Abstract
Contemporary science on how livestock influence nutrient cycling in grazing systems is limited, particularly in topographically complex (i.e., slopes and aspects) hill country landscapes. Prominent slope and aspect variation affects primary production, animal behaviour and nutrient return. Here, we embed recent scientific advancements in nutrient dynamics across complex landscapes to (1) set up a soil organic carbon (SOC) saturation function to an existing SOC and total soil phosphorus (TSP) model (Bilotto et al. J N Z Grassl 81:171–178, 2019. https://doi.org/10.33584/jnzg.2019.81.397), (2) include total soil nitrogen (TSN) dynamics, and (3) establish if the model (herein the Grass-NEXT model) can simulate the spatial and temporal changes of TSP, SOC and TSN in hill country. A long-term P fertiliser experiment with contrasting different P fertilisation levels and associated sheep stocking regimes (herein, ‘farmlets’) was used for model testing. The Grass-NEXT model predicted TSP and SOC stocks with strong accuracy and precision (model performance), and TSN with a moderate performance across farmlets [Concordance Correlation Coefficient (CCC), 0.75, 0.72 and 0.49, respectively]. Grass-NEXT model simulated TSP, SOC and TSN distribution with moderate/strong performance across slopes (CCC, 0.94, 0.80 and 0.70) and aspects (CCC, 0.83, 0.67 and 0.51). Consistent with observed data, modelled changes in TSP and TSN were greater on low slopes and eastern aspects, but no clear pattern was observed for SOC stocks. The Grass-NEXT model provides an intuitive research tool for exploring management options for increasing SOC and TSN, as well as an instrument for monitoring and reporting on nutrient dynamics in complex landscapes.
Similar content being viewed by others
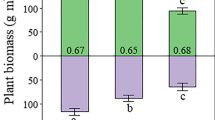
Avoid common mistakes on your manuscript.
Introduction
Globally, grasslands comprise a key land use vegetation cover, accounting for 26% of the ice-free land area (with about a third of this area in managed grasslands), store up to 343 Pg soil organic carbon (SOC) to 1 m depth (about 20% of the world’s total soil carbon stock) and a rate of sequestration of 0.5 Pg C year−1 (Lorenz and Lal 2018; Harrison et al. 2021). The availability of macro-nutrients, such as phosphorus (P) and nitrogen (N), are considered major drivers of the C cycle in grasslands soils (van Groenigen et al. 2017; Poeplau et al. 2018). While some studies have revealed that sustained P fertiliser inputs to temperate pastures increase soil C sequestration (Chan et al. 2010; Coonan et al. 2019), others have shown negligible effects (Cayley et al. 2002; Schipper et al. 2011; Condron et al. 2012; Young et al. 2016; Whitehead et al. 2018; Mackay et al. 2021a) or even a reduction of SOC stocks (Sochorová et al. 2016), especially under conditions of limited soil N (van Paassen et al. 2020). Including the effect of P fertiliser on soil N availability (from biological N fixation and other sources) might provide for a better understanding of the effects of P fertiliser on the cycling of C and SOC sequestration in grazed systems (Christie et al. 2018, 2020; Bilotto et al. 2021).
Several grassland models each in pursuit of specific research questions have been developed to simulate P, SOC and N dynamics in grazed systems [e.g., CENTURY (Parton 1996), DayCent (Parton et al. 1998; Del Grosso et al. 2008), DairyMod (Christie et al. 2018, 2020), APSIM (Holzworth et al. 2014)]. Some models focus on improving the representation of biochemical, biophysical and ecosystem processes in natural grasslands [e.g., Grassland Ecosystem Model GEM (Fitz et al. 1996)], some on describing C, N and water cycles in grazed systems [e.g., Hurley Pasture Model (Thornley 1998), PaSim (Riedo et al. 1998), Soil Plant Atmosphere System Model SPASMO (Cichota and Snow 2009)] or on simulating multiple grass species which compete for water and nutrients [e.g., DNDC (Li et al. 2012)]. Soil models such as RothC (Coleman et al. 1997; Jenkinson et al. 2008, Jenkinson and Coleman 2008) require relative stock change factors based on land use, land management, soil type and climatic region (weather data), and monthly input of plant residues and livestock excreta. As far as we are aware no model captures the transfer and spatial redistribution of P, C and N from animal excreta in grazed agroecosystems. In addition to the factors above, animal grazing and excreta return is also influenced by topography, botanical composition, and proximity to shelter, shade and water (Langworthy et al. 2018; Chen et al. 2021). All these are conducive to greater spatial variability in excreta return as the land surface slope increases, which can also increase the variability in micro-climatic and agro-ecological conditions (Cottle et al. 2016).
New Zealand hill country landscapes (slopes > 15º) cover 4.1 million ha, accounting for more than 50% of the country’s permanent grasslands and are the base for the sheep and beef pastoral industry (Kemp and López 2016). Modelling efforts to capture long-term soil nutrient dynamics under hill country grazing in New Zealand have been reported (Saggar et al. 1990; Hoogendoorn et al. 2011), but none of these reports capture the complex relationships between soil P, SOC and N stocks in soil and their interaction with topography. To account for some of these factors, Bilotto et al. (2019) built a spatial, process-based P and C cycling model following the P and sulphur (S) model of Saggar et al. (1990) and the C and N models by Hoogendoorn et al. (2011). In addition to slope, the model developed by Bilotto et al. (2019) accounted for aspect [the main compass direction (East, Northwest, Southwest) that each slope faces], increased soil depth (from 150 to 300 mm) to better account for P movement down the profile (Mackay and Costall 2016), and to explore temporal changes in P and SOC stocks and flows in these systems over time. The addition of aspect, extra soil depth and time components allowed for an improved prediction of P dynamics compared with the original model of Saggar et al. (1990). The model, however, overestimated SOC stocks to a 300 mm depth (Bilotto et al. 2019) compared with observed data (Mackay et al. 2021a). The objectives of this study are to i) include a C saturation function in the SOC model developed by Bilotto et al. (2019), ii) expand the model development to include the N dynamics of the grazed system (i.e., N pools and flows), and iii) establish if the new Grassland Nutrient EXplorer and Transfer (Grass-NEXT model) model can simulate the spatial distribution and temporal changes of P, SOC and N simultaneously in hill country as influenced by contrasting P fertiliser and associated sheep stocking and topography (slopes and aspects) over time.
Materials and methods
Field location and topography
The Ballantrae Hill Country Research Station is in southern Hawke’s Bay (40° 18′ S 175° 50′ E), about 300 m.a.s.l. with mean long-term annual air temperature and rainfall of 12.8 °C and 1270 mm, respectively. The soils are Andic Distrochrepts, Typic Distrochrepts, and Typic Eutrochrepts with a silt-loam texture (Lambert et al. 2000). The farmlets included in this study are part of a larger study described in Lambert et al. (2000). Three self-contained farmlets were examined that have been under different fertiliser and sheep grazing regimes since 1980:
-
NF (9.7 ha): 0 kg single superphosphate (SSP; 0-9-0-11N-P-K-S) ha−1 year−1.
-
LF (8.1 ha): 125 kg SSP ha−1 year−1.
-
HF (6.8 ha): 375 kg SSP/ha−1 year−1.
The farmlets have been continuously grazed with breeding ewes since 1975. Farmlets are stocked with sheep to maintain similar grazing pressure across farmlets [i.e., similar stock units (SU) carried per unit of net herbage accumulation (NHA)]. Long-term (1980–2014) annual mean stocking rates were 6.0, 10.6, and 16.1 SU ha−1 for the NF, LF, and HF farmlets, respectively (1 SU = 550 kg dry matter (DM); Woodford and Nicol 2004). For a thorough description of the farmlets and livestock carried, see Mackay et al. (2021a).
Three slope classes and three aspect locations were used to describe the topography of the landscapes within the farmlets: Low (LS; 0°–12°), Medium (MS; 13°–25°) and High slope (HS; > 25°) on different aspects grouped relative to the true north as East (E; 35–155°), Northwest (NW; 155°–275°) and Southwest aspect (SW; 275°–35°) (Fig. 1). Land area in each slope and aspect combination (expressed as a proportion of the total area in each farmlet) is described in Table 1.
Topographic representation of the long-term P fertiliser and sheep grazing experiment design at AgResearch Ballantrae Hill Country Research Station, North Island, New Zealand. a Slopes classes: green, yellow and red depict low (0°–12°), medium (13°–25°) and high (> 25°) slopes, respectively; b Aspect classes: red, blue and yellow depict East (E; 35°–155°), Southwest (SW; 275–35°) and Northwest aspect (NW; 155–275°); c Altitude in meters and digital contour map; d Images from AgResearch Ballantrae long-term experiment. Farmlets: NF: no single superphosphate (SSP) applied, LF: 125 kg SSP ha−1 year−1, HF: 375 kg SSP ha−1 year−1, since 1980. (Color figure online)
Soil samples from each farmlet were collected from permanently marked sites to a depth of 300 mm in 2003 and in 2020. A brief description of soil sampling procedures and soil sample analyses is presented in the supplement (Online Resource 1). The effect of farmlet, topographical feature (slope class and aspect location) and sampling year (and their interactions) on P, C and N concentrations, and P, C, and N stocks were subject to analysis of variance (ANOVA) according to a split-split-plot design (Online Resource 1). For more information on soil sampling procedures and sample analysis see Mackay et al. (2021a, b).
