Abstract
Introduction
The Laurén classification is widely used for Gastric Cancer (GC) histology subtyping. However, this classification is prone to interobserver variability and its prognostic value remains controversial. Deep Learning (DL)-based assessment of hematoxylin and eosin (H&E) stained slides is a potentially useful tool to provide an additional layer of clinically relevant information, but has not been systematically assessed in GC.
Objective
We aimed to train, test and externally validate a deep learning-based classifier for GC histology subtyping using routine H&E stained tissue sections from gastric adenocarcinomas and to assess its potential prognostic utility.
Methods
We trained a binary classifier on intestinal and diffuse type GC whole slide images for a subset of the TCGA cohort (N = 166) using attention-based multiple instance learning. The ground truth of 166 GC was obtained by two expert pathologists. We deployed the model on two external GC patient cohorts, one from Europe (N = 322) and one from Japan (N = 243). We assessed classification performance using the Area Under the Receiver Operating Characteristic Curve (AUROC) and prognostic value (overall, cancer specific and disease free survival) of the DL-based classifier with uni- and multivariate Cox proportional hazard models and Kaplan–Meier curves with log-rank test statistics.
Results
Internal validation using the TCGA GC cohort using five-fold cross-validation achieved a mean AUROC of 0.93 ± 0.07. External validation showed that the DL-based classifier can better stratify GC patients' 5-year survival compared to pathologist-based Laurén classification for all survival endpoints, despite frequently divergent model-pathologist classifications. Univariate overall survival Hazard Ratios (HRs) of pathologist-based Laurén classification (diffuse type versus intestinal type) were 1.14 (95% Confidence Interval (CI) 0.66–1.44, p-value = 0.51) and 1.23 (95% CI 0.96–1.43, p-value = 0.09) in the Japanese and European cohorts, respectively. DL-based histology classification resulted in HR of 1.46 (95% CI 1.18–1.65, p-value < 0.005) and 1.41 (95% CI 1.20–1.57, p-value < 0.005), in the Japanese and European cohorts, respectively. In diffuse type GC (as defined by the pathologist), classifying patients using the DL diffuse and intestinal classifications provided a superior survival stratification, and demonstrated statistically significant survival stratification when combined with pathologist classification for both the Asian (overall survival log-rank test p-value < 0.005, HR 1.43 (95% CI 1.05–1.66, p-value = 0.03) and European cohorts (overall survival log-rank test p-value < 0.005, HR 1.56 (95% CI 1.16–1.76, p-value < 0.005)).
Conclusion
Our study shows that gastric adenocarcinoma subtyping using pathologist’s Laurén classification as ground truth can be performed using current state of the art DL techniques. Patient survival stratification seems to be better by DL-based histology typing compared with expert pathologist histology typing. DL-based GC histology typing has potential as an aid in subtyping. Further investigations are warranted to fully understand the underlying biological mechanisms for the improved survival stratification despite apparent imperfect classification by the DL algorithm.
Similar content being viewed by others
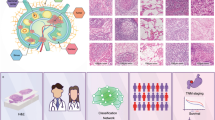
Avoid common mistakes on your manuscript.
Introduction
The development and application of DL, Machine Learning (ML) and Artificial Intelligence-based methods and techniques has seen an exponential rise within the field of Computational Pathology over the past decade [1, 2]. In particular, computer vision-based DL techniques using routine H&E-stained tissue sections have opened potential avenues for clinically relevant DL-based assessment of H&E-stained specimens [3,4,5,6].
GC is among the most prevalent cancers worldwide [7], and the vast majority of GC are adenocarcinomas. Since its introduction in 1965, the histopathological classification system of Laurén (hereinafter referred to as Laurén) is one of the most widely used histological classifications for adenocarcinomas of the stomach in the West [8]. It identifies two main histological subtypes: intestinal and diffuse types. These subtypes are genetically distinct and associated with different clinical outcomes. In the advanced disease stage, diffuse type GC are typically considered to be more aggressive resulting in poorer patient prognosis compared with intestinal type GC [9]. Research has been performed investigating the utility of Laurén for patient management decisions and several clinical trials are currently ongoing randomizing patients to different treatment options based on Laurén [9,10,11,12,13,14].
GC is well known for its high degree of histological inter- and intratumoral heterogeneity which is most likely the reason for high rates of intra- and interobserver variability of all known GC histological classification systems including Laurén [15, 16]. The inter- and intratumoral heterogeneity of GC and resulting challenges in accurate and reproducible histological classification [17, 18] may render prediction of patient outcomes difficult. The 4th edition of the WHO classification defined for the first time a ‘mixed type’ GC as presence of a poorly cohesive component (diffuse type according to Laurén) in combination with another histological subtype irrespective of relative amounts of each component [19]. This may lead to a further increase in interobserver variation of GC histology classification. Furthermore, the variation in the extent of tumor tissue sampling may lead to potentially ‘misclassified’ cases.
