%), hypothetical direct anchoring (Guardians of the Galaxy, Uncharted, GranTurismo; reach 96%), incentive-aligned indirect anchoring (Call of Duty, Assassin’s Creed, GranTurismo, reach 81%), hypothetical indirect anchoring (Ratchet & Clank, Assassin’s Creed, GranTurismo, reach 89%).Footnote 6 Importantly, if the retail chain only has access to hypothetical MaxDiff data bearing inferior predictive validity, they will miss a chance to list the Call of Duty game.
From a managerial perspective, applied researchers should strive to select the anchored MaxDiff variant that provides the highest predictive validity, not for the sake of validity but to maximize return on market research investments due to better meeting consumers’ tastes.
5 Limitations and future directions
The first limitation is the implementation of Ding et al.’s (2005) mechanism of incentive alignment, for which all product alternatives under research must be available, a rather untypical scenario (Dong et al., 2010; Hofstetter et al., 2021). Future research should examine other mechanisms (e.g., the RankOrder mechanism; Dong et al., 2010) that do not require the availability of all products. Likewise, marketing research should generally focus on making incentive alignment more practical. Due to stricter data privacy regulations and additional research costs, incentive alignment as a service comes with additional implementation hurdles (Hofstetter et al., 2021).
Second, we used a compound lottery with a 1:40 chance of winning for study disbursement. Future studies might evaluate different winning probabilities and expected payoffs (Yang et al., 2018). Moreover, we only tested incentive-aligned anchored MaxDiff. Future studies could include further conditions, for example, incentive-aligned CBC and other related CBC methods.
Finally, we implemented Sawtooth Software’s best–worst coding (Chrzan & Orme, 2019, p. 20), which assumes independence of best and worst choices, a debatable assumption. Researchers are invited to evaluate the interplay of the tested anchored MaxDiff variants and the application of different coding schemes (e.g., Chrzan & Orme, 2019, Chapter 3).
Data Availability
We provide all datasets and analysis scripts in R within the Open Science Framework (OSF): https://osf.io/5h4rk/.
Notes
A literature review (Web of Science, articles published between 2000 and 2023, search terms (Mühlbacher et al., 2016): “Best-Worst-Scaling” OR “MaxDiff” OR “Maximum Difference Scaling”) of the top marketing journals and Sawtooth Software Conference papers is presented in Table A1 in the Web Appendix.
https://aspredicted.org/SLH_95Q; the preregistration also provides information on sample size planning and screening.
We tested for differences in gender, age, income, gaming behavior, video console ownership, or ownership of one of the games (smallest p = .065; Table C1 in WA provides demographics split by conditions).
We used 80,000 warm-up iterations and 40,000 draws for estimation, set prior degrees of freedom to 2, and prior variance to 1.3 (Orme and Williams 2016). We provide further information on the model and the corresponding estimation on OSF in a mathematical appendix.
In all models, we first tested for significant interaction effects. Owing to insignificance, we only report main effects (R scripts provide detailed results). To assess the robustness of results, we removed participants with extreme response behavior (see OSF).
If two assortments had the same reach, we picked the one with the higher frequency score.
References
Allenby, G. M., & Ginter, J. L. (1995). Using extremes to design products and segment markets. Journal of Marketing Research, 32, 392–403. https://doi.org/10.1177/002224379503200402
Chapman, C., & Rodden, K. (2023). MaxDiff: Prioritizing features and user needs. In C. Chapman & K. Rodden (Eds.), Quantitative user experience research: Informing product decisions by understanding users at scale (pp. 185–234). Springer Science+Business Media LLC.
Chrzan, K., & Orme, B. K. (2019). Applied MaxDiff: A practitioner’s guide to best-worst scaling. Sawtooth Software.