Model development
The new Grassland Nutrient EXplorer and Transfer (Grass-NEXT) model was built using the web-based, general-purpose simulation and modelling tool Insight Maker (Fortmann-Roe 2014). The model recognises the inherent differences in a wide range of soil attributes and the influence of topography on primary production and animal behaviour, as well as how these affect the dynamics of the biogeochemical cycles of P, C and N soil stocks over time (Saggar et al. 1990; Hoogendoorn et al. 2011; Bilotto et al. 2019). The effect of aspect as it influences primary production (Lambert and Roberts 1978) and animal behaviour including grazing, camping, and nutrient return (López et al. 2003a), were added to this framework. Mackay and Costall (2016) reported that under high P fertiliser inputs, P was found in significant amounts in the 75–150 mm soil depth. This prompted the need to extend soil depth of modelling of P to 300 mm, which is also in agreement and harmonizes with the soil depth used in global reporting on changes in SOC stocks (FAO 2018).
A similar approach to that of Saggar et al. (2015) was used to account for the effect of spatial variability on nutrient excreta return from livestock. Equations that relate to slope classes were generated and adapted to aspects based on Saggar et al. (2015). In addition to those published in Bilotto et al. (2019), model parameters and equations used to calculate changes in soil P stocks (herein total soil P; TSP) and SOC stocks to a depth of 300 mm are in the supplement (Online Resource 2 and 3, respectively). Model parameters and equations used to calculate soil N stocks (herein total soil N; TSN) to a depth of 300 mm are also in the supplement (Online Resource 4).
Accounting for soil carbon saturation
The physical capacity of a soil to retain soil organic matter (SOM) is determined by the amount of C associated with the clay and silt fractions in the soil. This in turn determines the amount of newly added C in plant root and plant and animal litter that is retained after decomposition (Hassink and Whitmore 1997). As such, a C saturation function was added to the C model parameters of Bilotto et al. (2019) leading to an equilibrium of SOC stocks in response to limitations of soil properties and climate, as opposed to the continuous accumulation of SOC stocks over time. The addition of a C saturation function had been signaled, as a prior iteration of the model predicted C accumulation over time across all three farmlets (Bilotto et al. 2019), when field measurements (herein observed data) of SOC stocks have shown minimal change over the last four decades (Lambert et al. 2000; Mackay et al. 2021b).
Plant and soil respiration, and SOC stock decomposition depend on the current level of SOC stocks and C saturation level (Kemanian and Stöckle 2010; Pravia et al. 2019), according to the following:
where \({\mathrm{SOC}}_{{sa}_{i-1}}\) is annual SOC change and the components Clitter, Cexc and Croot and rhizo represent C inputs from plant litter, C returned as dung and C from root and rhizodeposition weighted by land proportion (Table 1) for slope and aspect combination (sa) and expressed in Mg C, respectively. Model parameters and equations used to calculate soil C stocks (herein total SOC) to a depth of 300 mm are also in the supplement (Online Resource 3).
The term \({\mathrm{ks}}_{sa}\) is the use as saturation ratio to regulate SOM turnover rate, which can be expressed as:
where \({\mathrm{kx}}_{sa}\) is the maximum undisturbed C decomposition rate, \({\mathrm{ft}}_{sa}\) is a tillage factor, \({\mathrm{fs}}_{sa}\) is a factor that accelerates the turnover as the saturation ratio increases, and \({\mathrm{fe}}_{sa}\) is an environmental factor.
dr = soil disturbance factor.
Long-term pastures have a null impact, a tool or operation rated lower for dr gently disturbs the soil, while a tool rated higher aggressively breaks up and mixes soil aggregates (Kemanian and Stöckle 2010).
\({f}_{clay}=0.2\), which represents the clay concentration to a 300 mm depth.
where \({SOC}_{s}\) and \({SOC}_{x}\) are current SOC concentration and maximum SOC concentration (or soil C saturation), respectively.
Assuming a SOC saturation deficit of 15 mg g−1 (top 150 mm) for soils in New Zealand pastures (McNally et al. 2017) and considering initial conditions for SOC stocks and bulk density from Mackay et al. (2021b) extended to a 300 mm depth, the SOC saturation deficit (SOCx def) for the soils of the long-term P fertiliser and sheep grazing experiment at Ballantrae was calculated as:
The SOC saturation (SOCx, in Mg ha−1) or maximum SOC concentration was:
The term hc refers to the organic humification rate, which represents the SOC gain due to plant residue decomposition and depends on the saturation ratio. When the soil is near saturation, the fractional stabilisation of microbial detritus into SOC or hc is 0 approaching the following equation:
where hx is the maximum hc.
It is worth noting that SOC deficits and potential SOC stocks can be altered by certain factors such as soil type (defining factors), climate (limiting factors) and farm management (reducing factors) (Ingram and Fernandes 2001).
Modelling nitrogen dynamics
To increase our understanding of the influence of the interplay between P, C and N processes in hill country landscapes, N dynamics were simulated using data from the most relevant national and international studies published in peer-reviewed journals (Fig. 2, Online Resources 2–4). Bowatte et al. (2006) have revealed that despite large P fertiliser applications and the inputs of legume N, New Zealand hill country pastures exhibited N deficiencies. The biological N fixation (Nfix) is a product of clover DM production, total N concentration and the proportion of legume N fixed from the atmosphere (Ledgard and Steele 1992; Rawnsley et al. 2019). The levels of Nfix through clover and other legumes vary among pastures with different botanical composition, grazing management, topography, soil quality and climate across the country (Hoglund and Brock 1978; Lambert et al. 1986; Lambert 1987). The amount of Nfix observed in unimproved hill country pastures is around 13 kg N ha−1 year−1 (Grant and Lambert 1979) to 185 kg N ha−1 year−1 for improved lowland pastures (Hoglund et al. 1979). Ledgard et al. (1987) suggested different proportionality constant values for Nfix at low slopes (Nfix-low slope, 0.03 kg N fixed × kg DM clover production) and sloping sites (Nfix-med or high slope, 0.04 kg N fixed × kg DM clover production which were assumed for the modelling purposes (Online Resource 4). Moreover, the sum of N fixation by non-symbiotic organisms and atmospheric deposition can be as important as legume N fixation. According to Lambert et al. (1982), non-symbiotic N fixation (Nnon-symb) can be assumed as 13 kg N ha−1 year−1 and the atmospheric N deposition by rain (Nrain) contribute with an extra 3 kg N ha−1 year−1. Considered as the main soil N output, the amount of plant N uptake (Nuptake) by pasture across slope and aspect combination was calculated from annual NHA and herbage N concentration. The values for herbage N concentration per farmlet, and slope class were taken from published data (Bowatte et al. 2006; Hoogendoorn et al. 2011). The calculation process was carried out by subtracting the contribution of Nfix from the herbage N pool (see Online Resource 4).
The proportion of pasture utilisation for each slope and aspect combination was used to estimate the amount of pasture N intake by the animals (Nintake) and the N returned to the soil through N plant litter (Nlitter). The proportion of dietary N removed as animal product (Nap) was assumed to decrease as the N concentration of the diet increases, while the remainder of the N intake was excreted (Ledgard et al. 2003). According to Pacheco et al. (2018), N partitioning in dung (Ndung) and urine (Nurine) will directly depend on the N concentration of the diet and total N intake. Higher N intake and herbage N content would increase N partitioned to Nurine.
Ammonia (NH3) volatilisation (Nvolatilisation) occurs from fertilisers, soils, fresh and stored animal excreta in grazing systems (Smith et al. 2021). Bowatte (2003) identified Nvolatilisation as a major pathway of N loss from urine patches in hill country pastures; these values ranged from 21 to 51% of the N in the urine patch. This model assumed that 33% of N excreted as urine is lost by Nvolatilisation from hill pasture. Based on the work of Hoogendoorn et al. (2011), N2O emissions (NNitrous Oxide) were assumed proportional to inputs of N in urine and dung with a proportionality constant that varied according to slope. To reflect the lower emission rates observed on MS and HS, the emissions of NNitrous Oxide were assumed to be 0.5% of urine deposited on LS and 0.08%, on MS and HS (van der Weerden et al. 2020). In addition, based on the low nitrification rates observed from hill country (Bowatte 2003), the model assumes that 30% of the N excreted as urine is leached from LS (< 12°), and no leaching losses occur from MS and HS (Parfitt et al. 2009; Hoogendoorn et al. 2011). Although the mineral N pool may fluctuate from day to day, net mineralisation (NMin) in the model was calculated on an annual basis as the difference between the inputs to the mineral N pool (urine, atmospheric deposition) and the losses from that pool [Nuptake, NNitrous Oxide, Nvolatilisation and leaching (Nleach)].