GC incidence is highest in Asian countries. In addition, GC patients from these regions often have higher rates of diffuse type GC and as such many Asian medical societies have developed their own histological classification systems which is more similar to the WHO classification system than to Laurén [20]. That said, conversion tables between classifications have been published [21, 22].
To the best of our knowledge, while intestinal and diffuse type GC has been attempted to be predicted genetically [11], DL-based classification of GC into diffuse and intestinal type has not previously been attempted systematically using large cohorts with clinical follow-up data. We have previously used DL to predict diffuse and intestinal type GC, but these results, while encouraging, were limited to a technical benchmark study [23].
We hypothesized that DL has the potential to reduce the above-mentioned inter- and intra-observer variation in classifying histological subtypes in GC and thus may improve accuracy and reproducibility of Laurén through its usage as a classification aid for pathologists.
The primary objective of the present investigation was to (1) establish a DL-based model to classify GC as diffuse or intestinal type and to (2) test the model performance on digital H&E-stained tissue sections from GC resection samples from European and Asian patients without outlining tumor regions. The secondary objective was to determine the hypothetical utility of such a model when used alongside pathologist classifications.
Methods
Ethics statement and patient cohorts
This study was performed in accordance with the Declaration of Helsinki. This study is a retrospective analysis of digital images of anonymized archival tissue samples of multiple cohorts of GC patients. The overall analysis was approved by the Ethics board at University Hospital Carl Gustav Carus, Dresden, Germany. The collection of patient samples was approved by the Ethics board at each institution as described below. The KCCH cohort was obtained from the Kanagawa Cancer Center Hospital in Yokohama, Japan. The KIEL cohort was obtained from the Department of Pathology, University Hospital Schleswig–Holstein, Kiel, Germany and the analysis was approved by the local ethical review board (D 453/10) of University Hospital Schleswig–Holstein [24]. The clinicopathological characteristics, inclusion and exclusion criteria of the KIEL and KCCH cohorts have been previously described [24, 25]. All patients of both cohorts were treatment-naive at time of surgical resection; i.e. patients did not receive neoadjuvant chemotherapy. The remaining half of the KCCH cohort received adjuvant chemotherapy. General population characteristics of the cohorts are reported in Suppl. Table 1. This study adheres to the TRIPOD guidelines (Suppl. Table 2, Suppl. Figure 1) [26,27,28].
Experimental design and statistics
We trained all neural networks on the TCGA-GC (“TCGA-STAD'' in the original TCGA nomenclature) dataset via stratified five-fold cross-validation at patient-level (“within-cohort experimentation” for Laurén). Expert pathologists from Japan (TA) and the West (HIG) reclassified all available TCGA-GC H&E stained tissue sections (usually one per case) according to the Laurén (Suppl. Table 3). Only samples in which the original classification provided in the TCGA database and the revised classification were identical were used for training, validation and testing. The intention of the previous procedure was the reduction of interobserver variability in classification and thereby improving the quality of the ground truth. On the basis of acceptable performance defined as a mean AUROC of greater than 85% of the aforementioned folds with a lower standard deviation (SD) above 80%, we proceeded to train a model using the TCGA-GC dataset (N = 166). The model was externally validated on KCCH and KIEL separately (Fig. 1A). We estimated survival probabilities using Kaplan–Meier curves (KMCs) for both cohorts. The KMCs included those for: model predicted classifications, pathologist classifications, model and pathologist agreement for each subtype as well as model and pathologist disagreement for each subtype. We performed pairwise log-rank tests using Overall Survival (OS) and Cancer-Specific Survival (CSS) for KIEL, and OS, CSS and Disease-Free Survival (DFS) for KCCH using the aforementioned stratifiers. A p value of < 0.05 was considered statistically significant. No correction for multiple testing was applied.
