Abstract
Distance-based morphometry is still widely used in ichthyology. Beside others, this methodology is often used to characterise species or to compare intraspecific group (i.e. population level) differences. However, scarce information is available about: (a) which are the most widely used variables for these purposes, (b) how certain variables are selected for the morphometric analyses, and (c) how variable set compositions and the number of variables affect the results. To answer these questions, a literature review was compiled and our own three datasets were analysed. The results showed that although a high number of variables can be used, previous authors have measured “common” ones most often, regardless of the taxonomic position of the studied group and the goal of the survey. Additionally, our review showed that authors rarely made a variable selection and often did not standardise their datasets; these are methodical problems that make the accuracy and usability of the results questionable. Analyses of our own three datasets showed that the number of variables and the variable set compositions in most cases strongly influenced stock subdivision and the percentage of correctly classified individuals. It was also shown that the most useable variable sets for morphometric purposes can differ considerably depending on the taxon and goal of the survey.
Similar content being viewed by others
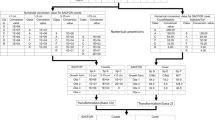
Avoid common mistakes on your manuscript.
Introduction
Morphometry is a scientific methodology which gives a qualitative description, analysis, and interpretation of shape and shape variation in biology (Rohlf, 1990). Morphometric methods are employed in many fields of science, from clinical diagnostics (Azaria et al., 2003) to palaeontology (Ball et al., 2016; Zhao et al., 2020). These methods have also been widely used in ichthyology to isolate stocks or species (González-Castro et al., 2012), to reveal ontogenetic changes (Biolé et al., 2019), to solve taxonomic problems (Golubtsov et al., 2012), and to answer certain ecological (Sibbing & Nagelkerke, 2001), evolutionary (Bookstein et al., 1985), and speciation questions (Barluenga et al., 2006).
Morphometric methods have changed significantly in the last decades. The introduction of the truss base system (Strauss & Bookstein, 1982) and image analyses (Cadrin & Friedland, 1999) has increased the accuracy of the traditional distance-based method. Moreover, the field of geometric morphometrics, which was devised in the mid-1990s (Rohlf & Marcus, 1993), has proven to be more accurate, less costly, and more time effective (Parsons et al., 2003; Maderbacher et al., 2008; Viscosi et al., 2009) than traditional distance measurements. These improvements, together with the newly developed statistical background (see: Zelditch et al., 2004) and the widespread use of personal computers, which can be used to perform more complex statistical calculations, have revolutionised this long-used research methodology (Adams et al., 2004). Thus, the number of morphometric-themed ichthyological articles, also using distance-based methods, has considerably increased in recent times (Fig. 1).
Number of articles indexed in the Web of Science database dealing with fish morphometry in the last 45 years (orange dots), and the number of morphometric articles presented using classic distance-based (blue rectangles) and geometric (grey triangles) methods. Polynomial trend lines were fitted to the data points. Search criteria for the total number of fish morphometric articles (Ntot = 3380): all fields: (morphometry) or topic: (geometric) and all fields: (landmark) all fields: (fish) and topic: (shape) refined by: Web of Science categories: (Fisheries or Zoology or Marine Freshwater Biology or Biology or Ecology) Search criteria for the number of distance-based fish morphometric articles (N = 959): all fields: (Morphometry) and all fields: (Fish) not all fields: (Geometric) not all fields: (Landmark) not topic: (Shape)
