Abstract
We introduce a novel geometric approach to the image labeling problem. Abstracting from specific labeling applications, a general objective function is defined on a manifold of stochastic matrices, whose elements assign prior data that are given in any metric space, to observed image measurements. The corresponding Riemannian gradient flow entails a set of replicator equations, one for each data point, that are spatially coupled by geometric averaging on the manifold. Starting from uniform assignments at the barycenter as natural initialization, the flow terminates at some global maximum, each of which corresponds to an image labeling that uniquely assigns the prior data. Our geometric variational approach constitutes a smooth non-convex inner approximation of the general image labeling problem, implemented with sparse interior-point numerics in terms of parallel multiplicative updates that converge efficiently.





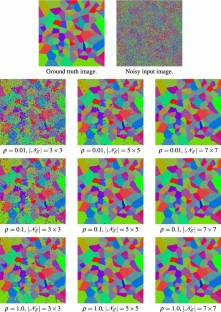








Similar content being viewed by others
Notes
For locations i close to the boundary of the image domain where patch supports \({\mathscr {N}}_{p}(i)\) shrink, the definition of the vector \(w^{p}\) has to be adapted accordingly.
References
Amari, S.I., Nagaoka, H.: Methods of Information Geometry. American Mathematical Society, Oxford University Press, Oxford (2000)
Aujol, J.F., Gilboa, G., Chan, T., Osher, S.: Structure-texture image decomposition-modeling, algorithms, and parameter selection. Int. J. Comput. Vis. 67(1), 111–136 (2006)
Ball, K.: An elementary introduction to modern convex geometry. In: Levy, S. (ed.) Flavors of Geometry, MSRI Publ., vol. 31, pp. 1–58. Cambridge University Press (1997)
Bayer, D., Lagarias, J.: The nonlinear geometry of linear programming. I. Affine and projective scaling trajectories. Trans. Am. Math. Soc. 314(2), 499–526 (1989)
Bayer, D., Lagarias, J.: The nonlinear geometry of linear programming. II. Legendre transform coordinates and central trajectories. Trans. Am. Math. Soc. 314(2), 527–581 (1989)
Bishop, C.: Pattern Recognition and Machine Learning. Springer, Berlin (2006)
Bomze, I., Budinich, M., Pelillo, M., Rossi, C.: Annealed replication: a new heuristic for the maximum clique problem. Discr. Appl. Math. 121, 27–49 (2002)
Bomze, I.M.: Regularity versus degeneracy in dynamics, games, and optimization: a unified approach to different aspects. SIAM Rev. 44(3), 394–414 (2002)
Buades, A., Coll, B., Morel, J.: A review of image denoising algorithms, with a new one. SIAM Multiscale Model. Simul. 4(2), 490–530 (2005)
Buades, A., Coll, B., Morel, J.M.: Neighborhood filters and PDEs. Numer. Math. 105, 1–34 (2006)
Cabrales, A., Sobel, J.: On the limit points of discrete selection dynamics. J. Econ. Theory 57, 407–419 (1992)
Čencov, N.: Statistical Decision Rules and Optimal Inference. American Mathematical Society, Providence (1982)
Chambolle, A., Cremers, D., Pock, T.: A convex approach to minimal partitions. SIAM J. Imaging Sci. 5(4), 1113–1158 (2012)
Chan, T., Esedoglu, S., Nikolova, M.: Algorithms for Finding Global Minimizers of Image Segmentation and Denoising Models. SIAM J. Appl. Math. 66(5), 1632–1648 (2006)
Hérault, L., Horaud, R.: Figure-ground discrimination: a combinatorial optimization approach. IEEE Trans. Pattern Anal. Mach. Intell. 15(9), 899–914 (1993)