Net herbage accumulation, herbage intake and utilisation
Annual NHA (kg DM ha−1) in response to changes in Olsen P values of the three farmlets (NF, LF, HF), slope classes (LS, MS, HS) and aspect locations (E, SW, NW) were simulated for the period 2003–2020 using data collected on site (Lambert et al. 2014; Mackay and Costall 2016; Mackay et al. 2021a). To represent this relationship, equations were developed with a triangular distribution fitted between the upper and lower limits estimated for the 95% confidence interval. According to Lambert et al. (1983), E was the most productive aspect over the nine-year period from 1972/73 to 1980/81 (9325, 9003 and 8388 kg DM ha−1 year−1 for E, SW and NW aspects, respectively).
According to López et al. (2003b), low and medium slopes have a higher probability of defoliation than steeper slopes. To represent animal behaviour, the probability of pasture defoliation for low (PDlow), medium (PDmed) and high slope (PDhigh) were assumed to be 0.48, 0.30 and 0.22, respectively. To keep selective defoliation constant, the allocation of Pastint was linked to the composition of slope and aspect area per farmlet. Pasture utilisation (PU, %) for each slope and aspect combination was calculated as the ratio between Pastint and NHA weighed by area (Online Resource 3 and 4).
Model evaluation
The model was evaluated by comparing observed vs predicted values obtained at the same time for TSP, SOC and TSN (in Mg ha−1 year−1) to a depth of 300 mm, across farmlets, and within slope and aspect classes. Observed field data on soil C and N concentration, and SOC and TSN stocks for each of three slope classes and aspect locations within farmlets have been published elsewhere (Mackay et al. 2021b), and a summary of this information is presented in Table 2. The association between observed and predicted values was assessed using the statistics concordance correlation coefficient (CCC; Lin 1989), Pearson correlation coefficient (ρ), and bias correction factor (Cb), as described in Tedeschi (2006). Briefly, the CCC evaluates the degree to which observed and predicted data pairs fall on a 45° line, and provides a measure of both accuracy and precision, and it is calculated as CCC = ρ × Cb, where ρ is a measure of precision and Cb is a measure of accuracy. The correlation coefficient ρ (also expressed as r) is a measure of how far each observation deviates from the best-fit line, whereas Cb is a measure of how far the best-fit line deviates from the 45° line through the origin (Tedeschi 2006). Values of CCC closer to 1 indicate a better performance of the model (Lin 1989).
The significance test of ρ provided by the statistical package in R was performed to decide if the correlation coefficients ρ were “close to zero” or “significantly different from zero” (R Core Team 2022). If the p-value is less than the significance level (α = 0.05), there is evidence to conclude that a significant linear relationship between observed and predicted data exists and ρ is significantly different from zero. The analysis of CCC does not consider significance test but the interpretation of the absolute magnitude of the observed CCC can be described as for Pearson correlation coefficient (ρ) with < 0.2 as poor, > 0.2 and < 0.6 as moderate, > 0.6 and < 0.8 as strong and > 0.8 as very strong correlation between variables (Akoglu 2018).
Results
Changes in observed soil phosphorus, organic carbon, and nitrogen stocks
Annual application of SSP rates increased TSP stocks (Table 2 and Online Resource 1). TSP stocks showed no change (853 to 841 kg ha−1), to a small (875 to 946 kg ha−1) and large increase (1331–1620 kg ha−1) on the NF, LF and HF farmlets, respectively, from 2003 to 2020 (Table 2). Both slope class and aspect location had significant (P < 0.001) effects on TSP stocks. These were greatest on LS (1333 kg ha−1) and least on HS areas (852 kg TSP ha−1) and were greatest on E (1187 kg ha−1) compared with the other two aspects (1070 and 977 kg ha−1 on SW and NW, respectively) (Online Resource 1).
Farmlet (as a combined effect of P fertilisation and livestock regime) and time (year of sampling) had minimal impacts (P > 0.5) on SOC sequestration, but topographic features such as slope and aspect had a sizeable impact (P < 0.001) on SOC accumulation (Table 2 and Online Resource 1). Similarly, TSN stocks were similar across farmlets and time (P > 0.5; 8.8 Mg ha−1), but again varied significantly (P < 0.001) with slope and aspect. These were greater on LS and MS (9.5 Mg ha−1) than on HS areas (7.4 Mg ha−1), and greater on E and SW (9.3 Mg ha−1) than on NW aspects (7.8 Mg ha−1) (Table 2 and Online Resource 1).
Model performance across farmlets, slopes and aspects
Overall, the precision and accuracy of the model for exploring changes in TSP, SOC and TSN stocks across the farmlets was moderate to strong, with CCC values of 0.75, 0.72 and 0.49, respectively (Fig. 3a–c). While the model predicts TSP and SOC stocks with high accuracy (Cb ≥ 0.8) and precision (ρ > 0.7) (Fig. 3a, b), the biases in predicting TSN affected CCC values (Fig. 3c). Despite the strong to very strong precision and accuracy of the model for predicting TSP stocks with slopes and aspects across the farmlets, the model overestimated TSP accumulation in the HF farmlet (blue symbols in Fig. 3a).
Concordance correlation coefficient (CCC), Pearson correlation coefficient (ρ), and bias correction factor (Cb) of observed vs predicted total soil P (TSP; a, d, g), soil organic carbon (SOC; b, e, h) and total soil nitrogen (TSN; c, f, i) stocks to 300 mm depth across farmlets, slope classes and aspect locations. In all cases, p is significant different from zero (p < 0.05). Farmlets: NF: no single superphosphate (SSP) applied; LF: 125 kg SSP ha−1 year−1; HF: 375 kg SSP ha−1 year−1, since 1980. Slope classes: LS: low slope (0°–12°), MS: medium slope (13°–25°), HS: high slope (> 25°). Aspect locations: E: East aspect (35°–155°), SW: Southwest aspect (275°–35°), NW: Northwest aspect (155°–275°); degrees relative to the true north. TSP, SOC and TSN stocks and across farmlets, slope and aspect combinations (a–c; n = 27), farmlets and slopes (d–f; n = 9), and farmlets and aspects (g–i; n = 9). (Color figure online)
Topographically, the model provided a range of predictions, from a strong prediction of TSP stock changes by slope [CCC, p and Cb values of 0.94, 0.99 and 0.95, respectively (Fig. 3d)], to a moderate prediction of TSN stock changes by aspect (corresponding CCC, p and Cb values of 0.51, 0.75 and 0.68, respectively) (Fig. 3i). The latter can be explained by an overestimation of TSN stocks in the SW and E aspects and an underestimation of TSN stocks in the NW aspect. Moderate to strong predictions were observed for SOC stocks by slope (Fig. 3e) and aspect (Fig. 3h).
Modelled phosphorus, organic carbon and nitrogen dynamics across farmlets
The contrasting annual amounts of P fertiliser applied, and associated sheep stocking regimes had a minimal effect on SOC and TSN stocks (< 0.4% change per year), with decreasing trends across the farmlets (Fig. 4). The highest P fertilisation level, which has seen a 35% increase in TSP stocks over the last 17 years (HF farmlet), limited the decline in the SOC balance and TSN losses compared to the LF farmlet, with no significant changes in the C:N ratio (Fig. 4, Online Resource 5a, 5b, 5c). Interestingly, no P fertilisation (NF farmlet) has slightly decreased SOC stocks while maintaining TSN levels; this combined effect can be seen in the slight downward trend of the C:N ratio.
Modelled annual mean phosphorus (P) in green, carbon (C) in red and nitrogen (N) in purple dynamics (pools and flows) and changes in soil P, C and N across farmlets. a NF: no single superphosphate (SSP) applied; b LF: 125 kg SSP ha−1 year−1; c HF: 375 kg SSP ha−1 year−1, since 1980. The parameter and symbol are listed and explained in the Online resources 2–4. (Color figure online)
Phosphorus fertiliser applied and sheep stocking regime affected NHA (8.2, 8.8 and 12.6 Mg DM ha−1 for NF, LF and HF, respectively) and nutrient dynamics of N and C (Fig. 4). Fertiliser represented 43% and 48% of P inputs in LF and HF, respectively. The higher P fertilisation rates has almost tripled P losses in the HF farmlet, and P removed by animal products (Prap). However, the HF farmlet has almost doubled the P recovered from plant litter (Plitter) and dung (Pexc) compared with the LF and NF farmlets, respectively.
Although the highest amount of soil C allocated from roots and rhizodeposition (Croot and rhizo) was observed in the HF farmlet, the proportion of C allocated to soils slightly decreased as the P fertility and livestock carrying capacity was increased across the farmlets (52–56%) (Fig. 4). The flux of Croot and rhizo into the soil has shown to be the main source of soil C stocks (62–68%), followed by litter decomposition (Clitter, 20–29%) and C from dung (Cexc, 9–13%). But with higher NHA, the levels of Cintake, Cmethane, and C respired by the animals (Cres-a) increased, leaving similar amounts of Clitter and C fluxes through plant and soil respiration (Cres-p), which showed an exponential behaviour (the latter accounts for 80–85% of total C losses).