Like most research methods, morphometry has its own characteristics and limitations. Although several methodological articles dealing with the applicability, usability, and sensitivity of morphometric methods have been published recently (Cadrin, 2000; Kocovsky et al., 2009; Baur & Leuenberger, 2011; Petrtýl et al., 2014; Takács et al., 2016; Takács et al., 2018), a number of issues relating to the execution of the measurements have still not been clarified. For example, although we can find reports summarising the usable/recommended variables for morphometric measurements (Cadrin, 2000; Armbruster, 2012), to our knowledge there is not a generally used protocol for distance-based fish morphometry. Nonetheless, for the sake of comparability, there were attempts to determine the variables to be measured on a taxonomic basis (Pravdin, 1966). Nowadays, however, it seems that the authors’ choice mostly relies on previous literature on a similar topic to determine the variables to be measured (Tulli et al., 2009; Sirakov et al., 2012). Similarly, it is still unclear what the optimal number of variables is, if any, to show differences at a population level. In some special cases, a few well-chosen variables may be sufficient to distinguish groups (Franklin et al., 2012). Conversely, some authors have often analysed as many variables as possible and the number of variables has sometimes exceeded thirty (e.g. Elliott et al., 1995). At the same time, the acquisition of morphometric data is a time- and energy-consuming process, and not all variables are equally informative in terms of group differentiation. Indeed, the employment of less changing variables may make it more difficult to separate groups. Additionally, there are variables that are more difficult to record, so their data are more frequently burdened with measurement errors (Yezerinac et al., 1992) which can make group segregation more difficult. Therefore, it can be rightly assumed that by including different morphometric variables in the analysis and by changing the number of variables used, the degree of group separation may also change considerably. Furthermore, the multivariate statistical methods commonly used in morphometry (e.g. canonical variance analysis) do not allow the number of variables measured to be greater than the number of investigated individuals (Zelditch et al., 2004). Indeed, in order to increase the number of analysed variables, the number of samples per group must also be increased. Additionally, to obtain a stable outcome from Principal Component and Discriminant Function analyses (PCA, DFA) three to eight times more individuals than the number of variables planned to be measured should be included in the analyses (Kocovsky et al., 2009). However, the examination of a larger number of individuals is not feasible in many cases (e.g. comparison of small populations of protected species). Thus, in terms of both time savings and the requirements of statistical analyses, there is a necessity to consider which variables are most worthy of being measured to define the optimal number of variables, if it can be established at all, for morphometric studies.
In our present work, we provide an overview of the number and kinds of variables that are most frequently used in distance-based morphometric analyses and how the variable number and variable set composition are affected by the goal of the surveys (i.e. intra- or interspecific group differences revealed). Using our own morphometric datasets we investigate if there are any generally used variables for intraspecific (population level) morphometric studies. We also reveal how the level of group differences changes depending on the number of variables analysed.
Materials and methods
Literature review
In the literature review, the data of 70 scientific articles employing classic distance-based morphometric methods were examined, in which the term “morphometry” and/or “morphology” was included in the title and/or keywords. Then, we distinguished the reviewed studies by topic into “interspecific” and “intraspecific” groups. Namely, we separated the articles where the morphometric method was used for the general characterisation of a species and/or to reveal interspecies differences, from those in which group/population level differences were revealed. The number of morphometric variables studied per article and the frequency of the variables used in these works were then recorded. Moreover, we revealed if the goal of the survey (to reveal inter- or intraspecific differences) had any effect on the number of variables and/or the variable set composition. Additionally, we recorded if the authors performed a data standardisation and variable selection.