Outline of the study and development of the classifier. A The cohorts used for the present studies and their regional origin. B Overview of study methodology. C Sankey graph of how TCGA classifications changed when independently evaluated by our in-house expert pathologist (HIG). D KMC for OS comparing pathologist-model unanimity and discordance for intestinal and diffuse type using concatenated validation results for the TCGA cross-validation folds. E ROC curve for the five-fold cross validation on the TCGA cohort using only WSIs in which both TCGA and our pathologist were in unanimity with when performing Laurén
Image preprocessing
All images from H&E-stained tissue sections obtained from resection specimens used in our analysis were preprocessed according to the “Aachen protocol for deep learning histopathology” [29]. Whole Slide Images (WSIs) were obtained using Hamamatsu C13210 and Leica Aperio digital slide scanners for the KIEL and KCCH cohorts, respectively. WSIs were tessellated into image tiles of 256 µm edge length, processed at 224 by 224 pixel edge length (effective resolution of 1.14 μm per pixel), normalized according to ImageNet’s image statistics and augmented by rotation up to 360° and vertical flipping. Tiles not containing tissue and blurry tiles were automatically removed using the edge quantity as described in previous studies [30]. Tiles in the training set were color-normalized with the Macenko method [4, 31]. We applied a DL technique known as attention Multiple Instance Learning (attMIL) [32], as described previously [33, 34], to train our model. AttMIL addresses a weakly supervised classification problem in which the objective is to predict a slide label from a collection of individual tiles. This mechanism assigns a weight to each tile, reflecting its importance for the classification task. The final case-based score is obtained by summing the product of vectors representing tiles generated by the embedding layer of the neural network and their corresponding attention weights and passing the resulting vector through a simple classifier. The attention mechanism allows our model to focus on the most informative regions within the whole slide image while considering the contribution of other tiles as well. We trained and tested a model on top of a frozen feature extractor trained with self-supervised learning. Wang et al. previously trained a ResNet-50 on 3200 WSIs from TCGA via the RetCCL self-supervised learning algorithm [35]. We used this pre-trained architecture to extract 2048 features per tile (Fig. 1B).
Visualization and explainability
Visualization of morphological features relevant to the decision-making processes of DL models was important for: 1) identifying unique phenotypic patterns for different biomarkers and 2) better comprehension of how a model’s output was derived from its input data. For visualization, we plotted highly scoring tiles (top tiles) and whole slide heatmaps. The top tiles were the highest scoring tiles from patients that were correctly classified with the strongest confidence, i.e., with the highest registered probability scores obtained from passing individual tiles through the attMIL model [36]. Finally, slide heatmaps displayed distributions of the tiles’ attention and prediction scores over a WSI.
Results
Internal cross-validation of TCGA GC Laurén
The original TCGA database had N = 238 labeled samples (intestinal n = 161, diffuse n = 62, mixed n = 15). Two expert gastrointestinal pathologists (TA, HIG) reclassified the available H&E stained tissue sections as intestinal type n = 129, diffuse type n = 63 using Laurén or mixed n = 46 using the WHO classification. Only GC in which there was concordance between expert pathologist and original TCGA database were used for model training, testing and validation (Fig. 1C) leaving 166 GC (n = 116 intestinal type, n = 48 diffuse type and n = 2 mixed type). By doing so, we ensured that all training cases had a consistent and reliable classification that was established in a fully blinded manner, while still reflecting a consensus among the experts. As there were only two GC classified as mixed type in both classifications, these GC were excluded from further analyses.
We performed five-fold cross-validation on the TCGA-GC dataset and achieved a mean AUROC of 93% with a lower SD of 86% (Fig. 1D–E). As the performance met our predefined conditions, we proceeded to train a binary classifier (intestinal type versus diffuse type) on the TCGA GC dataset and used this model for all further external validation.
DL-based histological GC classification and survival
Our single TCGA model was deployed on the KIEL and KCCH cohorts. N = 29 (33.3%) GC from the Kiel cohort and N = 82 (54.3%) GC from the KCCH cohort that were originally classified as diffuse type were reclassified as intestinal type by the DL model (Fig. 2A, D).
DL-based subtyping improves prognostication in external cohorts. A Sankey graph of how KIEL classifications changed when evaluated by our model B KMCs for 5-year OS comparing model classification with pathologist classification in the KIEL cohort. C KMCs for 5-year OS comparing model classification with pathologist classification in the KCCH cohort. D Sankey graph of how KCCH classifications changed when evaluated by our model. E KMCs for 5-year for CSS comparing model classification with pathologist classification in the KIEL cohort. F KMCs for CSS comparing model classification with pathologist classification in the KCCH cohort
In the KCCH and the KIEL cohort, there was no significant difference in 5-year OS, CSS or DFS using the histological classification originally provided by the pathologist (intestinal type, diffuse type, mixed type) to stratify patients.
When using the DL-based histological classifier, there was a significantly poorer survival of the DL model-based diffuse type compared to the DL-based intestinal type in both cohorts (Fig. 2B, 2C, 2E, 2F, Suppl. Tables 4–5).