The increasing levels of TSP stocks were correlated with legume N fixation (N fix) and N mineralisation per hectare (NMin) (Fig. 4). Moreover, higher stocking rates and NHA in the HF farmlet doubled the amount of N intake, N retained as animal product (Nap) and N excreted (Nurine and Ndung) compared with the NF farmlet. Partitioning of animal excreta into urine varied between 58–60% based on the level of Nintake and N concentration of the herbage. In addition, N losses in the HF farmlet (Nvolatilisation, NNitrous Oxide and Nleach) were two-fold greater than those simulated for the NF farmlet.
Modelled soil phosphorus, organic carbon and nitrogen stock dynamics across slopes and aspects
Mean modelled annual changes in TSP stocks were greater on the LS and E aspect than the other two slopes and aspects (Fig. 5a–c), but no clear pattern was observed for annual SOC changes (Fig. 5d–f). Annual changes in TSN stocks (Fig. 5g–i) showed similar trends to TSP stocks. The net loss of TSP from the MS and HS classes and NW aspect (Fig. 5) is most likely a consequence of the transfer of P in animal dung from MS and HS areas to LS areas, and the amount of dung returned to the different aspect locations, respectively. The spatial distribution of P, C and N excreted showed that 61%, 29% and 10% were deposited on LS, MS and HS areas, respectively, with a similar pattern across the three farmlets. In terms of aspect positions, about 50%, 33% and 17% of P, C and N excreted were returned on the E, SW and NW aspects, respectively (data not shown).
Modelled mean annual total soil phosphorus (TSP; a–c), soil organic carbon (SOC; d–f) and total soil nitrogen (TSN; g–i) changes across slope class and aspect location for the different farmlets. Farmlets: NF: no single superphosphate (SSP) applied, LF: 125 kg SSP ha−1 year−1, and HF: 375 kg SSP ha−1 year−1, since 1980. Slope classes: LS: low slope (0°–12°), MS: medium slope (13°–25°), HS: high slope (> 25°). Aspect locations: E: East aspect (35°–155°), SW: Southwest aspect (275°–35°), NW: Northwest aspect (155°–275°); degrees relative to the true north. (Color figure online)
The simulated NHA on the different slope class areas (14.0 ± 2.1, 10.0 ± 2.6 and 6.7 ± 2.2 Mg DM ha−1 for LS, MS and HS, respectively) resulted in higher proportion of C partitioning and translocation to Croot and rhizo at the HS areas (55% at HS vs 51% at LS, Online Resources 6a, 6b, 6c). Moreover, in LS and HS areas, Cres-p was up to 41% higher and up to 34% lower, respectively, than the average obtained across the farmlets (Fig. 4 and Online Resources 6a, 6b, 6c). In addition, the E aspect had a large proportion of C that remained in the above ground component (45–48% as Cshoot) and the NW aspect had the lowest Cres-p (24% below the average obtained across the farmlets) (Fig. 4 and Online Resources 6a, 6b, 7c).
Despite the increase of TSP, an inverse relationship was observed in TSN levels across the farmlets (Fig. 4). Within the farmlets there were similarities between the sites of increased annual TSP deposition and TSN changes (Fig. 5). No clear trend was observed in the TSN changes between aspects. There was an excess of N returned to LS areas. Most of the annual N losses occurred in MS, with N inputs barely covering N outputs in HS areas (Fig. 5g–i). The LS areas annual N input levels were from two to three, and four times greater than those observed on MS and HS areas, respectively (Online Resources 6a, 6b, 6c). While Nlitter was the main source of N inputs for MS and HS (32–63%), N excreta became the main source of N in LS areas (46–76% of N inputs). The MS areas were high Nfix suppliers (32–69% of N fix across the farmlets) with large values per ha (11.5–61.7 kg N ha−1). Interestingly, this amount of N only represented 6–19% of N inputs across farmlets for this slope class. The N losses modelled for LS were two to four times higher than those observed at MS and HS areas, respectively (Online Resources 6a, 6b, 6c). Pasture Nuptake was the main source of N outputs, being more important in relative terms at MS and HS (> 90% of N outputs) than LS areas (70–80%). Moreover, more than 50% of N emissions (Nvolatilisation and NNitrous Oxide) occurred on LS areas.
Discussion
Modelling integrated phosphorus, carbon and nitrogen cycles
The Grass-NEXT model was able to simultaneously simulate with high (TSP and SOC) to moderate (TSN) accuracy and precision stock change and distribution in a topographically complex (hill country) landscape, as influenced by contrasting P fertiliser and sheep stocking regimes from 2003 to 2020 (Fig. 3). Modelled changes in TSP distributed across hill country landscapes were a reasonable approximation of actual changes in TSP stocks (Table 2 and Fig. 3) and dynamics across the farmlets in terms of (i) pasture species, Puptake and Pintake; (2) the amount of pasture Plitter; and (3) the amounts of Pexc spatially deposited on slope and aspect combinations. Extension of the modelling of soil P to a 300 mm depth and the simulation of a longer time-period increased the precision of the model compared with the original version of the nutrient transfer model (Saggar et al. 1990). While the nutrient transfer model developed by Saggar et al. (1990) explained 89% and 79% (p, Pearson's correlation coefficient) of the variation between slopes and slope-aspect combinations across farmlets, corresponding TSP predictions in this study explained 99% and 87% of their variation (Fig. 3).
In comparison with the previous version of the C model (Bilotto et al. 2019), the addition of a C saturation component increased accuracy and precision of simulated SOC dynamics across farmlets, and topographically within slope classes (CCC’s values from 0.67 to 0.80) and aspect positions (CCC’s values from 0.47 to 0.67), respectively (Fig. 3). The N component of the model consistently calculated annual TSN stock changes in the LF and HF farmlets, capturing the observed trend towards TSN depletion over time (Fig. 4, Table 2).
Consistent annual improvements in soil P fertility can simultaneously increase soil P availability, NHA (Mackay and Lambert 2011; Lambert et al. 2014) and legume content in the sward (Sakadevan et al. 1993; Gillingham et al. 1998; Parfitt et al. 2005; Rawnsley et al. 2019). On the latter, legume contents sampled (expressed as a proportion of edible sward DM; mean ± SE) were 0.039 ± 0.005, 0.036 ± 0.013, and 0.125 ± 0.027 on the NF, LF and HF farmlets, respectively, during the 2016–2021 period (data not shown). While annual TSP stock change rates and animal production were dependent on P loading rate, this effect did not translate into significant temporal increases in SOC and TSN stocks (Fig. 4). In agreement with our findings, Schipper et al. (2011) did not find evidence to support that P fertiliser loading in grazing system altered SOC or TSN stock changes. Condron et al. (2012) also reported similar SOC stocks to a depth of 1 m across varying P fertiliser rates. These findings were attributed to greater stocking rates (Schipper et al. 2011) and a faster rate of SOM decomposition associated with greater herbage quality and earthworm activity (Condron et al. 2012) from an enhanced aboveground herbage biomass attained from P addition. Often, the cycling of macronutrients such as P, C, and N in grazing system is coupled through biologically mediated soil and plant processes involved in SOM turnover (Dungait et al. 2012), such that the extent of nutrient cycling can be closely related to CO2 fluxes (Rawnsley et al. 2016). However, as TSP is progressively increased, SOC and TSN concentrations ruled by isometric stoichiometry appear to be progressively decoupled from TSP concentrations (Cleveland and Liptzin 2007) (Online Resource 5).
The highest predicted C and N inputs were provided via litter turnover and N fix, along with large amounts of dung and urine excreted in the LF and HF farmlets (Fig. 4). Based on international studies conducted on long-term temperate grazing systems, a linear increase of SOC and TSN stocks would be expected in soils with low C levels or after crop rotations (Chan et al. 2010; Coonan et al. 2019). However, under circumstances of near C saturation, large amounts of C and N inputs in long-term pastures could be counterbalanced by an exacerbation of their losses (White et al. 2018; Stoner et al. 2021) or a significant reduction of inputs (Schipper et al. 2011), maintaining or slightly declining SOC (Di et al. 2018) and TSN stocks (Bowatte et al. 2006). On the one hand, soil C saturation suggests that even when increasing C inputs, SOC stocks have reached a maximum level, and the SOC accumulation rate will become asymptotic. In fact, the maximum observed C stocks (Table 2) fluctuated around the plateau predefined in the model for SOC stocks (~ 150 Mg ha−1). During this process, the overall rate of plant respiration and OM decomposition in the soil equals the annual input rate of organic material (Stewart et al. 2007; Stoner et al. 2021). This is consistent with the faster dung disappearance rate found on HF sites by Vibart et al. (2021). On the other hand, any increase in legume content most likely had a small effect on the TSN stocks (Bowatte et al., 2006). Largely driven by increases in pasture intake and total nutrients excreted on pasture, higher livestock stocking rates to maintain similar grazing pressure and residual biomass tend to a shift towards a new equilibrium (Parsons et al. 2013), often with larger N losses to water and the atmosphere (Parfitt et al. 2009; Luo et al. 2019), C released as methane and loss of ground cover (Hoogendoorn et al. 2011; Harrison et al. 2012a, b) (Fig. 4). Therefore, the excess of P fertilisation co-limited by N application and high SOC stocks can lead to a decoupling of C:N:P ratios (Online Resource 5).