Own data processing and analyses
To test the effect of variable composition on the results of the morphometric studies, we used our own datasets of two cyprinid taxa: the European gudgeon (Gobio gobio complex, Takács, 2018)—hereafter gudgeon, Petényi barbel (Barbus petenyi, Heckel, 1852)—hereafter barbel and the centrachid pumpkinseed sunfish Lepomis gibbosus (Linnaeus, 1758)—hereafter sunfish. We collected samples from five populations for each taxon using an electric fishing gear (permission numbers: PE-KTF/659-15/2017, ANPA Agentia Nationala pentru Pescuit si Acvacultura: 08/21.03.2016). The geographical position, numbering of sampled waterbodies, locations, and other important information are shown in Table 1 and Fig. 2. The captured individuals were placed flat on a polystyrol surface and their left sides were photographed from a perpendicular angle using a tripod-mounted Nikon D5300 digital camera with a fixed zoom range. The measurement of 35 morphometric variables was performed on these digital photos using the ImageJ software (Rasband, 2012). We recorded the shortest distances between the designated 25 landmark points (Fig. 3). To eliminate interobserver variability (Takács et al., 2016), all measurements were made by the same person. The name, abbreviations, and start and end points of the measured variables are shown in Table 2. The measurement data were standardised by the standard length (SL) using the formula of Elliott et al. (1995):
Geographical distribution of sampling sites (A) gudgeon (1–5) and barbel (6–10). The sunfish sampling sites on littoral region of Lake Balaton (11–16) are shown in insert B. Different shapes show the sampling sites of different species, circle: gudgeon, triangle: barbel, and rectangle: sunfish. The geographical position of the sampled area in Europe is shown in insert C. For more details see Table 1
The start and end points of the 35 morphometric distances measured. The names and codes of the measured morphometric variables are indicated in Table 2
where Madj is the value of the standardised variable, M is the value of the originally measured variable, Ls is the average of the standard body lengths of the subjects, L0 is the standard body length of the subject, and parameter “b” is the slope of the logarithmic values of the given variable and the linear regression line of the log-transformed standard body lengths. Spearman rank correlation analyses were performed between the standardised variables and the standard body lengths to check the elimination of size effects from the datasets.
The importance of each variable in group separation was determined by their F-values (Pope and Webster, 1972), which is the ratio of the sum of squares amongst and within groups. The variable with a higher F-value tends to have a greater importance in group separation. We applied a backward stepwise variable selection to reveal the effect of variable number on group differentiation (Cadrin, 2000). We used the F-values of the variables to determine the order in which they were omitted from the analyses. Altogether 33 canonical variance analyses (CVA) had decreasing (34, 33, 32…2) variable numbers. Initially, all variables were included in the variance analysis; then the variable with the lowest F-value was omitted from the dataset and then the next and so on, until we performed the CVA using the two variables characterised by the highest F-values. To characterise the level of group separation, three features of CVA—the squared Mahalanobis distances of the group centroids, the percentage of correctly classified individuals, and the percentage of significant pairwise group differentiations—were used, assigning the pairwise Bonferroni-corrected Hotelling’s to P < 0.05 (Zelditch et al., 2004). The datasets of squared pairwise Mahalanobis distances and the percentage of correctly classified cases were visualised using LOESS smoothing (Cleveland, 1979). Statistical analyses were performed using PAST 2.17 (Hammer et al., 2001).
Results
Literature review
Out of the 70 articles reviewed (listed in Supplementary Table 1), the morphometry was used to characterise interspecific differences in 39 scientific works. Intraspecific differences (e.g. population level) were revealed in the other 31 articles. The overall evaluation—including the data from all reviewed articles—showed that a total of 137 different morphometric variables were recorded. The average (± SD) number of recorded variables per scientific paper was 15.23 ± 9.34 (range 1–37). No single variable was found which was used in all reviewed articles. The most frequently recorded variables were the standard length (SL) and head length (HL) and both occurred in 86.6% of the reviewed articles. A further six variables were detected in more than the half of the reviewed works (Fig. 4). Only 37 variables had more than a 10% frequency of occurrence and about half of the morphometric variables (68) were recorded in only one case. Data standardisation was performed in 55 out of the 70 (78.6%) reviewed articles, whilst a variable selection was conducted in two cases (2.8%) only. Comparing the articles on different topics, we found that although more variables were used in interspecific works than in intraspecific studies (107 vs 89), 13 of the 15 most frequently used variables were the same in the two groups (Supplementary Table 2). On average, less variables were analysed in intraspecific (mean ± SD = 13.71 ± 8.6) than in interspecific studies (mean ± SD = 16.43 ± 98), but these differences were not significant (Mann–Whitney U test: T = Ub: 505, p(same) = 0.2411).