When performing univariate and multivariate (controlling for age, sex, UICC TNM stage, microsatellite instability (MSI), Epstein–Barr Virus (EBV)-, HER2- and cMET-status in the KIEL cohort, and in the KCCH cohort the aforementioned variables as well as tumor location, KRAS- and BRAF-mutation status, treatment type (surgery alone vs surgery followed by adjuvant chemotherapy), gastrectomy type and splenectomy status) Cox proportional hazards regression analyses, pathologist classification of diffuse and intestinal type GC was not related to survival (Suppl. Table 8, Suppl. Table 10). In contrast, univariate models stratifying patients by DL-based diffuse and intestinal type showed a significant relationship with all survival types in both cohorts. The DL-based classifier proved to be an independent prognostic marker in multivariate models in the KIEL cohort (Suppl. Table 11). While all multivariate models for KCCH outperformed their pathologist-labeled counterparts, none achieved a p value below 0.05 (p values of 0.06, 0.12 and 0.06 for OS, CSS and DFS, respectively) (Fig. 3A–D). Notably, even the lowest HR generated from DL-based classifier (multivariate CSS on the KCCH cohort) was higher than the best HR generated from pathologist-labeled data (univariate CSS on the KIEL cohort) (Fig. 3E–F).
Forest plots for multivariate Cox proportional hazards models. A Forest plot for multivariate Cox proportional hazards model for 5-year overall survival in the KCCH Cohort using model predictions. B Forest plot for multivariate Cox proportional hazards model for 5-year overall survival in the KCCH cohort using pathologist classifications. C Forest plot for multivariate Cox proportional hazards model for 5-year overall survival in the KIEL cohort using model predictions. D Forest plot for multivariate Cox proportional hazards model for 5-year overall survival in the KIEL Cohort using pathologist classifications. E Forest plot for Cox proportional hazards model in the KIEL cohort. F Forest plot for Cox proportional hazards model in the KCCH cohort. Asterisks indicate p-values < 0.05
Interpretability of DL-based histological classification
We qualitatively assessed a selection of attention and heatmaps for WSI’s in which the DL-based classification disagreed with the pathologist’s classification, as well as WSI’s in which the pathologist’s classification was ‘mixed-type’. (Fig. 4A) It seemed that the disagreement between DL-based classification and pathologist’s classification might be more common in poorly differentiated cancer. In the cases classified as mixed type by the pathologist, the DL-based classifier mainly highlighted areas with intestinal type cancer. Due to the binary nature of the classifier, DL would categorize WSI’s containing intestinal and diffuse type features according to the majority of tiles falling in one of the categories. Furthermore, the presence of extracellular mucin in WSI’s led to inaccurate attention by the model negatively impacting the model’s accuracy (Fig. 4B). Additionally, artifacts related to cell death and autolysis appear to be misinterpreted as diffuse type GC by the DL model.
Interpretability of DL models. A Unaltered whole slide image along with the attention and classification maps for the same regions generated by our model for a slide labeled as intestinal the pathologist and diffuse by our model. Brighter regions in the attention map indicate greater importance by the model. Redder regions in the heat map indicate the model evaluating the region as increasingly intestinal type in nature. B Unaltered whole slide image along with the attention and classification maps for the same regions generated by our model for a slide labeled as intestinal the pathologist and diffuse by our model. Brighter regions in the attention map indicate greater importance by the model. Bluer regions in the heat map indicate the model evaluating the region as increasingly diffuse type in nature
Utility of model classifications in conjunction with pathologist classifications
As patient survival stratification seemed to be improved when using the DL-based classification in particular for DL-based diffuse type GC, we considered the model’s potential utility when combined with pathologist labeling so as to classify a GC as diffuse type with more certainty (Fig. 5A). We performed a subgroup analysis of patients classified as diffuse type by the pathologist (n = 87 and n = 151 for KIEL and KCCH respectively), and stratified patients into those where the model agreed with the pathologist’s classification and those where the model classified the cancer as intestinal type and investigated the relationship with survival of this new groupings. In both the KCCH and KIEL cohorts, we observed statistically significant survival stratification for all available survival types. We determined this through the log-rank tests as well as Cox proportional hazards regression. We observed a noticeable stratification in KMCs (Fig. 5B–C). Notably, the cases classified by the model as intestinal broadly follow the same survival trendline as the true intestinal population.
