Abstract
Background
Gastrointestinal nematodes (GIN), liver flukes (Fasciola hepatica) and bovine lungworms (Dictyocaulus viviparus) are the most important parasitic agents in pastured dairy cattle. Endoparasite infections are associated with reduced milk production and detrimental impacts on female fertility, contributing to economic losses in affected farms. In quantitative-genetic studies, the heritabilities for GIN and F. hepatica were moderate, encouraging studies on genomic scales. Genome-wide association studies (GWAS) based on dense single nucleotide polymorphism (SNP) marker panels allow exploration of the underlying genomic architecture of complex disease traits. The current GWAS combined the identification of potential candidate genes with pathway analyses to obtain deeper insights into bovine immune response and the mechanisms of resistance against endoparasite infections.
Results
A 2-step approach was applied to infer genome-wide associations in an endangered dual-purpose cattle subpopulation [Deutsches Schwarzbuntes Niederungsrind (DSN)] with a limited number of phenotypic records. First, endoparasite traits from a population of 1166 Black and White dairy cows [including Holstein Friesian (HF) and DSN] naturally infected with GIN, F. hepatica and D. viviparus were precorrected for fixed effects using linear mixed models. Afterwards, the precorrected phenotypes were the dependent traits (rFEC-GIN, rFEC-FH, and rFLC-DV) in GWAS based on 423,654 SNPs from 148 DSN cows. We identified 44 SNPs above the genome-wide significance threshold (pBonf = 4.47 × 10− 7), and 145 associations surpassed the chromosome-wide significance threshold (range: 7.47 × 10− 6 on BTA 1 to 2.18 × 10− 5 on BTA 28). The associated SNPs identified were annotated to 23 candidate genes. The DAVID analysis inferred four pathways as being related to immune response mechanisms or involved in host-parasite interactions. SNP effect correlations considering specific chromosome segments indicate that breeding for resistance to GIN or F. hepatica as measured by fecal egg counts is genetically associated with a higher risk for udder infections.
Conclusions
We detected a large number of loci with small to moderate effects for endoparasite resistance. The potential candidate genes regulating resistance identified were pathogen-specific. Genetic antagonistic associations between disease resistance and productivity were specific for specific chromosome segments. The 2-step approach was a valid methodological approach to infer genetic mechanisms in an endangered breed with a limited number of phenotypic records.
Similar content being viewed by others
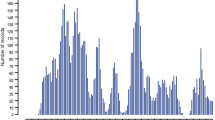
Background
Endoparasite infections imply impaired cattle health and increasing economic losses in pasture-based production systems [1, 2]. Gastrointestinal nematodes (GIN), the bovine lungworm (Dictyocaulus viviparus) and the liver fluke (Fasciola hepatica) are the most important parasitic helminths of pastured dairy cows [3, 4]. Subclinical infections were associated with reduced milk production [5, 6], impaired reproductive performance [7] and a decrease in product quality [8].
From a farm management perspective, prophylactic as well as diagnosis-based anthelmintic treatments can be applied to control endoparasite infections in affected dairy herds [4, 9]. However, anthelmintic treatments are expensive, and drug residues might pollute the environment and food products [10]. Furthermore, anthelmintic treatments contribute to anthelmintic resistance [11,12,13]. Hence, sustainable endoparasite control implies the consideration of proper breeding and selection strategies [9, 14]. In this regard, breeding approaches have focused on the selection of specific breeds or genetic lines representing enhanced resistance against specific endoparasites [15, 16].
In cattle, heritability estimates ranged from 0.04 to 0.36 for various definitions of GIN and D. viviparus infections [e.g., fecal egg count (FEC), antibody level], indicating a genetic component for pathogen-specific susceptibility [17, 18]. For the liver fluke F. hepatica, the heritabilities ranged from 0.09 to 0.33 [15, 19, 20]. The pronounced additive-genetic variances identified were stimuli to explore the underlying genomic architecture for endoparasite resistance in cattle and sheep, with a focus on GIN [21,22,23]. Genome-wide association studies (GWAS) using dense single-nucleotide polymorphism (SNP) marker panels and QTL mapping approaches contributed to the identification of candidate genes related to immune mechanisms (e.g., the IFNγ gene, major histocompatibility complex (MHC)-related genes) against GIN infections in cattle and sheep [24,25,26,27]. Coppieters et al. [27] based their studies on microsatellite mapping in Dutch HF cows, and they identified two genome-wide significant QTL on BTA 9 and on BTA 19 influencing FEC for GIN infections. In an experimental Angus population and using microsatellite markers, genome-wide suggestive QTL on BTA 8 and potential linkage with segments on BTA 4, 12 and 17 were associated with patent GIN infections [28]. A GWAS based on 50,000 SNPs identified 12 genomic regions on BTA 3, 5, 8, 15 and 27 as contributing to FEC variation in Angus cattle [29]. Potential candidate genes were related to immunological pathways, i.e., the toll-like receptor-signaling pathway and the cytokine-cytokine receptor interaction pathway [29]. In Angus cattle, association studies based on copy number variations (CNV) have identified immune-related genes, i.e., the genes involved in GIN resistance mechanisms [23, 30, 31]. Although infections with the liver fluke F. hepatica in dairy cattle represent a serious animal health problem worldwide [32], studies with a focus on the identification of genomic variants influencing F. hepatica resistance are lacking. In sheep, a QTL microsatellite mapping study [33] detected QTL for resistance against Fasciola gigantica on OAR 10, 13, 17, 18 and 19, 22.
In Europe, the rising importance of maintaining dairy cattle in grassland systems implies exposure to endoparasite infections and further pathogenic agents [34]. Thus, there is increasing interest in local breeds being best adapted to harsh environments and being less susceptible against infections. The local dual-purpose German Black Pied cattle (DSN, German: Deutsches Schwarzbuntes Niederungsrind) is the founder breed of the modern Holstein Friesian (HF) cattle, with a long breeding history in the grassland region of East Frisia, Lower Saxony, Germany [35]. DSN are an endangered breed because they are not competitive with HF regarding milk and protein yield. DSN are defined as robust cattle under harsh environmental conditions [36], and they show better female fertility parameters and a better health status for metabolic disorders after calving compared with HF [37]. Susceptibility to endoparasite infections as measured by the levels of endoparasite burden (i.e., resistance) may not reflect the host’s actual ability to limit the impact of endoparasite infections on fitness and production (i.e., tolerance) [38]. Hence, DSN cows with a high FEC for F. hepatica and larvae counts for D. viviparus had low somatic cell counts [15]. The udder somatic cell count is a commonly used indicator for mastitis and udder health in overall breeding goals [39]. High levels of somatic cells in milk reflect leukocyte recruitment and indicate udder inflammation. Pimentel et al. [40] discussed antagonistic associations among functional traits and between functional traits and productivity on quantitative-genetic and phenotypic scales. However, the correlations were partly favorable when only considering specific important chromosome segments. Hence, a deeper understanding of the physiological or biological trait interactions is imperative in order to infer the antagonistic relationships between resistance against endoparasites and resistance against udder infections.
