Abstract
Background
Studies attempted to estimate MASLD-related advanced fibrosis (AF) and cirrhosis (MC) prevalence utilized tests with low positive predictive value (PPV) which overestimates prevalence. AGILE3 + and 4 scores were developed to increase the PPV of both; respectively. In this study, we used these scores to assess the prevalence of AF and MC.
Methods
Participants aged ≥ 18 years with VCTE exam in the NHANES 2017–2018 cycle were included. We excluded pregnant women, patients with excessive alcohol intake, hepatitis B/C, and ALT or AST > 500 IU/L. MASLD was defined with CAP score > 248 dB/m. MASLD subjects with AGILE 3 + score of ≥ 0.68 and AGILE 4 score of ≥ 0.57 were considered to have advanced fibrosis and cirrhosis; respectively. AGILE 3 + of 0.45–0.67 and AGILE 4 of 0.25–0.57 were grey zone, whereas AGILE 3 + < 0.45 and AGILE 4 < 0.25 were considered a rule-out.
Results
1244 subjects were included in the final analysis. The Median age was 53 (51.4–54.6) years, 55.6% were male, median BMI was 33.8 kg/m2 and 41.1% had T2DM. Based on AGILE 3+, 80.3% of the MASLD population were at low risk for AF and 11.5% were in grey zone. The prevalence of AF due to MASLD was 8.1% corresponding to 4.5 million Americans. Based on AGILE 4 score, 96.5% of the MASLD population were at low risk for cirrhosis and 2.4% were in the grey zone. The prevalence of MASLD-cirrhosis was 1.1% corresponding to 610,000 Americans.
Conclusion
Our results suggest that approximately 4.5 million people in the U.S. have AF and 0.6 million have cirrhosis due to MASLD.
Similar content being viewed by others
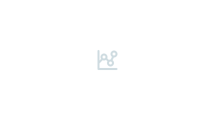
Introduction
Metabolic Dysfunction-Associated Steatotic Liver Disease (MASLD), previously known as Non-alcoholic Fatty Liver Disease (NAFLD), is a histological disease spectrum that ranges from fatty liver infiltration to steatohepatitis (previously known as Non-alcoholic Steatohepatitis, MASLD), advanced fibrosis and – eventually- cirrhosis. MASLD is a leading cause of end-stage liver disease worldwide and is predicted to emerge as the sole leading cause in the next few decades, affecting both adults and children [1, 2]. Owing to the obesity pandemic, the global prevalence of MASLD has been on the rise and is currently estimated to be ~ 25% (compared to ~ 15% on 2005). In a recent cross-sectional analysis in the United States, the biopsy-proven prevalence of MASLD in the U.S. adults has been ~ 21.9%, representing 51.6 million potentially affected individuals [3]. Liver biopsy has been the gold standard to determine the prevalence of MASLD fibrosis and cirrhosis; however, several noninvasive tests and scoring systems have been developed to estimate the prevalence of the two entities [5]. These scores include AST to platelet ratio index (APRI) which was originally developed for use among HCV patients and was later validated to predict MASLD-related advanced fibrosis with a sensitivity and specificity of 75% and 86%, respectively [6, 7]. Other scoring systems like the fibrosis-4 index (FIB-4) and the NAFLD fibrosis score (NFS) were also validated in patients with good accuracy [7, 8]. However, owing to relying on routine blood tests alone (Aspartate aminotransferase {AST}, alanine aminotransferase {ALT}, platelets, etc.), the accuracy of these tests can be easily affected by several factors that are not related to liver disease. More importantly, the positive predictive value (PPV) of these tests is relatively low, leading to potentially overestimating the true disease prevalence. For these reasons, AGILE 3 + and AGILE 4 scores have been recently developed by combining routine clinical variables, lab chemistry values and vibration-controlled elastography (VCTE) parameters to specifically increase the PPV of predicting MASLD-related advanced fibrosis and cirrhosis; respectively. Our aim in this study is to estimate the prevalence of MASLD-related advanced fibrosis and cirrhosis using these new scores.
Methodology
Database
Established by Center for Disease Control and Prevention (CDC), The National Health and Nutrition Examination Survey (NHANES) database serves as a major national database designed to understand the health and nutritional needs of children and adults in the United States since 1960s. NHANES databases program annually examines a nationally representative sample of ~ 5,000 individuals located in counties across the United States. The survey interview includes demographic, socioeconomic, dietary, and health-related questions. The examination further includes medical, physiological and laboratory measurements performed by highly trained medical personnel at a central laboratory, in addition to interview questionnaires and standardized physical examination. Data from the survey is used in epidemiological and health-related studies which help to design further health programs and services. The survey was approved by the Institutional Review Board at the Center for Disease Control and Prevention, and informed consent was obtained from all participants. Data from NHANES 2017–2018 is the most recent survey cycle that provided transient elastography information as determined by FibroScan® and was utilized for this analysis.