DL-based companion tool for improved clinical stratification. A Example workflow for how such a model can be used in assisting in patient stratification and prognostication. After an H&E slide is evaluated by a pathologist, if it has been labeled as diffuse type, it will then be handed over to the model for a second evaluation. In the event of pathologist-model unanimity, this patient will be stratified into the worst prognosis group. In the event of model-pathologist discordance, the patient will be stratified into the intermediate prognosis group. Finally if the patient is evaluated as intestinal type by the pathologist, this H&E slide will undergo no further assessment due to model-pathologist discordance being exceedingly rare and classified into the best prognosis group B Comparison of 5-year overall survival in the KIEL cohort when using the model as described in the first panel C Comparison of 5-year overall survival in the KCCH cohort when using the model as described in the first panel
Limitations
All specimens were obtained from resection specimens, however, tumor cell morphology changes from the tumor surface to the center and invasion front. Thus, it remains to be shown whether the DL-based classification can be applied to endoscopic tumor biopsies as treatment decisions for GC patients with resectable disease have to be made at the time of the diagnostic biopsy. Additionally, the highly heterogeneous nature of GC means that single tissue sections, as was used in the present study, may not be fully representative of the whole tumor. We also recognize that future work could benefit from investigating strategies for selecting the most representative sections of the tumor, taking into consideration the challenges posed by intratumoral heterogeneity in GC classification. Finally, we recognize the concern of overfitting in the TCGA cohort and acknowledge that some degree of overfitting is inevitable. We nonetheless believe that this study demonstrates the potential of DL-based histology typing as an aid in subtyping, serving as a strong foundation for further investigation.
Discussion
Laurén is a commonly used method for histologic subtyping in GC. Nevertheless, this classification is subjective with high interobserver variation, and there remains a lack of consensus regarding its value as a prognostic tool. To the best of our knowledge, this is the first study to attempt Laurén prediction in GC using current state-of-the-art DL techniques in a systematic manner. Our results show that a model trained on TCGA GC WSIs using pathologist’s Laurén as ground truth achieves an excellent performance when internally cross-validated with an AUROC of 93%. Furthermore, we show that despite imperfect external validation performance, the DL-based histological subtype was able to stratify patients by survival whereas the pathologist-based Laurén (ground truth) did not. These findings seem to suggest that a DL-based histology subtype model trained on the pathologist’s Laurén classification and not trained on the survival data is able to predict patient survival. Up to 54% of GC classified as intestinal type by the DL model were originally classified as diffuse type by the pathologist. This relatively large number of reclassified cases using the DL-based classification could explain why the pathologist-based Laurén subtypes did not stratify patients by survival. This could reflect the known difficulties pathologists have to decide whether a poorly differentiated cancer should be classified as intestinal type or diffuse type. Further detailed quantitative analyses of the misclassified cases are needed to fully understand the prognostic relationship.
In our study, we observed a discrepancy between the high accuracy of the DL model in the TCGA dataset and its performance in the external datasets. However, it is important to emphasize that our primary objective was to assess the potential prognostic utility of the DL-based classifier for GC histology subtyping, rather than achieving perfect alignment with the Laurén classification. One has to realize that a DL-based model attempting to predict histological phenotype in GC will not fully reproduce the human decision-making process, e.g. the way pathologists establish the histological subtype according to the Lauren classification for a particular case. Pathologists may have a more nuanced view, evaluating the histological features on an intestinal-diffuse continuum to reach a final decision about the histological subtype. This strongly differs from the strictly dichotomous nature of the DL-based classifier based on the majority of patches classified as a certain subtype. Thus, because of the known heterogeneity of GC and the above explained majority call by the DL model, it is expected that during post-hoc evaluation of the DL-based classification by an expert pathologist, some of the DL model classification were found to be inaccurate. Nonetheless, survival stratification of patients appeared to be superior when using DL-based histological subtypes. This could indicate that the model is focused on a subset of histological features which happen to be prognostically relevant which are currently not recognized as such by the pathologist. It would be of clinical value to further scrutinize these histological slides, potentially using a different set of image analysis tools, to identify these prognostically relevant features so pathologists can learn to understand and recognize these themselves in the future. Alternatively, one could consider using a DL-based model to assist in the diagnostic process. The Laurén classification may not always be suitable for image analysis to predict the prognosis of patients. As an alternative, developing a ML-original classification could potentially yield better results. Consequently, we propose to investigate the development of such a classification system and compare its performance and similarity with conventional classification methods, including the Laurén classification. This would enable us to explore new avenues for predicting patient prognosis through image analysis, and could provide valuable insights for refining our current models.