For small cattle populations, the limited number of records for complex quantitative traits, especially for health traits, is a special challenge in genomic studies [41]. The use of multibreed reference populations to train on data from several breeds simultaneously was suggested to increase genomic prediction accuracies for production traits [42, 43]. However, it remains challenging to harmonize recording schemes for novel traits across country borders. Within countries, a further methodological approach might be a 2-step strategy. Step 1 involves using a larger data pool of phenotypes from several breeds or genetic lines and correcting the data for fixed effects. Afterwards, in step 2, precorrected phenotypes are dependent traits in GWAS, considering just the small population. A similar strategy was applied to infer quantitative-genetic (co) variance components in small datasets including only daughter records from specific sires [44].
The objectives of the present study were i) to identify genome-wide associations for resistance or susceptibility (measured by FEC) to three endoparasite infections (GIN, D. viviparus and F. hepatica) in the endangered DSN breed using a 2-step approach; ii) to assess annotations to potential candidate genes and to infer physiological pathways; and iii) to estimate how SNP affects the correlations among endoparasite traits and between endoparasite traits with the test-day milk yield and test-day somatic cell count in chromosomal segments with an impact on disease resistance.
Methods
Animal ethics statement
The whole genotyping process from tissue sampling up to the SNP database development was embedded in the logistics and infrastructure of the cow genotyping activities in Germany, organized by the German Holstein breeding organization and their participating regional breeding organizations and farmers. This activity is the basis to implement a national cow training set for genomic selection. Farmers agreed to collect small fecal samples for endoparasite determinations. Such fecal collection does not influence the wellbeing of cows. Fecal samples were analyzed in the laboratory of the university. Thus, no ethical approval was required for this study.
Animals
The study was incorporated in the framework of a ‘pasture genetics project’ established in 2007 in northwestern Germany. In the framework of this project, a sample of 1166 German Black and White dairy cows distributed over 17 grassland farms was used for genetic line comparisons and quantitative-genetic studies [15]. The five Black and White genetic lines included an HF line selected for milk yield (HF milk); an HF line suited for grazing conditions (HF pasture); a New Zealand HF line (HF NZ); crosses between HF with Jersey, Angler or beef cattle sires (HF cross); and DSN. All cows were exposed to endoparasite infections (access to pasture before 1st of June with > 8 h per day) and not treated with anthelmintics in the sampling year.
A subset of 148 DSN cows from three different farms was selected for genotyping using a selective genotyping approach. In this regard, the selection criteria were i) the herd prevalence for GIN, as GIN was the endoparasite with the highest prevalence in the initial dataset of 1166 cows, ii) individual parasitological measurements per farm (i.e., considering the extreme phenotypes per herd for GIN), and iii) the pedigree-based genetic relationships. The aim was to minimize the average relationship coefficients between all cows selected for genotyping within and between GIN-infected and GIN-non infected cows.
Phenotypes
The endoparasite dataset considered FEC for GIN (FEC-GIN) and F. hepatica (FEC-FH) as well as fecal larvae counts (FLC) for D. viviparus (FLC-DV). Based on the coproscopical results, the predominant morphotype for GIN was strongylid eggs (Trichostrongylidae or Oesophagostomum and Bunostomum spp., respectively) followed by Strongyloides papillosus and Capillaria spp. eggs (see May et al. [45]). The whole dataset (n = 1166 cows) considered repeated measurements for 840 cows. The endoparasite trait definitions in the laboratory are described by May et al. [15]. The test-day production traits included repeated measurements from the whole lactation of the sampling year. Cows with less than five test-day records were excluded from the analysis. The somatic cell counts were log-transformed into somatic cell score: SCS = log2 (SCC/100.000) + 3 [46]. Descriptive statistics for the endoparasite traits (FEC-GIN, FEC-FH, FLC-DV) and test-day traits (MY, SCS) for all Black and White cows (whole dataset, n = 1166 cows) and for the genotyped DSN cows (genotype dataset, n = 148) are displayed in Table 1.
Genotypes and quality control
The DSN cows were genotyped using the BovineSNP50 Bead Chip V2 (50k SNP chip) following the Illumina Infinium assay protocol (Illumina Inc., San Diego, CA, USA). In the next step, the genotypes were imputed into Illumina HD Bead Chip level (700 k SNP chip) using a multibreed reference panel of 2188 animals. The reference panel considered 48 DSN animals genotyped with the Illumina HD 700 k Bead Chip array (Illumina Inc., San Diego, CA, USA) and 2140 sequenced animals (including 30 sequenced DSN animals) from the 1000 bull genome project database [47] downscaled to Illumina HD Bead Chip density. Imputation was performed using BEAGLE 4.1 software [48]. The average imputation accuracy from a leave-one-out approach [49] was 89.3%. Only SNP markers on autosomes with validated locations (i.e., based on BLAST analysis against the bovine genome assembly UMD3.1) were considered [49]. The imputed dataset included 587,615 SNP markers from 148 DSN cows. Quality control of the imputed genotype data was performed using the software package PLINK, version 1.9 [50]. SNP markers with a minor-allele frequency (MAF) < 0.05, significant deviation from Hardy-Weinberg equilibrium (HWE, p < 10− 6) or a call rate < 95% were discarded. Individuals with a call rate < 95% were also excluded. After quality control, the final dataset for GWAS contained 423,654 SNP marker genotypes.
Potential stratification in the dataset due to relatedness among sampled individuals was examined prior to the GWAS using a principal component analysis (PCA). The PCA based on the variance-standardized relationship matrix was derived from the SNP markers as implemented in the --pca option in PLINK.
Statistical models
Precorrection of phenotypic data
In the first step, phenotypes for the endoparasite traits (FEC-GIN, FLC-DV, FEC-FH) and the test-day traits MY and SCS were precorrected for fixed effects using the initial dataset from all 1166 Black and White cows (consideration of all genetic lines). Precorrection of the endoparasite traits for fixed effects was accomplished via a linear mixed model analysis (model 1) using the statistical software SAS, version 9.4 [51] (SAS PROC MIXED, ML method):
yijklm = observations for FEC-GIN, FEC-FH and FLC-DV; μ = overall mean effect; Fi = fixed effect of the ith farm (i = 1, …, 17); Pj = fixed effect of the jth parity number (j = 1, 2, 3, 4, > 4); GLk = fixed effect of the kth genetic line (k = HF milk, HF pasture, HF NZ, DSN, HF cross); SPl = fixed effect of the lth sampling period (l = June/July, September/October); LSm = fixed effect of the mth lactation stage according to Huth (1995) (m = < 14 days in milk (DIM), 14–77 DIM, 78–140 DIM, 141–231 DIM, ≥232 DIM); and eijklm = random residual effect.
