Abstract
Background
In metamotivational monitoring, students try to identify the declined motivational component in order to regulate their motivation. There is scarcity of evidence on which motivational components are targeted by the medical students when they use each motivational regulation strategies. This study aims were identifying motivational components in motivational regulation process, developing a measurement tool and, testing the predictive relationship between the motivational components and motivational regulation strategies.
Methods
This exploratory sequential design mixed method study is part of a project has been started from 2018 with medical students at Tehran University of Medical Science. First, in a qualitative study conducting a semi-structured in-depth interview, the motivational components were explored. The interviews continued until saturation of data. Then, in a psychometric study the validity and reliability evidence of questionnaire obtained. In the quantitative study, applying the convenience sampling method, 508 students completed the questionnaires. Predictive relation between the motivational regulation strategies and motivational components was assessed utilising Structural Equation Modelling. Path coefficients, T-Value, and R2 index were reported by SmartPLS software.
Results
In the Exploratory Factor Analysis of Motivational Components Questionnaire (MCQ), 6 factors were discovered that explained 74% of the total variance. All paths in seven models of SEM showed a T-Value above 1.96 that means there is a significant correlation between all strategies and components. In examining the predictive relationships, each of the four components of self-efficacy, intrinsic value, self-relevant value and promotion value were specifically predicted by two motivational regulation strategies.
Conclusions
Evidence of validity and reliability of the MCQ indicates that this questionnaire can be used in medical education contexts. Health Profession Educators can improve the academic motivation of students by identifying one or more declined motivational component and teaching specific motivational regulation strategies. It is recommended to hold training courses on motivational regulation strategies for medical school faculty, study-skills advisors, and students.
Similar content being viewed by others
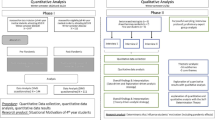
Background
Metamotivation is how people monitor and control their motivational states to achieve their goals [1]. Research findings indicate that some motivated students utilize specific strategies to enhance their motivational states [2], and consequently, improving their performance [3, 4]. A common issue is that learner role often gets skipped in controlling and regulating motivation in the context of medical education [5]. We know from the literature, outside the field of HPE, students are able to regulate their motivational components utilizing motivation regulation strategies [6]. The contextual aspects of medical education, such as basic science and clinical phase as well as highly specific educational environment, do not exist in other scientific disciplines. From this point of view, Norouzi et al. found that medical students use seven motivational regulation strategies, including regulation of value, regulation of situational interest, self-consequating, environmental structuring, promotional situational awareness, preventional situational awareness, and regulation of relatedness to monitor and control their academic motivation [7]. The identification of motivational regulation strategies is a significant step in understanding the metamotivational process, but another step, is identifying motivational components which are targeted by these motivational strategies [8].
A well-known and comprehensive model of metamotivation, developed by Miele & Scholer, define metamotivation as two reciprocal processes: A. Metamotivational monitoring; evaluating whether the person has selected the proper level (quantity) and type (quality) of motivation to perform his tasks. B. Metamotivational control; using the results of the monitoring phase and applying suitable strategies for adapting or changing the motivation [6, 9]. In the metamotivational monitoring phase, the motivational components play an essential role. When students feel like leaving a task, they monitor the motivational components to recognize which have declined and caused such a feeling. Also, when they think they have a wrong mindset or orientation for a task, they monitor their motivational components and alter them commensurate with the task at hand [8].
Considering three motivational theories (expectancy-value theory, self-determination theory, and regulatory focus theory), these researchers specified six motivational components include self-efficacy, intrinsic value, self-relevant value, external value, promotion value, and prevention value involved in the metamotivational model. They emphasized that to establish the nature of these motivational components, a survey should be designed in which not only various types of motivational regulation strategies are explored, but also the reasons for using strategies are determined and lead to clarity on the motivational components using factor analysis of responses [8]. On the other hand, it is expected that based on this model, every motivational component is predicted directly using a limited number of motivational regulation strategies.
Therefore, it seems that for the following reasons there was a need to identify the motivational components and design its measurement tool:
-
1-
Raising self-awareness of the status of motivational components as a trigger to use strategies,
-
2-
Identifying the influenced motivational components to choose the right strategy and
-
3-
Expanding the studies in the field of metamotivation.
