Abstract
A smart grid integrates communication networks with the conventional electrical grid. Due to their potential, smart grids are anticipated to achieve widespread deployment. A key component of the success and adoption of smart grids is reliability. Without knowing users’ impressions of the reliability of the smart grid, users will not easily accept and participate in it or its services. However, very few studies address smart grid reliability from the perspective of users. Thus, there is a urgent need to identify key factors that affect smart grid reliability from the user’s viewpoint. The goal of this paper is to examine user perceptions of smart grid reliability and assess their success factors in an effort to close the gap in the literature. This paper propose a model to investigate and determine the most crucial factors that affect the smart grid's reliability based on the Multiple-criteria decision-making (MCDM) method. Firstly, a comprehensive literature analysis was conducted to determine the criteria and sub-criteria used to construct the model; then, the model is constructed using fifteen sub-criteria covering big data, network systems, and grid efficiency criteria; finally, the Fuzzy Analytic Hierarchy Approach (FAHP) and fuzzy triangular numbers are used to evaluate and prioritize the criteria. Twenty smart grid experts were consulted to collect data. The results indicate the significance of the ‘Big Data’ criterion, closely followed by ‘Grid Efficiency’ criterion. Additionally, it is discovered that the sub-criteria of ‘Privacy’ and ‘Interoperability’ had a significant impact on the reliability of the smart grid. The sensitivity analysis shows the variation of factors ranking and the stability and robustness of the model and the results. The research presented in this study has practical applications for academics, engineers, decision-makers, and stakeholders.
Similar content being viewed by others
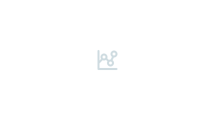
Introduction
The conventional energy grid can no longer keep up with the changes in operational conditions and the rise in electricity needs brought on by the new communication paradigms, such as the Internet of Things (IoT) (Mashal et al. 2015). For instance, compared to traditional homes with few light bulbs and electrical devices, smart homes typically have 500 or more smart devices and use more electricity (Mashal et al. 2020; Shuhaiber & Mashal 2019). To increase the efficiency and stability of the electrical grid, the smart grid has been proposed as a combination of the traditional electrical grid and information and communication technology (ICT) to optimize power generation, transmission, and distribution.
There is no universally accepted definition of a smart grid concept; each nation has its own definition based on its own vision (Dileep 2020; Fang et al. 2012). A smart grid, according to the U.S. Department of Energy, “uses digital technology to improve the reliability, security, and efficiency of the electricity system: from large generation through the delivery systems to electricity consumers and a growing number of distributed generation and storage resources” (Department of Energy 2009). A smart grid is an electrical network that can automatically combine the actions of all users linked to it—generators, consumers, and those who do both—to offer sustainable, affordable, and secure electricity supplies efficiently, according to the European Technology Platform (Bamberger et al. 2006).
To minimize greenhouse gas emissions (i.e., CO2), the smart grid largely relies on distributed energy resources (DERs) and renewable energy sources (RERs), such as solar and wind energy, to produce electricity. Smart grid uses and interconnects a lot of Intelligent Electronics Devices (IEDs) such as smart appliances, power storage units, smart control devices, smart meters, and Advanced Metering Infrastructure (AMI) to enable two-way real-time communications (Ghasempour 2019). Further, to allow Demand Response (DR), Demand-Side Management (DSM), and Electric Vehicles (EV) (Bayindir et al. 2016; Rehmani et al. 2018). The reliability of these technologies and the resulting smart grid are crucial (Ourahou et al. 2020). Reliability is one of the most important evaluation indicators of the smart grid (Sultan and Hilton 2019, Hasan Ghodusinejad et al. 2022).
Smart grids give customers real-time data regarding their electricity consumption patterns and pricing schemes. Thanks to this, users can manage their electricity bills and intelligently monitor and adjust their use habits (Fang et al. 2012). However, because smart grid is still in its early phases of development and the majority of users are unfamiliar with it, people are cautious about embracing and using its services. This could hinder the implementation of the smart grid and impede its development (Lin et al. 2022). It seems sensible that people would utilize smart grid services more frequently if they believe it is more reliable (Raimi and Carrico 2016). Therefore, it is crucial to identify and examine factors that affect smart grid reliability from the perspective of users (Broman Toft et al. 2014; Chou et al. 2014; Hashmi et al. 2011; Ponce et al. 2016). The smart grid's reliability is the smart grid's capacity to deliver regular and continuous functions and services to consumers for extended periods under acceptable conditions. The success of the smart grid concept depends on reliability, which is a key characteristic (Moslehi and Kumar 2010).
Many overlapping and conflicting aspects must be considered when measuring and evaluating the reliability of the smart grid, making it difficult to pinpoint the factors that influence it. Assessing and evaluating the smart grid's reliability might be seen as a challenging Multi-criteria Decision-Making (MCDM) challenge. Based on users’ comparisons and evaluations, MCDM techniques are used to evaluate and prioritize options and criteria that clash. Furthermore, the MCDM frequently focuses on addressing numerous, conflicting criteria at once and relies on both quantitative and qualitative approaches. MCDM can improve decision quality with more efficient and logical procedures than traditional processes, albeit it differs depending on the approaches (Alsalem et al. 2018). Users use natural language to express their subjective opinions. These opinions and judgments could be imprecise, vague, or unclear. Different MCDM techniques are integrated with fuzzy logic and fuzzy set theories to solve these issues.
Little academic research has been done on smart grid reliability from the viewpoint of users, and there has to be more research on the topic (Balali et al. 2023; Bohra and Anvari‐Moghaddam 2022). This work formulates the problem of smart grid reliability evaluation as an MCDM to explore the factors that affect it. In order to review the criteria and sub-criteria that affect the reliability of the smart grid reliability, a literature review is first done. Second, a new model is created to assess and weight these criteria. The model utilizes the triangular membership function and the Fuzzy Analytic Hierarchical Process (FAHP) technique. Third, Jordanian experts in smart grids were interviewed to gather their opinions and preferences regarding these factors. Fourth, the criteria are evaluated by weighting and ranking.
The major goals of this article are to:
-
Examine user perceptions of the smart grid’s reliability.
-
Create and test a model to analyze and evaluate the reliability of the smart grid.
-
Determine the most crucial factors influencing the reliability of the smart grid, then prioritize and rank them.
The rest of this paper is structured as follows. “Literature review” section examines preliminary research and pertinent studies. The methodology used to collect the experts' preferences is described in “Methodology” section. The suggested model is presented in “Research model” Section. The study’s results and findings are presented in “Results and findings” section. The sensitivity analysis of results is presented in “Discussion and implications” Section. Finally, “Conclusion” brings the conclusion of the paper. Abbreviations used throughout the paper are listed in Table 5 in “Appendix”.
Literature review
Using MCDM approaches has generated a lot of interest in recent years as researchers look at the smart grid in various contexts. Thirteen sub-criteria representing social, environmental, economic, and technical issues were utilized by Haddah et al. (2017) to evaluate renewable energy sources in Algeria. The most important factors were discovered to be social and environmental ones. The best source was discovered to be solar energy. Alaqeel and Suryanarayanan (2018) used the FAHP method to prioritize smart grid different technologies in Saudi Arabia using triangular fuzzy numbers. The findings revealed that Advanced Metering Infrastructure (AMI), Advanced Assets Management (AAM), Advanced Transmission Operations (ATO), and Advanced Distribution Operations (ADO) are the top technologies that Saudi electric utilities are interested in.