Heskes, T.: Convexity arguments for efficient minimization of the Bethe and Kikuchi free energies. J. Artif. Intell. Res. 26, 153–190 (2006)
Hofbauer, J., Siegmund, K.: Evolutionary game dynamics. Bull. Am. Math. Soc. 40(4), 479–519 (2003)
Hofman, T., Buhmann, J.: Pairwise data clustering by deterministic annealing. IEEE Trans. Pattern Anal. Mach. Intell. 19(1), 1–14 (1997)
Horst, R., Tuy, H.: Global Optimization: Deterministic Approaches, 3rd edn. Springer, Berlin (1996)
Hummel, R., Zucker, S.: On the foundations of the relaxation labeling processes. IEEE Trans. Pattern Anal. Mach. Intell. 5(3), 267–287 (1983)
Jost, J.: Riemannian Geometry and Geometric Analysis, 4th edn. Springer, Berlin (2005)
Kappes, J., Andres, B., Hamprecht, F., Schnörr, C., Nowozin, S., Batra, D., Kim, S., Kausler, B., Kröger, T., Lellmann, J., Komodakis, N., Savchynskyy, B., Rother, C.: A comparative study of modern inference techniques for structured discrete energy minimization problems. Int. J. Comput. Vis. 115(2), 155–184 (2015)
Kappes, J., Savchynskyy, B., Schnörr, C.: A bundle approach to efficient MAP-inference by Lagrangian relaxation. In: Proc. CVPR (2012)
Kappes, J., Schnörr, C.: MAP-inference for highly-connected graphs with DC-programming. In: Pattern Recognition—30th DAGM Symposium, LNCS, vol. 5096, pp. 1–10. Springer (2008)
Karcher, H.: Riemannian center of mass and mollifier smoothing. Commun. Pure Appl. Math. 30, 509–541 (1977)
Karcher, H.: Riemannian center of mass and so called karcher mean. arxiv:1407.2087 (2014)
Kass, R.: The geometry of asymptotic inference. Stat. Sci. 4(3), 188–234 (1989)
Kolmogorov, V.: Convergent tree-reweighted message passing for energy minimization. IEEE Trans. Pattern Anal. Mach. Intell. 28(10), 1568–1583 (2006)
Kolmogorov, V., Zabih, R.: What energy functions can be minimized via graph cuts? IEEE Trans. Pattern Anal. Mach. Intell. 26(2), 147–159 (2004)
Ledoux, M.: The Concentration of Measure Phenomenon. American Mathematical Society, Providence (2001)
Lellmann, J., Lenzen, F., Schnörr, C.: Optimality bounds for a variational relaxation of the image partitioning problem. J. Math. Imaging Vis. 47(3), 239–257 (2013)
Lellmann, J., Schnörr, C.: Continuous multiclass labeling approaches and algorithms. SIAM J. Imaging Sci. 4(4), 1049–1096 (2011)
Losert, V., Alin, E.: Dynamics of games and genes: discrete versus continuous time. J. Math. Biol. 17(2), 241–251 (1983)
Luce, R.: Individual Choice Behavior: A Theoretical Analysis. Wiley, New York (1959)
Milanfar, P.: A tour of modern image filtering. IEEE Signal Process. Mag. 30(1), 106–128 (2013)
Milanfar, P.: Symmetrizing smoothing filters. SIAM J. Imaging Sci. 6(1), 263–284 (2013)
Montúfar, G., Rauh, J., Ay, N.: On the fisher metric of conditional probability polytopes. Entropy 16(6), 3207–3233 (2014)
Nesterov, Y., Todd, M.: On the riemannian geometry defined by self-concordant barriers and interior-point methods. Found. Comput. Math. 2, 333–361 (2002)
Orland, H.: Mean-field theory for optimization problems. J. Phys. Lett. 46(17), 763–770 (1985)
Pavan, M., Pelillo, M.: Dominant sets and pairwise clustering. IEEE Trans. Pattern Anal. Mach. Intell. 29(1), 167–172 (2007)
Pelillo, M.: The dynamics of nonlinear relaxation labeling processes. J. Math. Imaging Vis. 7, 309–323 (1997)
Pelillo, M.: Replicator equations, maximal cliques, and graph isomorphism. Neural Comput. 11(8), 1933–1955 (1999)