Graphical presentation of the morphometric literature review. The frequency of occurrence of the 137 morphometric variables is shown in insert (A). The eight variables that occurred in more than half of the reviewed articles are indicated by red bars and presented on the insert (B), where the rank of each variable is shown in brackets. For the abbreviation of variable names and raw frequency data see Table 2 and Supplementary material 1. The number of recorded variables per literature article is presented on a boxplot (C), where the box represents the 25% and 75% quartiles of the dataset, and the band in the box is the median. The whiskers are drawn from the top of the box up to the largest data point 1.5 times the box height from the box and, similarly, below the box
The most informative morphometric variables
Raw datasets of the three studied species used for the morphometric analyses are available in the public depository of Mendeley (https://data.mendeley.com/datasets/c8856zg4hj/1). No significant correlations (Spearman’s D, P < 0.05) were evident between either of the standardised variables and the SL. Thus, the size effect was removed, and therefore all morphometric characters could be used for the further analyses. The standardised morphometric variables were set in descending order according to their F-values in the case of each of the studied species (Table 3). The results of F statistics showed that in the case of the two cyprinid species, almost half of the 15 most important variables (DPC, DVPL, EH, HL, Hh, Hmax, PPEC) were shared, and four variables (EH, HL, Hh, PPEC) were common amongst the three studied taxa. However the importance (rank) of these variables differed greatly amongst the taxa. The 15 morphometric variables which were most important in discriminating amongst the populations of each studied taxa are shown in Fig. 5.
Effects of variable number reduction on the results of CVA
The squared Mahalanobis distances of the compared populations varied between 0.05–29.65, 0.11–57.09, and 0.03–41.04 for the gudgeon, barbel, and sunfish, respectively. The percentage of correctly classified individuals ranged from 26 to 100%, 25 to 100%, and 20 to 100% for the gudgeon, barbel, and sunfish populations, respectively. The percentage of significant (P < 0.05) pairwise differences ranged from 0 to 90% for the gudgeon and barbel populations and between 0 and 60% for the sunfish population (see: Supplementary Table 3). The changes of the three CVA parameters in the function of the analysed variable numbers are presented in Fig. 6 (for the whole dataset see: Supplementary Table 3.
Changes in the squared pairwise Mahalanobis distances of group centroids (A–C), the correctly classified cases (D–F), and the proportion of significant pairwise group differences (G–I) in the function of morphometric variables included in the analysis. Grey dots on A–F subfigures are the individual data, whilst trend lines (red) were generated using LOESS smoothing, with 95% confidence bands (blue dashed lines) for the curve based on 999 random replicates
Discussion
Analysis of literature notes
The literature review showed that many authors have used a great variety of morphometric features. The total of 137 morphometric variables contained in the reviewed studies considerably exceeds the variable numbers listed in summary works (Pravdin, 1966; Winans, 1987; Armbruster, 2012). At the same time, the number of recorded variables showed a considerable deviation in the reviewed articles. The highest number of variables (37) was used to describe the morphology of a pikeperch hybrid (Specziár et al., 2009). Thus, there are articles in which the term “morphometry” is not used properly in the title, because only the standard length was measured amongst the studied individuals (Pulgar et al., 2011).
Assessing the literature notes by topic, it turned out that although more kinds of variables are used in the interspecific than in the intraspecific works, no relevant differences can be found neither in the measured variable number, nor in the variable set compositions. It also appears that 9–10 out of the average 15 generally recorded morphometric variables are commonly used, independently of the topic of the article. Beside these “common” variables, authors have often recorded specific ones that are supposed to be characteristic of the studied group. This could explain why almost half of the detected variables were used in only one study.
Comparing the results of the literature overview and our own analyses, we found considerable differences between the most frequently used and the most informative variables. In the reviewed literature, apart from the SL, which is generally only used for data standardisation (Elliott et al., 1995), only two variables—the head length (HL) and the horizontal eye diameter (EH)—were common with the 15 most informative variables (i.e. the ones with the highest F-values) in our analyses (Fig. 4, Table 3). These results indicate that the key morphometric variables change considerably from taxon to taxon. At the same time, several similar variables can be found amongst closely related species. Moreover, the common feature of these variables is that they are situated mainly toward the anterior part of the species’ body (Fig. 5).
