Abstract
Ship-induced wave wash affects the hydromorphological and ecological state of rivers through various mechanisms. The direct proximity of the riverbank is usually the most exposed, as the hydrodynamic stresses are the highest in these shallow water areas. Contrary to the steady and almost still, natural flow conditions (i.e., no waves of anthropogenic source), shoaling and breaking of ship waves increase the hydrodynamic stresses by orders of magnitudes, having notable ecological consequences, and resulting in bank erosion as well. Due to the shallow water depths and temporary drying, conventional measurement techniques are no longer applicable in these areas. In this study, large-scale particle image velocimetry (LSPIV) is used to quantify the prevailing flow conditions. In the absence of ground truth data in the wave breaking region, a high-resolution computational fluid dynamics model—verified with field pressure and acoustic Doppler velocimetry data—is used for the cross-validation of the LSPIV results. The results underline the applicability of LSPIV for the hydrodynamic analysis of wave velocities in this special riverine swash zone, which is of key importance from the aspect of ecology and bank erosion as well.
Graphical abstract

Similar content being viewed by others
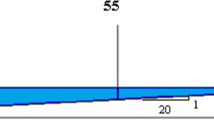
Avoid common mistakes on your manuscript.
1 Introduction
The ever-increasing demand for inland navigation and the efforts made for ecologically sustainable water usage have been resulting in conflicts of interests in water management. The construction of canals, for example, results in the removal of biogeographical boundaries, which may lead to the degradation of biodiversity through the spreading of nonnative, invasive species (Sala et al. 2000). Oil and fuel discharges from vessels directly affect the water quality (Jackivicz and Kuzminski 1973), while ship-induced waves have negative impacts on the function, structure and services of the aquatic ecosystem (Gabel et al. 2017).
Ship waves and their hydrodynamic impact in particular affect various levels of the ecosystem, from macroinvertebrates (Gabel et al. 2008, 2011, 2012) up to fishes (Fleit et al. 2021; Kucera-Hirzinger et al. 2009; Schludermann et al. 2014). As waves reach the shallower areas, bed shear stress increases gradually (Fleit et al. 2016), resulting in the potential resuspension of fine sediments as well (Fleit and Baranya 2021; Houser 2011; Rapaglia et al. 2015). Breaking waves are particularly responsible for even more intense sediment remobilization through mechanisms driven by organized vortices under the breakers (Otsuka et al. 2017; Voulgaris and Collins 2000). The resulting sediment remobilization and transport play a key role in bank erosion processes (Duró et al. 2020) and, moreover, have ecological impacts as well (Kucera-Hirzinger et al. 2009; Schallenberg and Burns 2004; Utne-Palm 2004). The ecological consequences of waves are often also the most intense in the surf zone, where shallow water depths are paired with intense hydrodynamic stresses caused by wave breaking (velocities, shear, turbulence). However, precisely due to the shallow water depths (few centimeters) and the temporary drying, the direct measurement of the hydrodynamic conditions in the surf zone is usually not possible with conventional instruments, like acoustic Doppler velocimeters (ADV).
Considering the relatively low number of studies dealing specifically with ship-related riverine wave wash, one must turn towards coastal engineering studies on the hydrodynamics of the swash zone. Due to the complexity of the prevailing flow conditions, the swash zone has been in the focus of (coastal) research in the last decades. Most researchers attempted to unveil the general hydrodynamic nature of uprushing, breaking and then retreating waves. Petti and Longo (2001) investigated different types of breaking waves in the swash zone in experimental conditions via laser Doppler velocimetry (LDV) and highlighted the asymmetric nature of uprushing and retreating flows from the aspect of turbulence. Shin and Cox (2006) have also identified key turbulence characteristics of swash zone waves, such as the vertical uniformity of time-averaged turbulent kinetic energy. Such controlled experiments with high-resolution (both in space and time) data significantly contribute to our understanding of the underlying hydrodynamics, which is key to tackling more complex problems, such as swash zone morphodynamics (Masselink and Puleo 2006).
The rapid development of imaging tools and computational capacities in the past decades initiated the development of image-based measurement and data acquisition techniques in a wide range of industrial and scientific applications. From the aspects of the hydraulic and mechanical engineering community, one of the most import tools arising from these disciplines was particle image velocimetry (PIV (Adrian 1991)). The fundamental principle behind PIV is based on the simple idea of approximating the flow velocity in a fluid based on the movement of objects travelling in it. PIV was originally developed for experimental environments, with special precision instruments (cameras, lasers, synchronizers, etc.). PIV-based methods are rather common in the hydroscience community and in the analysis of wave hydrodynamics in particular (e.g., Chang and Liu 2000; Cowen et al. 2003; Kimmoun and Branger 2007; Otsuka et al. 2017).
Bubble image velocimetry (BIV) has also become popular for the analysis of waves and green water problems in particular. BIV is a derivative of the PIV method, focusing on highly aerated, two-phase flows, such as (breaking) wave impacts on structures (Ariyarathne et al. 2012; Chuang et al. 2018; Ryu and Chang 2008). BIV allows for the investigation of horizontal wave velocities at the free surface as well. This is a notable advantage over classic PIV, where the analyses along a horizontal plane are only possible below the surface, where the plane of interest is virtually submerged.