Impacts of topography, animal behaviour, and vegetation on soil phosphorus, organic carbon, and nitrogen stocks
While disproportionate TSP and TSN accumulation was observed in LS and E and to a lesser extent SW aspects with nutrient depletion in HS and NW aspects, no clear pattern was observed for annual SOC changes (Fig. 5). The topographical features in hill country landscapes, slope class and aspect location, strong and divergent influences on TSP, SOC and TSN balances, can be attributed to multiple factors. Ruminant grazing and excretal behaviour show that a disproportionate amount of livestock excreta is deposited on LS areas (Saggar et al. 2015). Often, the nutrients taken up by livestock grazing HS pastures are transferred through urine and dung to LS and camping sites (Rowarth et al. 1992; Hoogendoorn et al. 2011). Soil P is an immobile anion generally transferred in animal dung and it is not subject to large losses by leaching or through atmospheric emissions like N or C. In agreement with Rowarth et al. (1992), higher P fertiliser levels and livestock carrying capacity have exacerbated animal P transfer from HS to LS in our study. Inversely related to the prevailing wind direction, sheep tend to camp on E and S aspects with larger amounts of excreta inputs (urine and dung), contributing to higher TSP and TSN stocks which allude to lower soil C:N ratios and a higher potential for mineralisation (Lambert and Roberts 1978). Temperature and moisture regimes by aspect also have a major influence on soil nutrient status (Lambert and Roberts 1976). As it was implicitly assumed in the model, moderate net radiation, soil temperature and potential evapotranspiration in E aspects create the best environment for NHA (Lambert and Roberts 1976; Lambert et al. 1983). With the addition of a SOC saturation function across slopes and aspects, the nutrient distribution by grazing animals is more influential in the redistribution of TSP and TSN than SOC (Fig. 5, Online Resources 6a, 6b, 6c). In line with our spatial analysis, Saggar et al. (1999) reported higher C respiration rates from soils in low slope areas. As well, lower SOC turnover rates have been suggested from observed slower dung disappearance rates on NW-facing slopes (Vibart et al. 2021). The greater capacity for C turnover of plant litter, root biomass and animal dung (Cres-p) with increasing P fertilisation levels may be associated with considerable changes in soil microbial biomass and fungal population size (Parfitt et al. 2010), and earthworm density, abundance and activity (Schon et al. 2019). Therefore, larger amounts of C inputs on low slopes created by higher P nutrient transfer and livestock carrying capacity were quickly vanished by an accelerated nutrient cycling and higher C losses (Mackay et al. 2021a).
According to Bowatte et al. (2006), hill country pastures suffer a chronic N deficiency caused in part by the disproportionate animal N transfer to LS, conducive to high nitrification rates and subsequent N losses. The actual losses would vary according to slope, soil porosity and stocking density. The TSN depletion in high fertility farmlets was boosted through higher herbage N content leading to more N intake converted into urine (Ledgard et al. 2003). The higher partitioning of N excreta into urine was responsible for the higher N losses through N emissions to air and water in LS areas (Online Resources 6a, 6b, 6c), in line with reports from previous studies (Harrison et al. 2016). To increase TSN stocks by stimulating clover growth and N fix, P fertilisers have been frequently applied at higher levels than recommended. However, this study has shown an inverse relationship between TSN and TSP stocks across the farmlets (Fig. 4). Recently, large-scale scale research in New Zealand has focused on urine patches on lower slopes as the main source of N losses to the atmosphere from sheep and beef hill country farms (ammonia volatilisation and N2O emissions) (Saggar et al. 2015; van der Weerden et al. 2020), overlooking the multiple impacts generated by animal behaviour and nutrient dynamics on the remaining hill country landscape. Interestingly, even though the large Nfix levels occurred in MS, these slope areas were the main source of N losses across the farmlets. In combination, the large proportion of N transfer to low slope areas (61% of N excreta return in LS), the significant amount of plant Nuptake to sustain the level of NHA in MS and the relative high N losses compared to HS, exerted a strong mining process in this topographical site (Bowatte et al. 2006; Hoogendoorn et al. 2011). Therefore, soil P fertilisation far in excess of plant growth needs could be considered as a debatable tool to increase SOC stocks in locations with high C concentrations and may potentially deplete TSN levels (Poeplau et al. 2016) by altering its spatial distribution.
Limitations of this study
Although the results obtained from Grass-NEXT model are a reasonable approximation of the TSP, SOC and TSN dynamics across the farmlets, several assumptions had to be made. These included (a) published probabilities of pasture utilisation in different slope classes based on selective grazing (López et al. 2003a, b), (b) 60% of the organic matter ingested was digestible and 40% of C from pasture intake ends up in soil, (c) the incorporation of a C saturation component in the model adapted to hill country conditions has increased accuracy and precision to simulate soil C dynamics but new refinements would include site specific information about soil respiration and SOC decomposition, (d) to better describe SOC in the profile, the main constraint is the absence of multiple SOC pools and depths as described in models like RothC (Coleman et al. 1997; Jenkinson et al. 2008, Jenkinson and Coleman 2008), (e) C root and rhizodeposition were solved by equations using limited datasets in number and scope (Saggar et al. 1997, 1999), and f) herbage N content was predefined by slope and farmlet according to relevant domestic research.
Stronger links between C saturation and N mineralisation, and the inclusion of a microbial pool, could improve TSN estimations in the model (White et al. 2014). Our study has shown lower net mineralisation with slightly lower C:N ratio in the NF farmlet compared to the LF and HF farmlets (Fig. 4 and Online Resource 5). The C:N ratio is often used for estimating net N mineralisation rates but limitations in its predictive capability have been reported (Bonanomi et al. 2019). Lower N mineralisation rates can occur even at low C:N ratios due to low C accessibility by the soil microbiome, limiting decomposition. Further work is required to obtain additional quantitative data for, and examine the sensitivity and behaviour of, each of these variables. Emerging technologies including GPS monitoring and remote monitoring sensing techniques could improve the quantification of grazing activities and excreta deposition (e. g., Plaza et al. 2022).
Conclusions
The process-based Grass-NEXT model provides a basis for accounting, monitoring and reporting on changes in total soil P, organic C and N stocks in response to current management practices in complex grazed systems. The inclusion of aspect, in addition to slope, and the ability to simultaneously simulate soil P, C and N change in a topographically complex landscape has expanded our understanding of the dynamics of these three nutrients in hill country pastures. The addition of a C saturation function improved the model’s ability to simulate SOC stocks change in a topographically challenged landscape (i.e., different slope classes and aspect locations). In agreement with observed data, the contrasting annual amounts of P fertiliser applied and associated sheep stocking regimes were reflected in increasing TSP predicted, but had a minimal effect on SOC and TSN stocks. Further developments of the model are proposed to explore the interactions between P and N fertiliser use efficiency in hill country, the contribution of legumes through biological N fixation, the spatial variability in greenhouse gas emissions and the impacts of predicted future climates on nutrients dynamics in topographically complex landscapes.
References
Akoglu H (2018) User’s guide to correlation coefficients. Turk J Emerg Med 18:91–93. https://doi.org/10.1016/j.tjem.2018.08.001
Bilotto F, Vibart R, Mackay A, Costall D (2019) Modelling long-term changes in soil phosphorus and carbon under contrasting fertiliser and grazing management in New Zealand hill country. J N Z Grassl 81:171–178. https://doi.org/10.33584/jnzg.2019.81.397
Bilotto F, Harrison MT, Migliorati MDA, Christie KM, Rowlings DW, Grace PR, Smith AP, Rawnsley RP, Thorburn PJ, Eckard RJ (2021) Can seasonal soil N mineralisation trends be leveraged to enhance pasture growth? Sci Total Environ 772:145031. https://doi.org/10.1016/j.scitotenv.2021.145031
Bonanomi G, Sarker TC, Zotti M, Cesarano G, Allevato E, Mazzoleni S (2019) Predicting nitrogen mineralization from organic amendments: beyond C/N ratio by 13C-CPMAS NMR approach. Plant Soil 441:129–146. https://doi.org/10.1007/s11104-019-04099-6
Bowatte S, Tillman R, Carran A, Gillingham A (2006) Can phosphorus fertilisers alone increase levels of soil nitrogen in New Zealand hill country pastures? Nutr Cycl Agroecosyst 75:57–66. https://doi.org/10.1007/s10705-006-9011-4
Bowatte S (2003) Urine nitrogen in hill country pasture soils. Doctoral thesis, Massey University, New Zealand.