Chrzan, K., & Peitz, M. (2019). Best-worst scaling with many items. Journal of Choice Modelling, 30, 61–72. https://doi.org/10.1016/j.jocm.2019.01.002
Ding, M., Grewal, R., & Liechty, J. (2005). Incentive-aligned conjoint analysis. Journal of Marketing Research, 42, 67–82. https://doi.org/10.1509/jmkr.42.1.67.56890
Dong, S., Ding, M., & Huber, J. (2010). A simple mechanism to incentive-align conjoint experiments. International Journal of Research in Marketing, 27, 25–32. https://doi.org/10.1016/j.ijresmar.2009.09.004
Finn, A., & Louviere, J. J. (1992). Determining the appropriate response to evidence of public concern: The case of food safety. Journal of Public Policy & Marketing, 11, 12–25. https://doi.org/10.1177/074391569201100202
Flynn, T. N., & Marley, A. (2014). Best-worst scaling: Theory and methods. In S. Hess & A. Daly (Eds.), Handbook of choice modelling (pp. 178–201). Edward Elgar Publishing.
Gelman, A., Carlin, J. B., Stern, H. S., Dunson, D. B., Vehtari, A., & Rubin, D. B. (2013). Bayesian data analysis (3rd ed.). CRC Press.
Hauser, J. R., Dong, S., & Ding, M. (2014). Self-reflection and articulated consumer preferences. Journal of Product Innovation Management, 31, 17–32. https://doi.org/10.1111/jpim.12077
Hofstetter, R., Miller, K. M., Krohmer, H., & Zhang, Z. J. (2021). A de-biased direct question approach to measuring consumers’ willingness to pay. International Journal of Research in Marketing, 38, 70–84. https://doi.org/10.1016/j.ijresmar.2020.04.006
Lagerkvist, C. J., Okello, J., & Karanja, N. (2012). Anchored vs. relative best–worst scaling and latent class vs. hierarchical Bayesian analysis of best–worst choice data: Investigating the importance of food quality attributes in a developing country. Food Quality and Preference, 25, 29–40. https://doi.org/10.1016/j.foodqual.2012.01.002
Lattery, K. (2010). Anchoring maximum difference scaling against a threshold – Dual response and direct binary responses. In Sawtooth Software (Ed.), Sawtooth software conference (pp. 91–106)
Lichters, M., Sarstedt, M., & Vogt, B. (2015). On the practical relevance of the attraction effect: A cautionary note and guidelines for context effect experiments. AMS Review, 5, 1–19. https://doi.org/10.1007/s13162-015-0066-8
Louviere, J. J., Lings, I., Islam, T., Gudergan, S., & Flynn, T. N. (2013). An introduction to the application of (case 1) best–worst scaling in marketing research. International Journal of Research in Marketing, 30, 292–303. https://doi.org/10.1016/j.ijresmar.2012.10.002
Lusk, J. L., Fields, D., & Prevatt, W. (2008). An incentive compatible conjoint ranking mechanism. American Journal of Agricultural Economics, 90, 487–498. https://doi.org/10.1111/j.1467-8276.2007.01119.x
Miaoulis, G., Parsons, H., & Free, V. (1990). Turf: A new planning approach for product line extensions. Marketing Research, 2(1), 28–40.