Conventional PIV analysis is, however, usually restricted to experimental (laboratory) conditions. Although examples can be found for the field application of PIV (e.g., Cameron et al. 2013; Nimmo Smith et al. 2002), these are usually slightly modified versions of ordinary PIV, with its costly and complex measurement system and restraints. The adaptation of such practical image-based techniques to field conditions is usually much desired by the hydraulic engineering community, as they tend to offer fast, cheap non-intrusive measurements. These efforts had led to the development of the so-called large-scale PIV (LSPIV) in the 1990s (Fujita et al. 1998). In case of LSPIV, the movement of surface patches (rather than particles) is exploited for the analysis of surface velocities. The patches can be artificial (some sort of seeding, e.g., wood shavings) or natural (debris, foam (similarly to BIV), turbulent boils, etc.) as well. LSPIV has become a widely used tool in the hydraulic engineering community, due to its straightforward adaptability and reasonable costs. It is mostly used to conduct fast discharge measurements in rivers (Muste et al. 2008), especially during floods of high velocities and debris, where conventional methods are not applicable (Dramais et al. 2011; Fujita and Kunita 2011; Le Coz et al. 2010; Muste et al. 2011). In addition, researchers have also reported the applicability of LSPIV for the assessment of complex flows in shallow water conditions. Examples can be found for the analysis of coherent two-dimensional flow features and vortex shedding (Muste et al. 2014; Weitbrecht et al. 2002), macro-scale turbulence (Fox and Patrick 2008; Fox and Belcher 2011), bedload transport (Ermilov et al. 2022) or even bedform propagation analysis (Baranya et al. 2016; Muste et al. 2016; Tsubaki et al. 2018). Nonetheless, only a very few examples can be found where LSPIV is used for the analysis of waves. Choi and Roh (2021) recently proposed an experimental setup for the analysis of transient cross see rip currents, where surface flow velocities were captured via LSPIV (using seeding). Moreover, the pioneering work of Holland et al. (2001) already suggested the applicability of LSPIV for the analysis of swash zone hydrodynamics in coastal areas two decades ago.
The recent developments in computational fluid dynamics (CFD) modeling also significantly improved our understanding of swash zone hydrodynamics (Briganti et al. 2016). Researchers have developed highly capable, multiphase CFD tools, allowing the accurate simulation of wave propagation and breaking (e.g., Bihs et al. 2016 or Jacobsen et al. 2012). Such simulation tools are also able to tackle the consequent morphodynamic processes as well (Jacobsen and Fredsoe 2014).
In this study, videos taken of the near-bank area of a large river during ship-induced wave events are analyzed with LSPIV. Due to the low water depths and temporary wetting/drying, no conventional field measurement devices are applicable in this riverine swash zone. On the one hand, this means that we have no information on the prevailing flows in these highly exposed regions. On the other hand, it also means that the direct measurement-based verification of the image velocimetry results is not possible. Using the velocity and pressure measurements performed in a slightly deeper area of the near bank zone (distance of 3–10 m), a high-resolution computational fluid dynamics (CFD) model was built and validated. The verified CFD model is capable of directly resolving swash zone hydrodynamics (including wave breaking) and thus, it is used for the cross-validation of the LSPIV results.
2 Methods
2.1 Field measurements
The field measurements and video recordings were performed at the Hungarian reach of the Danube River, in the area of Horány (river kilometer 1668, Fig. 1). This is a side arm of the Danube, where the river is divided into two branches by the 31-km-long Szentendrei Island. Two-third of the total discharge flows in the studied branch, with an annual mean of 1400 m3 s−1; hence, it is the main navigational route. The measurement campaign was held on June 19, 2017, below mean flow conditions (1250 m3 s−1). The main goal of the measurements was to capture the hydrodynamic impacts of navigation-induced wave events in the near bank (littoral) zone of a large river.
The field measurement setup consisted of two acoustic Doppler velocimeters (ADV) operating at a sampling frequency of 16 Hz, a pressure sensor near the riverbed (8 Hz) and a GoPro Hero 4 action camera (Fig. 1). The devices were deployed along a slice perpendicular to the bank which enabled the proper building, parametrization and verification of the computational wave model (see later). Acoustic water depth measurements and GPS surveying provided the input for the digital elevation model (Fig. 1a). The precise location of six ground reference points (GRP) was also surveyed for the orthorectification and georeferencing of the videos. The measurements were performed continuously to capture all the relevant hydrodynamic features related to riverine navigation, except for the videos, which were only taken during the actual wave events.
The near-bottom pressure measurements were used to reconstruct the surface elevation time series. These wave time series were the drivers (boundary condition) for the numerical model, while the ADV-based velocity time series were used for model validation.
2.2 Large-scale PIV
The (LS)PIV code used in this study is an in-house research tool developed by the authors (Fleit and Baranya 2019). The most important steps of the image processing are briefly presented in the following.
Firstly, the originally color (RGB) videos are converted to grayscale (8-bit) image sequences. The resulting images are then enhanced with contrast limited adaptive histogram equalization (CLAHE). CLAHE operates on smaller subsections of an image, and in every one of these so-called tiles, the most frequent intensities of the image histogram are spread out to the full range of data. As a result, both under and over exposed areas are optimized independently. The method reportedly improves the probability of valid vector detection (Shavit et al. 2007). It is noted that there is no agreement on a universal image pre-processing methodology—the best technique (or a specific combination of them) is usually case specific, especially in case of field conditions.
Image sequences of the water surface are recorded from the riverbank in a specific angle (Fig. 1c). In order to obtain proper flow data from such images, their orthorectification is done first. The transformation is derived through matching control points of known real coordinates (x, y, z) with their image coordinates (pixel indices, i, j) (Fujita et al. 1998):
The coefficients (ai, bi, ci) in the transformation equations above are derived with the method of least squares, requiring a minimum of six reference points (Fig. 2).
Image orthorectification results in uneven pixel densities, with more pixels per unit area closer to the sensors and vice versa. To facilitate the straightforward implementation of an efficient PIV algorithm, the grayscale intensity (GSI) pixels are linearly interpolated to a structured, equispaced, orthogonal grid. That is, a new image matrix is generated, now with an orthogonal viewing angle and known real coordinates.