Norouzi et al. explained the motivational regulation strategies and designed Metamotivational Strategies in Medical Students Questionnaire (MSMQ) to use in the medical education context [10]. However, the question remains, which underlying components of motivation is targeted by the medical students when taking advantage of these strategies? To answer this question first, we identified the motivational components in motivational regulation process in medical students and developed a measurement tool. Then, we tested the predictive relationship between the motivational components and motivational regulation strategies.
Methods
The aim, design and setting of the study
This exploratory sequential design mixed method study [11] is part of a big project has been started from 2018 with medical students at Tehran University of Medical Science. In this manuscript, we present the results of two phases (Table 1). A. Identifying motivational components in motivational regulation process and developing its measurement tool, B. Investigating the predictive relationship between the motivational components and motivational regulation strategies.
Study steps
Phase A
Step 1. Collecting the reasons for using motivational strategies
First, in a qualitative study, the motivational regulation strategies used by the medical students were explored. Participants were selected via purposive sampling. This study was conducted through a semi-structured, in-depth interview in which participants were asked to think about their real educational experiences where they felt their motivation states were challenged in the educational environment. Next, they explained the strategies used to monitor, sustain or control their learning motivation. After a reference to a strategy by the student, the researcher further probed for the reason behind the strategy (i.e., why do you use this strategy?). At the end of each interview, the reasons for using motivational regulation strategies were gathered. The interviews continued until saturation of data.
To ensure credibility, the principal investigator was continuously involved in the research topic for 8 consecutive months and used the methods of bracketing and member check. One expert out of the research team reviewed the analyses to ensure confirmability. To ensure data dependability, 10% of the data were encoded by two independent individuals. Furthermore, to achieve transferability, maximum variation was considered in sampling in terms of gender and phase of education. Further details of this part of study has been published in another article in Journal of Education and Health Promotion [12].
Step 2. Designing the Motivational Components Questionnaire (MCQ) items
A panel of five experts in medical education and psychology was formed. In this session, the reasons collected from the interviews were categorized, and questionnaire items were compiled according to the common concept of each category of reasons.
Step 3. Investigating of the evidence of Content Validity in MCQ
Fifteen experts in the field of medical education and psychology were enlisted to score the Content Validity Index (CVI) and Content Validity Ratio (CVR) indices. They were asked to rate each item's relevance, clarity, and simplicity on a four-point Likert scale to allow calculation of the CVI index [very relevant (4), relevant (3), somehow relevant (2), irrelevant (1)]. The CVI was then calculated using the Waltz and Bausell formula, for which the index is acceptable when the average score obtained from all items is higher than 0.79 [13].
The CVR was calculated using the Lawshe formula [14]. Experts were asked to categorize each question according to a 3-point Likert scale of "item is necessary," “item is useful but not necessary,” and “item is not necessary.” Then, CVR was calculated using the following formula. Taking into account the number of experts (N = 15) and the values in the Lawshe table, the items with CVR < 0.49 were eliminated from the questionnaire (N: total number of experts; Ne: number of experts who selected the item “necessary”)
Step 4. Examining the evidence of response process validity in MCQ
In this step, six medical students were interviewed. The aim of this step was to compare the students’ interpretation of each item with the intended purpose of the designers and assuring congruence through modification as needed. Students' perceptions about each item were investigated with “thinking aloud” and “concurrent verbal probing” methods.
Step 5. Examining the evidence of structural validity and reliability in MCQ
The Exploratory Factor Analysis (EFA) was used to evaluate the instrument and gather the evidence of structural validity. The convenience sampling method was also applied. The instrument was designed using the ePoll, an online test maker application, as an online questionnaire, and its link was sent to the medical students through social networks. Considering the number of items and the assumptions of the EFA, the minimum sample size was determined to be 120 (5 subjects per item). 224 students completed the questionnaire. The data were analyzed by the IBM SPSS Statistics 26 for Windows (IBM Corp. Armonk, NY). The Kaiser–Meyer–Olkin (KMO) index (> 0.7) was used to evaluate the adequacy of the sample size, and Bartlett's test of sphericity was used to determine the factorability of the data. Also, the Principal Component method was used with Varimax rotation to extract the components, and the items with a factor load greater than 0.4 were preserved. To identify the tool’s reliability evidence, Cronbach's alpha coefficient and Intraclass Correlation Coefficient (ICC) analysis were used. After EFA and determination of the tool’s structure, the Cronbach's alpha of subscales and the overall instrument were calculated. The final questionnaire was given twice to 23 students with a two-week interval to calculate the ICC. Common cut-off points for ICC assessment were used; > 0.90 (excellent), 0.75–0.90 (good), 0.60–0.75 (moderate), and < 0.60 (poor) [15].