Cayley JWD, McCaskill MR, Kearney GA (2002) Changes in pH and organic carbon were minimal in a long-term field study in the Western District of Victoria. Aust J Agric Res 53:115–126. https://doi.org/10.1071/AR01050
Chan KY, Oates A, Li GD, Conyers MK, Prangnell RJ, Poile G, Liu DL, Barchia IM (2010) Soil carbon stocks under different pastures and pasture management in the higher rainfall areas of south-eastern Australia. Soil Res 48:7–15. https://doi.org/10.1071/SR09092
Chen Y, Guerschman J, Shendryk Y, Henry D, Harrison MT (2021) Estimating pasture biomass using sentinel-2 imagery and machine learning. Remote Sens 13:603. https://doi.org/10.3390/rs13040603
Christie KM, Smith AP, Rawnsley RP, Harrison MT, Eckard RJ (2018) Simulated seasonal responses of grazed dairy pastures to nitrogen fertilizer in SE Australia: pasture production. Agric Syst 166:36–47. https://doi.org/10.1016/j.agsy.2018.07.010
Christie KM, Smith AP, Rawnsley RP, Harrison MT, Eckard RJ (2020) Simulated seasonal responses of grazed dairy pastures to nitrogen fertilizer in SE Australia: N loss and recovery. Agric Syst 182:102847. https://doi.org/10.1016/j.agsy.2020.102847
Cichota R, Snow VO (2009) Estimating nutrient loss to waterways—an overview of models of relevance to New Zealand pastoral farms. N Z J Agric Res 52:239–260. https://doi.org/10.1080/00288230909510509
Cleveland CC, Liptzin D (2007) C:N: P stoichiometry in soil: is there a “Redfield ratio” for the microbial biomass? Biogeochemistry 85:235–252. https://doi.org/10.1007/s10533-007-9132-0
Coleman K, Jenkinson D, Crocker G, Grace P, Klir J, Körschens M, Poulton P, Richter D (1997) Simulating trends in soil organic carbon in long-term experiments using RothC-26.3. Geoderma 81:29–44. https://doi.org/10.1016/S0016-7061(97)00079-7
Condron LM, Black A, Wakelin SA (2012) Effects of long-term fertiliser inputs on the quantities of organic carbon in a soil profile under irrigated grazed pasture. N Z J Agric Res 55:161–164. https://doi.org/10.1080/00288233.2012.662898
Coonan EC, Richardson AE, Kirkby CA, Kirkegaard JA, Amidy MR, Simpson RJ, Strong CL (2019) Soil carbon sequestration to depth in response to long-term phosphorus fertilization of grazed pasture. Geoderma 338:226–235. https://doi.org/10.1016/j.geoderma.2018.11.052
Cottle DJ, Harrison MT, Ghahramani A (2016) Sheep greenhouse gas emission intensities under different management practices, climate zones and enterprise types. Anim Prod Sci 56:507–518. https://doi.org/10.1071/AN15327
Del Grosso SJ, Parton WJ, Ojima DS, Keough CA, Riley TH, Mosier AR (2008) DAYCENT simulated effects of land use and climate on county level N loss vectors in the USA. In: Hatfield JL, Follett RF (eds) Nitrogen in the environment, 2nd edn. Academic Press, San Diego, pp 571–595. https://doi.org/10.1016/B978-0-12-374347-3.00018-4
Di J, Xu M, Zhang W, Tong X, He X, Gao H, Liu H, Wang B (2018) Combinations of soil properties, carbon inputs and climate control the saturation deficit dynamics of stable soil carbon over 17-year fertilization. Sci Rep 8:12653. https://doi.org/10.1038/s41598-018-31028-x
Dungait JAJ, Cardenas LM, Blackwell MSA, Wu L, Withers PJA, Chadwick DR, Bol R, Murray PJ, Macdonald AJ, Whitmore AP, Goulding KWT (2012) Advances in the understanding of nutrient dynamics and management in UK agriculture. Sci Total Environ 434:39–50. https://doi.org/10.1016/j.scitotenv.2012.04.029
FAO (2018) Measuring and modelling soil carbon stocks and stock changes in livestock production systems—Guidelines for assessment (Draft for public review). Livestock Environmental Assessment and Performance (LEAP) Partnership. FAO, Rome, Italy
Fitz HC, DeBellevue EB, Costanza R, Boumans R, Maxwell T, Wainger L, Sklar FH (1996) Development of a general ecosystem model for a range of scales and ecosystems. Ecol Modell 88:263–295. https://doi.org/10.1016/0304-3800(95)00112-3
Fortmann-Roe S (2014) Insight Maker: a general-purpose tool for web-based modeling & simulation. Simul Model Pract Theory 47:28–45. https://doi.org/10.1016/j.simpat.2014.03.013
Gillingham A, Gray M, Smith D (1998) Pasture responses to phosphorus and nitrogen fertilisers on dry hill country. Proc N Z Grassl Assoc 60:135–140. https://doi.org/10.33584/jnzg.1998.60.2319
Grant DA, Lambert MG (1979) V. Unimproved North Island hill country, “Ballantrae.” N Z J Exp Agric 7:19–22. https://doi.org/10.1080/03015521.1979.10426155
Harrison MT, Evans JR, Moore AD (2012a) Using a mathematical framework to examine physiological changes in winter wheat after livestock grazing: 1. Model derivation and coefficient calibration. Field Crops Res 136:116–126. https://doi.org/10.1016/j.fcr.2012.06.015
Harrison MT, Evans JR, Moore AD (2012b) Using a mathematical framework to examine physiological changes in winter wheat after livestock grazing: 2. Model validation and effects of grazing management. Field Crops Res 136:127–137. https://doi.org/10.1016/j.fcr.2012.06.014
Harrison MT, Cullen BR, Tomkins NW, McSweeney C, Cohn P, Eckard RJ (2016) The concordance between greenhouse gas emissions, livestock production and profitability of extensive beef farming systems. Anim Prod Sci 56:370–384. https://doi.org/10.1071/AN15515
Harrison MT, Cullen BR, Mayberry DE, Cowie AL, Bilotto F, Badgery WB, Liu K, Davison T, Christie KM, Muleke A, Eckard RJ (2021) Carbon myopia: the urgent need for integrated social, economic and environmental action in the livestock sector. Glob Chang Biol 27:5726–5761. https://doi.org/10.1111/gcb.15816
Hassink J, Whitmore AP (1997) A model of the physical protection of organic matter in soils. Soil Sci Soc Am J 61:131–139. https://doi.org/10.2136/sssaj1997.03615995006100010020x
Hoglund JH, Brock JL (1978) Regulation of nitrogen fixation in a grazed pasture. N Z J Agric Res 21:73–82. https://doi.org/10.1080/00288233.1978.10427385
Hoglund JH, Crush JR, Brock JL, Ball R, Carran RA (1979) Nitrogen fixation in pasture clovers. XII. General discussion. N Z J Exp Agric 7:45–51. https://doi.org/10.1080/03015521.1979.10426162
Holzworth DP, Huth NI, deVoil PG, Zurcher EJ, Herrmann NI, McLean G, Chenu K, van Oosterom EJ, Snow V, Murphy C, Moore AD, Brown H, Whish JPM, Verrall S, Fainges J, Bell LW, Peake AS, Poulton PL, Hochman Z, Thorburn PJ, Gaydon DS, Dalgliesh NP, Rodriguez D, Cox H, Chapman S, Doherty A, Teixeira E, Sharp J, Cichota R, Vogeler I, Li FY, Wang E, Hammer GL, Robertson MJ, Dimes JP, Whitbread AM, Hunt J, van Rees H, McClelland T, Carberry PS, Hargreaves JNG, MacLeod N, McDonald C, Harsdorf J, Wedgwood S, Keating BA (2014) APSIM—evolution towards a new generation of agricultural systems simulation. Environ Model Softw 62:327–350. https://doi.org/10.1016/j.envsoft.2014.07.009
Hoogendoorn CJ, Bowatte S, Tillman RW (2011) Simple models of carbon and nitrogen cycling in New Zealand hill country pastures: exploring impacts of intensification on soil C and N pools. N Z J Agric Res 54:221–249. https://doi.org/10.1080/00288233.2011.599395
Ingram JSI, Fernandes ECM (2001) Managing carbon sequestration in soils: concepts and terminology. Agric Ecosyst Environ 87:111–117. https://doi.org/10.1016/S0167-8809(01)00145-1
Jenkinson DS, Coleman K (2008) The turnover of organic carbon in subsoils. Part 2. Modelling carbon turnover. Eur J Soil Sci 59:400–413. https://doi.org/10.1111/j.1365-2389.2008.01026.x
Jenkinson D, Poulton P, Bryant C (2008) The turnover of organic carbon in subsoils. Part 1. Natural and bomb radiocarbon in soil profiles from the Rothamsted long-term field experiments. Eur J Soil Sci 59:391–399. https://doi.org/10.1111/j.1365-2389.2008.01025.x
Kemanian AR, Stöckle CO (2010) C-Farm: a simple model to evaluate the carbon balance of soil profiles. Eur J Agron 32:22–29. https://doi.org/10.1016/j.eja.2009.08.003
