Abstract
Purpose
To develop and investigate deep learning–based detectors for brain metastases detection on non-enhanced (NE) CT.
Methods
The study included 116 NECTs from 116 patients (81 men, age 66.5 ± 10.6 years) to train and test single-shot detector (SSD) models using 89 and 27 cases, respectively. The annotation was performed by three radiologists using bounding-boxes defined on contrast-enhanced CT (CECT) images. NECTs were coregistered and resliced to CECTs. The detection performance was evaluated at the SSD’s 50% confidence threshold using sensitivity, positive-predictive value (PPV), and the false-positive rate per scan (FPR). For false negatives and true positives, binary logistic regression was used to examine the possible contributing factors.
Results
For lesions 6 mm or larger, the SSD achieved a sensitivity of 35.4% (95% confidence interval (CI): [32.3%, 33.5%]); 51/144) with an FPR of 14.9 (95% CI [12.4, 13.9]). The overall sensitivity was 23.8% (95% CI: [21.3%, 22.8%]; 55/231) and PPV was 19.1% (95% CI: [18.5%, 20.4%]; 98/ of 513), with an FPR of 15.4 (95% CI [12.9, 14.5]). Ninety-five percent of the lesions that SSD failed to detect were also undetectable to radiologists (168/176). Twenty-four percent of the lesions (13/50) detected by the SSD were undetectable to radiologists. Logistic regression analysis indicated that density, necrosis, and size contributed to the lesions’ visibility for radiologists, while for the SSD, the surrounding edema also enhanced the detection performance.
Conclusion
The SSD model we developed could detect brain metastases larger than 6 mm to some extent, a quarter of which were even retrospectively unrecognizable to radiologists.






Similar content being viewed by others
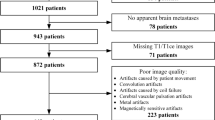
Data availability
The datasets and materials are available upon a reasonable request.
Code availability
The codes are available upon a reasonable request.
Abbreviations
- CE:
-
Contrast-enhanced
- DL:
-
Deep learning
- NE:
-
Non-enhanced
- R-CNN:
-
Region-based convolutional neural network
- SSD:
-
Single-shot detector
References
Valiente M, Ahluwalia MS, Boire A et al (2018) The evolving landscape of brain metastasis. Trends Cancer Res 4:176–196. https://doi.org/10.1016/j.trecan.2018.01.003
Cagney DN, Martin AM, Catalano PJ et al (2017) Incidence and prognosis of patients with brain metastases at diagnosis of systemic malignancy: a population-based study. Neuro Oncol 19:1511–1521. https://doi.org/10.1093/neuonc/nox077
Barnholtz-Sloan JS, Sloan AE, Davis FG et al (2004) Incidence proportions of brain metastases in patients diagnosed (1973 to 2001) in the Metropolitan Detroit Cancer Surveillance System. J Clin Oncol 22:2865–2872. https://doi.org/10.1200/JCO.2004.12.149
Holle D, Obermann M (2013) The role of neuroimaging in the diagnosis of headache disorders. Ther Adv Neurol Disord 6:369–374. https://doi.org/10.1177/1756285613489765
Stelzer KJ (2013) Epidemiology and prognosis of brain metastases. Surg Neurol Int 4:S192-202. https://doi.org/10.4103/2152-7806.111296
Gilbert JW, Johnson KM, Larkin GL, Moore CL (2012) Atraumatic headache in US emergency departments: recent trends in CT/MRI utilisation and factors associated with severe intracranial pathology. Emerg Med J 29:576–581. https://doi.org/10.1136/emermed-2011-200088
Comelli I, Lippi G, Campana V et al (2017) Clinical presentation and epidemiology of brain tumors firstly diagnosed in adults in the Emergency Department: a 10-year, single center retrospective study. Ann Transl Med 5:269. https://doi.org/10.21037/atm.2017.06.12
Chan H-P, Samala RK, Hadjiiski LM, Zhou C (2020) Deep learning in medical image analysis. Adv Exp Med Biol 1213:3–21. https://doi.org/10.1007/978-3-030-33128-3_1
Suzuki K (2017) Overview of deep learning in medical imaging. Radiol Phys Technol 10:257–273. https://doi.org/10.1007/s12194-017-0406-5
Kuo W, Hӓne C, Mukherjee P et al (2019) Expert-level detection of acute intracranial hemorrhage on head computed tomography using deep learning. Proc Natl Acad Sci U S A 116:22737–22745. https://doi.org/10.1073/pnas.1908021116
Arbabshirani MR, Fornwalt BK, Mongelluzzo GJ et al (2018) Advanced machine learning in action: identification of intracranial hemorrhage on computed tomography scans of the head with clinical workflow integration. NPJ Digit Med 1:9. https://doi.org/10.1038/s41746-017-0015-z