Phase B
Assessment of predictive relation between the motivational regulation strategies and motivational components
In this step MCQ and Metamotivational Strategies in Medical Students Questionnaires (MSMQ) [10] were used. The important question was to identify which motivational component is targeted when using any of the seven strategies?
The students were asked to answer the questions related to each motivational strategy i.e., determine how much they use that strategy and then, determine their reasons (by answering MCQ questions) when using that specific strategy. Therefore, seven online questionnaires were designed separately and sent to seven different groups of medical students (One questionnaire for each strategy). In this stage, we formed seven structural models (see Additional file 3: Appendix 3). Based on the 10-times rule [16] in the Partial Least Squares (PLS) method, the minimum sample size was estimated 60 per model.
The SmartPLS software (SmartPLS 2.0.M3. Hamburg) analyzed collected data. The validity was assessed using convergent validity, divergent validity, composite reliability, and Cronbach's alpha coefficient. In the PLS, the convergent validity includes the Average Variance Extracted (AVE) of subscale questions. Accordingly, convergent validity is confirmed when the AVE is more than 0.5. Divergent validity was tested via the cross-loading method. According to this index, if the item related to a subscale has a higher factor load on another subscale, it will be removed from the model. Composite reliability and Cronbach's alpha coefficient were also confirmed with a reliability greater than 0.7.
The predictive relationship between the variables was calculated through SEM. Path coefficient, T-Value, and R2 index were used to investigate the predictive relations between latent internal and external variables. When the T-Value of a path is greater than 1.96, it is confirmed at a significance level of < 0.05. The statistic R2 indicates the level of changes in the endogenous variable that the exogenous variable can predict. Commonly, for the dependent variable in the structural model, the values of 0.19, 0.33, and 0.67 have been described as weak, medium, and significant, respectively. However, if the latent endogenous variable is affected by a small number of the exogenous variables (one or two), the medium values of R2 could also be accepted [17, 18]. Considering that, in the present study, all latent endogenous variables were affected by only one exogenous variable, the values of R2 higher than 0.33 were deemed acceptable.
Results
Phase A: identifying motivational components and designing its measurement tool
After interviewing the medical students, the data were analyzed, and 207 phrases were gathered as the specific reasons for using the motivational regulation strategies. In the expert panel, the conceptually related reasons were categorized into 24 categories. Then, an item was formulated for each group of reasons, which conceptually covered all the reasons in that category. The responses to the items were also scored on a 5-point Likert scale (never, rarely, sometimes, usually, and always). In the analysis of the CVI index, the instrument gained a score above 0.79 for transparency, relevancy, and simplicity. The CVR index also gained a score above 0.49 for all items, and none of the items was eliminated from the study (see Additional file 1: Appendix 1).
Students’ interpretations confirmed the evidence of response process validity. In the EFA, the KMO test obtained a score of 0.89, and Bartlett's test of sphericity was significant. This finding indicates that the sample size was adequate and the data are factorable. The total variance explained table shows that six factors with an eigenvalue greater than one could estimate 74% of the variance. The result indicated that, the items of the first factor refer to reasons such as making the academic achievement enjoyable and attractive. This factor was entitled “intrinsic value.” Also, conceptually, the items of factor 2 referred to the student's efficacy in confronting academic challenges. This factor was entitled “self-efficacy.” In the items of factor 3, the students tried to provide reasons for using the motivational regulation strategies, where they referred to the prevention of problematic situations. So, this factor was entitled “prevention value.” On the other hand, in the items of factor 4, the students tried to provide reasons for using the motivational regulation strategies, where they referred to the academic achievements. This factor was, thus, called the “promotion value.” In addition, the reasons referred to in the items of factor 5 were conceptually related to achieving academic values and benefits. This factor was entitled “self-relevant value.” Finally, the items of factor 6, which included concepts such as awards and rewards, were entitled “external value.” The lowest factor load was 0.61, and the highest was 0.89. The alpha coefficient of the overall instrument was 0.92. The alpha coefficient of the subscales is provided in Table 2. Calculation of the ICC index indicated that all subscales are at the “excellent” (higher than 0.90) and “good” (0.75–0.90) levels.