Kemp P, López I (2016) Hill country pastures in the southern North Island of New Zealand: an overview. NZGA Res Pract Ser 16:289–297
Lambert MG (1987) Nitrogen fixation during improvement of North Island hill country pastures. N Z J Exp Agric 15:267–270. https://doi.org/10.1080/03015521.1987.10425570
Lambert MG, Roberts E (1976) Aspect differences in an unimproved hill country pasture. I. Climatic differences. N Z J Agric Res 19:459–467. https://doi.org/10.1080/00288233.1976.10420975
Lambert MG, Roberts E (1978) Aspect differences in an unimproved hill country pasture. II. Edaphic and biotic differences. N Z J Agric Res 21:255–260. https://doi.org/10.1080/00288233.1978.10427407
Lambert M, Renton S, Grant D (1982) Nitrogen balance studies in some North Island hill pastures. Nitrogen Balances N Z Ecosyst. https://doi.org/10.1080/00288233.1985.10430441
Lambert MG, Clark DA, Grant DA, Costall DA, Fletcher RH (1983) Influence of fertiliser and grazing management on North Island moist hill country 1. Herbage accumulation. N Z J Agric Res 26:95–108. https://doi.org/10.1080/00288233.1983.10420958
Lambert MG, Clark DA, Grant DA, Costall DA, Gray YS (1986) Influence of fertiliser and grazing management on North Island moist hill country 4. Pasture species abundance. N Z J Agric Res 29:23–31. https://doi.org/10.1080/00288233.1986.10417970
Lambert MG, Clark DA, Mackay AD, Costall DA (2000) Effects of fertiliser application on nutrient status and organic matter content of hill soils. N Z J Agric Res 43:127–138. https://doi.org/10.1080/00288233.2000.9513414
Lambert MG, Mackay AD, Ganesh S, Upsdell MP (2014) Responses of grazed New Zealand hill pastures to rates of superphosphate application. N Z J Agric Res 57:149–164. https://doi.org/10.1080/00288233.2014.898663
Langworthy AD, Rawnsley RP, Freeman MJ, Pembleton KG, Corkrey R, Harrison MT, Lane PA, Henry DA (2018) Potential of summer-active temperate (C3) perennial forages to mitigate the detrimental effects of supraoptimal temperatures on summer home-grown feed production in south-eastern Australian dairying regions. Crop Pasture Sci 69:808–820. https://doi.org/10.1071/CP17291
Ledgard SF, Steele KW (1992) Biological nitrogen fixation in mixed legume/grass pastures. Plant Soil 141:137–153. https://doi.org/10.1007/BF00011314
Ledgard SF, Brier GJ, Littler RA (1987) Legume production and nitrogen fixation in hill pasture communities. N Z J Agric Res 30:413–421. https://doi.org/10.1080/00288233.1987.10417951
Ledgard SF, Luo J, Monaghan R (2003) Partitioning of excreta nitrogen from grazing animals into urine and dung nitrogen. Report for MAF. Hamilton, New Zealand
Li C, Salas W, Zhang R, Krauter C, Rotz A, Mitloehner F (2012) Manure-DNDC: a biogeochemical process model for quantifying greenhouse gas and ammonia emissions from livestock manure systems. Nutr Cycl Agroecosyst 93:163–200. https://doi.org/10.1007/s10705-012-9507-z
Lin LI-KA (1989) A concordance correlation coefficient to evaluate reproducibility. Biometrics 45:255–268. https://doi.org/10.2307/2532051
López IF, Lambert MG, Mackay AD, Valentine I (2003a) The influence of topography and pasture management on soil characteristics and herbage accumulation in hill pasture in the North Island of New Zealand. Plant Soil 255:421–434. https://doi.org/10.1023/A:1026062502566
López IF, Hodgson J, Hedderley DI, Valentine I, Lambert MG (2003b) Selective defoliation by sheep according to slope and plant species in the hill country of New Zealand. Grass Forage Sci 58:339–349. https://doi.org/10.1046/j.1365-2494.2003.00386.x
Lorenz K, Lal R (2018) Carbon sequestration in grassland soils. In: Carbon sequestration in agricultural ecosystems. Springer, Cham. https://doi.org/10.1007/978-3-319-92318-5_4.
Luo J, Saggar S, van der Weerden T, de Klein C (2019) Quantification of nitrous oxide emissions and emission factors from beef and dairy cattle excreta deposited on grazed pastoral hill lands. Agric Ecosyst Environ 270–271:103–113. https://doi.org/10.1016/j.agee.2018.10.020
Mackay A, Costall D (2016) Long-term changes in soil fertility in hill country. Hill Ctry Grassl Res Pract Ser 16:157–162
Mackay A, Lambert M (2011) Long-term changes in soil fertility and pasture production under no, low and high phosphorus fertiliser inputs. Proc N Z Grassl Assoc 73:37–42. https://doi.org/10.33584/jnzg.2011.73.2848
Mackay AD, Vibart R, McKenzie C, Costall D, Bilotto F, Kelliher FM (2021a) Soil organic carbon stocks in hill country pastures under contrasting phosphorus fertiliser and sheep stocking regimes, and topographical features. Agric Syst 186:102980. https://doi.org/10.1016/j.agsy.2020.102980
Mackay A, Vibart RE, McKenzie C, Devantier B, Noakes E, Bilotto F (2021) The resilience of soil organic carbon stocks under contrasting hill country pasture management practices. Resilient Pastures Grassl Res Pract Ser 17:101–106. https://doi.org/10.33584/rps.17.2021.3449
McNally SR, Beare MH, Curtin D, Meenken ED, Kelliher FM, Calvelo Pereira R, Shen Q, Baldock J (2017) Soil carbon sequestration potential of permanent pasture and continuous cropping soils in New Zealand. Glob Chang Biol 23:4544–4555. https://doi.org/10.1111/gcb.13720
Pacheco D, Waghorn G, Rollo M (2018) Methodology for splitting nitrogen between livestock dung and urine. Final Report. MPI Technical Paper No: 2018/72
Parfitt RL, Yeates GW, Ross DJ, Mackay AD, Budding PJ (2005) Relationships between soil biota, nitrogen and phosphorus availability, and pasture growth under organic and conventional management. Appl Soil Ecol 28:1–13. https://doi.org/10.1016/j.apsoil.2004.07.001
Parfitt RL, Mackay AD, Ross DJ, Budding PJ (2009) Effects of soil fertility on leaching losses of N, P and C in hill country. N Z J Agric Res 52:69–80. https://doi.org/10.1080/00288230909510490
Parfitt RL, Yeates GW, Ross DJ, Schon NL, Mackay AD, Wardle DA (2010) Effect of fertilizer, herbicide and grazing management of pastures on plant and soil communities. Appl Soil Ecol 45:175–186. https://doi.org/10.1016/j.apsoil.2010.03.010
Parsons AJ, Thornley JHM, Newton PCD, Rasmussen S, Rowarth JS (2013) Soil carbon dynamics: the effects of nitrogen input, intake demand and off-take by animals. Sci Total Environ 465:205–215. https://doi.org/10.1016/j.scitotenv.2013.02.019
Parton WJ (1996) The CENTURY model. Springer, Berlin Heidelberg, pp 283–291. https://doi.org/10.1007/978-3-642-61094-3_23
Parton WJ, Hartman M, Ojima D, Schimel D (1998) DAYCENT and its land surface submodel: description and testing. Glob Planet Change 19:35–48. https://doi.org/10.1016/S0921-8181(98)00040-X
Plaza J, Sánchez N, Palacios C, Sánchez-García M, Abecia JA, Criado M, Nieto J (2022) GPS, LiDAR and VNIR data to monitor the spatial behavior of grazing sheep. J Anim Behav Biometeorol 10:2214. https://doi.org/10.31893/jabb.22014
Poeplau C, Bolinder MA, Kirchmann H, Kätterer T (2016) Phosphorus fertilisation under nitrogen limitation can deplete soil carbon stocks: evidence from Swedish meta-replicated long-term field experiments. Biogeosciences 13:1119–1127. https://doi.org/10.5194/bg-13-1119-2016
Poeplau C, Zopf D, Greiner B, Geerts R, Korvaar H, Thumm U, Don A, Heidkamp A, Flessa H (2018) Why does mineral fertilization increase soil carbon stocks in temperate grasslands? Agric Ecosyst Environ 265:144–155. https://doi.org/10.1016/j.agee.2018.06.003
Pravia MV, Kemanian AR, Terra JA, Shi Y, Macedo I, Goslee S (2019) Soil carbon saturation, productivity, and carbon and nitrogen cycling in crop-pasture rotations. Agric Syst 171:13–22. https://doi.org/10.1016/j.agsy.2018.11.001
R Core Team (2022) R: a language and environment for statistical computing. R Foundation for Statistical Computing, Vienna, Austria. URL https://www.R-project.org/.