In DL classification tasks within the field of computational histopathology, pathologist’s classification is generally treated as the ground truth when training the model [37]. Models are thus trained as best possible to imitate the pathologist’s classification. As a logical step from this, receiver operating characteristic curves and precision recall curves measuring the ability of the model to match pathologist classification are usually used as the primary form of model validation. However, in the case of certain classification tasks known to have high interobserver disagreement, one must consider carefully whether or not such methodologies are able to capture model performance accurately. Models are, as a general principle, only as good as the data they are trained, tested and validated on. There is an appreciable need for greater access and utilization of endpoints with greater objectivity such as survival, treatment response and other metrics with a lower propensity for imprecision and inaccuracies. These metrics are not without their own set of pitfalls, such a disagreement in treatment response or unclear cause of death due to a paucity of autopsies. However, these outcomes nonetheless serve to provide additional dimensions for interpreting the strengths and weaknesses of a given model. As such, when developing a model in which the ground truth is subjective and known for high levels of inter- and intra-observer disagreement, it may be worthwhile to incorporate such metrics to provide a more holistic perspective.
Conclusion
Our study shows that gastric adenocarcinoma subtyping on the basis of the Laurén classification can be performed using current state of the art deep learning techniques. Our DL-based classifier was able to stratify patients by survival, whereas the pathologist-based histology subtype was unable to do so. This seems to be primarily driven by the model reclassifying many resections as intestinal type which were originally classified as diffuse type. Further validation in endoscopic biopsies and detailed investigation to identify the histological survival-relevant features recognized by the DL model but not the pathologist are warranted.
Code availability
All codes are open source and available at https://github.com/KatherLab/marugoto.
Data availability
Some of the data that support the findings of this study are publicly available, and some are proprietary datasets provided under collaboration agreements. All data (including histological images) from the TCGA database are available at https://portal.gdc.cancer.gov/. All molecular data for patients in the TCGA cohorts are available at https://cbioportal.org/. All other data are under controlled access according to the local ethical guidelines and can only be requested directly from the respective study groups that independently manage data access for their study cohorts. Access to KIEL and KCCH was obtained via the Department of Pathology, Christian Albrechts University of Kiel, Kiel, Germany (https://www.medizin.uni-kiel.de/en/institutes-departments/institutes-of-clinical-theory/department-of-pathology) and the Kanagawa Cancer Center, Yokohama, Japan (https://kcch.kanagawa-pho.jp/en.html), respectively.
References
Bera K, Schalper KA, Rimm DL, Velcheti V, Madabhushi A. Artificial intelligence in digital pathology-new tools for diagnosis and precision oncology. Nat Rev Clin Oncol. 2019;16:703–15.
Shmatko A, Ghaffari Laleh N, Gerstung M, Kather JN. Artificial intelligence in histopathology: enhancing cancer research and clinical oncology. Nat Cancer. 2022;3:1026–38.
Echle A, Rindtorff NT, Brinker TJ, Luedde T, Pearson AT, Kather JN. Deep learning in cancer pathology: a new generation of clinical biomarkers. Br J Cancer. 2021;124:686–96.
Kather JN, Pearson AT, Halama N, Jäger D, Krause J, Loosen SH, et al. Deep learning can predict microsatellite instability directly from histology in gastrointestinal cancer. Nat Med. 2019;25:1054–6.
Muti HS, Heij LR, Keller G, Kohlruss M, Langer R, Dislich B, et al. Development and validation of deep learning classifiers to detect Epstein-Barr virus and microsatellite instability status in gastric cancer: a retrospective multicentre cohort study. Lancet Digit Health. 2021. https://doi.org/10.1016/S2589-7500(21)00133-3.
Cifci D, Foersch S, Kather JN. Artificial intelligence to identify genetic alterations in conventional histopathology. J Pathol. 2022;257:430–44.
Ilic M, Ilic I. Epidemiology of stomach cancer. World J Gastroenterol. 2022;28:1187–203.
Laurén P. The two histological main types of gastric carcinoma: Diffuse and so-called intestinal-type carcinoma. Acta Pathol Microbiol Scand. 1965;64:31–49.
Jimenez Fonseca P, Carmona-Bayonas A, Hernández R, Custodio A, Cano JM, Lacalle A, et al. Lauren subtypes of advanced gastric cancer influence survival and response to chemotherapy: real-world data from the AGAMENON national cancer registry. Br J Cancer. 2017;117:775–82.
Ma J, Shen H, Kapesa L, Zeng S. Lauren classification and individualized chemotherapy in gastric cancer. Oncol Lett. 2016;11:2959–64.
Tan IB, Ivanova T, Lim KH, Ong CW, Deng N, Lee J, et al. Intrinsic subtypes of gastric cancer, based on gene expression pattern, predict survival and respond differently to chemotherapy. Gastroenterology. 2011;141(476–85):485.e1-11.
Götze TO, Piso P, Lorenzen S, Bankstahl US, Pauligk C, Elshafei M, et al. Preventive HIPEC in combination with perioperative FLOT versus FLOT alone for resectable diffuse type gastric and gastroesophageal junction type II/III adenocarcinoma - the phase III “PREVENT”- (FLOT9) trial of the AIO /CAOGI /ACO. BMC Cancer. 2021;21:1158.