A model was put up by Zhao and Li (2016) to assess the effectiveness of the smart grid in China. The model has 12 sub-criteria that fall under the four main criteria of economics, society, environment, and technology. The model combines fuzzy Technique for Order Preference by Similarity to Ideal Solution (TOPSIS) and stochastic AHP. The findings indicate that the environment sub-criteria are more significant than the economy sub-criteria. Zhao et al. (2018) select 21 sub-criteria to assess the performance of Chinese energy grid firms. The entropy is used to obtain the weights of objective sub-criteria, whereas the Best–Worst Technique (BWM) is used to find the weights of subjective sub-criteria. Finally, the VlseKriterijumska Optimizacija Kompromisno Resenje (VIKOR) method is employed to assess the alternatives.
Mokhtar et al. (2015) introduce a framework for choosing the most effective demand side management strategies. Six criteria are weighted using the AHP. For rating seven alternatives, TOPSIS and the Preference Ranking Organization Method for Enrichment Evaluation (PROMETHEE) are both employed. Taylan et al. (2020) combined FAHP, TOPSIS, and fuzzy VIKOR to identify the Saudi Arabia’s finest energy systems. The model has nine criteria and eight energy systems that need to be evaluated. A model to assess the advantages of renewable energy taking into account economic, social, and environmental factors was proposed by Zhao and Guo (2015). The Entropy Weighting Method (EWM) is utilized to determine weights of the objective sub-criteria, while the superiority linguistic rating is used to determine the weights of subjective sub-criteria. Then, alternatives are ranked using the fuzzy Grey Relation Analysis (GRA).
Dong et al. (2018) analyzed Chinese demand response systems using combination of FAHP and the Shannon entropy approaches to determine the weights of the various criteria. Additionally, the VIKOR method using trapezoidal fuzzy numbers is employed to rank alternatives. Three important sub-criteria were discovered to be the “Price Elasticity,” “Smart Meter Use Rate,” and “Degree of Smart Home Application.”. Similarly, Dong et al. (2016) utilized FAHP to determine the weights of 15 sub-criteria including economic, social, environmental, and technical criteria and used fuzzy TOPSIS to rate DSM performance in China. A paradigm for evaluating the effectiveness of demand response systems was also developed by Dong et al. (2017) based on the fuzzy VIKOR method and an L2-metric distance approach. The FAHP and Criteria Importance Through Intercriteria Correlation (CRITIC) approaches were combined in the model to determine criteria weights. The criteria were established using the fuzzy Delphi approach. The economy, society, technology, environment, and management were the five key factors chosen. Higher ratings were given to the sub-criteria of society and environment than to the other.
Naseem et al. (2022) proposed a MCDM model to analyze performance of the smart grids in Pakistan based on T-Spherical Fuzzy Power Maclaurin Symmetric Mean (TSFPMSM) operator. Narayanamoorthy et al. (2023) introduced a Linear Diophantine Hesitant Fuzzy Sets (LDHFS) to evaluate five alternatives of energy storage technology in India. Criteria are cost, performance, technology, social, and environmental aspects. Hasheminasab et al. (2023) presented a framework to evaluate Energy Poverty (EP) in EU countries. Indifference Threshold-based Attribute Ratio Analysis (ITARA) method is used to weigh the criteria. Then, Measurement Alternatives and Ranking according to COmpromise Solution (MARCOS) (Stević et al. 2020) is used. Balali et al. (2020) proposed a model to identify and prioritize factors that helps in reducing energy consumption in buildings based on SWARA and COPRAS methods. Similarly, Balali et al. (2021) used BWM and EDAS to analyze and rank strategies used to reduce energy consumption in hospitals. Ghodusinejad (2022) investigate the performance of a PV system in five different cities in Iran to select the best place to deploy PV system. The cities are ranked based on SMART method.
Shorabeh et al. (2021) employed FANP to investigate environmental and economic criteria to select the best locations to establish renewable energy farms in Iran. Weighted Linear Combination (WLC) method was used to conduct suitability analysis. Usman et al. (2022) assess the energy systems in various nations using CRITIC-TOPSIS, which combines criteria including energy savings, electricity cost, and thermal energy cost. Krishankumar et al. (2022) apply attitudinal Choquet integral and hesitant fuzzy linguistic to measure criteria importance, then they apply CRITIC to rank renewable energy alternatives. Dagtekin et al. (2022) proposed a model to select the best distributed generation systems out of seven to be used in Turkish building. Five criteria are weighted using AHP and six various raking method are used to rank alternatives. Soomro et al. (2022) use the AHP to rank three cook stove technologies based on four criteria and 12 sub-criteria in Pakistan. Criteria include environmental, technological, social, and economical. Reliability is considered as a single sub-criterion of the technological criterion, without breaking it down. Mehr et al. (2022) propose a model to select the best solar panels technology of nine used technologies. The model is based on five criteria (economic, climate, technical, mechanical, and electrical aspects) and 20 sub-criteria. The best–worst technique is used to weight the criteria and MULTIMOOSRAL method is used to rank alternatives. Lakshmi et al. (2022) use TOPSIS to rank and select the best alternative of six for bioenergy source. The CRITIC and Entropy methods are used to weight seven selected criteria. Manirambona et al. (2022) used AHP and TOPSIS methods to evaluate Kenyan power planet using economic, social, environmental, and technical factors.
The goal of all prior research has been to determine the optimum electricity source or to evaluate electrical technology based on four factors: social, technological, environmental, and economic (Siksnelyte-Butkiene et al. 2020). Technological criteria include devices performance time, renewable fraction, and reliability. However, these studies consider reliability as a factor and don’t further study it deeply and break it down to smaller components. For example, Yousefi et al. (2022) considered reliability as the need to import energy from outside the energy grid in a specific province. This study ignores social, economic, and environmental factors in favor of focusing solely on technology criteria of smart grid.
Methodology
Data collection
The fuzzy comparisons matrix is built based on twenty expert’s opinions and evaluation of criterion and sub-criteria using linguistic variables through a questionnaire. All of the experts have more than four years of specialized field or research expertise in the IoT and smart grid. Five of the twenty experts are professors at universities who are now researching IoT and smart grids. Five experts occupy position in electrical utilities, including management and engineering. Four experts are working in the Jordanian Electricity Regulatory Commission. Three experts are from information and communication technology industry sector and the last three experts are from the telecommunication operators. The questionnaire was conducted in October and November 2022. It is worth mentioning that the majority of the MCDM research articles in the energy and smart grid context are done with the help of expert consultation (Estévez et al. 2021).
Data analysis
Eighteen respondents were accepted because their CR value is less than 0.1, which ensures the consistency of their answers. The CR values for the other two respondents were between 0.1 and 0.2, and thus not used for analysis. The geometric mean approach was used to aggregate the eighteen accepted respondents (Forman and Peniwati 1998).
The two rejected respondents could be resulted from the nature of FAHP. As mentioned before, FAHP method depends on expert’s subjective judgments. Thus, experts need to fully understand the FAHP process and its limitations in addition to understand the criteria and the subcriteria in the model to get consistant results and avoiding indistinct and imprecise decisions. To ensure minimum inconsistance, the experts were informed about the objectives of the study, the criteria and sub-criteria, and the fuzzy linguistic terms and how these are to be used in the comparison.
Fuzzy analytic hierarchy process
Many different methods have been used to calculate criteria weights. These methods include DEMATEL, BWM, SWARA, and many other. However, these methods face drawbacks. For example, BWM assume that there is only one best criterion and one worst criterion, which is not the case in this study where criteria are overlap with high uncertainty. Thus, BWM cannot be used in this study. Traditional AHP is an effective approach for resolving MCDM and has been employed in several research across numerous areas. However, AHP, and other conventional MCDM methods, faces difficulties when specialists make indistinct, vague, and imprecise choices and judgments. Thus, the AHP method was integrated with fuzzy set theory to create the FAHP, which is more effective than standard AHP for computing conflicting criterion weights (Ilbahar et al. 2019; van Laarhoven & Pedrycz 1983). In fact FAHP is the mostly used method for MCDM problems in energy context. This justify using FAHP for criteria weighting in this study. Due to their simplicity, the Triangular Fuzzy Numbers (TFNs), Fig. 1, and triangular membership function, Eq. 1, are frequently utilized with FAHP models (Basílio et al. 2022). TFNs are represented by three numbers representing high, middle, low values, and are denoted by \(\widetilde{A}=(l,m,u)\).