Hereafter, the precorrected phenotypes (residuals) for the endoparasite traits (FEC-GIN, FEC-FH, FLC-DV) are denoted as rFEC-GIN, rFEC-FH and rFLC-DV, respectively. Precorrected phenotypes were available from 148 genotyped DSN cows. The distribution of residuals for the endoparasite traits was checked and visually inspected. To further validate the precorrection approach, we correlated the estimated breeding values (EBVs) for all three endoparasite traits from the animal models in May et al. [15] with EBVs from animal models based on precorrected phenotypes. For all traits the EBV correlations between models were > 0.95.
Accordingly, the test-day traits were precorrected for fixed effects via linear mixed model applications (model 2) (SAS PROC MIXED, ML method):
yijklm = observations for MY and SCS; HTDi = fixed effect of the ith herd-test-date; Pj = fixed effect of jth parity number (1, 2, 3, 4, > 4); TSk = fixed effect of kth time span between each test-day record and the endoparasite sampling date (≤ − 200, > − 200 and ≤ − 100, > − 100 and ≤ 0, > 0 and ≤ 100, > 100); YSl = fixed effect of lth year-season of last calving (spring, summer, autumn, winter within each year); DIMm = covariate for days in milk modeled with Legendre polynomials of order 3; and eijklm = random residual effect. Hereafter, the precorrected phenotypes (residuals) for the test-day MY and SCS are denoted as rMY and rSCS, respectively.
Genome-wide association analyses
In the second step, precorrected phenotypes (i.e., residuals from step 1: rFEC-GIN, rFEC-FH, rFLC-DV, rMY, rSCS) were used as dependent variables in single-trait GWAS as implemented in the software package GCTA [52]. All association analyses were performed using the --mlma option in GCTA. The following statistical model for testing single-locus SNP effects was applied:
where y = vector of precorrected phenotypes (rFEC-GIN, rFLC-DV, rFEC-FH, rMY and rSCS); μ = the overall mean; b = additive fixed effect of the candidate variant tested for association; x = vector of genotypes for the candidate SNP; \( \boldsymbol{u}\sim N\left(0,\boldsymbol{G}{\sigma}_u^2\right) \) = vector of random polygenic effects; G = genomic relationship matrix (GRM); \( {\sigma}_u^2 \) = polygenic variance estimated from a null model (i.e., y = 1μ + u + e); and \( \boldsymbol{e}\sim N\left(0,\boldsymbol{I}{\sigma}_e^2\right) \) = vector of random residual effects, where I = an identity matrix and \( {\sigma}_e^2 \) = the residual variance.
An adjusted Bonferroni correction was applied to account for multiple testing. The traditional Bonferroni correction (i.e., relating the genome-wide significance threshold of 0.05 to the total number of SNP) tends to produce many false-negative results [53]. Therefore, the effective number of independent SNP markers in the analysis (n = 111,901) was estimated based on the LD between markers using the software GEC [54]. The adjusted Bonferroni-corrected genome-wide significance threshold with (p = 0.05 / n) was pBonf = 4.47 × 10− 7. In addition, we considered a chromosome-wide significance threshold (pCand = 0.05 / nc), where nc is the effective number of independent SNP markers of the respective chromosome. In this regard, we applied GEC [54]. Chromosome-wide significance thresholds ranged from 7.47 × 10− 6 (BTA 1) to 2.18 × 10− 5 (BTA 28) (Additional file 1: Table S1).
Candidate gene annotation and pathway analyses
The biomaRt package [55, 56] from the Bioconductor project was applied to retrieve ‘rs accession numbers’ of associated SNP markers using the getBM() function. Potential candidate genes were queried and assigned to associated SNP markers using the current gene annotations from the ENSEMBL (Version 90) [57] and NCBI (Version 105) [58] databases. A gene was considered as a candidate gene if at least one associated SNP marker above pCand was positioned i) in the respective gene and/or ii) within 5 kb up- and downstream of the respective candidate gene. Regions including the candidate gene ±5 kb up- and downstream are hereafter referred to as regions of interest (ROI). The potential candidate genes identified were manually submitted to the DAVID database (Version 6.8) [59] for pathway and enrichment analyses. In addition, physiological functions and positions of potential candidate genes were further manually reviewed in the KEGG [60], ENSEMBL [57] and NCBI [58] databases.
Calculation of SNP effect correlations between traits
SNP effect correlations were calculated i) among rFEC-GIN, rFEC-FH and rFLC-DV for the respective potential candidate genes for each trait within all identified ROI and ii) within identified ROI for rFEC-GIN, rFEC-FH and rFLC-DV with rMY and rSCS. SNP effects were not correlated for four ROI for rFLC-DV (corresponding genes: FAM124B, ISL2, RCN2, and SCAPER) due to the limited number of marker associations (see Table 2) or identical SNP effects within traits (no variance for the respective ROI).
Results
Population stratification
Additional file 2: Figure S1 includes the top two PCs plotted against each other to visualize the population structure with additional color representation for i) individual farm affiliations and ii) individual endoparasite phenotypes. The analysis revealed three main clusters within the whole population caused primarily by the three different farms. Relationships were closer between the individuals of farm 1 (41 cows) and farm 2 (66 cows), whereas the individuals of farm 3 (41 cows) were not closely linked among each other or to farm 1 and farm 2 individuals. Generally, we only found slight stratification induced by kinship. Hence, we did not account for population stratification via the consideration of PCs in the models for GWAS.
Genome-wide association analysis for endoparasite traits
Manhattan plots from the GWAS and corresponding Q-Q plots for rFEC-GIN, rFEC-FH and rFLC-DV are given in Fig. 1. For rFEC-GIN, GWAS identified 17 associated SNP markers based on pCand on 9 chromosomes (Additional file 3: Table S2). None of the SNP markers reached the pBonf level. Most of the associations were detected on BTA 2 (n = 4) and BTA 18 (n = 3). For rFEC-FH, GWAS identified three SNP markers above pBonf on BTA 7 (Additional file 4: Table S3). In total, three additional variants surpassed the suggestive candidate thresholds pCand on the three chromosomes BTA 1, 7 and 28. GWAS for rFLC-DV identified 41 associations according to the pBonf threshold on BTA 2, 5, 8, 15, 17, 21 and 24 (Additional file 5: Table S4). Moreover, 125 additional variants exceeded the pCand level with a majority (n = 44) positioned on BTA 29.
Manhattan plot displaying the GWAS results (p-values and corresponding Q-Q plot of observed p-values against the expected p-values) for a rFEC-GIN, b rFEC-FH, and c rFLC-DV. Bonferroni-corrected genome-wide significance (red line), SNP marker above pBonf (marked in red) and SNP marker above suggestive of the chromosome-wide significance threshold (range: p = 7.47 × 10− 6 on BTA 1 to p = 2.18 × 10− 5 on BTA 28) (marked in blue) are also shown
Gene annotation and pathway analysis
We identified five potential candidate genes for rFEC-GIN (Table 2). More than two neighboring significantly associated SNP markers without any non-associated marker positioned between them were defined as an association cluster. One association cluster including two variants on BTA 24 was related to the PHLPP1 (PH domain and leucine rich repeat protein phosphatase 1) gene. The PHLPP1 gene is a protein coding gene involved in immunological processes (PI3K-Akt signaling pathway; KEGG entry: bta04915), e.g., the regulation of T cell energy (Table 3). Functional annotation from rFEC-GIN candidate genes revealed the estrogen signaling pathway (KEGG entry: bta04915) for the candidate gene EGFR (Table 3). We identified three immunological pathways for the EGFR (Epidermal growth factor receptor) gene, with the regulating cells involved in innate as well as adaptive inflammatory host defenses (Table 3). The ALCAM (Activated leukocyte cell adhesion molecule) gene was related to rFEC-FH on BTA 1 (Table 2). This gene was annotated to the (immune) cell adhesion molecules (CAMs) pathway (KEGG entry: bta04514) (Table 3).