The results of the literature review also showed that almost a quarter of the authors did not perform any data standardisation processes, and only two publications were found in which a variable selection was made. These findings suggest that the published results may be burdened by methodological errors. Indeed, it can be assumed that an incorrectly compiled variable set (compiled by including non-separating variables in it) may produce an inadequate/underestimated group separation. The lack of standardisation is also a considerable problem (Zelditch et al., 2004), since the differences between groups can be significantly overestimated because the effect of body size is not taken into account.
Effects of variable number reduction on the CVA results
The analyses of our own three datasets showed that the variable number reduction considerably influenced the results of morphometric studies. The squared Mahalanobis distance values of the group centroids showed a continuous decrease for all studied taxa (Fig. 6A–C) with the reduction of the variable number. The percentage of correctly classified individuals showed a decrease as well, but these values initially showed only slight changes. If the number of variables included in the analysis was reduced from 34 to 17, the percentage of the correctly classified individuals decreased from 100 to 92% for the barbel, and from 92 to 83% for the gudgeon and sunfish. However, the decline accelerated below this variable number in all three cases (Fig. 6D–F). No significant group differences were evident above 25, 29, and 28 analysed variables for the gudgeon, barbel, and sunfish populations, respectively. The percentage of the significant pairwise group differences showed considerable increases up to 90% for the two cyprinid taxa. The proportion of significant group differences decreased with a further reduction of the number of variables analysed (Fig. 6G–I). In the case of the sunfish, after the initial rise, the curve plateaued at the 50% pairwise group difference level, with a single 60% peak at ten analysed variables (Fig. 6).
Therefore, these three characteristics showed a similar trend for all studied taxa. Solely in the case of the sunfish, the percentage of significant group separations showed lower values than the two cyprinid species. This difference on the one hand can be explained by the lower spatial scale of the sampling. Namely, we collected all the studied sunfish assemblages from the artificial, boulder-covered littoral region (rip-raps) of the Lake Balaton, whereas the sampled populations of the two cyprinid taxa were obtained from remote drainage systems (Fig. 2, Table 1). Moreover, this non-indigenous species first appeared in the lake just over a century ago (Vutskits, 1912). Therefore, it is likely that the invasion of the sunfish reduced its genetic diversity (Grapputo et al., 2006) and may have had an impact on its morphology as well (Hauser et al., 1995).
Our results showed that the number of significant group separations can be maximised with an analysis of 5–10 variables. At the same time, the percentage of correctly classified cases and the distance of the group centroids showed the highest values when all 34 variables were included in the analysis. Interestingly, in the reviewed literature, around 15 variables were analysed on average. This number is higher than is needed to maximise the significance of group separations, but lower than required to maximise the percentage of correctly classified individuals and the distance of the group centroids (see Fig. 6). We therefore suggest setting the employed variable number according to the goal of the survey, by performing a variable selection using the results of F statistics prior to the CVA analyses.
Conclusion
The literature review showed that although previous authors could choose from a large number of variables, they commonly measured a limited number and mainly selected commonly used morphometric variables. Indeed, it appears that the topic of the survey (intra- or interspecific) did not affect the variable number nor the variable set composition. This fact, together with the lack of a variable selection and data standardisation, can easily cause a misestimation of morphometric differences amongst the studied groups. The results of our analyses partly reinforced the assumption that there is no universally usable variable set. At the same time, the most informative variables can be measured on the anterior part of the body of each of the studied species. For related taxa, the best set of variables to use to isolate their stocks may be more similar. Nonetheless, our results suggest that for the best performance of the distance-based morphometric method, it may be worthwhile recording as many variables as possible. The more variables are recorded, the more efficiently the individuals can be classified into their source populations. This process also allows the variable set, which is the most appropriate to be selected, in order to reach the highest level of significance of group separation. However, it is nonetheless worth determining the number of variables to be measured according to the specific goals of the morphometric study because different purposes require a different number of variables. Indeed, more variables must be recorded to determine the source population of an individual than to reveal differences at the population level.