The cross-correlation (Rab) between subsections of successive images (interrogation areas (IA)) is used as a similarity index. The IA in the first image is centered in a calculation node, where the displacement/velocity evaluation is performed. The pattern within the IA of the first image is not searched throughout the whole of the second image, but only in the proximity of the original IA, within the so-called search area (SA). The SA has a predefined size, which determines the upper bounds of detected displacement in each Cartesian direction. Thus, the dimensions of the SA must be carefully determined to avoid velocity capping and preserve computational demand at the same time. Rab is then calculated between the IA in the first image and all possible IA displacements within the SA of the following image. The two-dimensional displacement vector with the highest Rab value is selected as the candidate vector. The cross-correlation can be written as (Fujita et al. 1998):
where MX and MY are the sizes of the IAs and axy and bxy are the distributions of the GSI in the two IAs separated by the time interval dt. Having the displacement vector and knowing dt, the two-dimensional velocities can be formulated.
The general precision of PIV-based methods is inherently restricted by the resolution of the used images, as the IA matching only offers candidate displacements in sizes of integer number of pixels. This issue is overcome with sub-pixel displacement corrections. In this study, the two-dimensional Gaussian function fit-based method is used (Nobach and Honkanen 2005). The Gaussian function is fitted to the correlation values around the original correlation peak (± 1 pixel in all directions, that is 9 points in total). The peak of the resulting analytical function can be derived, and it is accepted as a valid sub-pixel displacement. The candidate displacement vector components are then corrected with these values.
Outlier velocities (a result of inhomogeneous tracer distribution and/or illumination) are detected with a robust procedure proposed by (Westerweel and Scarano 2005), which is based on a normalized median test. Removed data are then replaced with an iterative gap filling method presented by (Wang et al. 2012), explicitly using data from both time and space for the prediction of missing values. The final smoothing of the results is done with an algorithm based on a penalized least squares method proposed by Garcia (2010).
The parameters of the LSPIV algorithm can usually be set properly if the general nature of the flow problem can be reasonably approximated prior the analyses (e.g., flow direction, maximal flow velocities). The settings of an optimal LSPIV parameter setup can then be refined iteratively considering the quality of the results and computational costs as well. The most relevant parameters of the herein used setup are summarized in Table 1.
2.3 CFD modeling
2.3.1 Numerical background
Considering that no ground truth data are available for the verification of LSPIV, image velocimetry data were compared with results from a verified computational fluid dynamics (CFD) model. The model was set up and verified with the pressure and velocity measurements, respectively. The CFD model REEF3D (Bihs et al. 2016) was used for the simulation of measured wave events. The open-source code solves the incompressible Reynolds-averaged Navier–Stokes (RANS) equations with finite difference method on a structured, orthogonal computational grid, along with the continuity equation:
where u is the velocity averaged over time t, ρ is the fluid density, p is the pressure, ν is the kinematic viscosity, νt is the turbulent eddy viscosity, and g is the acceleration due to gravity. Indexes i and j refer to Cartesian components of vector variables, and terms containing j are implicitly summed over j = 1…3. The advective terms are treated with the fifth-order accurate, weighted essentially non-oscillatory (WENO) scheme (Liu et al. 1994), while time marching is achieved with a third-order total variation diminishing Runge–Kutta scheme (Gottlieb and Shu 1998). The effect of turbulence is considered through the eddy viscosity concept—the two-equation k-ω model (Wilcox 1994) was used to model the turbulent features.
Being the main feature of waves, the complex free surface requires special treatment in the numerical simulations, especially since it may be vertically multivalued upon wave breaking. The interface between the two phases (water and air) is calculated using the level set method (Sussman 1994). The model has been extensively tested and validated for the modeling of various wave hydrodynamics related problems (Alagan Chella et al. 2015; Kamath et al. 2015; Wang et al. 2020) and the runup of ship-induced waves as well (Fleit et al. 2016, 2019, 2021).
2.3.2 Numerical setup
The modeling domain is simplified to a two-dimensional vertical slice (2DV) which allows for high-resolution wave modeling with reasonable computational costs. The bed elevation profile of the near bank area was generated based on data from the GPS surveying. The computational domain is 20.0 m long × 2.0 m high × 1 cell wide with a uniform grid resolution of dx = 0.01 m (Fig. 3).
Sketch of the computational domain. Location of the velocity probes (ADV) is presented (P1, P2), as well as the section analyzed via LSPIV (modified after Fleit et al. (2021))
The initial flatbed section (x = 0.0–5.0 m) of the domain is the so-called wave generation zone, where the boundary conditions (velocities, pressure and free surface position) are gradually introduced to the computational domain with the relaxation method (Bihs et al. 2016; Jacobsen et al. 2012). The inlet boundary conditions are defined using the wave reconstruction method (Aggarwal et al. 2018), offering the explicit generation of measured wave time series. The measured surface elevation time series are decomposed via discrete Fourier transform (DFT), from which the irregular wave trains can be reconstructed at the inlet boundary by superposing each individual linear wave component according to linear wave theory:
where Ai is the amplitude, ki is the wavenumber, ωi is the angular frequency, d is the total water depth, z is the signed distance from the mean free surface, and ϵi is the phase of each (i = 1…N) wave component. Since the measured wave signal was representative of the end of the wave generation zone (x = 5.0 m, the location of the pressure gauge during the field measurements), the backward transformation of phases was performed to the beginning of the computational domain (x = 0.0 m).
3 Results
Three shorter subsections of a measured wave event were used for the verifications (Fig. 4). The 20-s-long sections (S1, S2, S3) were selected in a way to represent waves of different intensity and duration, ranging from long-lasting mild waves (S3), up to short-term extremes (S2). The effect of primary (low frequency) wave components (i.e., the wave signals are temporarily biased compared to the still water level) is also accounted for during the numerical simulations. Considering that the pressure gauge (inlet boundary condition) was in a 10 m distance from the bank, the selected wave trains (S1, S2, S3) were extended in time in both directions for the modeling. This ensured that the waves have time to reach the bank, and that the initial hydrodynamic state in the near-bank area is as close to reality as possible.