Rosenfeld, A., Hummel, R., Zucker, S.: Scene labeling by relaxation operations. IEEE Trans. Syst. Man Cybern. 6, 420–433 (1976)
Singer, A., Shkolnisky, Y., Nadler, B.: Diffusion interpretation of non-local neighborhood filters for signal denoising. SIAM J. Imaging Sci. 2(1), 118–139 (2009)
Sutton, R., Barto, A.: Reinforcement Learning, 2nd edn. MIT Press, Cambridge (1999)
Swoboda, P., Shekhovtsov, A., Kappes, J., Schnörr, C., Savchynskyy, B.: Partial optimality by pruning for MAP-inference with general graphical models. IEEE Trans. Patt. Anal. Mach. Intell. 38(7), 1370–1382 (2016)
Wainwright, M., Jordan, M.: Graphical models, exponential families, and variational inference. Found. Trends Mach. Learn. 1(1–2), 1–305 (2008)
Weickert, J.: Anisotropic Diffusion in Image Processing. B.G Teubner, Leipzig (1998)
Werner, T.: A linear programming approach to max-sum problem: a review. IEEE Trans. Pattern Anal. Mach. Intell. 29(7), 1165–1179 (2007)
Yedidia, J., Freeman, W., Weiss, Y.: Constructing free-energy approximations and generalized belief propagation algorithms. IEEE Trans. Inf. Theory 51(7), 2282–2312 (2005)
Acknowledgements
Support by the German Research Foundation (DFG) was gratefully acknowledged, Grant GRK 1653.
Author information
Authors and Affiliations
Corresponding author
Appendices
Appendix 1: Basic Notation
For \(n \in {\mathbb {N}}\), we set \([n] = \{1,2,\ldots ,n\}\). \({\mathbbm {1}}= (1,1,\ldots ,1)^{\top }\) denotes the vector with all components equal to 1, whose dimension can either be inferred from the context or is indicated by a subscript, e.g., \({\mathbbm {1}}_{n}\). Vectors \(v^{1}, v^{2},\ldots \) are indexed by lowercase letters and superscripts, whereas subscripts \(v_{i},\, i \in [n]\), index vector components. \(e^{1},\ldots ,e^{n}\) denotes the canonical orthonormal basis of \(\mathbb {R}^{n}\).
We assume data to be indexed by a graph \({\mathscr {G}}=({\mathscr {V}},{\mathscr {E}})\) with nodes \(i \in {\mathscr {V}}=[m]\) and associated locations \(x^{i} \in \mathbb {R}^{d}\), and with edges \({\mathscr {E}}\). A regular grid graph and \(d=2\) is the canonical example. But \({\mathscr {G}}\) may also be irregular due to some preprocessing like forming super-pixels, for instance, or correspond to 3D images or videos (\(d=3\)). For simplicity, we call i location although this actually is \(x^{i}\).
If \(A \in \mathbb {R}^{m \times n}\), then the row and column vectors are denoted by \(A_{i} \in \mathbb {R}^{n},\, i \in [m]\) and \(A^{j} \in \mathbb {R}^{m},\, j \in [n]\), respectively, and the entries by \(A_{ij}\). This notation of row vectors \(A_{i}\) is the only exception from our rule of indexing vectors stated above.
The componentwise application of functions \(f :\mathbb {R}\rightarrow \mathbb {R}\) to a vector is simply denoted by f(v), e.g.,
Likewise, binary relations between vectors apply componentwise, e.g., \(u \ge v \;\Leftrightarrow \; u_{i} \ge v_{i},\; i \in [n]\), and binary componentwise operations are simply written in terms of the vectors. For example,
where the latter operation is only applied to strictly positive vectors \(q > 0\). The support \({{\mathrm{supp}}}(p) = \{p_{i} \ne 0 :i \in {{\mathrm{supp}}}(p)\} \subset [n]\) of a vector \(p \in \mathbb {R}^{n}\) is the index set of all non-nonvanishing components of p.