Data availability
The raw morphometric datasets are available in the public depository of Mendeley: https://data.mendeley.com/datasets/c8856zg4hj/1. All other data are available in the text or in the supplementary material.
Code availability
Not applicable.
References
Adams, D. C., F. J. Rohlf & D. E. Slice, 2004. Geometric morphometrics: Ten years of progress following the ‘revolution.’ Italian Journal of Zoology 71: 5–16.
Armbruster, J. W., 2012. Standardized measurements, landmarks, and meristic counts for cypriniform fishes. Zootaxa 3586: 8–16.
Azaria, R., N. Adler, R. Silfen, D. Regev & D. J. Hauben, 2003. Morphometry of the adult human earlobe: A study of 547 subjects and clinical application. Plastic and Reconstructive Surgery 111: 2398–2402.
Ball, T. B., A. L. Davis, R. R. Evett, J. L. Ladwig, M. Tromp, W. A. Out & M. Portillo, 2016. Morphometric analysis of phytoliths: Recommendations towards standardization from the International Committee for Phytolith Morphometrics. Journal of Archaeological Science Elsevier Ltd 68: 106–111.
Barluenga, M., K. N. Stölting, W. Salzburger, M. Muschick & A. Meyer, 2006. Sympatric speciation in Nicaraguan crater lake cichlid fish. Nature 439: 719–723.
Baur, H. & C. Leuenberger, 2011. Analysis of ratios in multivariate morphometry. Systematic Biology 60(6): 813–825.
Biolé, F. G., R. C. Fortunato, G. A. Thompson & A. V. Volpedo, 2019. Application of otolith morphometry for the study of ontogenetic variations of Odontesthes argentinensis. Environmental Biology of Fishes 102(10): 1301–1310.
Bookstein, F. L., B. Chernoff, R. L. Elder, J. M. Humphries, G. R. Smith, & R. E. Strauss, 1985. Morphometrics in evolutionary biology, the geometry of size and shape change with examples from fishes. Acad. Nat. Sci. Philadelphia Spec. Pub. 15.
Cadrin, S. X., 2000. Advances in morphometric identification of fishery stocks. Reviews in Fish Biology and Fisheries 10: 91–112.
Cadrin, S. X. & K. D. Friedland, 1999. The utility of image processing techniques for morphometric analysis and stock identification. Fisheries Research 43: 129–139.
Cleveland, W. S., 1979. Robust locally weighted regression and smoothing scatterplots. Journal of the American Statistical Association 74: 829–836.
Elliott, N. G., K. Haskard & J. A. Koslow, 1995. Morphometric analysis of orange roughy (Hoplostethus atlanticus) off the continental slope of southern Australia. Journal of Fish Biology 46: 202–220.
Franklin, D., A. Cardini, A. Flavel & A. Kuliukas, 2012. The application of traditional and geometric morphometric analyses for forensic quantification of sexual dimorphism: Preliminary investigations in a Western Australian population. International Journal of Legal Medicine 126: 549–558.
Golubtsov, A. S., S. E. Cherenkov & F. Tefera, 2012. High morphological diversity of the genus Garra in the Sore River (the White Nile Basin, Ethiopia): One more cyprinid species flock? Journal of Ichthyology 52: 817–820.
González-Castro, M., A. L. Ibáñez, S. Heras, M. I. Roldán & M. B. Cousseau, 2012. Assessment of lineal versus landmark-based morphometry for discriminating species of Mugilidae (Actinopterygii). Zoological Studies 51: 1515–1528.
Grapputo, A., A. Bisazza & A. Pilastro, 2006. Invasion success despite reduction of genetic diversity in the European populations of eastern mosquitofish (Gambusia holbrooki). Italian Journal of Zoology 73: 67–73.
Hammer, Ø., D. A. Harper & P. D. Ryan, 2001. PAST: Paleontological statistics software package for education and data analysis. Palaeontologia Electronica 4: 9.
Hauser, L., G. R. Carvalho & T. J. Pitcher, 1995. Morphological and genetic differentiation of the African clupeid Limnothrissa miodon 34 years after its introduction to Lake Kivu. Journal of Fish Biology 47: 127–144.