Phase B: predictive relation between the motivational regulation strategies and motivational components
Using convenience sampling method, 508 medical students completed the questionnaires. The descriptive statistics of those who completed the seven questionnaires can be observed in Table 3.
The results of model validity assessments are provided in Additional file 2: Appendix 2. Divergent validity assessment showed that only in the model of “environmental structuring” “motivational components”, item 4 of the subscale of “prevention value” had a factor load of 0.45, and 0.55 on Self-efficacy subscale. Therefore, this item was eliminated from the model, and the model was formulated again. In other models, all factor loads were confirmed.
The AVE index of all subscales in the seven models was above 0.5. Composite reliability and Cronbach's alpha coefficient of all subscales were also above 0.7. These results indicated that both instruments have best evidences of reliability and validity.
The path coefficients and the standardized loading factors for all structural models are presented in Additional file 3: Appendix 3. Investigation of the significance of path coefficients and loading factors indicated that the T-Value of all paths in all seven models was above 1.96. This showed a positive and significant correlation between all motivational regulation strategies and all motivational components. Table 4 shows the path coefficients, T-Value and R2 values in all models.
The regulation of value predicted 41%, 52%, and 53% of the variations of self-efficacy, intrinsic value, and self-relevant value, respectively. The regulation of situational interest also predicted 51% and 56% of the intrinsic value and promotion value, respectively. Promotional situational awareness predicted 33% and 43% of self-efficacy and promotion value. The environmental structuring was a predictor of the self-relevant value with a value of 33%.
The two components of external value and prevention value could not be predicted well by the motivational regulation strategies. The three strategies of regulation of relatedness, preventional situational awareness, and self-consequating could not predict the motivational components at acceptable level.
Discussion
The present study identifies the motivational components targeted by motivation regulation strategies, creates a tool to measure them, and determines a predictive relation between the motivational components and motivational regulation strategies. The high percentage of the total variance explained in the EFA as well as the lack of cross factor loading between the items indicates that the factors have been appropriately explained and are major and distinguished components in this process. Also, there is proper evidence of the reliability and validity of the results obtained using this instrument. Motivational components identified in this study are entirely adapted to the taxonomy of motivational components in the Miele and Scholer's metamotivational model [8].
The results of analyzing the validity of models in Phase B indicated that both instruments provide best evidence of structural validity and reliability. Investigation of convergent validity in all models showed that the motivational components and motivational regulation strategies had been appropriately explained. On the other hand, the divergent validity of the items indicated that all items had a proper factor load in their subscale. In addition, a positive and significant relationship between all motivational regulation strategies and motivational components in the seven models was another essential result that indicated the reason for the comprehensive explanation of strategies and components.
The findings of this study regarding the intrinsic value mainly were in line with the expectations of models of Miele and Scholer. These researchers speculated that this motivational component is specifically affected by the regulation of value strategy [8]. We should notice that the metamotivational approach is built on the assumption that students have awareness to why they engage in certain strategies. it is relevant to look at people’s beliefs, and it is likely true that students often do have good insight into their motivation. However, like metacognitive process they can occur implicitly or automatically [9].
This study also showed that the regulation of value and regulation of situational interest both predict the intrinsic value at acceptable level. The intrinsic value originates from the self-determination theory [19]. The highest quality of motivation is intrinsic and autonomous types of motivation which is related to deep learning and better performance of learners [19, 20].
Self-efficacy is the student’s belief in his/her potentials for the successful performance of tasks [21]. It is one of the most potent predictors of academic effort, and perseverance [22]. In his study, Wolters concluded that the students could affect the self-efficacy through their motivational regulation strategies [2]. Studies in medical education also indicates that self-beliefs and self-efficacy facilitate the learning and development of medical students [22]. Pelaccia mentioned the perceived self-efficacy as one of the main components of effort, regulation, perseverance, and management of academic performance in medical students [23]. According to the Miele and Scholer's model, it is expected that efficacy self-talk and proximal goal setting could predict the self-efficacy [8]. This study demonstrates that these two strategies of regulation of value and promotional situational awareness could correctly predict the self-efficacy. This is even though the results of Norouzi et al. and Wang and Wolters indicate that the efficacy management strategy could not serve as a comprehensive strategy for metamotivational monitoring [2, 10, 24].