Definitions and inclusion criteria
NHANES 2017–2018 is the most recent survey cycle that provided transient elastography data. Our study population included participants aged ≥ 18 years old who had a complete transient elastography exam. NHANES used FibroScan® model 502 V2 Touch equipped with medium and extra-large probes. We excluded pregnant women, missing alcohol data, hepatitis B or C, and ALT or AST > 500 IU/L. Among these patients, we stratified based on excessive alcohol consumption defined as > 2 drinks/day for males and > 1 drink/day for females and obesity defined as a Body Mass Index (BMI) of 30 kg/m2 or more. The inclusion criteria and participants stratification algorithm are shown in Fig. 1. We further stratified based on steatosis (CAP ≥ 248 dB/m) to identify MASLD. Our final sample was 1244 participants.
Statistical analysis
Characteristics of study participants
In addition to the demographic information, data collected included: body mass index (BMI), comorbidities (type 2 diabetes {T2DM}, obesity), and lab values (Alanine Aminotransferase {ALT}, Aspartate Aminotransferase {AST}, total bilirubin, albumen, platelet count, and Hemoglobin A1C {HBA1C}) and VCTE values (liver stiffness measurement {LSM}, CAP) were collected. Our cohort consisted of 1244 subjects with evidence of MASLD. The Median age was 52.5 (50.7–54.2) years, 54.9 (48.6–61.2) were male, and median BMI was 32.7 (31.8–33.6) kg/m2. 64.2% of patients were of White race (58.9–69.2), 17.1% Hispanics (12.9–22.3) and 8.8% Black (5.9–13). 36.2% of patients tended to have T2DM (31.8–40.8) whereas 59.4% were obese (53.8–64.8). The characteristics of the study participants is shown in Table 1.
Outcome measures
We calculated AGILE 3 + score based on the equation:
We analyzed the prevalence of AGILE 3 + based on the cutoff criteria < 0.45 as rule out, 0.45–0.68 as indeterminate, and ≥ 0.68 as rule in to identify advanced fibrosis in MASLD patients.
Furthermore, using the same population, we calculated AGILE 4 score based on the equation:
We analyzed the prevalence of AGILE 4 based on the cutoff criteria < 0.25 as rule out, 0.25–0.57 as indeterminate, and ≥ 0.57 as rule in to identify cirrhosis in MASLD patients. Appropriate survey weights were applied for all analyses which were performed using Stata version 17 (StataCorp. 2021. Stata Statistical Software: Release 17. College Station, TX: StataCorp LLC.).
Results
The prevalence of Advanced Fibrosis utilizing AGILE 3 + score
Based on AGILE 3+, 80.3% (95% CI: 77.1–83.2) of the MASLD population were at low risk for AF and 11.5% (9.2–14.5) were in the grey zone. The overall prevalence of AF due to MASLD was 8.1% (6.2–10.6) corresponding to 4.5 million Americans. Compared to those without AF, the rule-in population tended to be older (64.4 vs. 49.6 years), have higher BMI (37.9 vs. 32), significantly higher T2DM (86% vs. 24.3%) and obesity (80.1% vs. 56.4%) prevalence. There was no significant gender pattern preference, and patients of White race seemed to be most likely to be affected. Those with AF tended to have slightly higher AST (26.04 vs. 21.13 IU/L) and hemoglobin A1C (6.78 vs. 5.92%) but remarkably lower platelet count (201.69 vs. 252.21 per 10^3 cells/uL). Moreover, those with AF had significantly higher LSM measurement (16.65 vs. 5.25 kPa), CAP (331.38 vs. 304.61 dB) and FAST score (0.35 vs. 0.12) compared to those without. The characteristics of AGILE 3 + stratified patients are shown in Table 2.