\(\langle x, y \rangle \) denotes the standard Euclidean inner product and \(\Vert x\Vert = \langle x, x \rangle ^{1/2}\) the corresponding norm. Other \(\ell _{p}\)-norms, \(1 \le p \ne 2 \le \infty \), are indicated by a corresponding subscript, \( \Vert x\Vert _{p} = \big (\sum _{i \in [d]} |x_{i}|^{p}\big )^{1/p}, \) except for the case \(\Vert x\Vert = \Vert x\Vert _{2}\). For matrices \(A, B \in \mathbb {R}^{m \times n}\), the canonical inner product is \( \langle A, B \rangle = \hbox {tr}(A^{\top } B) \) with the corresponding Frobenius norm \(\Vert A\Vert = \langle A, A \rangle ^{1/2}\). \({{\mathrm{Diag}}}(v) \in \mathbb {R}^{n \times n},\, v \in \mathbb {R}^{n}\), is the diagonal matrix with the vector v on its diagonal.
Other basic sets and their notation are
-
the positive orthant
$$\begin{aligned} \mathbb {R}_{+}^{n} = \{ p \in \mathbb {R}^{n} :p \ge 0 \}, \end{aligned}$$(6.3) -
the set of strictly positive vectors
$$\begin{aligned} \mathbb {R}_{++}^{n} = \{p \in \mathbb {R}^{n} :p > 0\}, \end{aligned}$$(6.4) -
the ball of radius r centered at p
$$\begin{aligned} {\mathbb {B}}_{r}(p) = \{p \in \mathbb {R}^{n} :\Vert p\Vert \le r\}, \end{aligned}$$(6.5) -
the unit sphere
$$\begin{aligned} {\mathbb {S}}^{n-1} = \{p \in \mathbb {R}^{n} :\Vert p\Vert =1\}, \end{aligned}$$(6.6) -
the probability simplex
$$\begin{aligned} \varDelta _{n-1} = \{p \in \mathbb {R}_{+}^{n} :\langle {\mathbbm {1}}, p \rangle = 1 \} \end{aligned}$$(6.7) -
and its relative interior
$$\begin{aligned} {\mathscr {S}}&= \mathring{\varDelta }_{n-1} = \varDelta _{n-1} \cap \mathbb {R}_{++}^{n}, \end{aligned}$$(6.8a)$$\begin{aligned} {\mathscr {S}}_{n}&= {\mathscr {S}}\;\text {with concrete value of} n (e.g.,~{\mathscr {S}}_{3}), \end{aligned}$$(6.8b) -
closure (not regarded as manifold)
$$\begin{aligned} \overline{{\mathscr {S}}} = \varDelta _{n-1}, \end{aligned}$$(6.9) -
the sphere with radius 2
$$\begin{aligned} {\mathscr {N}} = 2 {\mathbb {S}}^{n-1}, \end{aligned}$$(6.10) -
the assignment manifold
$$\begin{aligned} {\mathscr {W}} = {\mathscr {S}} \times \cdots \times {\mathscr {S}}, \quad (\text {m times}) \end{aligned}$$(6.11) -
and its closure (not regarded as manifold)
$$\begin{aligned} \overline{{\mathscr {W}}} = \overline{{\mathscr {S}}} \times \cdots \times \overline{{\mathscr {S}}}, \quad (\text {m times}). \end{aligned}$$(6.12)
For a discrete distribution \(p \in \varDelta _{n-1}\) and a finite set \(S=\{s^{1},\ldots ,s^{n}\}\) vectors, we denote by
the mean of S with respect to p.
Let \({\mathscr {M}}\) be a any differentiable manifold. Then \(T_{p}{\mathscr {M}}\) denotes the tangent space at base point \(p \in {\mathscr {M}}\) and \(T{\mathscr {M}}\) the total space of the tangent bundle of \({\mathscr {M}}\). If \(F :{\mathscr {M}} \rightarrow {\mathscr {N}}\) is a smooth mapping between differentiable manifold \({\mathscr {M}}\) and \({\mathscr {N}}\), then the differential of F at \(p \in {\mathscr {M}}\) is denoted by
If \(F :\mathbb {R}^{m} \rightarrow \mathbb {R}^{n}\), then \(DF(p) \in \mathbb {R}^{n \times m}\) is the Jacobian matrix at p, and the application DF(p)[v] to a vector \(v \in \mathbb {R}^{m}\) means matrix-vector multiplication. We then also write DF(p)v. If \(F = F(p,q)\), then \(D_{p}F(p,q)\) and \(D_{q}F(p,q)\) are the Jacobians of the functions \(F(\cdot ,q)\) and \(F(p,\cdot )\), respectively.