The theoretical basis of the two components of promotion value and prevention value is regulatory focus theory. Predominantly promotion-focused individuals state their goals as ideals and use eager strategies to achieve their goals. On the other hand, predominantly prevention-focused individuals take their academic goals as tasks and responsibilities and prefer vigilant strategies to achieve their academic goals [25]. It seems the medical students who think of their promotion and development, or those who are sensitive to inability in their academic improvement, target their promotion value in metamotivational monitoring. On the other hand, the students think about escaping from illiteracy, not getting the lowest marks, and not failing exams, seek to affect their prevention value. The predictions of the promotion value were relatively in line with the Miele and Scholer's model [8]. The present study results indicate that the two strategies of regulation of situational interest and promotional situational awareness can strongly predict this component. Even though the Miele and Scholer's model use mastery self-talk strategy as the likely predictor of prevention value [8], the results of this study indicated that no one of the motivational regulation strategies could predict this component at acceptable level (In Norouzi et al., promotional/ preventional situational awareness, as more comprehensive strategies, replaced regulation of mastery/performance goals [10]).
The self-relevant value is equivalent to identified and integrated regulation in the self-determination theory [8]. Using some strategies, the medical students try to remind themselves of the importance and suitability of medicine and educational factors to obtain a professional identity and become aware of the values of their field of study. In Miele and Scholer's model, the regulation of value has been taken as the predictor of self-relevant value. The results of this study have affirmed this idea. The two strategies of regulation of value and environmental structuring could predict 53% and 32% variations of the self-relevant value, respectively. The external value is also equivalent to external and introjected regulation in self-determination theory [8]. The individual indicates the reasons for doing things such as receiving rewards, escaping punishment, avoiding shame, etc. [19]. In the present study, the external value was not adequately predicted by any motivational regulation strategies. However, according to Miele and Scholer's model, it was expected that there would be a predictive relation between this component and self-consequating.
Despite their significant relationship with all motivational components, the three strategies of regulation of relatedness, preventional situational awareness, and self-consequating could not predict a substantial share of one of the motivational components alone. The finding enhances the likelihood that these three strategies could be used simultaneously with other strategies to improve effectiveness. That’s because, according to Miele and Scholer, there are strategies that increase the likelihood of inclusive engagement with tasks [9]. Using the regulation of relatedness and other motivational regulation strategies, medical students are likely to meet two or more academic goals at a time, thereby managing their motivation. For example, when a medical student tries to enter clinical education in hospital departments with a group of friends, he tries to both increase his relations with friends as well as his interest in attending that department by creating a fun atmosphere in the educational environment, so that he could strengthen his intrinsic motivation.
Implication of this study
In this study, a tool with acceptable validity and reliability evidence was designed that researchers can use in their future studies in the field of metamotivation. This tool can be used by educationists in order to know the motivational components of students and based on the obtained information, targeted educational interventions can be designed. Also, this tool can be used for students' self-awareness of motivational status. Using the information obtained from the second phase of the study, psychologists and educational counselors can suggest motivational strategies which fit to students motivation states.
Limitations and strengths
Real experiences of medical students were obtained through deep interviews, it can therefore be claimed that none of the identified components include an abstract aspect, and none have been inferred from the personal opinions and perceptions of the students. On the other hand, we tried to evaluate and prove the nature of the interview results through EFA.
The focus of this study was on medical students. Therefore, there is doubt in generalizing the findings of this study to other medical and non-medical students. Considering that we were looking for the ultimate reason for using strategies in phase 1; It was not easy for students to express these reasons that may have affected the identified components. It should also be noted that the interviews were held virtually due to the covid 19 pandemic. It is possible that conducting face-to-face interviews will make more and better-quality data available to the researcher.
Other limitation of our study is its relatively small sample size for the estimation of each structural model in Phase B. Although the minimum sample size was calculated based on the assumptions of SEM, it is evident that the higher the number of samples, the better the generalizability of the results.
Conclusion and recommendations
The identification of motivational components is a very fundamental idea in the Miele and Scholer's model. This idea is a very significant effort for making metamotivational studies more purposeful. The results of this study indicate that the six motivational components of the Miele and Scholer's model are the key and essential components in this process. Although the expectations of the metamotivational model as to the predictive relation between the strategies and components were not fully met, in this study, the fundamental assumption of the metamotivational model, i.e., the direct relationship between one or two motivational regulation strategies and the motivational components was proven.