Linguistic variables are utilized to compare criteria and sub-criteria importance against each other and results are mapped to TFN and stored in the fuzzy comparison matrix. For instance, when two criteria are thought to have equal importance, they are given the fuzzy weight \(\widetilde{1}\) which is mapped to (1,1,1) TFN. When one criterion is estimated strongly more important than the other criterion, it gets the fuzzy weight equal to \(\widetilde{7}\) which is represented as TFN (6,7,8). Next, the fuzzy comparison matrix is checked for consistency using both the Consistency Index (CI) and the Consistency Ratio (CR), which are given in Eq. (2) and Eq. (3), respectively. CI is calculated based on the number of criteria (n) and the maximum eigenvalue of the fuzzy comparison matrix (\({\uplambda }_{\mathrm{max}}\)). CR is calculated using Random Index (\(RI\)) which is a fixed random value that relies on the number of criteria. When the CR value of the fuzzy comparison matrix is less than 0.10, it is allowed to use it.
In the following steps, the fuzzy weight for every row is determined using the geometric mean as given in Eq. (4) (Buckley 1985) and the fuzzy weights for each criterion is calculated using Eq. (5), which need to be converted to crisp weights by applying the de-fuzzification process using Center of Area (COA) approach as shown in Eq. (6) (Chou and Chang 2008).
The crisp weights are normalized using Eq. (7). The weights of the alternatives are multiplied by the weight of the relevant criterion to determine the global weight of the alternatives using Eq. (8). Finally, alternatives are sorted, and the best alternative is chosen based on its global weight.
Research model
In this section we present a three layers model. The top layer of the model represents the objective; the identification and prioritization of the factors influencing the reliability of the smart grid. The second layer defines main criteria. The sub-criteria for the second layer’s criteria are contained in the third layer. In order to identify different criteria and sub-criteria to be used in the model, an extensive literature review was carried out. Various popular criteria were identified and analyzed, these criteria cover economic, environmental, social, and technological aspects. It was clearly noticed that no literature considered the interactions of big data criteria and smart grid reliability. Irrelevant criteria to the objectives of the paper was neglected. Then, the criteria were judged by smart grid experts to select the most important criteria. Based on their experiences, three primary criteria were selected: (1) the ‘Network System’ criterion focuses on the communication network system’s requirements and specifications. (2) the “Big Data” criterion represents the properties and characteristics of big data processing. (3) the ‘Grid efficiency’ criterion assess the capabilities of smart grid. The hierarchy is displayed in Fig. 2.
Network system
Various communication network types can be found in smart grids. The smallest network for tying together smart home equipment and appliances is called the Home Area Network (HAN). HANs account for more than 50% of total electricity consumption (Heile 2010). The backbone of the communication networks that connects all smart grid components together is the Wide Area Networks (WAN). Neighborhood Area Network (NAN) is a network that sits in the middle of HANs and WANs, transferring data between them (Kuzlu and Pipattanasomporn 2013). These networks differ in their complexity, heterogeneous architectures, technologies, and requirements that should be satisfied (security, bandwidth, and coverage range) (Kuzlu and Pipattanasomporn 2013; Kuzlu et al. 2014). We specify the main sub-criteria listed below:
-
1.
Network technology: Different technologies are used by smart grid components to communicate and exchange data in a two-way manner. These technologies are either wired or wireless varying in their data rate, coverage area, and Quality of Service (QoS) of these technologies vary (Fang et al. 2012). Digital Subscriber Line (DSL), Fiber-optic, and Power Line Communications (PLC) are wired technologies. ZigBee, WiFi, WirelessHART, and 6LoWPAN are wireless technologies (Ancillotti et al. 2013; Tightiz et al. 2020). Due to their interesting characteristics, such as quick deployment, affordable installation, high mobility, and flexibility, wireless technologies are becoming prevalent in the smart grid.
-
2.
Delay: Smart grid deals with very sensitive and timely data that needs fast transmission and immediate processing. The smart gird’s reliability is significantly impacted by delay (Ghasempour 2019). In fact, different delay requirements apply to different smart grid applications. For example, data transfer between smart equipment and data aggregation centers should be completed in a matter of milliseconds as opposed to the longer time required for data transfer between control centers (Alam et al. 2017; Gao et al. 2012).
-
3.
Network security: Smart grids are susceptible to both deliberate and inadvertent attacks, on their infrastructures. Attackers might exploit smart grid vulnerabilities to gain access and sabotage or disrupt the smart grid operations (Yan et al. 2013). Smart grid must ensure continues operations and minimum network failures by deploying different security mechanisms such as encryption and intrusion detection (Hawk and Kaushiva 2014).
-
4.
Data rate: Smart grid applications have different data rate requirements (Bian et al. 2019) depending on the protocols utilized and the kind of network and the protocols that are used (Daki et al. 2017; Qarabsh et al. 2020). Zigbee, for instance, has a low data rate when compared to other technologies.
Big data
Various components of smart grid, such smart meters, generate large volume of data which requires analyzing and processing. In order to benefits to the smart grid utility, they must manage, store, process, analyze, and visualize this data to get insights (Qarabsh et al. 2020; Stefan et al. 2017). The following are some challenges related to big data that smart grids must overcome.
-
1.
Heterogeneous data: The generation and collection of data in the smart grid occurs on a massive scale from enormous smart devices, making the type, format, quality, and completeness of this data diverse. Data in the smart grid, for instance, can be unstructured, semi-structured, or structured, which makes it challenging to process, integrate, and analyze the data (Wang 2017).
-
2.
Data visualization: Proper, accurate, and meaningful display of real-time data is crucial for the smart grid utility and the customers. Visualization tools enable smart grid utilities to monitor behavior, evaluate, analyze, and enhance the grid quality and performance. Users are able to control their electricity usage and electricity bills thanks to the visualization of the electricity consumption of their smart home appliances (Saleem et al. 2019). However, smart grid data is high dimensional which makes it difficult to choose the best data visualization method (Daki et al. 2017; Nga et al. 2012; Saleem et al. 2019; Sanchez-Hidalgo and Cano 2018; Sanchez and Rivera 2017; Stefan et al. 2017).
-
3.
Data storage: Smart grid utility must store all generated data in order to be processed later. The decision on data storage is what allows smart grid utilities to effectively process the massive volume of generated data (Diamantoulakis et al. 2015). Smart grid utility can store data in one location in a centralized manner or in multiple different sites in a distributed fashion using powerful and effective cloud computing platforms (Daki et al. 2017; Lněnička 2015; Siddiqa et al. 2017).
-
4.
Data analytics: In order to efficiently manage and analyze the massive amount of real-time data and optimize the operation of the smart grid, using analytics technologies are crucial (Diamantoulakis et al. 2015; Saleem et al. 2019). Two data analytics methods are employed; MapReduce and stream. MapReduce technique divides non-real-time and non-sensitive data into small sets (batches) that are processed simultaneously. On the other hand, as its name implied, stream processing stream and process massive data in real-time and great fault tolerance (Saleem et al. 2019), which makes it suitable to be used in smart grids (Jaradat et al. 2015; Lněnička 2015).
-
5.
Data privacy: Data privacy is a major concern for customers since the smart grid utilities collect user personal information including location, electricity use, and usage patterns. These data are susceptible to risks and attacks and can be used maliciously. Therefore, smart grid utilities are responsible to ensure that users’ data is protected and kept private by making sure that only users with the proper authorizations may access private data and that unauthorized users cannot gain or access this data. To protect data privacy, the smart grid must use several strategies at each tier of its architecture. Data aggregation, data anonymization, authentication, and trust management are some of these methods (Bari et al. 2014; Jain et al. 2016; Wang et al. 2019).