We detected 17 potential candidate genes for rFLC-DV (Table 2). An association cluster including 24 consecutively positioned and associated SNP markers was detected on BTA 21. This cluster is related to the SCAPER (S-Phase cyclin A associated protein in the endoplasmic reticulum) gene. The GSG1 (Germ cell associated 1) gene and the RIMKLB (Ribosomal modification protein RimK-like family member B) gene on BTA 5 revealed association clusters, too. Functional annotation from the rFLC-DV candidate genes revealed the cell adhesion molecules (CAMs) pathway for the CDH2 gene on BTA 24 (Table 3).
The NAV3 (Neuron navigator 3) gene was the only candidate gene associated with more than one trait (rFEC-GIN and rFLC-DV). NAV3 is involved in the regulation of interleukin 2 production by T cells. However, the marker associations within NAV3 did not overlap between rFEC-GIN and rFLC-DV. For rFEC-GIN, one SNP marker positioned in the middle of NAV3 was identified above pCand. For rFLC-DV, two SNP markers positioned near the gene start position were significantly associated. The space between both association signals for rFEC-GIN and rFLC-DV in NAV3 was approximately 235 kb.
SNP effect correlations between endoparasite traits
The number of SNP markers within all identified ROI ranged from 8 to 197. For three of the five ROI for rFEC-GIN, we found antagonistic (negative) SNP effect correlations (− 0.32 to − 0.69) between rFEC-GIN with rFEC-FH (Fig. 2). Only on BTA 5 (6,772,101 – 7,683,220) and within the ROI on BTA 24 (bp 61,563,972 – 61,797,092) were correlations slightly positive (Fig. 2). We detected moderate to high SNP effect correlations (0.34 to 0.87) between rFEC-GIN and rFLC-DV within all ROI for rFEC-GIN. The SNP effect correlation between marker effects for rFEC-FH and rFEC-GIN was negative (− 0.17) for the ROI identified for rFEC-FH (Fig. 2). For the same ROI, the correlation between SNP effects for rFEC-FH and rFLC-DV was 0.73 (Fig. 2). Considering the identified ROI for rFLC-DV, correlations ranged from − 0.53 on BTA 24 to 0.99 on BTA 5 between marker effects for rFLC-DV and rFEC-GIN (Fig. 2). The correlations between the marker effects for rFLC-DV and rFEC-FH ranged from − 0.47 on BTA 5 to 0.99 on BTA 24 (Fig. 2). The correlation was 0.78 between the marker effects for rFLC-DV and rFEC-GIN considering the common ROI on BTA 5 (ROI: bp 6,772,101 – 7,683,220; including the NAV3 gene) (Fig. 3).
SNP effect correlations between endoparasite traits and test-day traits
The SNP effect correlations between rFEC-GIN, rFEC-FH and rFLC-DV with rMY and rSCS are presented in Fig. 4. The correlations between the marker effects for rFEC-GIN and rMY were negative (− 0.10 to − 0.42) for three ROI. The marker effect correlations between rFEC-GIN and rMY were moderate to large (0.31 to 0.73) for two ROI. Differing correlations between rFEC-GIN and rSCS were estimated for different ROI, i.e., positive correlations (0.02 to 0.32) on BTA 5 and 22 but negative correlations (− 0.47 to − 0.99) on BTA 4, 18 and 24.
SNP effect correlations between the residuals of endoparasite traits and the residuals of test-day traits somatic cell score (SCS) and milk yield (MY) for the identified genomic regions of potential physiological significance (candidate gene position plus 5 kb up- and downstream) for a rFEC-GIN, b rFEC-FH and c rFLC-DV
Regarding the ROI identified for rFEC-FH, the correlation between the SNP effects for rFEC-FH and rMY was − 0.67, and it was − 0.44 between the SNP effects for rFEC-FH and rSCS. The correlations between the SNP effects for rFLC-DV and rMY were in a positive range (0.00 to 0.80) for four ROI, with the largest correlation on BTA 21. Additionally, differing correlations between rFLC-DV and rMY were detected for different ROI from the same BTA on BTA 5. The SNP effect correlations between rFLC-DV and rSCS were positive (0.10 to 0.99) for 12 ROI and neutral or negative (0.00 to − 0.15) for two ROI. The correlations between rFLC-DV and rSCS differed for different ROI on BTA 24. Positive correlations for different ROI were observed on BTA 5. For seven ROI on BTA 2, 5 and 26, the correlations between the SNP effects for rFLC-DV and rMY ranged from − 0.11 to − 0.99, whereas those with rSCS were positive (ranging from 0.11 to 0.99).
Discussion
Genome-wide association analysis for endoparasite traits
In local breeds with a small population size (e.g., DSN), reduced genetic variation and diversity compared with the intensively selected HF breed is reported [61, 62]. Thus, in comparison with HF or beef cattle breeds [27,28,29], other SNP variants associated with endoparasite resistance have been expected. The cattle selection lines best adapted to harsh grassland environments (e.g., New Zealand HF lines, DSN) [35, 63] are often described as more robust and less susceptible to endoparasite infections [15]. One explanation addresses genetic resistance to disease or endoparasite infections via cellular immunological mechanisms and adaptive immune responses, which differ between breeds or selection lines [9, 64]. Hence, selection signatures were identified when grouping subpopulations according to DSN or HF gene percentages, and when focusing on genomic regions with an impact on disease resistance [65]. In addition, higher levels of genomic homogeneity and of genetic relatedness in DSN than in HF contribute to a decrease in polymorphism, influencing the power to detect marker associations [66].
For rFEC-GIN, the majority of SNP marker associations were detected on BTA 2. Candidate genes were identified on BTA 4, 5, 18, 22 and 24. In contrast, in Angus beef cattle, genomic regions on BTA 3, 5, 15 and 27 were significantly associated (−log10 P = 4.3) with GIN infections [29]. In Scottish Blackface sheep, evidence for associations with GIN infections were detected on OAR 6 and 14 [66], but the SNPs located on OAR 3 and 12 affected FEC in crossbred sheep (Martinique Black Belly x Romane sheep) [67]. For Dutch Holstein-Friesian dairy cattle, genome-wide suggestive QTL on BTA 11, 14, 21, 24, 25 and 27 were reported using a within-family analysis based on a dataset of 768 phenotyped cows [27]. In the same study, genome-wide significant QTL were identified on BTA 9 and 19 in an across-family analysis [27]. In agreement with the other studies based on FEC, we also detected significantly associated SNPs for GIN resistance (according to pCand) on BTA 5, 9 and 24.