The prevalence of MASLD cirrhosis utilizing AGILE 4 score
Based on AGILE 4 score, 96.5% (95% CI: 94.8–97.6) of the MASLD population were at low risk for MASLD cirrhosis and 2.4% (95% CI: 1.4–4.1) were in the grey zone. The overall prevalence of MASLD cirrhosis was 1.1% (95% CI: 0.5–2.3) corresponding to 610,000 Americans. Compared to those without MASLD-cirrhosis, the rule-in population tended to be older (54.5 vs. 52.3 years), have higher BMI (44.7 vs. 32.4), significantly higher T2DM (85.1% vs. 34.9%) and obesity (95.6% vs. 58.3%) prevalence. Men seemed to be more likely to have cirrhosis (56.3% vs. 43.7%) and the patients of White race seemed to be most likely to be affected. Those with cirrhosis tended to have slightly higher AST (27.89 vs. 21.5 IU/L). Cirrhosis patients also had slightly higher hemoglobin A1C (6.54 vs. 6.09%) but remarkably lower platelet count (175.8 vs. 246.71 per 10^3 cells/uL). Total bilirubin (0.6 vs. 0.5 mg/dL) and albumen values (3.91 vs. 4.02 g/dL) were not significantly different between the two groups. Moreover, those with MASLD cirrhosis had significantly higher LSM measurement (37.22 kPa) and FAST score (0.52 vs. 0.15) compared to those without, and higher CAP (318.73 vs. 307.75 dB). The characteristics of AGILE 4 stratified patients are shown in Table 3.
Discussion
The noninvasive blood tests such as APRI, NFS and FIB-4 are highly effective at ruling out advanced fibrosis and cirrhosis in MASLD patients but have a relatively low specificity and PPV [9]. Several studies have shown that elastography measurements (CAP, LSM) significantly improve the diagnostic accuracy for MASLD. For example, a prospective analysis of MASLD patients by Eddowes et al. demonstrated an increased prevalence for identifying liver steatosis and fibrosis using FibroScan, thereby showing increased negative predictive values (AURCO 0.87) [10]. Another study by Siddiqui et al. showed that the diagnostic accuracy of VCTE in detecting adults with MASLD accurately distinguishes advanced vs. earlier stages of fibrosis using liver histology as the standard reference [11]. Elastography is more sensitive and specific than traditional ultrasound in detecting fatty liver in the presence of moderate and high probability for fibrosis, however; combining it with blood biomarkers can further enhance the diagnostic accuracy [12, 13].
AGILE 3 + and AGILE 4 scores are an amalgam of elastography measurements (LSM), lab chemistry values (transaminases, platelets) and clinical parameters (presence of diabetes, gender and age), and are thus an attempt to enhance the overall accuracy of diagnostic testing for advance fibrosis and cirrhosis among MASLD patients, respectively. The incorporation of clinical parameters such as diabetes, gender and age into AGILE scores stems from the blatant evidence of their contribution to pathogenesis [14]. Furthermore, AGILE scores utilize a “dual cut-off algorithm” approach which has been shown to correctly classify advanced fibrosis and cirrhosis in hepatitis B patients, reduce the indeterminate grey zone and the false positive rate, and hence reducing the need for liver biopsy [15].
Similar “combining scores” have also been previously developed. For example, Newsome et al. developed FAST score by combining elastography measurements (LSM and CAP) and lab values (AST, ALT, or AST: ALT ratio) [16]. One of the shortcomings of this scoring system is that unlike AGILE scores, FAST score does not take clinical parameters into account. Furthermore, it primarily focuses on patients with fibrosis stage 2 or higher, whereas F ≥ 3 is more clinically relevant. In our study, we aimed to estimate the prevalence of MASLD-related advanced fibrosis and cirrhosis using the AGILE 3 + AGILE 4 scores; respectively, in a U.S. representative sample. In this study of 1244 patients with evidence of MASLD, the overall prevalence of AF due to MASLD based on AGILE 3 + score was 8.1%, corresponding to 4.5 million Americans, whereas based on the AGILE 4 score, the overall prevalence of MASLD cirrhosis was 1.1%, corresponding to 610,000 Americans.
The AGILE scoring system is a non-proprietary test and can be calculated using routinely collected clinical, elastography and laboratory parameters. It is crucial to balance the accuracy of the diagnostic tools and the feasibility of obtaining the diagnostic information in population-based epidemiological studies like this.
Limitations
The limitations of our study stem mainly from the nature of the AGILE scoring system itself. For instance, individuals who fall under the grey zone (11.5% using AGILE 3 + and 2.4% using AGILE 4) require further evaluation for the presence of MASLD cirrhosis, and therefore evaluating the cost-effectiveness of using AGILE3 + and AGILE 4 as a screening tool in high-risk populations is needed before implementing in clinical practice. Furthermore, despite using a nationally representative sample (NHANES database), the question whether the selected population truly represents the U.S population after applying the inclusion and exclusion criteria remains difficult to answer. The cut off for steatosis () was used based on published literature to maximize sensitivity [17], and different cut off may be used in the clinical settings.
Data availability
Datasets used in this analysis can be found online on NHANES database website (https://wwwn.cdc.gov/nchs/nhanes/) for free public access.