Grid efficiency
Smart grid has been developed as the next generation electricity grids to address problems of the traditional grids such energy waste and rising demand. Indeed, smart grids utilize number of advanced functions and cutting-edge technologies to facilitate its operations (Gârdan et al. 2023). Therefore, improving the functionality of the smart grid’s and all its systems is crucial. To assess the effectiveness of smart grid functions, the following sub-criteria are employed.
-
1.
Interoperability: Ensuring that the smart grid’s heterogeneous components can cooperatively use, share, and exchange data presents one of the key obstacles in smart grid. The smart equipment in the smart grid needs a common consensus on the data and message formats and communication protocols utilization in order to function properly (Saleem et al. 2019).
-
2.
Self-healing: The smart grid’s self-healing capability is one of its key features. The smart grid utilities’ extensive deployment of smart devices and sensors enables it to remotely monitor and assess the smart grid’s status as well as the condition of every piece of equipment. This makes it possible for smart grid utilities to immediately respond to failures, isolate malfunctioning parts, reduce blackouts, and discover and evaluate problems without the need for human involvement. Long electricity outages generate financial loss, which is reduced by smart grid self-healing capabilities (Dileep 2020; Zhao and Li 2016). The smart grid will be more reliable as a result.
-
3.
Scalability: The majority of smart grids today are deployed on a modest scale with local experimental design. Globalization of the smart grid, however, necessitates large-scale implementation (Ma et al. 2018; Rodriguez-Calvo et al. 2018; Sigrist et al. 2016). On a wide scale, smart grid will confront severe difficulties and might not function properly. Scalability is the main element that lowers obstacles for smart grid implementation on a broad scale. Scalability enables the smart grid to handle more smart objects and applications without impacting its performance. Scalable smart grid solutions and technologies are necessary to avoid failures of its operations and functions.
-
4.
Availability: A smart grid should be able to operate continuously and uninterruptedly at all times by guaranteeing the availability and functionality of its elements and equipment and preventing or minimizing malfunctions and breakdowns. The smart grid must also give users quick and direct access to the real-time personal information that is gathered from their smart devices whenever they choose (Fan & Gong 2013; Pokorni 2019).
-
5.
Controllability: Controllability has a significant impact on the reliability of the smart grid. The entire grid and its subsystems, including demand-side response, energy generation, and energy storage, must be under the supervision of smart grid utilities (Léonard et al. 2016). For instance, in case of emergency, utility companies should be capable to disconnect energy or any of its services of a specific user at any moment. However, this will be a big concern for the users since it can be done without users’ permission or without prior notification (AlAbdulkarim et al. 2012).
-
6.
Standardization: As mentioned before, smart grid is based on heterogeneous smart devices and communication technologies. Thus, standardization plays a crucial role in smart grid realization. Smart grid standardization aims to achieve interoperability between the heterogeneous components. Several standardization efforts have taken place on smart grids by organizations such as IEEE, ANSI, NIST, and IETF. However, these are individual standardization efforts focus on standardization of communication, more efforts are needed to address other issues (Saleem et al. 2019).
Results and findings
Local weights of criteria
Table 1 displays comparison results of the main criteria. As can be seen from the table, the “Big Data” is the most significant criterion and is deemed to be four times more significant than the “Network System” criterion and equally important with the “Grid Efficiency” criterion. It is determined that the “Grid Efficiency” criterion is three times more significant than the “Network System”. By using Eq. (4)–(7) the comparison results in Table 1 is converted to weights for all the three criteria. The “Big Data” is the most significant criterion with the highest weight equals 0.46, followed by the “Grid Efficiency” criterion has a weight of 0.41 while “Network System” criterion has a weight value of 0.13.
Compared to the other “Big Data” sub-criteria, the “Data Privacy” received the highest local weight of 0.52. The “Data Analytics” comes next with a weight of 0.23, followed by the “Data Visualization” weight is 0.13, The “Data Storage” has a weight of 0.07, and the “Heterogeneous Data” a weight of 0.05. Comparing the sub-criteria associated with the ‘Network System’ reveals that the ‘Network Security’ received the highest local weight, which is equivalent to 0.53. The ‘Delay’ received a local weight of 0.34 and is ranked second. The ‘Network Technology’ local weight is 0.08 and rated third. The ‘Data Rate’ is in the fourth position with 0.05 score. The ‘Grid Efficiency’ criterion has the largest number of sub-criteria. The weights for the ‘Interoperability’, the ‘Availability’, and the ‘Scalability’ are each equal to 0.34, 0.23, and 0.15, respectively. The ‘Self-Healing’ has a weight of 0.11, followed by ‘Controllability’ which scored a weight of 0.08. The ‘Standardization’ criterion was rated as the least essential criterion with a score of 0.06.
Global weights of criteria
To calculate the global weight for each sub-criteria, the local weights of the sub-criteria (optained from the previous sub-section) are multiplied by the weight of the main criteria that they are linked to in the hierarchy using Eq. (8). For instance, the global weight for the sub-criterion “Data Privacy” is calculated by multiplying its local weight (0.52) by the local weight of “Big Data” main cretrion (0.46). The result equals (0.236). Based on the overall global weights, sub-criteria are ranked and prioritized. Table 2 lists the sub-criteria’s weights and rankings.
The six sub-criteria of the ‘Grid Efficiency’ main criterion occupy second, fourth, sixth, eighth, and tenth places. This shows importance of the ‘Grid Efficiency’. Experts highly evaluate the crucial role ‘Grid Efficiency’ plays in smart grid reliability. The ‘Interoperability’ is ranked second receiving the global weight of 0.143. The ‘Availability’ in fourth place with global weight of 0.096. The ‘Scalability’ is placed at sixth place with global weight equal to 0.065. The ‘Self-Healing’, the ‘Controllability’, and the ‘Standardization’ are found to be less significant with global weights 0.047, 0.035, and 0.027, respectively.
The ‘Privacy’ criterion of the ‘Big Data’ scores global weight 0.236 and occupies the top of the ranking indicating its importance. The ‘Analytics’ global weight equal of 0.107 makes it in the third place. The ‘Visualization’ comes in the seventh place with global weight 0.061 and the ‘Storage’ at the eleventh place with global weight equal of 0.033. The ‘Heterogeneous data’ criterion, which is ranked thirteenth and has a global weight of 0.019, is the least significant criterion.
The most important criterion for the ‘Network System’ is “Network security,” which ranks fifth and its global weight is 0.07. The ninth-placed is occupied by the ‘Delay’ and is given a global weight of 0.045. The ‘Network Technology’ and the ‘Data Rate’ are judged to be the least important, ranking fourteenth and fifteenth, with global weights of 0.01 and 0.007, respectively.
Sensitivity analysis
Sensitivity analysis must be carried out in order to evaluate the proposed model’s and results’ robustness (Triantaphyllou and Sánchez 1997; Yazdani et al. 2016). Sensitivity analysis shows how changing the weights of the criteria might affect how they are ranked (Govindan et al. 2014). Since “Big Data” has the biggest weight of the three primary criteria, we alter its weight. The weight of the “Big Data” criterion is increased from 0.1 to 0.9 with a step equal to 0.1. The “Interoperability” criterion is rated first with the maximum global weight of 0.235 when the “Big Data” criterion weight is set to 0.1. The global weight of 0.16 makes the “Availability” in the second place. The ‘Network security’ comes in third with the weight of 0.11. On the other hand, the ‘Heterogeneous data’ is rated last and has the lowest global weight of 0.004. The ‘Interoperability’ maintains the first place when the ‘Big Data’ weight is 0.2 and 0.3. When the “Big Data” weight reaches 0.4, many changes can be reported. The ‘Interoperability’ takes over the second place. The ‘Privacy’ rises to first place with global weight equal to 0.207, while the ‘Availability’ is degraded to third place. Table 3 displays the changes to the global weights of all sub-criteria. Figure 3 illustrates the effect of changing “Big Data” criterion weight on sub-criteria ranking. From the explanation above, it can be seen that the main criterion of “Big Data” significantly affects the reliability of the smart grid.