The pre-selection of cattle according to phenotypes or allele frequencies, the phenotyping strategy (e.g., utilization of experimental vs. field data) [28, 29], and the differences in trait definitions and parasitological examination techniques are further possible explanations for the different GWAS results in different populations or breeds. In the current study, we did not distinguish among the different species according to the GIN morphotypes. We assumed that the diversity of GIN species in our study follows the distribution usually reported for cattle, with Ostertagia ostertagi as the most common species [68] followed by Cooperia spp. and Trichostrongylus spp. [69]. In field data studies, differences in infection exposure among individuals and environments and the variable infection pressure over time explain the reduced power to detect SNP associations and potentially mask the genetic signals [70,71,72]. The total number of associations was highest for rFLC-DV although a low prevalence was detected for D. viviparus in the multibreed dataset of 1166 cows [15]. False-negative marker associations could be the outcome in a dataset with a low number of infected cows. From this perspective, associations for rFLC-DV should be viewed with caution. However, the low prevalence of D. viviparus in our genotyped DSN cows reflected the phenotypic trait distribution in the whole cattle population.
GWAS results for GIN infections in previous studies [27, 29] reflect the findings for rFLC-DV. D. viviparus and GIN represent the same biological order (Strongylida). Thus, overlaps in marker associations between both species have been expected. We found signals on seven common chromosomes, with impacts on both the rFLC-DV and rFEC-GIN traits. A large number of associations and potential candidate genes were identified on BTA 2 and BTA 24 for rFEC-GIN and rFLC-DV. The NAV3 gene on BTA 5 was detected for both traits; however, no same SNP was identified to be simultaneously associated. Different SNP variants for different nematode species [trichostrongylids (herein referred to as GIN) and Nematodirus spp., which belong to the same biological order] were detected in Scottish Blackface lambs [66]. Furthermore, a GWAS for ectoparasites (different tick species) in cattle revealed SNP associations on different chromosomes for the ixodid tick species A. hebraeum and R. evertsi evertsi [73].
Regarding rFEC-FH, the three significant markers based on pBonf were not related to potential candidate genes. Efforts to characterize genes or genomic regions for liver fluke traits in ruminants were reported for Fasciola gigantica in sheep [33]. Thus, the current findings present a novelty for enhancing disease resistance to F. hepatica in cattle breeds.
Gene annotation and pathway analysis
Our study identified the cytokine-cytokine receptor interaction pathway for the EGFR gene for rFEC-GIN. In addition, this pathway was identified in a GWAS for Angus cattle [29]. The most interesting finding was the estrogen signaling pathway involving the potential candidate gene EGFR for rFEC-GIN. Experiments in mice have indicated that parasites can exploit the hormonal host microenvironment to favor their establishment, growth and reproduction rate [74, 75]. In this regard, for the tapeworm Taenia crassiceps, an increase in the physiological concentrations of the (host) hormone 17-ß-estradiol was associated with an increase in the reproductive capacity of T. crassiceps cysticerci [76]. Moreover, steroid hormone synthesis (e.g., progesterone, testosterone) influenced the fertility of Schistosoma mansoni and increased the length of Ascaris suum larvae in its host [77, 78]. However, it remains unclear whether similar mechanisms of host exploitation via the regulation of host hormones such as estradiol are also due to infections with GIN. The PI3K-Akt signaling pathway was annotated to several candidate genes for rFEC-GIN. There is evidence that the phosphatidylinositol-3 kinase (PI3K) plays a decisive role in cellular immune response, activated by costimulatory receptors of B and T cells in mice [79, 80]. Furthermore, the PI3K-Akt signaling pathway was identified for the protozoa Neospora caninum, an intracellular parasite that causes high economic losses in the cattle industry [81].
The activated leukocyte cell adhesion molecule (ALCAM) gene on BTA 1 detected for rFEC-FH is related to the cell adhesion molecules (CAMs) pathway, and it plays a crucial role in immune response mechanisms, e.g., cell adhesion interactions of T cells. Interestingly, the same pathway was also detected for D. viviparus as being related to the CDH2 (Cadherin 2, type 1, N-cadherin) gene on BTA 24. Our GWAS revealed several genes and three pathways as being involved in T lymphocyte interactions for rFEC-GIN, rFEC-FH and rFLC-DV. The cellular mechanisms mediated by T lymphocyte recruitment are typical features of immune response to endoparasite (especially helminth) infections in its host [82,83,84]. In cattle, natural infections with F. hepatica induce Th2-associated reactions, with simultaneous inhibition of Th1 cell activity [85]. Immune response against GIN mainly involves Th2 mechanisms in order to decrease the number of adult worms and of FEC. A mixed Th1/Th2 response follows infections with D. viviparus in cattle [85]. An interesting finding was made in Nelore cattle, where the immune response to the GIN species Cooperia punctata and Haemonchus placei was probably mediated by Th2 cytokines in the resistant cattle group and induced by Th1 cytokines in the susceptible ones [86, 87]. Thus, variations in identified immunological pathways might be expected when applying GWAS based on a stringent case-control (resistance and susceptible groups) design. The CDH2 and PCDH15 genes on BTA 24 and 26 for rFLC-DV coded for adhesion molecules, and they were expressed in the cattle selected for either resistance or susceptibility to nematode parasites [88].
Correlations between SNP effects for endoparasite traits
Differing correlations for SNP effects between rFEC-GIN, rFEC-FH and rFLC-DV indicate the complexity of resistance against different infectious agents. In most cases, negative SNP effect correlations were observed between rFEC-FH and rFEC-GIN, implying that genomic selection on improved resistance to F. hepatica infections simultaneously increased the susceptibility to GIN. The pedigree-based genetic correlations ranged from − 0.10 to 0.17 between different GIN and liver fluke trait definitions [15, 20]. One explanation for the negative correlations on a genomic scale between rFEC-FH and rFEC-GIN and for the divergent marker associations as well as gene annotations might be due to the variations in immune response mechanisms for different endoparasite species. In cattle, the specific immune response for F. hepatica (antibody response of the IgG1, the cellular response associated with the cytokines interleukin IL4, IL10, TNF-ß) differs from those for GIN and D. viviparus [89], where the immune response is predominantly mediated by IgA, IgE, IgG and IgM [90, 91].
The correlations based on the SNP effects between the two nematode traits rFEC-GIN and rFLC-DV were positive for most of the identified ROI, confirming the estimates from a quantitative-genetic study [15]. High positive genetic correlations between the different endoparasite species were reported in sheep [92, 93], which simplifies selection strategies.