Wang K, Li E, Busuttil RA, Kong JC, Pattison S, Sung JJY, et al. A cohort study and meta-analysis of the evidence for consideration of Lauren subtype when prescribing adjuvant or palliative chemotherapy for gastric cancer. Ther Adv Med Oncol. 2020;12:1758835920930359.
Schirren R, Novotny A, Oesterlin C, Slotta-Huspenina J, Friess H, Reim D. Significance of lauren classification in patients undergoing neoadjuvant/perioperative chemotherapy for locally advanced gastric or gastroesophageal junction cancers-analysis from a large single center cohort in Germany. Cancers. 2021. https://doi.org/10.3390/cancers13020290.
Gullo I, Carneiro F, Oliveira C, Almeida GM. Heterogeneity in gastric cancer: from pure morphology to molecular classifications. Pathobiology. 2018;85:50–63.
Gao J-P, Xu W, Liu W-T, Yan M, Zhu Z-G. Tumor heterogeneity of gastric cancer: From the perspective of tumor-initiating cell. World J Gastroenterol. 2018;24:2567–81.
Stelzner S, Emmrich P. The mixed type in Laurén’s classification of gastric carcinoma. Histologic description and biologic behavior. Gen Diagn Pathol. 1997;143:39–48.
Pyo JH, Lee H, Min B-H, Lee JH, Choi MG, Lee JH, et al. Early gastric cancer with a mixed-type Lauren classification is more aggressive and exhibits greater lymph node metastasis. J Gastroenterol. 2017;52:594–601.
Nagtegaal ID, Odze RD, Klimstra D, Paradis V, Rugge M, Schirmacher P, et al. The 2019 WHO classification of tumours of the digestive system. Histopathology. 2020;76:182–8.
Japanese Gastric Cancer Association. Japanese gastric cancer treatment guidelines 2018 (5th edition). Gastric Cancer. 2021;24:1–21.
Gill S, Shah A, Le N, Cook EF, Yoshida EM. Asian ethnicity-related differences in gastric cancer presentation and outcome among patients treated at a canadian cancer center. J Clin Orthod. 2003;21:2070–6.
Jin H, Pinheiro PS, Callahan KE, Altekruse SF. Examining the gastric cancer survival gap between Asians and whites in the United States. Gastric Cancer. 2017;20:573–82.
Ghaffari Laleh N, Truhn D, Veldhuizen GP, Han T, van Treeck M, Buelow RD, et al. Adversarial attacks and adversarial robustness in computational pathology. Nat Commun. 2022;13:1–10.
Ulase D, Heckl S, Behrens H-M, Krüger S, Röcken C. Prognostic significance of tumour budding assessed in gastric carcinoma according to the criteria of the International Tumour Budding Consensus Conference. Histopathology. 2020;76:433–46.
Hayashi T, Yoshikawa T, Bonam K, Sue-Ling HM, Taguri M, Morita S, et al. The superiority of the seventh edition of the TNM classification depends on the overall survival of the patient cohort: comparative analysis of the sixth and seventh TNM editions in patients with gastric cancer from Japan and the United Kingdom. Cancer. 2013;119:1330–7.
Collins GS, Reitsma JB, Altman DG, Moons KGM. Transparent Reporting of a multivariable prediction model for Individual Prognosis or Diagnosis (TRIPOD): the TRIPOD statement. Ann Intern Med. 2015;162:55–63.
Moher D, Hopewell S, Schulz KF, Montori V, Gøtzsche PC, Devereaux PJ, et al. CONSORT 2010 explanation and elaboration: updated guidelines for reporting parallel group randomised trials. Int J Surg. 2012;10:28–55.
Moher D, Schulz KF, Altman D, CONSORT Group (Consolidated Standards of Reporting Trials). The CONSORT statement: revised recommendations for improving the quality of reports of parallel-group randomized trials. JAMA. 2001;285:1987–91.
Loeffler, Echle, Heij, Buelow, Krause. The Aachen protocol for deep learning histopathology: a hands-on guide for data preprocessing. Zenodo: Aachen.
Ghaffari Laleh N, Muti HS, Loeffler CML, Echle A, Saldanha OL, Mahmood F, et al. Benchmarking weakly-supervised deep learning pipelines for whole slide classification in computational pathology. Med Image Anal. 2022;79: 102474.
Macenko M, Niethammer M, Marron JS, Borland D, Woosley JT, Guan X, et al. A method for normalizing histology slides for quantitative analysis. 2009 IEEE International Symposium on Biomedical Imaging: From Nano to Macro. 2009; pp. 1107–1110.