The gradient of a differentiable function \(f :\mathbb {R}^{n} \rightarrow \mathbb {R}\) is denoted by \(\nabla f(x) = \big (\partial _{1} f(x),\ldots ,\partial _{n} f(x)\big )^{\top }\), whereas the Riemannian gradient of a function \(f :{\mathscr {M}} \rightarrow \mathbb {R}\) defined on Riemannian manifold \({\mathscr {M}}\) is denoted by \(\nabla _{{\mathscr {M}}} f\). Eq. (2.5) recalls the formal definition.
The exponential mapping [21, Def. 1.4.3]
maps the tangent vector v to the point \(\gamma _{v}(1) \in {\mathscr {M}}\), uniquely defined by the geodesic curve \(\gamma _{v}(t)\) emanating at p in direction v. \(\gamma _{v}(t)\) is the shortest path on \({\mathscr {M}}\) between the points \(p, q \in {\mathscr {M}}\) that \(\gamma _{v}\) connects. This minimal length equals the Riemannian distance \(d_{{\mathscr {M}}}(p,q)\) induced by the Riemannian metric, denoted by
i.e., the inner product on the tangent spaces \(T_{p}{\mathscr {M}},\,p \in {\mathscr {M}}\), that smoothly varies with p. Existence and uniqueness of geodesics will not be an issue for the manifolds \({\mathscr {M}}\) considered in this paper.
Remark 8
The exponential mapping \({{\mathrm{Exp}}}_{p}\) should not be confused with
-
the exponential function \(e^{v}\) used, e.g., in (6.1);
-
the mapping \(\exp _{p} :T_{p}{\mathscr {S}} \rightarrow {\mathscr {S}}\) defined by Eq. (3.8a).
The abbreviations “l.h.s.” and “r.h.s.” mean left-hand side and right-hand side of some equation, respectively. We abbreviate with respect to by “wrt.”
Appendix 2: Proofs and Further Details
1.1 Proofs of Section 2
Proof
(of Lemma 1) Let \(p \in {\mathscr {S}}\) and \(v \in T_{p}{\mathscr {S}}\). We have
and \(\big \langle \psi (p), D\psi (p)[v] \big \rangle = \langle 2 \sqrt{p}, \frac{v}{\sqrt{p}} \rangle = 2 \langle {\mathbbm {1}}, v \rangle = 0\), that is, \(D\psi (p)[v] \in T_{\psi (p)}{\mathscr {N}}\). Furthermore,
i.e., the Riemannian metric is preserved and hence also the length L(s) of curves \(s(t) \in {\mathscr {N}},\, t \in [a,b]\): Put \(\gamma (t) = \psi ^{-1}\big (s(t)\big ) = \frac{1}{4} s^{2}(t) \in {\mathscr {S}},\, t \in [a,b]\). Then \(\dot{\gamma }(t)=\frac{1}{2} s(t) \dot{s}(t) = \frac{1}{2} \psi \big (\gamma (t)\big ) \dot{s}(t) = \sqrt{\gamma (t)} \dot{s}(t)\) and
\(\square \)
Proof
(of Prop. 1) Setting \(g:{\mathscr {N}} \rightarrow \mathbb {R}\), \(q \mapsto g(s) := f\big (\psi ^{-1}(s)\big )\) with \(s = \psi (p) = 2 \sqrt{p}\) from (2.3), we have
because the 2-sphere \({\mathscr {N}}=2{\mathbb {S}}^{n-1}\) is an embedded submanifold, and hence the Riemannian gradient equals the orthogonal projection of the Euclidean gradient onto the tangent space. Pulling back the vector field \(\nabla _{{\mathscr {N}}} g\) by \(\psi \) using
we get with (7.1), (7.4) and \(\Vert s\Vert =2\) and hence \(s/\Vert s\Vert = \frac{1}{2} \psi (p)=\sqrt{p}\)
which equals (2.6). We finally check that \(\nabla f_{{\mathscr {S}}}(p)\) satisfies (2.5) (with \({\mathscr {S}}\) in place of \({\mathscr {M}}\)). Using (2.1), we have
\(\square \)
Proof
(of Prop. 2) The geodesic on the 2-sphere emanating at \(s(0) \in {\mathscr {N}}\) in direction \(w=\dot{s}(0) \in T_{s(0)}{\mathscr {N}}\) is given by