Table 4 shows the changes in sub-criteria ranking as their weights change. To improve the readability of the paper, we make bold the top ranked sub-criterion in each weight variance of the “Big Data” criterion.
Discussion and implications
This paper suggests an MCDM model to analyze and examine various criteria that play a significant role in the smart grid reliability. By addressing the research question: What are the significant factors that influence the reliability of the smart grid from the perspective of users? This study’s implications assist smart grid utilities in delivering reliable and effective functions and services. The findings of this study demonstrate the importance of ‘Big Data’ in comparison to other criteria. The results show that the ‘Privacy’ criterion is quite important. Users of traditional information systems and technology have always been quite concerned about their privacy. Emerging technologies and innovations bring more challenges and threats for the privacy. In the context of a smart grid, collected data can disclose a lot about the customers may be used to threaten them in different ways. Thus, smart grid utilities need to deploy advances technologies and tools to protect the privacy.
The second most influential factor is the ‘Interoperability’. As mentioned previously, smart grids incorporate large number of smart equipment and devices. These devices have different features and capabilities by nature. Therefore, overcoming heterogeneity and maintaining interoperability among these various devices is essential for the reliability of the smart grid. Smart grid utilities and smart device providers may collaborate to compel interoperability by integrating used communication protocols, architecture, and functionalities. Smart grid utilities can analyze and manage collected data thanks to the analytics technologies and tools. Smart grid utilities must utilize powerful tools and algorithms to process collected data and to derive insights from this data this will eventually increase the reliability of the smart grid. Additionally, researchers and academics should put more effort into researching to create smart grid analytics tools. The ‘‘Interoperability’criterion was found to have moderated importance in (Mashal 2022).
An interesting finding of this paper is that "Privacy" and "Interoperability" were found important and are ranked highly. This is due to the fact that the experts highly evaluated these two sub-criteria under their corresponding main criteria. The "Privacy" received the highest evaluation from the experts, similarly, the “Interoperability” was the most important criterion under the “Grid Efficiency”. The experts are aware of the critical role and the mutual relation between both privacy and interoperability in smart grid. Experts emphasize that ensuring interoperability among different components of smart grid should not affect or harm the "Privacy". It is clear that giving more importance to one of them will reducing the importance of the second one. For example, when the "Big Data" criterion weight was set to 0.1, the "Interoperability" was ranked first and "Privacy" was ranked eighth. When the "Big Data" criterion weight was set to 0.9, the "Privacy" was ranked first and the "Interoperability" was ranked sixth. This could be understood in light of the conflict relation between both criteria. It is worth mentioning that the relation between the “Privacy” and the “Interoperability” and their effect on smart grid reliability have not been studied from the perspective of users before.
The ‘Availability’ of their services must be guaranteed by smart grid utilities. In order to provide decent level of availability, smart grid utilities must, in practice, incorporate three separate levels: defending against attacks, guaranteeing continuity of essential real-time operations, and improving communications architecture and infrastructure. For instance, smart grid utilities can utilize technologies, such as cognitive radio, to ensure availability of their grids. These technologies expand bandwidth, improve channel utilization, and strengthening resource sharing. Future studies of the availability of the smart grid should be conducted (Kim 2012). The ‘Availability’ criterion was found to have moderated importance in (Mashal 2022). It’s interesting to note that the smart grid’s ‘Self-Healing’ criterion was shown to have little influence on its reliability. The same result was mentioned in (Zhao and Li 2016).
The sub-criteria “Network Security” is considered important. Attacks on the smart grid put its reliability in danger and erode customers’ trust in it. Monitoring, data capturing, and data manipulation are security attacks on smart grids. Smart device security vulnerabilities are another possible security risk for the smart grid. Smart devices lack update procedures to reduce security vulnerabilities, making them extremely vulnerable to attacks. Therefore, smart grid utilities must identify and handle every security threat and risk using authorization, authentication, and encryption techniques to safeguard data transit and storage. Researchers and smart grid utilities are urged to design and create new and effective defense mechanisms. It is worth mentioning that “Network Security” was found to be an important factor for smart grid reliable operation (Mashal 2021) and was found to have moderated importance in (Mashal 2022).
Despite the fact that smart grid lack of standards (Luthra et al. 2014), the ‘Standardization’ was found to be insignificant criterion. One reason for this result is that experts don’t think ‘Standardization’ is urgent at this stage since creating standards needs long time. It is worth mentioning that ‘Standardization’ was found to be insignificant in the general IoT domain (Mohammadzadeh et al. 2018). But in order to overcome smart grids difficulties and challenges and ensure system interoperability, standards are necessary. Traditional electricity grid standards for electricity generating and distribution which are currently used are required to be replaced by standards designed especially for smart grids. The smart grid standardization compasses many topics such as smart grid design, communication protocol, data processing methods, and security requirements. As a result, it is important for different smart grid stakeholders to create a standard for the smart grid, which will ensure the interoperability (Daki et al. 2017).
This study enhances the literature on smart grids and enriches our understanding of them. The suggested model uses a fuzzy AHP approach to identify and rank the criteria that have an impact on the reliability of the smart grid. The current work is the first to investigate the criteria and sub-criteria employed in the suggested model. Understanding smart grid reliability from the viewpoint of users is made possible by this study. It ought to motivate scientists to verify the suggested model more thoroughly and look into new criteria for a clearer comprehensive understanding of smart grid reliability.
This study offers several practical applications that are helpful. The empirical findings of this study provide critical considerations and reflections for decision-makers, stakeholders, and managers of smart grid utilities. It is crucial for smart grid utilities to pay attention to the issues presented in this study since implementing the smart grid with all of its intricate sub-systems and services takes a large commitment of both time and money. The creation of a thorough evaluation methodology to assess the reliability of the electric power sector generally and the smart grid particularly is of utmost relevance. Smart grid managers and decision-makers need to understand how customers view the technology in order to provide successful services and operations. By presenting several expert ideas, viewpoints, and concerns, this study represents a significant step in the direction of understanding smart grid reliability. The insightful information provided in this study can also serve as the foundation for manufacturers and suppliers of smart objects to create stronger and more reliable products.
The work in this paper is both theoretically and practically robust. However, there are some limitations. First, the number of criteria and sub-criteria used to build the model is relatively small; many other criteria can be integrated in the model to get holistic understanding of smart grid reliability. Criteria include resiliency, software component, software reliability, and standards have received very little attention from researchers. Thus, researchers are advised to study and analyze these criteria based on expert’s opinion to help improving smart grid reliability. Second, the number of interviewed experts is small and from one country, Jordan. There is a need to interview more experts from different countries to avoid cultural and demographical biases and perform cross-courtiers study.