Qiu W, Kuang H, Teleg E et al (2020) Machine learning for detecting early infarction in acute stroke with non–contrast-enhanced CT. Radiology 294:638–644. https://doi.org/10.1148/radiol.2020191193
Charron O, Lallement A, Jarnet D et al (2018) Automatic detection and segmentation of brain metastases on multimodal MR images with a deep convolutional neural network. Comput Biol Med 95:43–54. https://doi.org/10.1016/j.compbiomed.2018.02.004
Zhou Z, Sanders JW, Johnson JM et al (2020) Computer-aided detection of brain metastases in T1-weighted MRI for stereotactic radiosurgery using deep learning single-shot detectors. Radiology 295:407–415. https://doi.org/10.1148/radiol.2020191479
Amemiya S, Takao H, Kato S et al (2021) Automatic detection of brain metastases on contrast-enhanced CT with deep-learning feature-fused single-shot detectors. Eur J Radiol 136:109577. https://doi.org/10.1016/j.ejrad.2021.109577
Zhang M, Young GS, Chen H et al (2020) Deep-learning detection of cancer metastases to the brain on MRI. J Magn Reson Imaging 52:1227–1236. https://doi.org/10.1002/jmri.27129
Grøvik E, Yi D, Iv M et al (2020) Deep learning enables automatic detection and segmentation of brain metastases on multisequence MRI. J Magn Reson Imaging 51:175–182. https://doi.org/10.1002/jmri.26766
Dikici E, Ryu JL, Demirer M et al (2020) Automated brain metastases detection framework for T1-weighted contrast-enhanced 3D MRI. IEEE J Biomed Health Inform 24:2883–2893. https://doi.org/10.1109/JBHI.2020.2982103
Liu W, Anguelov D, Erhan D et al (2016) SSD: single shot MultiBox detector. In: Computer Vision – ECCV 2016. Springer International Publishing, pp 21–37. https://doi.org/10.1007/978-3-319-46448-0_2
Whitehead MT, Cardenas AM, Corey AS et al (2019) ACR Appropriateness Criteria® headache. J Am Coll Radiol 16:S364–S377. https://doi.org/10.1016/j.jacr.2019.05.030
He K, Zhang X, Ren S, Sun J (2015) Delving deep into rectifiers: surpassing human-level performance on imagenet classification. In: Proceedings of the IEEE international conference on computer vision. pp 1026–1034
Nair V, Hinton GE (2010) Rectified linear units improve restricted boltzmann machines. In: Proceedings of the Twenty Seventh International conference on Machine Learning, pp 807–814
Kingma DP, Ba LJ (2014) Adam: a method for stochastic optimization. arXiv:14126980
Girshick R, Donahue J, Darrell T, Malik J (2014) Rich feature hierarchies for accurate object detection and semantic segmentation. In: Proceedings of the IEEE conference on computer vision and pattern recognition. pp 580–587
Ren S, He K, Girshick R, Sun J (2017) Faster R-CNN: towards real-time object detection with region proposal networks. IEEE Trans Pattern Anal Mach Intell 39:1137–1149. https://doi.org/10.1109/TPAMI.2016.2577031
Redmon J, Divvala S, Girshick R, Farhadi A (2016) You only look once: unified, real-time object detection. In: Proceedings of the IEEE conference on computer vision and pattern recognition. pp 779–788
Ghatwary N, Zolgharni M, Ye X (2019) Early esophageal adenocarcinoma detection using deep learning methods. Int J Comput Assist Radiol Surg 14:611–621. https://doi.org/10.1007/s11548-019-01914-4
Funding
No funding was received for this study.
Author information
Authors and Affiliations
Corresponding author
Ethics declarations
Conflict of interest
None to declare.
Ethical approval
All generating datasets and analysis were in accordance with the ethical standards of the institutional and/or national research committee and with the 1964 Helsinki declaration and its later amendments or comparable ethical standards.
Informed consent
Informed consent was waived off due to the retrospective nature of the study.
Additional information
Publisher’s note
Springer Nature remains neutral with regard to jurisdictional claims in published maps and institutional affiliations.
Rights and permissions
About this article
Cite this article
Kato, S., Amemiya, S., Takao, H. et al. Automated detection of brain metastases on non-enhanced CT using single-shot detectors. Neuroradiology 63, 1995–2004 (2021). https://doi.org/10.1007/s00234-021-02743-6
Received:
Accepted:
Published:
Issue Date:
DOI: https://doi.org/10.1007/s00234-021-02743-6