Kocovsky, P. M., J. V. Adams & C. R. Bronte, 2009. The Effect of Sample Size on the Stability of Principal Components Analysis of Truss-Based Fish Morphometrics. Transactions of the American Fisheries Society 138: 487–496.
Maderbacher, M., C. Bauer, J. Herler, L. Postl, L. Makasa & C. Sturmbauer, 2008. Assessment of traditional versus geometric morphometrics for discriminating populations of the Tropheus moorii species complex (Teleostei: Cichlidae), a Lake Tanganyika model for allopatric speciation. Journal of Zoological Systematics and Evolutionary Research 46: 153–161.
Parsons, K. J., B. W. Robinson & T. Hrbek, 2003. Getting into shape: an empirical comparison of traditional truss-based morphometric methods with a newer geometric method applied to New World cichlids. Environmental Biology of Fishes 67: 417–431.
Petrtýl, M., L. Kalous & D. Memiş, 2014. Comparison of manual measurements and computer-assisted image analysis in fish morphometry. Turkish Journal of Veterinary and Animal Sciences 38: 88–94.
Pope, P. T. & J. T. Webster, 1972. The use of an F-statistic in stepwise regression procedures. Technometrics 14: 327–340.
Pravdin, I. F., 1966. Manual on study of fishes. Pischevaya propishlennost Moscow, 376. Moscow.
Pulgar, J., M. Alvarez, J. Morales, M. Garcia-Huidobro, M. Aldana, F. P. Ojeda & V. M. Pulgar, 2011. Impact of oceanic upwelling on morphometric and molecular indices of an intertidal fish scartichthys viridis (Blenniidae). Marine and Freshwater Behaviour and Physiology 44: 33–42.
Rasband, W. S., 2012. ImageJ: Image processing and analysis in Java. ascl, ascl-1206.
Rohlf, F. J., 1990. Morphometrics. Annual Review of Ecology and Systematics 21: 299–316.
Rohlf, F. J. & L. F. Marcus, 1993. A revolution morphometrics. Trends in Ecology & Evolution 8: 129–132.
Sibbing, F. A., & L. A. Nagelkerke, 2001. Resource partitioning by Lake Tana barbs predicted from fish morphometrics and prey characteristics. Reviews in Fish biology and Fisheries.
Sirakov, I., Y. Staykov, E. Ivancheva, G. Nikolov & A. Atanasov, 2012. Morphometric characteristic of European perch (Perca fluviatilis) related to sex dimorphism. Agricultural Science and Technology 4: 203–207.
Specziár, A., M. Bercsényi & T. Müller, 2009. Morphological characteristics of hybrid pikeperch (Sander lucioperca ♀ × Sander volgensis ♂) (Osteichthyes, Percidae). Acta Zoologica Academiae Scientiarum Hungaricae 55: 39–54.
Strauss, R. E. & F. L. Bookstein, 1982. The Truss: Body Form Reconstructions in Morphometrics. Systematic Zoology 31: 113–135.
Takács, P., 2018. Megjegyzések a Magyarországon előforduló, Gobio genusba tartozó küllők taxonómiai helyzetével és névhasználatával kapcsolatban = Notes on the taxonomic position and naming problems of the Hungarian stream dwelling gudgeons (Gobio). Pisces Hungarici 12: 63–66.
Takács, P., Z. Vitál, Á. Ferincz & Á. Staszny, 2016. Repeatability, reproducibility, separative power and subjectivity of different fish morphometric analysis methods. PLoS ONE 11: 1–16.
Takács, P., Á. Ferincz, Á. Staszny & Z. Vitál, 2018. Effect of bodyside-specific data processing on the results of fish morphometric studies. Fundamental and Applied Limnology 192: 137–144.
Tulli, F., I. Balenovic, M. Messina & E. Tibaldi, 2009. Biometry traits and geometric morphometrics in sea bass (Dicentrarchus labrax) from different farming systems. Italian Journal of Animal Science 8: 881–883.