Conclusion
The huge potentials and capabilities of smart grids make it plays a key role in our daily lives in the near future. A key factor for the success and adoption of smart grids is reliability. This study proposes a model that uses three criteria and fifteen supporting sub-criteria to assess smart grid reliability from the viewpoint of users. The FAHP approach is used to analyze the model and the data that was gathered. This study differ from those in the literature review by integrating the concept of big data and its different dimensions to improve reliability and efficiency of smart grid. No previouse studies have considered this relation and analyzed it to study its effect on smart grid reliability. Moreover, previouse studies have addressed smart grid reliability from technical perspectives. This study is one of the first studies to investigate smart grid reliability fom experts prospective. The results demonstrate that the major criterion ‘Big Data’ is the most significant, while the main criterion ‘Networks System’ is viewed as the least significant. Moreover, among all the sub-criteria, the data ‘Privacy’ obtained the highest global weight, while the sub-criterion ‘Data Rate’ was determined to be the least important. The sensitivity analysis demonstrates reliability, effectiveness, and robust of the suggested model. Helpful implications to many stakeholders such as academics, engineers, and decision-makers are presented. Future research may use qualitative and fuzzy numbers to interpret the decision-maker’s imprecision, employ the QFD method to consider stakeholders’ attitudes and needs, and assess the smart grid reliability in light of unstable economic, social, and cultural conditions. Additionally, in more complicated situations, it can be necessary to weigh criteria using different approaches like ANP. In future work, we plan to use other fuzzy methods, such as Gaussian fuzzy numbers, Trapezoidal Fuzzy Numbers (TrFNs), Hesitant Fuzzy Sets (HFS) and Intuitionistic Fuzzy Sets (IFS), to calculate criteria weights and compare the results of these different method.
Availability of data and materials
The datasets used and/or analysed during the current study are available from the corresponding author on reasonable request.
References
AlAbdulkarim L, Lukszo Z, Fens T. Acceptance of privacy-sensitive technologies: smart metering case in the Netherlands. In: Third international engineering systems symposium CESUN. 2012.
Alam S, Sohail MF, Ghauri SA, Qureshi IM, Aqdas N (2017) Cognitive radio based smart grid communication network. Renew Sustain Energy Rev 72:535–548. https://doi.org/10.1016/j.rser.2017.01.086
Alaqeel TA, Suryanarayanan S (2018) A fuzzy analytic hierarchy process algorithm to prioritize smart grid technologies for the Saudi electricity infrastructure. Sustain Energy Grids Netw 13:122–133. https://doi.org/10.1016/j.segan.2017.12.010
Alsalem M, Zaidan A, Zaidan B, Hashim M, Albahri OS, Albahri AS, Hadi A, Mohammed K (2018) Systematic review of an automated multiclass detection and classification system for acute Leukaemia in terms of evaluation and benchmarking, open challenges, issues and methodological aspects. J Med Syst 42:1–36
Ancillotti E, Bruno R, Conti M (2013) The role of communication systems in smart grids: architectures, technical solutions and research challenges. Comput Commun 36(17):1665–1697. https://doi.org/10.1016/j.comcom.2013.09.004
Balali A, Valipour A (2021) Prioritization of passive measures for energy optimization designing of sustainable hospitals and health centres. J Build Eng 35:101992. https://doi.org/10.1016/j.jobe.2020.101992
Balali A, Hakimelahi A, Valipour A (2020) Identification and prioritization of passive energy consumption optimization measures in the building industry: an Iranian case study. J Build Eng 30:101239. https://doi.org/10.1016/j.jobe.2020.101239
Balali A, Yunusa-Kaltungo A, Edwards R (2023) A systematic review of passive energy consumption optimisation strategy selection for buildings through multiple criteria decision-making techniques. Renew Sustain Energy Rev 171:113013. https://doi.org/10.1016/j.rser.2022.113013
Bamberger Y, Baptista J, Belmans R, Buchholz BM, Chebbo M, Doblado JL, Efthymiou V, Gallo L, Handschin E, Hatziargyriou N (2006). Vision and strategy for europe’s electricity networks of the future: European Technology PlatformSmartGrids
Bari A, Jiang J, Saad W, Jaekel A (2014) Challenges in the smart grid applications: an overview. Int J Distrib Sens Netw 10(2):974682
Basílio MP, Pereira V, Costa HG, Santos M, Ghosh A (2022) A systematic review of the applications of multi-criteria decision aid methods (1977–2022). Electronics 11(11):1720
Bayindir R, Colak I, Fulli G, Demirtas K (2016) Smart grid technologies and applications. Renew Sustain Energy Rev 66:499–516
Bian D, Kuzlu M, Pipattanasomporn M, Rahman S, Shi D (2019) Performance evaluation of communication technologies and network structure for smart grid applications. IET Commun 13(8):1025–1033
Bohra SS, Anvari-Moghaddam A (2022) A comprehensive review on applications of multicriteria decision-making methods in power and energy systems. Int J Energy Res 46(4):4088–4118
Broman Toft M, Schuitema G, Thøgersen J (2014) The importance of framing for consumer acceptance of the smart grid: a comparative study of Denmark, Norway and Switzerland. Energy Res Soc Sci 3:113–123. https://doi.org/10.1016/j.erss.2014.07.010
Buckley JJ (1985) Fuzzy hierarchical analysis. Fuzzy Sets Syst 17(3):233–247. https://doi.org/10.1016/0165-0114(85)90090-9
Chou SW, Chang YC (2008) The implementation factors that influence the ERP (enterprise resource planning) benefits. Decis Support Syst 46(1):149–157. https://doi.org/10.1016/j.dss.2008.06.003
Chou JS, Yutami IG (2014) Smart meter adoption and deployment strategy for residential buildings in Indonesia. Appl Energy 128:336–349. https://doi.org/10.1016/j.apenergy.2014.04.083
Dagtekin Y, Kaya S, Besli N (2022) Distributed energy system selection for a commercial building by using multi criteria decision making methods. Int J Hydrog Energy 47(86):36672–36692. https://doi.org/10.1016/j.ijhydene.2022.08.208
Daki H, El Hannani A, Aqqal A, Haidine A, Dahbi A (2017) Big Data management in smart grid: concepts, requirements and implementation. J Big Data 4(1):1–19
Department of Energy U (2009). 2010 Smart grid system report
Diamantoulakis PD, Kapinas VM, Karagiannidis GK (2015) Big data analytics for dynamic energy management in smart grids. Big Data Res 2(3):94–101. https://doi.org/10.1016/j.bdr.2015.03.003
Dileep G (2020) A survey on smart grid technologies and applications. Renew Energy 146:2589–2625. https://doi.org/10.1016/j.renene.2019.08.092
Dong J, Huo H, Guo S (2016) Demand side management performance evaluation for commercial enterprises. Sustainability 8(10):1041
Dong J, Huo H, Liu D, Li R (2017) Evaluating the comprehensive performance of demand response for commercial customers by applying combination weighting techniques and fuzzy VIKOR approach. Sustainability 9(8):1332
Dong J, Li R, Huang H (2018) Performance evaluation of residential demand response based on a modified fuzzy VIKOR and scalable computing method. Energies 11(5):1097
Estévez RA, Espinoza V, Ponce Oliva RD, Vásquez-Lavín F, Gelcich S (2021) Multi-criteria decision analysis for renewable energies: research trends, gaps and the challenge of improving participation. Sustainability 13(6):3515
Fan X, Gong G (2013) Security challenges in smart-grid metering and control systems. Technol Innov Manag Rev 3(7):42