Ilse M, Tomczak JM, Welling M. Attention-based Deep Multiple Instance Learning. arXiv [cs.LG]. 2018; Available: http://arxiv.org/abs/1802.04712
Saldanha OL, Loeffler CML, Niehues JM, van Treeck M, Seraphin TP, Hewitt KJ, et al. Self-supervised deep learning for pan-cancer mutation prediction from histopathology. bioRxiv. 2022;p. 2022.09.15.507455. https://doi.org/10.1101/2022.09.15.507455
Seraphin TP, Luedde M, Roderburg C, van Treeck M, Schneider P, Buelow RD, et al. Prediction of heart transplant rejection from routine pathology slides with self-supervised Deep Learning. medRxiv. 2022; https://doi.org/10.1101/2022.09.29.22279995
Wang X, Du Y, Yang S, Zhang J, Wang M, Zhang J, et al. RetCCL: clustering-guided contrastive learning for whole-slide image retrieval. Med Image Anal. 2022. https://doi.org/10.1016/j.media.2022.102645.
Brockmoeller S, Echle A, Ghaffari Laleh N, Eiholm S, Malmstrøm ML, Plato Kuhlmann T, et al. Deep learning identifies inflamed fat as a risk factor for lymph node metastasis in early colorectal cancer. J Pathol. 2022;256:269–81.
Kleppe A, Skrede O-J, De Raedt S, Liestøl K, Kerr DJ, Danielsen HE. Designing deep learning studies in cancer diagnostics. Nat Rev Cancer. 2021;21:199–211.
Funding
Open Access funding enabled and organized by Projekt DEAL. JNK is supported by the German Federal Ministry of Health (DEEP LIVER, ZMVI1-2520DAT111) and the Max-Eder-Programme of the German Cancer Aid (grant #70113864), the German Federal Ministry of Education and Research (PEARL, 01KD2104C; CAMINO, 01EO2101; SWAG, 01KD2215A; TRANSFORM LIVER, 031L0312A), the German Academic Exchange Service (SECAI, 57616814), the German Federal Joint Committee (Transplant.KI, 01VSF21048) and the European Union (ODELIA, 101057091; GENIAL, 101096312). HIG and JNK are supported in part by the National Institute for Health and Care Research (NIHR, NIHR213331) Leeds Biomedical Research Centre. The views expressed are those of the authors and not necessarily those of the NHS, the NIHR or the Department of Health and Social Care. GPV is supported by BMBF (Federal Ministry of Education and Research) in DAAD project 57616814 (SECAI, School of Embedded Composite AI, https://secai.org/) as part of the program Konrad Zuse Schools of Excellence in Artificial Intelligence.
Author information
Authors and Affiliations
Contributions
GPV and JNK designed the study; GPV performed the experiments; DC contributed technical experise; GPV, HIG and JNK analyzed the data; GPV performed statistical analyses; CR, HMB, TY, TO provided clinical and histopathological data; all authors provided clinical expertise and contributed to the interpretation of the results. GPV wrote the manuscript and all authors corrected the manuscript and collectively made the decision to submit for publication.
Corresponding author
Ethics declarations
Conflict of interest
HIG has received fees for advisory board activities by AstraZeneca and BMS, not related to this study. JNK reports consulting services for Owkin, France, Panakeia, UK and DoMore Diagnostics, Norway and has received honoraria for lectures by MSD, Eisai and Fresenius.
Additional information
Publisher's Note
Springer Nature remains neutral with regard to jurisdictional claims in published maps and institutional affiliations.
Supplementary Information
Below is the link to the electronic supplementary material.
Rights and permissions
Open Access This article is licensed under a Creative Commons Attribution 4.0 International License, which permits use, sharing, adaptation, distribution and reproduction in any medium or format, as long as you give appropriate credit to the original author(s) and the source, provide a link to the Creative Commons licence, and indicate if changes were made. The images or other third party material in this article are included in the article's Creative Commons licence, unless indicated otherwise in a credit line to the material. If material is not included in the article's Creative Commons licence and your intended use is not permitted by statutory regulation or exceeds the permitted use, you will need to obtain permission directly from the copyright holder. To view a copy of this licence, visit http://creativecommons.org/licenses/by/4.0/.
About this article
Cite this article
Veldhuizen, G.P., Röcken, C., Behrens, HM. et al. Deep learning-based subtyping of gastric cancer histology predicts clinical outcome: a multi-institutional retrospective study. Gastric Cancer 26, 708–720 (2023). https://doi.org/10.1007/s10120-023-01398-x
Received:
Accepted:
Published:
Issue Date:
DOI: https://doi.org/10.1007/s10120-023-01398-x