Setting \(s(0)=\psi (p)\) and \(w = D\psi (p)[v]=v/\sqrt{p}\), the geodesic emanating at \(p=\gamma _{v}(0)\) in direction v is given by \(\psi ^{-1}\big (s(t)\big )\) due to Lemma 1, which results in (2.7a) after elementary computations. \(\square \)
1.2 Proofs of Section 3 and Further Details
Proof
(of Prop. 3) We have \(p = \exp _{p}(0)\) and
which confirms (3.10), is equal to (3.9) at \(t=0\) and hence yields the first expression of (3.11). The second expression of (3.11) follows from a Taylor expansion of (2.7a)
\(\square \)
Proof
(of Lemma 4) By construction, \(S(W) \in {\mathscr {W}}\), that is, \(S_{i}(W) \in {\mathscr {S}},\; i \in [m]\). Consequently,
The upper bound corresponds to matrices \(\overline{W}^{*} \in \overline{{\mathscr {W}}}\) and \(S(\overline{W}^{*})\) where for each \(i \in [m]\), both \(\overline{W}^{*}_{i}\) and \(S_{i}(\overline{W}^{*})\) equal the same unit vector \(e^{k_{i}}\) for some \(k_{i} \in [m]\). \(\square \)
Proof
(Explicit form of (3.27)) The matrices \(T^{ij}(W) = \frac{\partial }{\partial W_{ij}} S(W)\) are implicitly given through the optimality condition (2.9) that each vector \(S_{k}(W),\, k \in [m]\), defined by (3.13) has to satisfy
Writing
while temporarily dropping below W as argument to simplify the notation, and using the indicator function \(\delta _{{\mathrm {P}}} = 1\) if the predicate \({\mathrm {P}}={\mathrm {true}}\) and \(\delta _{{\mathrm {P}}} = 0\) otherwise, we differentiate the optimality condition on the r.h.s. of (7.12),
Since the vectors \(\phi (S_{k},L_{r})\) given by (7.13) are the negative Riemannian gradients of the (locally) strictly convex objectives (2.8) defining the means \(S_{k}\) [21, Thm. 4.6.1], the regularity of the matrices \(H^{k}(W)\) follows. Thus, using (7.14f) and defining the matrices
results in (3.27). The explicit form of this expression results from computing and inserting into (7.14f) the corresponding Jacobians \(D_{p}\phi (p,q)\) and \(D_{q}\phi (p,q)\) of
and
The term (7.16b) results from mapping back the corresponding vector from the 2-sphere \({\mathscr {N}}\),
where \(\psi \) is the sphere map (2.3) and \(d_{{\mathscr {N}}}\) is the geodesic distance on \({\mathscr {N}}\). The term (7.16c) results from directly evaluating (3.12). \(\square \)
Proof
(of Lemma 5) We first compute \(\exp _{p}^{-1}\). Suppose
Then
and
Thus, in view of (3.9), we approximate
Applying this to the point set \({\mathscr {P}}\), i.e., setting
step (3) of (3.31) yields
Finally, approximating step (4) of (3.31) results in view of Prop. 3 in the update of p
\(\square \)
Rights and permissions
About this article
Cite this article
Åström, F., Petra, S., Schmitzer, B. et al. Image Labeling by Assignment. J Math Imaging Vis 58, 211–238 (2017). https://doi.org/10.1007/s10851-016-0702-4
Received:
Accepted:
Published:
Issue Date:
DOI: https://doi.org/10.1007/s10851-016-0702-4
Keywords
- Image labeling
- Assignment manifold
- Fisher–Rao metric
- Riemannian gradient flow
- Replicator equations
- Information geometry
- Neighborhood filters
- Nonlinear diffusion