3.1 Verification of the CFD model
The CFD model offers depth-resolved hydrodynamic solutions including pressure, flow velocities and turbulent intensity as well. To give an impression on the nature of wave runup in cases of different wave intensities, the cross-shore velocity (ux) distribution in the swash zone is presented in Fig. 5. In case of medium-sized waves (S1), a collapsing-type wave breaking is observed. An intense plunging breaker forms in case of the highest waves (S2), followed by a long wave runup. In case of the mildest waves (S3), no wave breaking is observed, with modest wave runups. These qualitative results show that the model is indeed capable of reproducing the dominant features in the swash zone: wave breaking, wave runup, uprush and retreat (Fig. 5).
Velocities from the numerical model are compared with field measured, high frequency (ADV) velocity time series in the sampled points P1 and P2 (see Fig. 3 for instrument locations). Considering the spatiotemporal complexity of the flow conditions and the simplifications made during the modeling (e.g., 2DV modeling approach), a perfect match is not expected. Nonetheless, the comparison shows a decent agreement between measured and modeled values (Fig. 6). Although slight phase errors are observed in all cases, the general nature and amplitude of the simulated velocities are in good agreement with the measurements. It is also noted that the high-frequency turbulent fluctuations observed in the measured data are not resolved (but accounted for) in the model due to the Reynolds-averaged approach. Based on the results presented in Fig. 6, the CFD model is considered verified. Thus, the hydrodynamic solution for the rest of the computational domain is validly used for the verification of the LSPIV results in the following.
Comparison of measured (ADV) and modeled velocity time series in the two sampled points P1 (top row) and P2 (bottom row) (modified after Fleit et al. (2021))
3.2 Verification of LSPIV
LSPIV was applied for the videos of the very same three section of the wave event. Contrary to the vertically resolved CFD model results, LSPIV only provides information regarding the surface velocities. The instantaneous velocity distributions presented in Fig. 7 show the nature of the LSPIV results and wave runup in general. At t = 0.0 and t = 0.8 s, wave runup and the following velocity attenuation is observed. Between t = 1.6–2.4 s, the high negative velocities represent the retreat towards the channel after the preceding breaking and uprush. Between t = 3.2–4.0 s, a wave breaking, and the following uprush is observed. The notable attenuation and dispersion of cross-shore velocities is highlighted, as a result of wave breaking. The velocity vectors show that although the swash zone is mainly dominated by the cross-shore velocities, a weak y-wise component is present as well, underlining the natural complexity of near-bank wave phenomena.
LSPIV offers horizontal distributions of surface velocities, while the CFD model is vertically resolved, yet horizontally one-dimensional. The common ground between the two approaches—necessary for any comparison—is the surface velocity distribution along a cross-shore slice. Thus, slice along the y-wise middle of the LSPIV results is applied; while surface velocities are extracted from the CFD results to make the comparisons possible. The resulting cross-shore horizontal surface velocities (ux) for the three sections (S1, S2, S3) are compared in the form of space–time distributions (Fig. 8). This representation provides a good overview on the dynamics and nature of wave-runup and the prevailing velocity magnitudes during uprush and downrush (retreat) as well.
Although the CFD model has been verified with velocity time series measured in a few meters distance from the shoreline, there were no control data for the swash zone before. In this sense, the results presented in Fig. 8 can also be interpreted as a cross-validation of the two methods. Even in the light of the notable simplifications made during the modeling, the simulation and image velocimetry results show reasonable agreement. The velocity magnitudes, the phases and the wave-runup lengths all show good agreement between CFD and LSPIV. The best match is observed in case of the most intense waves (S2), while the quality of the LSPIV results gradually decreases with lower wave intensities (S1 and S3). This systematic deterioration of quality is ascribed to the correlation between wave intensity and the density of natural tracers. That is, higher waves and more intense wave breaking results in more foam, more intense turbulent boils and sediment plumes. It is however noted that the velocity magnitude estimates are still reasonable in all cases.
In comparison with the measured (ADV) velocities in the surf zone (Fig. 6), significantly higher values are observed in Fig. 8. Maximal flow velocities for uprushing/downwashing flows (max(ux)/ − min(ux)) are around 0.6/0.5, 0.8/0.7 and 0.4/0.3 m/s for sections S1, S2 and S3, respectively. This asymmetric behavior is expected, as most of its kinetic energy is dissipated during wave breaking and runup. These values are roughly two time higher than the ones measured by the ADVs in a 3–4 m distance from the bank. The gradual increase of velocities is observed for the different intensity waves, underlying the relevance of a transient wave analysis method for the impacts of ship-induced waves.
Having (cross-)verified the LSPIV method for the three shorter subsections, it was applied to the complete wave event as well (Fig. 4). The evolution of velocity magnitudes corresponds well to the original (measured) surface elevation time series. The method offers high-resolution velocity data (both in space and time), which allows for detailed, quantitative inspection of the hydrodynamic impacts of ship waves in the near-bank area. The space–time distribution of instantaneous velocity magnitudes is presented in Fig. 9a, in a similar manner as earlier. It is noted that in addition to the hydrodynamic information, the LSPIV data implicitly contain wave-runup information as well.
LSPIV analysis of a complete wave event. a Space–time distribution of instantaneous surface velocity magnitudes in the middle cross-shore slice of the LSPIV ROI; b space–time distribution of period-averaged surface velocity magnitudes in the middle cross-shore slice of the LSPIV ROI; c temporal evolution of space-averaged velocity magnitudes along the cross-shore slice
In order to get a smoother overview on the temporal evolution of near-bank impacts of the wave wash, phase-averaged velocities have also been plotted (Fig. 9b). The average wave period (≈ 4.5 s) was derived from a DFT-based analysis of the LSPIV velocity time series.