Fang X, Misra S, Xue G, Yang D (2012) Smart Grid—The new and improved power grid: a survey. IEEE Commun Surv Tutor 14(4):944–980. https://doi.org/10.1109/SURV.2011.101911.00087
Forman E, Peniwati K (1998) Aggregating individual judgments and priorities with the analytic hierarchy process. Eur J Oper Res 108(1):165–169. https://doi.org/10.1016/S0377-2217(97)00244-0
Gao J, Xiao Y, Liu J, Liang W, Chen CLP (2012) A survey of communication/networking in smart grids. Future Gener Comput Syst 28(2):391–404. https://doi.org/10.1016/j.future.2011.04.014
Gârdan IP, Micu A, Paștiu CA, Micu AE, Gârdan DA (2023) Consumers’ attitude towards renewable energy in the context of the energy crisis. Energies 16(2):676
Ghasempour A (2019) Internet of things in smart grid: architecture, applications, services, key technologies, and challenges. Inventions 4(1):22
Govindan K, Kaliyan M, Kannan D, Haq AN (2014) Barriers analysis for green supply chain management implementation in Indian industries using analytic hierarchy process. Int J Prod Econ 147:555–568. https://doi.org/10.1016/j.ijpe.2013.08.018
Haddad B, Liazid A, Ferreira P (2017) A multi-criteria approach to rank renewables for the Algerian electricity system. Renew Energy 107:462–472. https://doi.org/10.1016/j.renene.2017.01.035
Hasan Ghodusinejad M, Ghodrati A, Zahedi R, Yousefi H (2022) Multi-criteria modeling and assessment of PV system performance in different climate areas of Iran. Sustain Energy Technol Assess 53:102520. https://doi.org/10.1016/j.seta.2022.102520
Hasheminasab H, Streimikiene D, Pishahang M (2023) A novel energy poverty evaluation: study of the European Union countries. Energy 264:126157. https://doi.org/10.1016/j.energy.2022.126157
Hashmi M, Hänninen S, Mäki K (2011) Survey of smart grid concepts, architectures, and technological demonstrations worldwide. In: 2011 IEEE PES conference on innovative smart grid technologies Latin America (ISGT LA)
Hawk C, Kaushiva A (2014) Cybersecurity and the smarter grid. Electr J 27(8):84–95. https://doi.org/10.1016/j.tej.2014.08.008
Heile B (2010) Smart grids for green communications [industry perspectives]. IEEE Wirel Commun 17(3):4–6. https://doi.org/10.1109/MWC.2010.5490972
Ilbahar E, Cebi S, Kahraman C (2019) A state-of-the-art review on multi-attribute renewable energy decision making. Energy Strategy Rev 25:18–33. https://doi.org/10.1016/j.esr.2019.04.014
Jain P, Gyanchandani M, Khare N (2016) Big data privacy: a technological perspective and review. J Big Data 3(1):25. https://doi.org/10.1186/s40537-016-0059-y
Jaradat M, Jarrah M, Bousselham A, Jararweh Y, Al-Ayyoub M (2015) The internet of energy: smart sensor networks and big data management for smart grid. Proc Comp Sci 56:592–597. https://doi.org/10.1016/j.procs.2015.07.250
Kim M (2012) A survey on guaranteeing availability in smart grid communications. In: 2012 14th International Conference on Advanced Communication Technology (ICACT)
Krishankumar R, Pamucar D, Deveci M, Aggarwal M, Ravichandran KS (2022) Assessment of renewable energy sources for smart cities’ demand satisfaction using multi-hesitant fuzzy linguistic based choquet integral approach. Renew Energy 189:1428–1442. https://doi.org/10.1016/j.renene.2022.03.081
Kuzlu M, Pipattanasomporn M (2013) Assessment of communication technologies and network requirements for different smart grid applications. In: 2013 IEEE PES Innovative Smart Grid Technologies Conference (ISGT)
Kuzlu M, Pipattanasomporn M, Rahman S (2014) Communication network requirements for major smart grid applications in HAN, NAN and WAN. Comp Netw 67:74–88. https://doi.org/10.1016/j.comnet.2014.03.029
Lakshmi BM, Mathew M, Kinol AMJ, Vedagiri B, Perumal SB, Madhu P, Dhanalakshmi CS (2022) An integrated CRITIC-TOPSIS- and Entropy-TOPSIS-based informative weighting and ranking approach for evaluating green energy sources and its experimental analysis on pyrolysis. Environ Sci Pollut Res 29(40):61370–61382. https://doi.org/10.1007/s11356-022-20219-9
Léonard C, Chartres S, Chiappini F, Drouineau M, Nekrassov A, Hadjsaïd N (2016) Methodology, results and key success factors of Smart grid assessment in France. In: 2016 IEEE Power and Energy Society General Meeting (PESGM)
Lin CY, Chau KY, Moslehpour M, Linh HV, Duong KD, Ngo TQ (2022) Factors influencing the sustainable energy technologies adaptation in ASEAN countries. Sustain Energy Technol Assess 53:102668. https://doi.org/10.1016/j.seta.2022.102668
Lněnička M (2015) AHP model for the big data analytics platform selection. Acta Inform Prag 4(2):108–121
Luthra S, Kumar S, Kharb R, Ansari MF, Shimmi SL (2014) Adoption of smart grid technologies: an analysis of interactions among barriers. Renew Sustain Energy Rev 33:554–565. https://doi.org/10.1016/j.rser.2014.02.030
Ma S, Zhang H, Xing X (2018) Scalability for smart infrastructure system in smart grid: a survey. Wirel Pers Commun 99(1):161–184. https://doi.org/10.1007/s11277-017-5045-y
Manirambona E, Talai SM, Kimutai SK (2022) Sustainability evaluation of power generation technologies using multi-criteria decision making: the Kenyan case. Energy Rep 8:14901–14914. https://doi.org/10.1016/j.egyr.2022.11.055
Mashal I (2021) Evaluation and assessment of smart grid reliability using fuzzy multi-criteria decision-making. In: Rahmani-Andebili M (ed) Applications of fuzzy logic in planning and operation of smart grids. Springer International Publishing, Cham, pp 67–104. https://doi.org/10.1007/978-3-030-64627-1_3
Mashal I (2022) Smart grid reliability evaluation and assessment. Kybernetes. https://doi.org/10.1108/K-12-2020-0910
Mashal I, Alsaryrah O, Chung T-Y, Yang C-Z, Kuo W-H, Agrawal DP (2015) Choices for interaction with things on Internet and underlying issues. Ad Hoc Netw 28:68–90. https://doi.org/10.1016/j.adhoc.2014.12.006
Mashal I, Shuhaiber A, Daoud M (2020) Factors influencing the acceptance of smart homes in Jordan. Int J Electron Mark Retail 11(2):113–142
Mohammadzadeh AK, Ghafoori S, Mohammadian A, Mohammadkazemi R, Mahbanooei B, Ghasemi R (2018) A Fuzzy Analytic Network Process (FANP) approach for prioritizing internet of things challenges in Iran. Technol Soc 53:124–134. https://doi.org/10.1016/j.techsoc.2018.01.007
Mokhtar MR, Abdullah MP, Hassan MY, Hussin F (2015) Combination of AHP-PROMETHEE and TOPSIS for selecting the best Demand Side Management (DSM) options. In: 2015 IEEE Student Conference on Research and Development (SCOReD)
Moslehi K, Kumar R (2010) Smart Grid—a reliability perspective. In: 2010 Innovative Smart Grid Technologies (ISGT)
Nadizadeh Shorabeh S, Argany M, Rabiei J, Karimi Firozjaei H, Nematollahi O (2021) Potential assessment of multi-renewable energy farms establishment using spatial multi-criteria decision analysis: a case study and mapping in Iran. J Clean Prod 295:126318. https://doi.org/10.1016/j.jclepro.2021.126318
Narayanamoorthy S, Brainy JV, Shalwala RA, Alsenani TR, Ahmadian A, Kang D (2023) An enhanced fuzzy decision making approach for the assessment of sustainable energy storage systems. Sustain Energy Grids Netw 33:100962. https://doi.org/10.1016/j.segan.2022.100962
Naseem A, Ullah K, Akram M, Božanić D, Ćirović G (2022) Assessment of smart grid systems for electricity using power maclaurin symmetric mean operators based on T-spherical fuzzy information. Energies 15(21):7826
Nga DV, See OH, Xuen CY, Chee LL (2012) Visualization techniques in smart grid. Smart Grid Renew Energy 3(03):175
Ourahou M, Ayrir W, El Hassouni B, Haddi A (2020) Review on smart grid control and reliability in presence of renewable energies: Challenges and prospects. Math Comput Simul 167:19–31