Correlations between SNP effects for endoparasite and test-day traits
The SNP effect correlations between rFEC-GIN and rMY were negative for three of the five identified ROI, corresponding to the estimates from pedigree-based random regression models [15]. Twomey et al. [20] detected genetic correlations close to zero between milk yield and antibodies for Ostertagia ostertagi, the most common GIN species in cattle. In our study, mostly negative SNP correlations were inferred between rMY and rFLC-DV. Thus, on a genomic scale, breeding for higher milk production reduces larvae shedding of the bovine lungworm. Highly positive SNP effect correlations between rFLC-DV and rMY were detected for ROI on BTA 5 and 21, indicating a coregulation of both traits in these regions. Another possibility is that the genes affecting FLC for D. viviparus and MY were in a low linkage disequilibrium.
Regarding the associations between endoparasite traits and SCS, the SNP effect correlations between rFEC-GIN, rFEC-FH and rFLC-DV with rSCS for the ROI partly reflect quantitative-genetic estimates. May et al. [15] estimated positive (i.e., favorable from a breeding perspective) genetic correlations between FEC-GIN and SCS throughout lactation. In contrast, for most of the identified ROI, correlations based on SNP effects were unfavorable between rFEC-GIN and rSCS, but the SNP effect correlations between rFLC-DV and rSCS were positive (i.e., favorable from a breeding perspective). The negative SNP effect correlation between rFEC-FH and rSCS for the ROI on BTA 1 (including the ALCAM gene) reflects the pedigree-based estimates, i.e., the negative genetic correlations in the course of lactation [15]. Hence, breeding for reduced FEC for GIN or F. hepatica induces an increase in SCS. Vice versa, breeding on low somatic cells implies increasing FEC for GIN and F. hepatica. Such findings have practical relevance when developing breeding programs with a focus on both disease resistance and tolerance [94]. In particular, the antagonistic relationship between SCS and egg or larvae counts for endoparasite traits put into question the suitability of SCS as an indicator for udder health. Only moderate phenotypic and genetic correlations between SCS and clinical mastitis, as well as major pathogen susceptibility for cows with extremely low SCS [39], are a further justification in this regard. Mechanisms that decrease somatic cells in milk do not necessarily eliminate the causative pathogens during mastitis [95]. Phenotypically, the correlations between F. hepatica infections and SCS were close to zero (− 0.04 to 0.03 for different test-days around the parasitological examination date), reflecting the results from previous studies in HF dairy cow populations [6, 96]. Association analyses between ectoparasite and endoparasite infections with milk composition traits were of great interest in previous studies [20, 97]. Nevertheless, to our knowledge, this is the first approach focusing on the underlying genetic background between endoparasite infections and host defense mechanisms to further pathogen infections (e.g., increase in somatic cells).
Conclusions
The 2-step approach using precorrected phenotype data based on a larger dataset of related genetic lines was a valid approach to estimating SNP marker effects and to inferring possible candidate genes and biological pathways for endoparasite resistance in a small sample of genotyped dual-purpose DSN cows. Such a methodological approach might be suitable for genomic studies with a focus on novel traits in small populations. In total, 23 potential candidate genes were annotated to SNP marker associations for rFEC-GIN, rFEC-FH and rFLC-DV. A shared ROI (including the NAV3 gene) was only identified for GIN and D. viviparus on BTA 5. Five of the identified possible candidate genes were directly involved in immune response mechanisms. The inferred estrogen signaling pathway is involved in host-parasite interactions, and it appears to be specific for rFEC-GIN. Functional gene annotations identified a common immunological pathway (e.g., cell adhesion molecules pathway for rFEC-FH and rFLC-DV) for different endoparasite traits. The SNP effect correlations between rFEC-GIN and rFLC-DV were quite large for most of the ROI, indicating a partly joint genetic basis for traits representing the same biological order. The negative SNP effect correlation between rSCS and rFEC-FH is in agreement with pedigree-based genetic correlations, and it indicates an antagonistic association between disease resistance for udder and endoparasite infections. Generally, we demonstrated that resistance to the nematodes GIN and D. viviparus and to the trematode F. hepatica is under polygenic control through a large number of loci with moderate to small effects. The SNP effect correlations for specific endoparasite ROI provided deeper insight into trait associations and contributed to physiological explanations of possible genetic antagonisms between disease resistance and productivity. Predominantly negative SNP effect correlations between GIN or F. hepatica with SCS indicate the complexity of immune response mechanisms but also raise critical questions regarding breeding strategies on low somatic cell scores.
Abbreviations
- BLAST:
-
Basic Local Alignment Search Tool
- Bp:
-
Base pairs
- BTA:
-
Bos taurus chromosome
- CAMs:
-
Cell adhesion molecules
- CNV:
-
Copy number variations
- DSN:
-
Deutsches Schwarzbuntes Niederungsrind (German Black Pied cattle)
- EPG:
-
Eggs per gram feces
- FEC:
-
Fecal egg count
- FEC-FH:
-
Fecal egg count for Fasciola hepatica (liver flukes)
- FEC-GIN:
-
Fecal egg count for gastrointestinal nematodes
- FLC:
-
Fecal larvae count
- FLC-DV:
-
Fecal larvae count for Dictyocaulus viviparus (bovine lungworm)
- GIN:
-
Gastrointestinal nematodes
- GRM:
-
Genomic relationship matrix
- GWAS:
-
Genome-wide association analysis
- HF:
-
Holstein Friesian
- HTD:
-
Herd-test date
- HWE:
-
Hardy-Weinberg equilibrium
- IFNγ:
-
Interferon-Gamma
- IgG:
-
Immunoglobulin
- KEGG:
-
Kyoto Encyclopedia of Genes and Genomes
- LD:
-
Linkage disequilibrium
- MAF:
-
Minor allele frequency
- Mb:
-
Megabases
- MHC:
-
Major Histocompatibility Complex
- MY:
-
Milk yield
- NCBI:
-
National Center for Biotechnology Information
- OAR:
-
Ovis aries chromosome
- p Bonf :
-
Bonferroni-corrected genome-wide threshold (p = 4.47 × 10− 7)
- PCA:
-
Principal component analysis
- p Cand :
-
Suggestive chromosome-wide significance threshold (range p = 7.47 × 10− 6 on BTA 1 to p = 2.18 × 10− 5 on BTA 28)
- QTL:
-
Quantitative trait loci
- rFEC-FH:
-
Residuals of FEC-FH
- rFEC-GIN:
-
Residuals of FEC-GIN
- rFLC-DV:
-
Residuals of FLC-DV
- rMY:
-
Residuals of milk yield
- rSCS:
-
Residuals of somatic cell score
- SCC:
-
Somatic cell count
- SCS:
-
Somatic cell score
- SNP:
-
Single nucleotide polymorphism
References
Holzhauer M, van Schaik G, Saatkamp HW, Ploeger HW. Lungworm outbreaks in adult dairy cows: estimating economic losses and lessons to be learned. Vet Rec. 2011;196:494.
Knubben-Schweizer G, Deplazes P, Torgerson PR, Rapsch C, Meli ML, Braun U. Bovine fasciolosis in Switzerland: relevance and control. Schweiz Arch Tierheilkd. 2010;152:223–9.
Charlier J, van der Voort M, Kenyon F, Skuce P, Vercruysse J. Chasing helminths and their economic impact on farmed ruminants. Trends Parasitol. 2014;30:361–7.