Figure 9c presents a simple statistical evaluation of the LSPIV data. The temporal evolution of the slice-wise median and 25/75% quantile of phase-averaged velocity magnitudes was derived. The time series presented in Fig. 9c offer an easily interpretable summary of the hydrodynamic impacts of the wave event. The spatial compression of the data to the selected three statistical scalars offers a clear and well-comparable (with other wave events) overview on the wave event. It is noted that the above presented analysis and visualization of the data is arbitrary—the post-processing methodology is to be tailored to fit specific fields of application in the future.
4 Discussion
The popularity of image-based methods—and LSPIV in particular—is growing in the hydroscience community, mostly due to its fast and simple applicability. An in-house PIV code (Fleit and Baranya 2019) was used here for the quantification of surface wave velocities in the ship-wave-related swash zone of a large river. Considering that conventional velocity measurement techniques (e.g., acoustic Doppler velocimetry) are no longer applicable in these areas because of temporary drying and shallow water depths, the results wish to fill a knowledge gap. Although examples can be found for the field application of PIV for wave analyses (e.g., Nimmo Smith et al. 2002), these are usually slightly modified (field-adapted) versions of ordinary PIV, with its costly and complex measurement system and restraints. In this study, conventional LSPIV was applied to investigate ship-induced wave wash in a large river. The advantages and disadvantages of the method were discussed. The LSPIV measurements were performed within the framework of a detailed wave measurement campaign. This allowed the novel cross-verification of the image velocimetry results with CFD model output driven by acoustic velocity and pressure measurements.
A number of numerical simulation tools are available for the high-fidelity solution of wave-related flow problems (e.g., Bihs et al. 2016 or Jacobsen et al. 2012). These models are offer insights to swash zone hydrodynamics in various spatial and temporal scales (Briganti et al. 2016). In this study, the numerical model REEF3D was used for the simulation of ship-induced waves, following the earlier works of the authors (e.g., Fleit et al. 2019, 2021). The model was built and successfully verified against data from a field measurements campaign, allowing for an established cross-validation with the image velocimetry results.
The results presented in this study are a demonstration of the capabilities of LSPIV for the analysis of (ship-induced) wave-runup. It is noted that the spatiotemporally extensive wave velocity data provided by LSPIV could be further post-processed with methods tailored to specific applications. The authors believe that the proposed methodology could have a strong relevance in the long-term monitoring of the impact of waves of banks and beaches. Although the presented case study was focusing on the impacts of ship-induced waves, the authors underline that LSPIV could also be used for wave-runup analyses in different environments (lacustrine, coastal (see Holland et al. 2001)) as well.
Even though the focus of this study was on the presentation and verification of the LSPIV-based methodology itself—rather than on an in-depth analysis of swash zone wave hydrodynamics, some important conclusions can already be drawn from the first results. For instance, velocities during the uprush phase were found to be higher compared to backwash velocities, which coincides with the experimental results of Cowen et al. (2003) and Petti and Longo (2001). This shows that the image velocimetry method is able to capture the special characteristics of swash zone hydrodynamics. Cowen et al. (2003) also showed that the dominating turbulence in uprushing flows is different and stronger than fully developed boundary layer turbulence. As the resulting asymmetric hydrodynamic stresses play a major role in swash zone morphodynamics as well (Fuhrman et al. 2009; Jacobsen et al. 2014), it is considered promising, that the LSPIV captures these features.
As the key hydrodynamic characteristics are near-bed flow velocities or the bed shear stress in particular from many aspects (e.g., bank erosion (Duró et al. 2020) or impacts on the benthos (Gabel et al. 2012)), the fact that LSPIV only offers surface velocities is a notable limitation. It is, however, encouraging that the flow velocities in the swash zone were found to be almost uniform over the depths for most phases of the motion by Petti and Longo (2001). Moreover, the experimental work of Shin and Cox (2006) revealed that time-averaged turbulent kinetic energy is also uniform throughout the vertical in the inner surf and swash zones. The fact that surface velocity data can be used to derive hydrodynamic information along the vertical, further emphasizes the relevance of LSPIV-based investigations for the assessment of said impacts (morphodynamics, ecology) in the future.
Considering the advantages of LSPIV in general (low cost, fast, easy applicability), its future application for the quantitative monitoring of wave wash could yield data for the long-term analysis of ecological and bank erosion processes. Setting up such a 24/7 measurement station would require the application of an infrared image sensor for night recordings as well, along with the necessary adaptation of the LSPIV methodology. Such sensors have already been successfully used in image velocimetry applications (e.g., space–time image velocimetry by Fujita (2017)).
Coupling the obtained data from a future monitoring station with a navigational automatic identification system (AIS) could offer a solid database for further analyses. Such data could be exploited to investigate the influence of various ship-related parameters (e.g., size, speed, travelling distance from bank) on the wave load at different stages (effect in varying hydrological conditions). Assuming large datasets of video footages and related navigational information, artificial intelligence-based methods could well support the interrelation of ship parameters with wave impacts. Detailed hydrodynamic analysis of wave wash could then be exploited for a quantitative evaluation and prediction of potential bank erosion processes and vice versa: It could be utilized to formulate regulations for reaches of high ecological/economical values.
5 Conclusions
This study presented a proof-of-concept for the LSPIV-based assessment of surface velocities in a riverine swash zone. The cross-validation of the LSPIV results with a verified, high-resolution CFD model showed reasonable agreements. The proposed novel application of LSPIV for the quantification of ship-induced wave wash provides detailed hydrodynamic information in areas where conventional measurement techniques are no longer applicable due to limited depth conditions and temporary wetting/drying. This near-bank region is usually of high ecological relevance and, moreover, is highly exposed from the aspects of bank erosion processes as well. The application of the presented methodology in a monitoring fashion could improve our understanding of the ongoing, long-term hydrodynamic processes, which is the key to resolve the wave-related ecological, sedimentological and economic issues.