Miller, K. M., Hofstetter, R., Krohmer, H., & Zhang, Z. J. (2011). How should consumers’ willingness to pay be measured? An empirical comparison of state-of-the-art approaches. Journal of Marketing Research, 48, 172–184. https://doi.org/10.1509/jmkr.48.1.172
Mühlbacher, A. C., Kaczynski, A., Zweifel, P., & Johnson, F. R. (2016). Experimental measurement of preferences in health and healthcare using best-worst scaling: An overview. Health Economics Review, 6, 2. https://doi.org/10.1186/s13561-015-0079-x
Orme, B. K., & Williams, W. (2016). What are the optimal HB priors settings got CBC and MaxDiff studies? Sawtooth Software, Inc. https://sawtoothsoftware.com/resources/technical-papers/what-are-the-optimal-hb-priorssettings-for-cbc-and-maxdiff-studies. Accessed 2 Jan 2024
Orme, B. K. (2009a). Anchored scaling in MaxDiff using dual response. Sawtooth Software, Inc. https://sawtoothsoftware.com/resources/technical-papers/anchored-scaling-in-maxdiff-using-deal-response. Accessed 2 Jan 2024
Orme, B. K. (2009b). Using Calibration Questions to Obtain Absolute Scaling in MaxDiff. https://sawtoothsoftware.com/resources/technical-papers/using-calibration-questions-to-obtain-absolute-scaling-inmaxdiff. Accessed 2 Jan 2024
Rausch, T. M., Baier, D., & Wening, S. (2021). Does sustainability really matter to consumers? Assessing the importance of online shop and apparel product attributes. Journal of Retailing and Consumer Services, 63, 102681. https://doi.org/10.1016/j.jretconser.2021.102681
Sablotny-Wackershauser, V., Lichters, M., Guhl, D., Bengart, P., & Vogt, B. (2024). Crossing incentive alignment and adaptive designs in choice-based conjoint: A fruitful endeavor. Journal of the Academy of Marketing Science, 1–24. https://doi.org/10.1007/s11747-023-00997-5
Sawtooth Software Inc. (2022a). Lighthouse studio 9. Sawtooth Software Inc.
Sawtooth Software Inc. (2022). Report on conjoint analysis usage among sawtooth software customers. Sawtooth Software, Inc. https://content.sawtoothsoftware.com/assets/6701379a-e25b-44bd-bb60-b34ddb02cded?download. Accessed 2 Jan 2024
Yang, L. C., Toubia, O., & de Jong, M. G. (2018). Attention, information processing, and choice in incentive-aligned choice experiments. Journal of Marketing Research, 55, 783–800. https://doi.org/10.1177/0022243718817004
Funding
Open Access funding enabled and organized by Projekt DEAL. Both authors received funding from the Chemnitz University of Technology (Germany) for the submitted work. Author 2 received additional funding from the Otto von Guericke University Magdeburg (Germany). Author 1 receives an academic grant from Sawtooth Software, Inc. (USA).
Author information
Authors and Affiliations
Contributions
Conceptualization: Joshua Benjamin Schramm, Marcel Lichters; methodology: Joshua Benjamin Schramm, Marcel Lichters; formal analysis and investigation: Joshua Benjamin Schramm, Marcel Lichters; writing—original draft preparation: Joshua Benjamin Schramm; writing—review and editing: Joshua Benjamin Schramm, Marcel Lichters; funding acquisition: Joshua Benjamin Schramm, Marcel Lichters; resources: Joshua Benjamin Schramm, Marcel Lichters; supervision: Marcel Lichters.
Corresponding author
Ethics declarations
Ethics approval
The studies were approved by the ethics board of Chemnitz University of Technology (case number 101540674). Study participants provided informed written consent before enrolling.
Competing interests
The authors declare no competing interests.
Additional information
Publisher's Note
Springer Nature remains neutral with regard to jurisdictional claims in published maps and institutional affiliations.
Supplementary Information
Below is the link to the electronic supplementary material.
Rights and permissions
Open Access This article is licensed under a Creative Commons Attribution 4.0 International License, which permits use, sharing, adaptation, distribution and reproduction in any medium or format, as long as you give appropriate credit to the original author(s) and the source, provide a link to the Creative Commons licence, and indicate if changes were made. The images or other third party material in this article are included in the article's Creative Commons licence, unless indicated otherwise in a credit line to the material. If material is not included in the article's Creative Commons licence and your intended use is not permitted by statutory regulation or exceeds the permitted use, you will need to obtain permission directly from the copyright holder. To view a copy of this licence, visit http://creativecommons.org/licenses/by/4.0/.
About this article
Cite this article
Schramm, J.B., Lichters, M. Incentive alignment in anchored MaxDiff yields superior predictive validity. Mark Lett (2024). https://doi.org/10.1007/s11002-023-09714-2
Accepted:
Published:
DOI: https://doi.org/10.1007/s11002-023-09714-2