Vercruysse J, Clearebout E. Treatment vs non-treatment of helminth infections in cattle: defining the threshold. Vet Parasitol. 2001;98:195–214.
Charlier J, Clearebout E, Duchateau L, Vercruysse J. A survey to determine relationships between bulk tank milk antibodies against Ostertagia ostertagi and milk production parameters. Vet Parasitol. 2005;129:67–75.
Mezo M, Gonzalez-Warleta M, Antonio Castro-Hermida J, Muino L, Ubeira FM. Association between anti-F. hepatica antibody levels in milk and production losses in dairy cows. Vet Parasitol. 2011;180:237–42.
Charlier J, Duchateau L, Clearebout E, Williams D, Vercruysse J. Associations between anti-Fasciola hepatica antibody levels in bulk-tank milk samples and production parameters in dairy herds. Prev Vet Med. 2007;78:57–66.
Charlier J, De Cat A, Forbes A, Vercruysse J. Measurement of antibodies to gastrointestinal nematodes and liver fluke in meat juice of beef cattle and associations with carcass parameters. Vet Parasitol. 2009;166:235–40.
Stear MJ, Doligalska M, Donskow-Schmelter K. Alternatives to anthelmintics for the control of nematodes in livestock. Parasitology. 2007;134:139–51.
Wall R, Strong L. Environmental consequences of treating cattle with the antiparasitic drug ivermectin. Nature. 1987;327:418–21.
Gasbarre LC. Anthelmintic reistance in cattle nematodes in the US. Vet Parasitol. 2014;204:3–11.
McMahon C, Bartley DJ, Edgar HWJ, Ellison SE, Barley JP, Malone FE, et al. Anthelmintic resistance in Northern Ireland (I): prevalence of resistance in ovine gastrointestinal nematodes, as determined through fecal egg count reduction testing. Vet Parasitol. 2013;195:122–30.
Sutherland IA, Leathwick DM. Anthelmintic resistance in nematode parasites of cattle: a global issue? Trends Parasitol. 2011;27:176–81.
Bisset SA, Morris CA, McEwan JC, Vlassoff A. Breeding sheep in New Zealand that are less reliant on anthelmintics to maintain health and productivity. N Z Vet J. 2001;49:236–46.
May K, Brügemann K, Yin T, Scheper C, Strube C, König S. Genetic line comparisons and genetic parameters for endoparasite infections and test-day milk production traits. J Dairy Sci. 2017;100:7330–44.
Oliveira MCS, Alencar MM, Chagas ACS, Giglioti R, Oliveira NH. Gastrointestinal nematode infection in beef cattle of different genetic groups in Brazil. Vet Parasitol. 2009;166:249–54.
Barlow R, Piper LR. Genetic analyses of nematode egg counts in Hereford and crossbred Hereford cattle in the subtropics of New South Wales. Livest Prod Sci. 1985;12:79–84.
Burrow HM. Variances and covariances between productive and adaptive traits and temperament in a composite breed of tropical beef cattle. Livest Prod Sci. 2001;70:213–33.
Twomey AJ, Sayers RG, Carroll RI, Byre N, O’Brien E, Doherty ML, et al. Genetic parameters for both a liver damage phenotype caused by Fasciola hepatica and antibody response to Fasciola hepatica phenotype in dairy and beef cattle. J Anim Sci. 2016;94:4109–19.
Twomey AJ, Carroll RI, Doherty ML, Byrne N, Graham DA, Sayers RG, et al. Genetic correlations between endo-parasite phenotypes and economically important traits in dairy and beef cattle. J Anim Sci. 2018;96:407–21.
Dominik S. Quantitative trait loci for internal nematode resistance in sheep: a review. Genet Sel Evol. 2005;37:38–96.
Gasbarre LC, Leighton EA, Sonstegard T. Role of the bovine immune system and genome in resistance to gastrointestinal nematodes. Vet Parasitol. 2001;98:51–64.
Liu GE, Brown T, Hebert DA, Cardone MF, Hou Y, Choudhary RK, et al. Intitial analysis of copy number variations in cattle selected for reistance or susceptibility to intestinal nematodes. Mamm Genome. 2011;22:111–21.
Sayre BL, Harris GC. Systems genetics approach reveals candidate genes for parasite resistance from quantitative trait loci studies in agricultural species. Anim Genet. 2011;43:190–8.
Benavides MV, Sonstegard TS, Kemp S, Mugambi JM, Gibson JP, Baker RL, et al. Identification of novel loci associated with gastrointestinal parasite resistance in a red Maasai x Dorper backcross population. PLoS One. 2015;10:e0122797.
Davies G, Stear MJ, Benothman M, Abuagob O, Kerr A, Mitchell S, et al. Quantitative trait loci associated with parasitic infection in Scottish blackface sheep. Heredity. 2006;96:252–8.
Coppieters W, Mes THM, Druet T, Farnir F, Tamma N, Schrooten C, et al. Mapping QTL influencing gastrointestinal nematode burden in Dutch Holstein-Friesian dairy cattle. BMC Genomics. 2009;10:96.
Kim ES, Sonstegard TS, Silva MV, Gasbarre LC, van Tassell CP. Identification of quantitative trait loci affecting gastrointestinal parasite resistance in an experimental Angus population. Anim Genet. 2014;45:117–21.
Kim ES, Sonstegard TS, Silva MV, Gasbarre LC, van Tassell CP. Genome-wide scan of gastrointestinal nematode resistance in closed Angus population selected for minimized influence of MHC. PLoS One. 2015;10:e0119380.
Hou Y, Liu GE, Bickhart DM, Matukumalli LK, Li C, Song J, et al. Genomic regions showing copy number variation associate with resistance or susceptibility to gastrointestinal nematodes in Angus cattle. Funct Integr Genomics. 2012;12:81–92.
Xu L, Hou Y, Bickhart DM, Song J, van Tassell CP, Sonstegard TS, et al. A genome-wide survey reveals a deletion polymorphism associated with resistance to gastrointestinal nematodes in Angus cattle. Funct Integr Genomics. 2014;14:333–9.
Mehmood K, Zhang H, Sabir AJ, Abbas RZ, Ijaz M, Durrani AZ, et al. A review on epidemiology, global prevalence and economical losses of fasciolosis in ruminants. Microb Pathog. 2017;109:253–62.
Piedrafita D, Spithill TW, Smith RE, Raadsma HW. Improving animal and human health through understanding liver fluke immunology. Parasite Immunol. 2010;32:572–81.
Gordon DK, Roberts LCP, Lean N, Zadoks RN, Sargison ND, Skuce PJ. Identification of the rumen fluke, Calicophoron daubneyi, in GB livestock: possible implications for liver fluke diagnosis. Vet Parasitol. 2013;195:65–71.
Mügge B, Lutz WE, Südbeck H, Zelfel S. Deutsche Holsteins: Die Geschichte einer Zucht. Stuttgart: Verlag Eugen Ulmer; 1999.