References
Adrian R (1991) Particle-imaging techniques for experimental fluid-mechanics. Annu Rev Fluid Mech 23(1):261–304. https://doi.org/10.1146/annurev.fluid.23.1.261
Aggarwal A, Pákozdi C, Bihs H, Myrhaug D, Alagan Chella M (2018) Free surface reconstruction for phase accurate irregular wave generation. J Mar Sci Eng 6(3):105. https://doi.org/10.3390/jmse6030105
Alagan Chella M, Bihs H, Myrhaug D (2015) Characteristics and profile asymmetry properties of waves breaking over an impermeable submerged reef. Coast Eng 100:26–36. https://doi.org/10.1016/j.coastaleng.2015.03.008
Ariyarathne K, Chang KA, Mercier R (2012) Green water impact pressure on a three-dimensional model structure. Exp Fluids 53(6):1879–1894. https://doi.org/10.1007/s00348-012-1399-9
Baranya S, Muste M, Pratt TC, Abraham D (2016) Acoustic Mapping Velocimetry (AMV) for in-situ bedload transport estimation. In: River Flow 2016: proceedings of the international conference on Fluvial Hydralics (River Flow 2016), St. Louis, USA, 11–14 July 2016, 1–7
Bihs H, Kamath A, Alagan Chella M, Arntsen ØA (2016) Breaking-wave interaction with tandem cylinders under different impact scenarios. J Waterw Port Coast Ocean Eng 142(5):04016005. https://doi.org/10.1061/(asce)ww.1943-5460.0000343
Briganti R, Torres-Freyermuth A, Baldock TE, Brocchini M, Dodd N, Hsu T-J, Jiang Z, Kim Y, Pintado-Patino JC, Postacchini M (2016) Advances in numerical modelling of swash zone dynamics. Coast Eng 116:26–41. https://doi.org/10.1016/j.coastaleng.2016.05.001
Cameron SM, Nikora VI, Albayrak I, Miler O, Stewart M, Siniscalchi F (2013) Interactions between aquatic plants and turbulent flow: a field study using stereoscopic PIV. J Fluid Mech 732:345–372. https://doi.org/10.1017/jfm.2013.406
Chang KA, Liu PLF (2000) Pseudo turbulence in PIV breaking-wave measurements. Exp Fluids 29(4):331–338. https://doi.org/10.1007/s003489900090
Choi J, Roh M (2021) A laboratory experiment of rip currents between the ends of breaking wave crests. Ocean Eng 164:103812. https://doi.org/10.1016/j.coastaleng.2020.103812
Chuang W, Chang KA, Mercier R (2018) Green water flow on a fixed model structure in a large wave basin under random Waves. In: Proceedings of the International Conference on Offshore Mechanics and Arctic Engineering–OMAE, 7B(6), 1–26. https://doi.org/10.1115/OMAE2018-77184
Cowen EA, Mei Sou I, Liu PL-F, Raubenheimer B (2003) Particle image velocimetry measurements within a laboratory-generated swash zone. J Eng Mech 129(10):1119–1129. https://doi.org/10.1061/(ASCE)0733-9399(2003)129:10(1119)
Dramais G, Le Coz J, Camenen B, Hauet A (2011) Advantages of a mobile LSPIV method for measuring flood discharges and improving stage-discharge curves. J Hydro-Environ Res 5(4):301–312. https://doi.org/10.1016/j.jher.2010.12.005
Duró G, Crosato A, Kleinhans MG, Roelvink D, Uijttewaal WSJ (2020) Bank erosion processes in regulated navigable rivers. J Geophys Res Earth Surf. https://doi.org/10.1029/2019JF005441
Ermilov AA, Fleit G, Conevski S, Guerrero M, Baranya S, Rüther N (2022) Bedload transport analysis using image processing techniques. Acta Geophys. https://doi.org/10.1007/s11600-022-00791-x
Fleit G, Baranya S (2019) An improved particle image velocimetry method for efficient flow analyses. Flow Meas Instrum 69:101619. https://doi.org/10.1016/j.flowmeasinst.2019.101619
Fleit G, Baranya S (2021) Acoustic measurement of ship wave-induced sediment resuspension in a large river. J Waterw Port Coast Ocean Eng 147(2):04021001. https://doi.org/10.1061/(asce)ww.1943-5460.0000627
Fleit G, Baranya S, Krámer T, Bihs H, Józsa J (2019) A practical framework to assess the hydrodynamic impact of ship waves on river banks. River Res Appl 35(9):1428–1442. https://doi.org/10.1002/rra.3522
Fleit G, Baranya S, Rüther N, Bihs H, Krámer T, Józsa J (2016) Investigation of the effects of ship inducedwaves on the littoral zone with field measurements and CFD modeling. Water (switzerland) 8(7):300. https://doi.org/10.3390/W8070300
Fleit G, Hauer C, Baranya S (2021) A numerical modeling-based predictive methodology for the assessment of the impacts of ship waves on YOY fish. River Res Appl 37(3):373–386. https://doi.org/10.1002/rra.3764
Fox JF, Patrick A (2008) Large-scale eddies measured with large scale particle image velocimetry. Flow Meas Instrum 19(5):283–291. https://doi.org/10.1016/j.flowmeasinst.2008.01.003
Fox JF, Belcher BJ (2011) Comparison of macroturbulence measured using decomposition of PIV, ADV and LSPIV data. J Hydraul Res 49(1):122–126. https://doi.org/10.1080/00221686.2010.535704
Fujita I (2017) Discharge measurements of snowmelt flood by space-time image velocimetry during the night using far-infrared camera. Water (switzerland) 9(4):269. https://doi.org/10.3390/w9040269
Fujita I, Kunita Y (2011) Application of aerial LSPIV to the 2002 flood of the Yodo River using a helicopter mounted high density video camera. J Hydro-Environ Res 5(4):323–331. https://doi.org/10.1016/j.jher.2011.05.003
Fujita I, Muste M, Kruger A (1998) Large-scale particle image velocimetry for flow analysis in hydraulic engineering applications. J Hydraul Res 36(3):397–414. https://doi.org/10.1080/00221689809498626