Rawnsley R, Dynes RA, Christie KM, Harrison MT, Doran-Browne NA, Vibart R, Eckard R (2016) A review of whole farm-system analysis in evaluating greenhouse-gas mitigation strategies from livestock production systems. Anim Prod Sci 58:980–989. https://doi.org/10.1071/AN15632
Rawnsley RP, Smith AP, Christie KM, Harrison MT, Eckard RJ (2019) Current and future direction of nitrogen fertiliser use in Australian grazing systems. Crop Pasture Sci 70:1034–1043. https://doi.org/10.1071/CP18566
Riedo M, Grub A, Rosset M, Fuhrer J (1998) A pasture simulation model for dry matter production, and fluxes of carbon, nitrogen, water and energy. Ecol Modell 105:141–183. https://doi.org/10.1016/S0304-3800(97)00110-5
Rowarth JS, Tillman RW, Gillingham AG, Gregg PEH (1992) Phosphorus balances in grazed, hill-country pastures: the effect of slope and fertiliser input. N Z J Agric Res 35:337–342. https://doi.org/10.1080/00288233.1992.10427511
Saggar S, Mackay AD, Hedley MJ, Lambert MG, Clark DA (1990) A nutrient-transfer model to explain the fate of phosphorus and sulphur in a Grazed Hill-Country pasture. Agric Ecosyst Environ 30:295–315. https://doi.org/10.1080/00288233.1990.10430669
Saggar S, Hedley CA, Mackay A (1997) Partitioning and translocation of photosynthetically fixed 14C in grazed hill pastures. Biol Fertil Soils 25:152–158. https://doi.org/10.1007/s003740050296
Saggar S, Mackay AD, Hedley CB (1999) Hill slope effects on the vertical fluxes of photosynthetically fixed 14C in a grazed pasture. Soil Res 37:655–666. https://doi.org/10.1071/SR98114
Saggar S, Giltrap DL, Davison R, Gibson R, de Klein CAM, Rollo M, Ettema P, Rys G (2015) Estimating direct N2O emissions from sheep, beef, and deer grazed pastures in New Zealand hill country: accounting for the effect of land slope on the N2O emission factors from urine and dung. Agric Ecosyst Environ 205:70–78. https://doi.org/10.1016/j.agee.2015.03.005
Sakadevan K, Hedley MJ, Mackay AD (1993) Mineralisation and fate of soil sulphur and nitrogen in hill pastures. N Z J Agric Res 36:271–281. https://doi.org/10.1080/00288233.1993.10417763
Schipper LA, Dodd MB, Fisk LM, Power IL, Parenzee J, Arnold G (2011) Trends in soil carbon and nutrients of hill-country pastures receiving different phosphorus fertilizer loadings for 20 years. Biogeochemistry 104:35–48. https://doi.org/10.1007/s10533-009-9353-5
Schon NS, Mackay AD, Gray RA (2019) Changes in the abundance and diversity of earthworms in hill soils under different long-term fertiliser and sheep stocking regimes. N Z J Agric Res 64:162–177. https://doi.org/10.1080/00288233.2019.1581238
Smith AP, Christie KM, Harrison MT, Eckard RJ (2021) Ammonia volatilisation from grazed, pasture based dairy farming systems. Agric Syst 190:103119. https://doi.org/10.1016/j.agsy.2021.103119
Sochorová L, Jansa J, Verbruggen E, Hejcman M, Schellberg J, Kiers ET, Johnson NC (2016) Long-term agricultural management maximizing hay production can significantly reduce belowground C storage. Agric Ecosyst Environ 220:104–114. https://doi.org/10.1016/j.agee.2015.12.026
Stewart CE, Paustian K, Conant RT, Plante AF, Six J (2007) Soil carbon saturation: concept, evidence and evaluation. Biogeochemistry 86:19–31. https://doi.org/10.1007/s10533-007-9140-0
Stoner SW, Hoyt AM, Trumbore S, Sierra CA, Schrumpf M, Doetterl S, Baisden WT, Schipper LA (2021) Soil organic matter turnover rates increase to match increased inputs in grazed grasslands. Biogeochemistry 156:145–160. https://doi.org/10.1007/s10533-021-00838-z
Tedeschi LO (2006) Assessment of the adequacy of mathematical models. Agric Syst 89:225–247. https://doi.org/10.1016/j.agsy.2005.11.004
Thornley JH (1998) Grassland dynamics: an ecosystem simulation model. CAB International, Wallingford
van der Weerden T, Noble A, Luo J, de Klein C, Saggar S, Giltrap D, Gibbs J, Rys G (2020) Meta-analysis of New Zealand’s nitrous oxide emission factors for ruminant excreta supports disaggregation based on excreta form, livestock type and slope class. Sci Total Environ 732:139235. https://doi.org/10.1016/j.scitotenv.2020.139235
van Groenigen JW, van Kessel C, Hungate BA, Oenema O, Powlson DS, van Groenigen KJ (2017) Sequestering soil organic carbon: a nitrogen dilemma. Environ Sci Technol 51:4738–4739. https://doi.org/10.1021/acs.est.7b01427
van Paassen JG, Britton AJ, Mitchell RJ, Street LE, Johnson D, Coupar A, Woodin SJ (2020) Legacy effects of nitrogen and phosphorus additions on vegetation and carbon stocks of upland heaths. New Phytol 228:226–237. https://doi.org/10.1111/nph.16671
Vibart RE, Mackay A, Devantier B, Noakes E, Maclean P (2021) Sheep dung disappearance from grazed hill country landscapes. J N Z Grassl 83:171–177. https://doi.org/10.33584/jnzg.2021.83.3509
White CM, Kemanian AR, Kaye JP (2014) Implications of carbon saturation model structures for simulated nitrogen mineralization dynamics. Biogeosciences 11:6725–6738. https://doi.org/10.5194/bg-11-6725-2014
White RE, Davidson B, Lam SK, Chen D (2018) A critique of the paper ‘Soil carbon 4 per mille’ by Minasny et al. (2017). Geoderma 309:115–117. https://doi.org/10.1016/j.geoderma.2017.05.025
Whitehead D, Schipper LA, Pronger J, Moinet GYK, Mudge PL, Calvelo Pereira R, Kirschbaum MUF, McNally SR, Beare MH, Camps-Arbestain M (2018) Management practices to reduce losses or increase soil carbon stocks in temperate grazed grasslands: New Zealand as a case study. Agric Ecosyst Environ 265:432–443. https://doi.org/10.1016/j.agee.2018.06.022
Woodford K, Nicol A (2004) A re-assessment of the stock unit system. A report prepared for the Ministry of Agriculture and Forestry, Wellington, New Zealand
Young R, Cowie A, Harden S, McLeod R (2016) Soil carbon and inferred net primary production in high- and low-intensity grazing systems on the New England Tableland, eastern Australia. Soil Res 54:824–839. https://doi.org/10.1071/SR15316
Acknowledgements
Model development and analysis, and preparation of the manuscript was partly funded by the Sustainable Land Management and Climate Change fund of the Ministry for Primary Industries and the AgResearch Strategic Science Investment Fund. FB was funded by the New Zealand Government through the LEARN Awards programme, and hosted by AgResearch Grasslands, Palmerston North.
Funding
Open Access funding enabled and organized by CAUL and its Member Institutions.
Author information
Authors and Affiliations
Corresponding author
Ethics declarations
Conflict of interest
The authors declare no competing interests.
Additional information
Publisher's Note
Springer Nature remains neutral with regard to jurisdictional claims in published maps and institutional affiliations.
Supplementary Information
Below is the link to the electronic supplementary material.
Rights and permissions
Open Access This article is licensed under a Creative Commons Attribution 4.0 International License, which permits use, sharing, adaptation, distribution and reproduction in any medium or format, as long as you give appropriate credit to the original author(s) and the source, provide a link to the Creative Commons licence, and indicate if changes were made. The images or other third party material in this article are included in the article's Creative Commons licence, unless indicated otherwise in a credit line to the material. If material is not included in the article's Creative Commons licence and your intended use is not permitted by statutory regulation or exceeds the permitted use, you will need to obtain permission directly from the copyright holder. To view a copy of this licence, visit http://creativecommons.org/licenses/by/4.0/.
About this article
Cite this article
Bilotto, F., Vibart, R., Mackay, A. et al. Towards an integrated phosphorus, carbon and nitrogen cycling model for topographically diverse grasslands. Nutr Cycl Agroecosyst 124, 153–172 (2022). https://doi.org/10.1007/s10705-022-10231-3
Received:
Accepted:
Published:
Issue Date:
DOI: https://doi.org/10.1007/s10705-022-10231-3