Future studies are recommended for the identification of the phenomenological experiences of the six mentioned components. The discovery of the structural relationship between these components and the medical students' desire and intention of the medical students to perform their academic tasks is a need. It is expected that future studies investigate the effect of simultaneous use of several motivational regulation strategies on the motivational components. If the goal is to use this scale in other research, it seems that it might be useful to have an additional study that links strategy use to student outcomes or other measures. It is also suggested to investigate metamotivational processes in the field of faculty members in future studies.
Availability of data and materials
The datasets used and/or analysed during the current study are available from the corresponding author on reasonable request.
References
Scholer AA, Miele DB, Murayama K, Fujita K. New directions in self-regulation: the role of metamotivational beliefs. Curr Dir Psychol Sci. 2018;27(6):437–42.
Wolters CA. Regulation of motivation: evaluating an underemphasized aspect of self-regulated learning. Educ Psychol. 2003;38(4):189–205. Available from: http://www.tandfonline.com/doi/abs/10.1207/S15326985EP3804_1.
Kryshko O, Fleischer J, Waldeyer J, Wirth J, Leutner D. Do motivational regulation strategies contribute to university students’ academic success? Learn Individ Differ. 2020;82:101912. Available from: https://doi.org/10.1016/j.lindif.2020.101912.
Schwinger M, Stiensmeier-Pelster J. Effects of motivational regulation on effort and achievement: a mediation model. Int J Educ Res. 2012;56:35–47. Available from: https://linkinghub.elsevier.com/retrieve/pii/S0883035512000651.
Lyons KM, Cain JJ, Haines ST, Gasevic D, Brock TP. The clinical educator’s guide to fostering learner motivation: AMEE Guide No. 137. Med Teach. 2021;43(5):492–500. https://doi.org/10.1080/0142159X.2020.1837764.
Miele DB, Scholer AA, Fujita K. Metamotivation: Emerging research on the regulation of motivational states. In: Elliot AJ, editor. Advances in Motivation Science. Elsevier; 2020. p. 1–42. Available from: http://www.sciencedirect.com/science/article/pii/S221509191930015X
Norouzi A, Parmelee D, Shariati M, Norouzi S, Nedjat S, Alizadeh M. The development and validation of metamotivational strategies in medical students questionnaire. Med Teach. 2021;43(10):1186–95. Available from: https://www.tandfonline.com/doi/full/10.1080/0142159X.2021.1922656.
Miele DB, Scholer AA. The role of metamotivational monitoring in motivation regulation. Educ Psychol. 2018;53(1):1–21. Available from: https://www.tandfonline.com/doi/full/10.1080/00461520.2017.1371601.
Miele DB, Scholer AA. Self-regulation of motivation. In K. R. Wentzel & D. B. Miele (Eds.), Handbook of Motivation at School. New York: Taylor & Francis. 2016. pp. 363–384.
Norouzi A, Parmelee D, Shariati M, Norouzi S, Nedjat S, Alizadeh M. The development and validation of metamotivational strategies in medical students questionnaire. Med Teach. 2021;43(10):1186–95.
Creswell JW. Steps in conducting a scholarly mixed methods study. 2013.
Norouzi A, Alizadeh M, Parmelee D, Nedjat S, Norouzi S, Shariati M. Metamotivation in medical students: explaining motivation regulation strategies in medical students. J Educ Health Promot. 2022;11(1):157. Available from: http://www.https//jehp.net//text.asp?2022/11/1/157/347159.
Waltz CF Bausell RB. Nursing Research : Design Statistics and Computer Analysis. Philadelphia: F.A. Davis; 1981.
Lawshe CH. A quantitative approach to content validity. Pers Psychol. 1975;28(4):563–75.
Koo TK, Li MY. A Guideline of Selecting and Reporting Intraclass Correlation Coefficients for Reliability Research. J Chiropr Med. 2016;15(2):155–63. Available from: https://pubmed.ncbi.nlm.nih.gov/27330520. 2016/03/31.
Kock N, Hadaya P. Minimum sample size estimation in PLS-SEM: the inverse square root and gamma-exponential methods. Inf Syst J. 2018;28(1):227–61.