Fuhrman DR, Fredsøe J, Sumer BM (2009) Bed slope effects on turbulent wave boundary layers: 2. Comparison with skewness, asymmetry, and other effects. J Geophys Res 114:C03025. https://doi.org/10.1029/2008JC005053
Gabel F, Garcia XF, Brauns M, Sukhodolov A, Leszinski M, Pusch MT (2008) Resistance to ship-induced waves of benthic invertebrates in various littoral habitats. Freshw Biol 53(8):1567–1578. https://doi.org/10.1111/j.1365-2427.2008.01991.x
Gabel F, Garcia XF, Schnauder I, Pusch MT (2012) Effects of ship-induced waves on littoral benthic invertebrates. Freshw Biol 57(12):2425–2435. https://doi.org/10.1111/fwb.12011
Gabel F, Lorenz S, Stoll S (2017) Effects of ship-induced waves on aquatic ecosystems. Sci Total Environ 601–602:926–939. https://doi.org/10.1016/j.scitotenv.2017.05.206
Gabel F, Stoll S, Fischer P, Pusch MT, Garcia XF (2011) Waves affect predator-prey interactions between fish and benthic invertebrates. Oecologia 165(1):101–109. https://doi.org/10.1007/s00442-010-1841-8
Garcia D (2010) Robust smoothing of gridded data in one and higher dimensions with missing values. Comput Stat Data Anal 54(4):1167–1178. https://doi.org/10.1016/j.csda.2009.09.020
Gottlieb S, Shu C-W (1998) Total variation diminishing Runge-Kutta schemes. Math Comput 67:73–85. https://doi.org/10.1090/S0025-5718-98-00913-2
Holland KT, Puleo JA, Kooney TN (2001) Quantification of swash flows using video-based particle image velocimetry. Coast Eng 44:65–77. https://doi.org/10.1016/S0378-3839(01)00022-9
Houser C (2011) Sediment resuspension by vessel-generated waves along the Savannah River, Georgia. J Waterw Port Coast Ocean Eng 137(5):246–257. https://doi.org/10.1061/(asce)ww.1943-5460.0000088
Jackivicz TP, Kuzminski LN (1973) The effects of the interaction of outboard motors with the aquatic environment-A review. Environ Res 6(4):436–454. https://doi.org/10.1016/0013-9351(73)90058-3
Jacobsen NG, Fuhrman DR, Fredsøe J (2012) A wave generation toolbox for the open-source CFD library: OpenFoam®. Int J Numer Meth Fluids 70(9):1073–1088. https://doi.org/10.1002/fld.2726
Jacobsen NG, Fredsøe J (2014) Formation and development of a breaker bar under regular waves. Part 2: sediment transport and morphology. Coast Eng 88:55–68. https://doi.org/10.1016/j.coastaleng.2014.01.015
Jacobsen NG, Fredsøe J, Jensen JH (2014) Formation and development of a breaker bar under regular waves. Part 1: model description and hydrodynamics. Coast Eng 88(2014):182–193. https://doi.org/10.1016/j.coastaleng.2013.12.008
Kamath A, Bihs H, Chella MA, Arntsen ØA (2015) CFD simulations of wave propagation and shoaling over a submerged bar. Aquatic Procedia 4(2214):308–316. https://doi.org/10.1016/j.aqpro.2015.02.042
Kimmoun O, Branger H (2007) A particle image velocimetry investigation on laboratory surf-zone breaking waves over a sloping beach. J Fluid Mech 588:353–397. https://doi.org/10.1017/S0022112007007641
Kucera-Hirzinger V, Schludermann E, Zornig H, Weissenbacher A, Schabuss M, Schiemer F (2009) Potential effects of navigation-induced wave wash on the early life history stages of riverine fish. Aquat Sci 71(1):94–102. https://doi.org/10.1007/s00027-008-8110-5
Le Coz J, Hauet A, Pierrefeu G, Dramais G, Camenen B (2010) Performance of image-based velocimetry (LSPIV) applied to flash-flood discharge measurements in Mediterranean rivers. J Hydrol 394(1–2):42–52. https://doi.org/10.1016/j.jhydrol.2010.05.049
Liu X-D, Osher S, Chan T (1994) Weighted essentially non-oscillatory schemes. J Comput Phys 115(1):200–212. https://doi.org/10.1006/jcph.1994.1187
Masselink G, Puleo JA (2006) Swash-zone morphodynamics. Cont Shelf Res 26:661–680. https://doi.org/10.1016/j.csr.2006.01.015
Muste M, Baranya S, Tsubaki R, Kim D, Ho H, Tsai H, Law D (2016) Acoustic mapping velocimetry. Water Resour Res 52(5):4132–4150. https://doi.org/10.1002/2015WR018354
Muste M, Fujita I, Hauet A (2008) Large-scale particle image velocimetry for measurements in riverine environments. Water Resources Res 46(4):1–14. https://doi.org/10.1029/2008WR006950
Muste M, Hauet A, Fujita I, Legout C, Ho HC (2014) Capabilities of large-scale particle image velocimetry to characterize shallow free-surface flows. Adv Water Resour 70:160–171. https://doi.org/10.1016/j.advwatres.2014.04.004
Muste M, Ho HC, Kim D (2011) Considerations on direct stream flow measurements using video imagery: Outlook and research needs. J Hydro-Environ Res 5(4):289–300. https://doi.org/10.1016/j.jher.2010.11.002
Nimmo Smith WAM, Atsavapranee P, Katz J, Osborn TR (2002) PIV measurements in the bottom boundary layer of the coastal ocean. Exp Fluids 33(6):962–971. https://doi.org/10.1007/s00348-002-0490-z
Nobach H, Honkanen M (2005) Two-dimensional Gaussian regression for sub-pixel displacement estimation in particle image velocimetry or particle position estimation in particle tracking velocimetry. Exp Fluids 38(4):511–515. https://doi.org/10.1007/s00348-005-0942-3
Otsuka J, Saruwatari A, Watanabe Y (2017) Vortex-induced suspension of sediment in the surf zone. Adv Water Resour 110:59–76. https://doi.org/10.1016/j.advwatres.2017.08.021