Viscosi, V., O. Lepais, S. Gerber & P. Fortini, 2009. Leaf morphological analyses in four European oak species (Quercus) and their hybrids: A comparison of traditional and geometric morphometric methods. Plant Biosystems 143: 564–574.
Vutskits, G., 1912. Az amerikai származású naphal meghonosodása a Balatonban. Természettudományi Közlöny 44: 467–468.
Winans, G. A., 1987. Using morphometrics and meristic characters for identifying stocks of fish. In H.E. Kumpf, R.N. Vaught, C.B. Grimes, A.G. Johnson, and E.L. Nakamura (editors), Proceedings of the Stock Identification Workshop. Panama City Beach, Florida, 5–7 November 1985. .
Yezerinac, S. M., S. C. Lougheed & P. Handford, 1992. Measurement error and morphometric studies: statistical power and observer experience. Systematic Biology 41: 471–482.
Zelditch, M. L., D. L. Swiderski, H. D. Sheets & W. L. Fink, 2004. Geometric morphometrics for biologists: a primer, Elsevier Academic Press, San Diego:
Zhao, W., J. Liu & R. D. C. Bicknell, 2020. Geometric morphometric assessment of guanshan trilobites (Yunnan province, China) reveals a limited diversity of palaeolenid taxa. Palaeontologia Electronica. https://doi.org/10.26879/1062.
Acknowledgements
We would like to thank Árpád Ferincz, András Attila Nagy, István Imecs, Gábor Maász, Zita Zrínyi, Bálint Preiszner, Tibor Erős, István Czeglédi, Nóra Boross, and Zoltán Vitál for their help in the sample collections. We are grateful to Andrew Hamer who corrected the grammar of the manuscript. We would like to thank Pauliina Louhi Associate editor and two anonymous reviewers for their helpful comments and discussion.
Funding
Open access funding provided by Balaton Limnological Research Institute. Bálint Bánó was supported by the ÚNKP-21-3 New National Excellence Programme of the Ministry for Innovation and Technology from the source of the National Research, Development and Innovation Fund and by the EFOP-3.6.3-VEKOP-16-2017-00008 of the European Union and the European Social Fund. Péter Takács was supported by the OTKA FK140902 Grant and the Bolyai Fellowship of the Hungarian Academy of Sciences. The research presented in this article was carried out with the support of the NKFIH–872–3/2020 project.
Author information
Authors and Affiliations
Contributions
The study was designed by PT, and BB made measurements. PT and BB made the statistical analyses and wrote the manuscript. Both authors read and approved the final manuscript.
Corresponding author
Ethics declarations
Conflict of interest
The authors declare that they have no competing interests.
Consent to participate
Not applicable.
Consent for publication
Not applicable.
Ethical approval
Not applicable.
Additional information
Handling Editor: Pauliina Louhi
Publisher's Note
Springer Nature remains neutral with regard to jurisdictional claims in published maps and institutional affiliations.
Supplementary Information
Below is the link to the electronic supplementary material.
Rights and permissions
Open Access This article is licensed under a Creative Commons Attribution 4.0 International License, which permits use, sharing, adaptation, distribution and reproduction in any medium or format, as long as you give appropriate credit to the original author(s) and the source, provide a link to the Creative Commons licence, and indicate if changes were made. The images or other third party material in this article are included in the article's Creative Commons licence, unless indicated otherwise in a credit line to the material. If material is not included in the article's Creative Commons licence and your intended use is not permitted by statutory regulation or exceeds the permitted use, you will need to obtain permission directly from the copyright holder. To view a copy of this licence, visit http://creativecommons.org/licenses/by/4.0/.
About this article
Cite this article
Bánó, B., Takács, P. Effects of the analysed variable set composition on the results of distance-based morphometric surveys. Hydrobiologia 849, 2267–2280 (2022). https://doi.org/10.1007/s10750-022-04864-2
Received:
Revised:
Accepted:
Published:
Issue Date:
DOI: https://doi.org/10.1007/s10750-022-04864-2