Pokorni SJ (2019) Reliability and availability of the internet of things. Vojnoteh Glas 67(3):588–600
Ponce P, Polasko K, Molina A (2016) End user perceptions toward smart grid technology: acceptance, adoption, risks, and trust. Renew Sustain Energy Rev 60:587–598. https://doi.org/10.1016/j.rser.2016.01.101
Qarabsh NA, Sabry SS, Qarabash HA (2020) Smart grid in the context of industry 40: an overview of communications technologies and challenges. Indones J Electr Eng Comput Sci 18(2):656–665
Raimi KT, Carrico AR (2016) Understanding and beliefs about smart energy technology. Energy Res Soc Sci 12:68–74
Rehmani MH, Reisslein M, Rachedi A, Erol-Kantarci M, Radenkovic M (2018) Integrating renewable energy resources into the smart grid: Recent developments in information and communication technologies. IEEE Trans Indus Infor 14(7):2814–2825
Rodriguez-Calvo A, Cossent R, Frías P (2018) Scalability and replicability analysis of large-scale smart grid implementations: approaches and proposals in Europe. Renew Sustain Energy Rev 93:1–15. https://doi.org/10.1016/j.rser.2018.03.041
Saleem Y, Crespi N, Rehmani MH, Copeland R (2019) Internet of things-aided smart grid: technologies, architectures, applications, prototypes, and future research directions. IEEE Access 7:62962–63003. https://doi.org/10.1109/ACCESS.2019.2913984
Sanchez A, Rivera W (2017) Big data analysis and visualization for the smart grid. In: 2017 IEEE International congress on big data (big data congress)
Sanchez-Hidalgo M-A, Cano M-D (2018) A survey on visual data representation for smart grids control and monitoring. Sustain Energy Grids Netw 16:351–369. https://doi.org/10.1016/j.segan.2018.09.007
Shayani Mehr P, Hafezalkotob A, Fardi K, Seiti H, Movahedi Sobhani F, Hafezalkotob A (2022) A comprehensive framework for solar panel technology selection: A BWM- MULTIMOOSRAL approach. Energy Sci Eng 10(12):4595–4625. https://doi.org/10.1002/ese3.1292
Shuhaiber A, Mashal I (2019) Understanding users’ acceptance of smart homes. Technol Soc 58:101110. https://doi.org/10.1016/j.techsoc.2019.01.003
Siddiqa A, Karim A, Gani A (2017) Big data storage technologies: a survey. Front Inform Technol Electron Eng 18(8):1040–1070. https://doi.org/10.1631/FITEE.1500441
Sigrist L, May K, Morch A, Verboven P, Vingerhoets P, Rouco L (2016) On scalability and replicability of smart grid projects—a case study. Energies. https://doi.org/10.3390/en9030195
Siksnelyte-Butkiene I, Zavadskas EK, Streimikiene D (2020) Multi-criteria decision-making (MCDM) for the assessment of renewable energy technologies in a household: a review. Energies 13(5):1164
Soomro H, Shah SF, Sahito WS, Uqaili MA, Kumar L, Nixon JD, Harijan K (2022) Assessment of sustainable biomass energy technologies in Pakistan using the analytical hierarchy process. Sustainability 14(18):11388
Stefan M, Lopez JG, Andreasen MH, Olsen RL (2017) Visualization techniques for electrical grid smart metering data: a survey. In: 2017 IEEE third international conference on big data computing service and applications (bigdataservice)
Stević Ž, Pamučar D, Puška A, Chatterjee P (2020) Sustainable supplier selection in healthcare industries using a new MCDM method: Measurement of alternatives and ranking according to COmpromise solution (MARCOS). Comput Ind Eng 140:106231
Taylan O, Alamoudi R, Kabli M, AlJifri A, Ramzi F, Herrera-Viedma E (2020) Assessment of energy systems using extended fuzzy AHP, fuzzy VIKOR, and TOPSIS approaches to manage non-cooperative opinions. Sustainability 12(7):2745
Tightiz L, Yang H, Piran MJ (2020) A survey on enhanced smart micro-grid management system with modern wireless technology contribution. Energies 13(9):2258
Triantaphyllou E, Sánchez A (1997) A sensitivity analysis approach for some deterministic multi-criteria decision-making methods*. Decis Sci 28(1):151–194. https://doi.org/10.1111/j.1540-5915.1997.tb01306.x
Usman M, Jonas D, Frey G (2022) A methodology for multi-criteria assessment of renewable integrated energy supply options and alternative HVAC systems in a household. Energy Build 273:112397. https://doi.org/10.1016/j.enbuild.2022.112397
van Laarhoven PJ, Pedrycz W (1983) A fuzzy extension of Saaty’s priority theory. Fuzzy Sets Syst 11(1):229–241. https://doi.org/10.1016/S0165-0114(83)80082-7
Wang L (2017) Heterogeneous data and big data analytics. Autom Control Inform Sci 3(1):8–15
Wang Y, Chen Q, Hong T, Kang C (2019) Review of smart meter data analytics: applications, methodologies, and challenges. IEEE Transact Smart Grid 10(3):3125–3148. https://doi.org/10.1109/TSG.2018.2818167
Yan Y, Qian Y, Sharif H, Tipper D (2013) A survey on smart grid communication infrastructures: motivations, requirements and challenges. IEEE Commun Surv Tutor 15(1):5–20. https://doi.org/10.1109/SURV.2012.021312.00034
Yazdani M, Zavadskas EK, Ignatius J, Abad MD (2016) Sensitivity analysis in MADM methods: application of material selection. Eng Econ 27(4):382–391
Yousefi H, Ghodusinejad MH, Ghodrati A (2022) Multi-criteria future energy system planning and analysis for hot arid areas of Iran. Energies 15(24):9405
Zhao H, Guo S (2015) External benefit evaluation of renewable energy power in China for sustainability. Sustainability 7(5):4783–4805
Zhao H, Li N (2016) Performance evaluation for sustainability of strong smart grid by using stochastic AHP and fuzzy TOPSIS methods. Sustainability 8(2):129
Zhao H, Zhao H, Guo S (2018) Comprehensive performance evaluation of electricity grid corporations employing a novel MCDM model. Sustainability 10(7):2130
Acknowledgements
Not applicable.
Funding
No funding was used for this project.
Author information
Authors and Affiliations
Contributions
IM initiated the research project, developed the model, collected the data, wrote the main manuscript text. OK analyzed and interpreted the result, reviewed the findings. MH and MA completed the literature review, proofread/edited the final manuscript. All authors read and approved the final manuscript.
Corresponding author
Ethics declarations
Ethical approval and consent to participate
Not applicable.
Consent for publication
Not applicable.
Competing interests
The authors declare that they have no competing interests.
Additional information
Publisher's Note
Springer Nature remains neutral with regard to jurisdictional claims in published maps and institutional affiliations.
Appendix
Rights and permissions
Open Access This article is licensed under a Creative Commons Attribution 4.0 International License, which permits use, sharing, adaptation, distribution and reproduction in any medium or format, as long as you give appropriate credit to the original author(s) and the source, provide a link to the Creative Commons licence, and indicate if changes were made. The images or other third party material in this article are included in the article's Creative Commons licence, unless indicated otherwise in a credit line to the material. If material is not included in the article's Creative Commons licence and your intended use is not permitted by statutory regulation or exceeds the permitted use, you will need to obtain permission directly from the copyright holder. To view a copy of this licence, visit http://creativecommons.org/licenses/by/4.0/.
About this article
Cite this article
Mashal, I., Khashan, O.A., Hijjawi, M. et al. The determinants of reliable smart grid from experts’ perspective. Energy Inform 6, 10 (2023). https://doi.org/10.1186/s42162-023-00266-3
Received:
Accepted:
Published:
DOI: https://doi.org/10.1186/s42162-023-00266-3