Abbreviations
- MASLD:
-
Non-alcoholic liver disease
- ALD:
-
Alcoholic liver disease
- VCTE:
-
vibration-controlled transient elastography
- NHANES:
-
The National Health and Nutrition Examination Survey
- NCHS:
-
National Center for Health Statistics
- CDC:
-
Center for Disease Control and Prevention
- LSM:
-
Liver stiffness measurement
- CAP:
-
Controlled attenuation parameter
- ALT:
-
Alanine Aminotransferase
- AST:
-
Aspartate Aminotransferase
- BMI:
-
Body Mass Index {BMI}
- HBA1C:
-
Haemoglobin A1C
References
Younossi Z, Anstee QM, Marietti M, Hardy T, Henry L, Eslam M, George J, Bugianesi E. Global burden of MASLD and MASLD: trends, predictions, risk factors and prevention. Nat Rev Gastroenterol Hepatol. 2018;15(1):11–20. https://doi.org/10.1038/nrgastro.2017.109. Epub 2017 Sep 20. PMID: 28930295.
Brown GT, Kleiner DE. Histopathology of nonalcoholic fatty liver disease and nonalcoholic steatohepatitis. Metabolism. 2016;65:1080–6.
Wong RJ, Liu B, Bhuket T. Significant burden of nonalcoholic fatty liver disease with advanced fibrosis in the US: a crosssectional analysis of 2011–2014 National Health and Nutrition Examination Survey. Aliment Pharmacol Ther. 2017;46:974–80.
Matteoni C, Younossi ZM, Gramlich T, et al. Nonalcoholic fatty liver disease: a spectrum of clinical and pathological severity. Gastroenterology. 1999;116:1413–9.
Williams CD, Stengel J, Asike MI, et al. Prevalence of nonalcoholic fatty liver disease and nonalcoholic steatohepatitis among a largely middle-aged population utilizing ultrasound and liver biopsy: a prospective study. Gastroenterology. 2011;140:124–31.
Wai CT, Greenson JK, Fontana RJ, et al. A simple noninvasive index can predict both signifi cant fi brosis and cirrhosis in patients with chronic hepatitis C. Hepatology. 2003;38:518–26.
Kruger FC, Daniels CR, Kidd M, et al. APRI: a simple bedside marker for advanced fi brosis that can avoid liver biopsy in patients with MASLD/MASLD. South Afr Med J. 2011;101:477–80.
Sterling RK, Lissen E, Clumeck N, et al. Development of a simple noninvasive index to predict significant fibrosis in patients with HIV/HCV coinfection. Hepatology. 2006;43:1317–25.
Hernaez R, Lazo M, Bonekamp S, Kamel I, Brancati FL, Guallar E, Clark JM. Diagnostic accuracy and reliability of ultrasonography for the detection of fatty liver: a meta-analysis. Hepatology. 2011;54(3):1082–90. https://doi.org/10.1002/hep.24452. PMID: 21618575; PMCID: PMC4197002.
Eddowes PJ, Sasso M, Allison M, Tsochatzis E, Anstee QM, Sheridan D, Guha IN, Cobbold JF, Deeks JJ, Paradis V, Bedossa P, Newsome PN. Accuracy of FibroScan Controlled Attenuation parameter and liver stiffness measurement in assessing steatosis and fibrosis in patients with nonalcoholic fatty liver disease. Gastroenterology. 2019;156(6):1717–30. https://doi.org/10.1053/j.gastro.2019.01.042. Epub 2019 Jan 25. PMID: 30689971.
Siddiqui MS, Vuppalanchi R, Van Natta ML, Hallinan E, Kowdley KV, Abdelmalek M, Neuschwander-Tetri BA, Loomba R, Dasarathy S, Brandman D, Doo E, Tonascia JA, Kleiner DE, Chalasani N, Sanyal AJ, MASLD Clinical Research Network. Vibration-controlled transient elastography to assess fibrosis and steatosis in patients with nonalcoholic fatty liver disease. Clin Gastroenterol Hepatol. 2019;17(1):156–e1632. Epub 2018 Apr 26. PMID: 29705261; PMCID: PMC6203668.
Nahon P, Kettaneh A, Tengher-Barna I, Ziol M, de Lédinghen V, Douvin C, Marcellin P, Ganne-Carrié N, Trinchet JC, Beaugrand M. Assessment of liver fibrosis using transient elastography in patients with alcoholic liver disease. J Hepatol. 2008;49(6):1062–8. Epub 2008 Oct 7. PMID: 18930329.