Petti M, Longo S (2001) Turbulence experiments in the swash zone. Coast Eng 43:1–24. https://doi.org/10.1016/S0378-3839(00)00068-5
Rapaglia J, Zaggia L, Parnell K, Lorenzetti G, Vafeidis AT (2015) Ship-wake induced sediment remobilization: effects and proposed management strategies for the Venice Lagoon. Ocean Coast Manag 110:1–11. https://doi.org/10.1016/j.ocecoaman.2015.03.002
Ryu Y, Chang KA (2008) Green water void fraction due to breaking wave impinging and overtopping. Exp Fluids 45(5):883–898. https://doi.org/10.1007/s00348-008-0507-3
Sala OE, Chapin FS, Armesto JJ, Berlow E, Bloomfield J, Dirzo R, Huber-Sanwald E, Huenneke LF, Jackson RB, Kinzig A, Leemans R, Lodge DM, Mooney HA, Oesterheld M, Poff NLR, Sykes MT, Walker BH, Walker M, Wall DH (2000) Global biodiversity scenarios for the year 2100. Science 287(5459):1770–1774. https://doi.org/10.1126/science.287.5459.1770
Schallenberg M, Burns CW (2004) Effects of sediment resuspension on phytoplankton production: Teasing apart the influences of light, nutrients and algal entrainment. Freshw Biol 49(2):143–159. https://doi.org/10.1046/j.1365-2426.2003.01172.x
Schludermann E, Liedermann M, Hoyer H, Tritthart M, Habersack H, Keckeis H (2014) Effects of vessel-induced waves on the YOY-fish assemblage at two different habitat types in the main stem of a large river (Danube, Austria). Hydrobiologia 729(1):3–15. https://doi.org/10.1007/s10750-013-1680-9
Shavit U, Lowe RJ, Steinbuck JV (2007) Intensity Capping: A simple method to improve cross-correlation PIV results. Exp Fluids 42(2):225–240. https://doi.org/10.1007/s00348-006-0233-7
Shin S, Cox D (2006) Laboratory observations of inner surf and swash-zone hydrodynamic on a steep slope. Cont Shelf Res 26:561–573. https://doi.org/10.1016/j.csr.2005.10.005
Sussman M (1994) A level set approach for computing solutions to incompressible two-phase flow. J Comput Phys 114(1):146–159. https://doi.org/10.1006/jcph.1994.1155
Tsubaki R, Baranya S, Muste M, Toda Y (2018) Spatio-temporal patterns of sediment particle movement on 2D and 3D bedforms. Exp Fluids 59(6):93. https://doi.org/10.1007/s00348-018-2551-y
Utne-Palm AC (2004) Effects of larvae ontogeny, turbidity, and turbulence on prey attack rate and swimming activity of Atlantic herring larvae. J Exp Mar Biol Ecol 310(2):147–161. https://doi.org/10.1016/j.jembe.2004.04.005
Voulgaris G, Collins MB (2000) Sediment resuspension on beaches: response to breaking waves. Mar Geol 167(1–2):167–187. https://doi.org/10.1016/S0025-3227(00)00025-6
Wang G, Garcia D, Liu Y, de Jeu R, Johannes Dolman A (2012) A three-dimensional gap filling method for large geophysical datasets: application to global satellite soil moisture observations. Environ Model Softw 30:139–142. https://doi.org/10.1016/j.envsoft.2011.10.015
Wang W, Kamath A, Martin T, Pákozdi C, Bihs H (2020) A comparison of different wave modelling techniques in an open-source hydrodynamic framework. J Mar Sci Eng 8(7):526. https://doi.org/10.3390/JMSE8070526
Weitbrecht V, Kühn G, Jirka GH (2002) Large scale PIV-measurements at the surface of shallow water flows. Flow Meas Instrum 13(5–6):237–245. https://doi.org/10.1016/S0955-5986(02)00059-6
Westerweel J, Scarano F (2005) Universal outlier detection for PIV data. Exp Fluids 39(6):1096–1100. https://doi.org/10.1007/s00348-005-0016-6
Wilcox DC (1994) Turbulence modeling for CFD. DCW Industries Inc., La Canada
Acknowledgements
The second author acknowledges the support of the Bolyai János research fellowship of the Hungarian Academy of Sciences. The study was supported by the MTA-BME Water Management Research Group of the Eötvös Loránd Research Network.
Funding
Open access funding provided by Budapest University of Technology and Economics.
Author information
Authors and Affiliations
Corresponding author
Additional information
Publisher's Note
Springer Nature remains neutral with regard to jurisdictional claims in published maps and institutional affiliations.
Rights and permissions
Open Access This article is licensed under a Creative Commons Attribution 4.0 International License, which permits use, sharing, adaptation, distribution and reproduction in any medium or format, as long as you give appropriate credit to the original author(s) and the source, provide a link to the Creative Commons licence, and indicate if changes were made. The images or other third party material in this article are included in the article's Creative Commons licence, unless indicated otherwise in a credit line to the material. If material is not included in the article's Creative Commons licence and your intended use is not permitted by statutory regulation or exceeds the permitted use, you will need to obtain permission directly from the copyright holder. To view a copy of this licence, visit http://creativecommons.org/licenses/by/4.0/.
About this article
Cite this article
Fleit, G., Baranya, S. LSPIV analysis of ship-induced wave wash. Exp Fluids 63, 160 (2022). https://doi.org/10.1007/s00348-022-03508-4
Received:
Revised:
Accepted:
Published:
DOI: https://doi.org/10.1007/s00348-022-03508-4