Chin WMG. The partial least squares approach to structural formula modeling. Adv Hosp Leis. 1998;8(2):5. Available from: https://books.google.com/books?hl=en&lr=&id=EDZ5AgAAQBAJ&oi=fnd&pg=PA295&dq=The+partial+least+squares+approach+to+structural+equation+modeling&ots=49uH6qt2lk&sig=Fwg2GGFWp3LUMMjxMu9h4jbOXnA.
Henseler J, Ringle CM, Sinkovics RR. The use of partial least squares path modeling in international marketing. In Advances in International Marketing|Adv Int Mark. 2009(20):277–319. (Advances in International Marketing). Emerald Publishing Limited. https://doi.org/10.1108/S1474-7979(2009)0000020014.
Deci EL, Ryan RM. Self-determination theory. 2012.
Levesque C, Zuehlke AN, Stanek LR, Ryan RM. Autonomy and competence in German and American university students: a comparative study based on self-determination theory. J Educ Psychol. 2004;96(1):68–84.
Bandura A, Schunk DH. Cultivating competence, self-efficacy, and intrinsic interest through proximal self-motivation. J Pers Soc Psychol. 1981;41(3):586–98.
Klassen RM, Klassen JRL. Self-efficacy beliefs of medical students: a critical review. Perspect Med Educ. 2018;7:76–82.
Pelaccia T, Viau R. Motivation in medical education*. Med Teach. 2017;39(2):136–40.
Wang L. Understanding EFL Learners’ Learning Motivational Regulation Strategies: an exploratory evidence from students in a Chinese-foreign cooperative project. Rev Cercet si Interv Soc. 2019;66:41–58. Available from: https://www.rcis.ro/images/documente/rcis66_03.pdf.
Jansen E. The Role of Metamotivation in Managing the Motivation of Others: A Leadership Perspective. UWSpace. 2019. http://hdl.handle.net/10012/14948.
Acknowledgements
The authors wish to thank all students who participated in the study.
Funding
Tehran University of Medical Sciences, school of medicine, deputy of research is the founder of the study.
Author information
Authors and Affiliations
Contributions
All authors (AN, DP, MS, SN and MA) contributed to data collection, analysis and interpretation of data for writing the manuscript. All authors read and approved the final manuscript.
Corresponding author
Ethics declarations
Ethics approval and consent to participate
Methods were carried out in accordance with relevant guidelines and regulations. We confirm that informed consent was obtained from all participants.
The study was approved by IR.TUMS.MEDICINE.REC.1398.818 from Tehran University of Medical Sciences, school of medicine, research committee.
Consent for publication
Not applicable.
Competing interests
The authors declare no competing interests.
Additional information
Publisher’s Note
Springer Nature remains neutral with regard to jurisdictional claims in published maps and institutional affiliations.
Supplementary Information
Additional file 1: Appendix 1.
CVI and CVR index values.
Additional file 2: Appendix 2.
Investigation of the validity evidence and reliability in seven models.
Additional file 3: Appendix 3.
Structural model of predictive relationship between motivational regulation strategies and motivational components (The numbers inside ellipses are the R2 values).
Rights and permissions
Open Access This article is licensed under a Creative Commons Attribution 4.0 International License, which permits use, sharing, adaptation, distribution and reproduction in any medium or format, as long as you give appropriate credit to the original author(s) and the source, provide a link to the Creative Commons licence, and indicate if changes were made. The images or other third party material in this article are included in the article's Creative Commons licence, unless indicated otherwise in a credit line to the material. If material is not included in the article's Creative Commons licence and your intended use is not permitted by statutory regulation or exceeds the permitted use, you will need to obtain permission directly from the copyright holder. To view a copy of this licence, visit http://creativecommons.org/licenses/by/4.0/. The Creative Commons Public Domain Dedication waiver (http://creativecommons.org/publicdomain/zero/1.0/) applies to the data made available in this article, unless otherwise stated in a credit line to the data.
About this article
Cite this article
Norouzi, A., Parmelee, D., Shariati, M. et al. The role of motivational components in metamotivational monitoring in medical students: a mixed method study. BMC Med Educ 23, 108 (2023). https://doi.org/10.1186/s12909-023-04081-y
Received:
Accepted:
Published:
DOI: https://doi.org/10.1186/s12909-023-04081-y