YounossiZM, Noureddin M, Bernstein D. Role of noninvasive tests in clinical gastroenterology practices to identify patients with nonalcoholic steatohepatitis at high risk of adverse outcomes: expert panel recommendations. Am J Gastroenterol. 2021;116(2):254–62. https://doi.org/10.14309/ajg.000000000000105433284184.
Kawano Y, Cohen DE. Mechanisms of hepatic triglyceride accumulation in non-alcoholic fatty liver disease. J Gastroenterol. 2013;48:434–41. https://doi.org/10.1007/s00535-013-0758-5.
Viganò M, Paggi S, Lampertico P, Fraquelli M, Massironi S, Ronchi G, Rigamonti C, Conte D, Colombo M. Dual cut-off transient elastography to assess liver fibrosis in chronic hepatitis B: a cohort study with internal validation. Aliment Pharmacol Ther. 2011;34(3):353 – 62. doi: 10.1111/j.1365-2036.2011.04722.x. Epub 2011 Jun 1. PMID: 21631559.
Newsome PN, Sasso M, Deeks JJ, Paredes A, Boursier J, Chan WK, Yilmaz Y, Czernichow S, Zheng MH, Wong VW, Allison M, Tsochatzis E, Anstee QM, Sheridan DA, Eddowes PJ, Guha IN, Cobbold JF, Paradis V, Bedossa P, Miette V, Fournier-Poizat C, Sandrin L, Harrison SA. FibroScan-AST (FAST) score for the non-invasive identification of patients with non-alcoholic steatohepatitis with significant activity and fibrosis: a prospective derivation and global validation study. Lancet Gastroenterol Hepatol. 2020;5(4):362–373. doi: 10.1016/S2468-1253(19)30383-8. Epub 2020 Feb 3. Erratum in: Lancet Gastroenterol Hepatol. 2020;5(4):e3. PMID: 32027858; PMCID: PMC7066580.
Alkhouri N, Almomani A, Le P, et al. The prevalence of alcoholic and nonalcoholic fatty liver disease in adolescents and young adults in the United States: analysis of the NHANES database. BMC Gastroenterol. 2022;22:366. https://doi.org/10.1186/s12876-022-02430-7.
Acknowledgements
Not Applicable.
Author information
Authors and Affiliations
Contributions
Naim Alkhouri- Principal Investigator and first co-author; Ashraf Almomani- First co-author; Phuc Le and Julia Y. Payne- data analysts; Imad Asaad, Prido Polanco, Phillip Leff, Prabhat Kumar and Mazen Noureddin - manuscript authors.
Corresponding author
Ethics declarations
Ethics approval and consent to participate
This study was done by retrospective analysis of de-identified data. Institutional Review Board (IRB) approval and Informed Consents were obtained by the dataset creators (Protocol #2018-01). No consent or IRB approval was obtained for this study since it is utilizing a de-identified data.
Consent for publication
Since the data used in this analysis are de-identified data, the consent for publication is not applicable. However, all related consents were obtained by the NHANES dataset authors and can be found online (https://wwwn.cdc.gov/nchs/nhanes/).
Competing interests
The authors declare no competing interests.
Additional information
Publisher’s Note
Springer Nature remains neutral with regard to jurisdictional claims in published maps and institutional affiliations.
Rights and permissions
Open Access This article is licensed under a Creative Commons Attribution 4.0 International License, which permits use, sharing, adaptation, distribution and reproduction in any medium or format, as long as you give appropriate credit to the original author(s) and the source, provide a link to the Creative Commons licence, and indicate if changes were made. The images or other third party material in this article are included in the article’s Creative Commons licence, unless indicated otherwise in a credit line to the material. If material is not included in the article’s Creative Commons licence and your intended use is not permitted by statutory regulation or exceeds the permitted use, you will need to obtain permission directly from the copyright holder. To view a copy of this licence, visit http://creativecommons.org/licenses/by/4.0/. The Creative Commons Public Domain Dedication waiver (http://creativecommons.org/publicdomain/zero/1.0/) applies to the data made available in this article, unless otherwise stated in a credit line to the data.
About this article
Cite this article
Alkhouri, N., Almomani, A., Le, P. et al. The prevalence of metabolic dysfunction-associated steatotic liver disease (MASLD)-related advanced fibrosis and cirrhosis in the United States population utilizing AGILE 3 + and AGILE 4 scores: analysis of the NHANES 2017–2018 cycle. BMC Gastroenterol 24, 218 (2024). https://doi.org/10.1186/s12876-024-03295-8
Received:
Accepted:
Published:
DOI: https://doi.org/10.1186/s12876-024-03295-8