Al-Kanaan A. Heat stress response for physiological traits in dairy and dual purpose cattle populations on phenotypic and genetic scales. PhD thesis: Faculty of Organic Agriculture. Kassel: University of Kassel; 2016.
Jaeger M, Brügemann K, König S. Genetic relationships and trait comparison between and within lines of local dual purpose cattle. Belfast: 67th Annual Meeting of the European Association for Animal Production; 2016.
Bishop SC. A consideration of resistance and tolerance for ruminant nematode infections. Front Genet. 2012;3:168.
Martin P, Barkema HW, Brito LF, Narayana SG, Miglior F. Symposium review: novel strategies to genetically improve mastitis resistance in dairy cattle. J Dairy Sci. 2018;101:2724–36.
Pimentel ECG, Bauersachs S, Tietze M, Simianer H, Tetens J, Thaller G, et al. Exploration of relationships between production and fertility traits in dairy cattle via association studies of SNPs within candidate genes derived by expression profiling. Anim Genet. 2011;42:251–62.
Schöpke K, Swalve HH. Review: opportunities and challanges for small populations of dairy cattle in the era of genomics. Animal. 2016;10:1050–60.
Hayes BJ, Bowman PJ, Chamberlain AC, Verbyla K, Goddard ME. Accuracy of genomic breeding values in multi-breed dairy cattle populations. Genet Sel Evol. 2009;41:51–60.
Karoui S, Carabaño MJ, Díaz C, Legarra A. Joint genomic evaluation of French dairy cattle breeds using multi-trait models. Genet Sel Evol. 2012;44:39–49.
König S, Simianer H, Swalve HH. Genetic relationships between dairy performance under large-scale farm and family farm conditions estimated from different groups of common sires. Proc. 7th World Congr. Genet. Appl. Livest. Prod., Montpellier, France; 19-23 August 2002.
May K, Brügemann K, König S, Strube C. Patent gastrointestinal nematode infections in organically and conventionally pastured dairy cows and their impact on individual milk and fertility parameters. Vet Parasitol. 2017;245:119–27.
Ali AKA, Shook GE. An optimum transformation for somatic cell concentration in milk. J Dairy Sci. 1980;63:487–90.
Daetwyler HD, Capitan A, Pausch H, Stothard P, van Binsbergen R, Brøndum RF, et al. Whole-genome sequencing of 234 bulls facilitates mapping of monogenic and complex traits in cattle. Nat Genet. 2014;46:858–65.
Browning BL, Browning SR. Genotype Imputation with millions of reference samples. Am J Hum Genet. 2016;98:116–26.
Korkuć P, Arends D, Scheper C, May K, König S, Brockmann GA. 1-step versus 2-step imputation: a case study in German Black Pied cattle. Proc. 11th World Congr. Genet. Appl. Livest. Prod., Auckland, New Zealand; 11-16 February 2018.
Purcell S, Neale B, Todd-Brown K, Thomas L, Ferreira MA. PLINK: a tool set for whole-genome association and population-based linkage analyses. Am J Hum Genet. 2007;81:559–75.
SAS Institute Vers. 9.4. SAS Insitute, Inc., Cary, NC.
Yang J, Lee SH, Goddard ME, Visscher PM. GCTA: a tool for genome-wide complex trait analysis. Am J Hum Genet. 2011;88:76–82.
Wellcome Trust Case Control Consortium. Genome-wide association study of 14,000 cases of seven common diseases and 3,000 shared controls. Nature. 2007;447:661–78.
Li M-X, Yeung JMY, Cherny SS, Sham PC. Evaluating the effective numbers of independent tests and significant p-value thresholds in commercial genotyping arrays and public imputation reference datasets. Hum Genet. 2012;131:747–56.
Durinck S, Moreau Y, Kasprzyk A, Davis S, De Moor B, Brazma A, et al. BioMart and Bioconductor: a powerful link between biological databases and microarray data analysis. Bioinformatics. 2005;21:3439–40.
Durinck S, Spellman P, Birney E, Huber W. Mapping identifiers for the integration of genomic datasets with the R/Bioconductor package biomaRt. Nat Protoc. 2009;4:1184–91.
ENSEMBL genome browser. http://www.ensembl.org/index.html
National Center for Biotchnology Information (NCBI): Bovine genome resource. http://ncbi.nlm.nih.gov.
Huang DW, Sherman BT, Lempicki RA. Systematic and integrative analysis of large gene lists using DAVID bioinformatics resources. Nat Protoc. 2008;4:44–57.
Kanehisa M, Goto S, Sato Y, Kawashima M, Furumichi M, Tanabe M. Data, information, knowledge and principle: back to metabolism in KEGG. Nucleic Acids Res. 2014;42:199–205.
Hartwig S, Bennewitz J. Züchterische Aspekte zur Konsolidierung und Weiterentwicklung lokaler Rinderpopulationen. Züchtungskunde. 2014;86:19–24.
Biedermann G, Poppinga O, Weitemeyer I. Die genetische Struktur der Population des Schwarzbunten Niederungsrindes. Züchtungskunde. 2005;77:3–14.
McCarthy S, Horan B, Dillon P, O’Connor P, Rath M, Shalloo L. Economic comparison of divergent strains of Holstein-Friesian cows in various pasture-based production systems. J Dairy Sci. 2007;90:1493–505.
Bannerman DD, Springer HR, Paape MJ, Kauf ACW, Goff JP. Evaluation of breed-dependent differences in the innate immune responses of Holstein and Jersey cows to Staphylococcus aureus intramammary infection. J Dairy Res. 2008;75:291–301.
Naderi S. Exploring the potential of machine learning methods and selection signature analyses for the estimation of genomic breeding values, the estimation of SNP effects and the identification of possible candidate genes in dairy cattle. PhD thesis: Institute of Animal Breeding and Genetics. Gießen: Justus-Liebig-University of Gießen; 2018.
Riggio V, Matika O, Pon-Wong R, Stear MJ, Bishop SC. Genome-wide association and regional heritability mapping to identify loci underlying variation in nematode resistance and body weight in Scottish blackface lambs. Heredity. 2013;110:420–9.
Sallé G, Jacquiet P, Gruner L, Cortet J, Sauvé C, Prévot F. A genome scan for QTL affecting resistance to Haemonchus contortus in sheep. J Anim Sci. 2012;90:4690–750.
Bellet C, Green MJ, Vickers M, Forbes A, Berrx E, Kaler J. Ostertagia spp., rumen fluke and liver fluke single- and poly-infections in cattle: an abattoir study of prevalence and production impacts in England and Wales. Prev Vet Med. 2016;132:98–106.
Borgsteede FHM, Tibben J, Cornelissen JBWJ, Agneessens J, Gaasenbeek CPH. Nematode parasites of adult dairy cattle in the Netherlands. Vet Parasitol. 2000;89:287–96.
Bishop SC, Doeschl-Wilson AB, Woolliams JA. Uses and implications of field disease data for livestock genomic and genetics studies. Front Genet. 2012;3:114.
Bishop SC, Woolliams JA. Genomics and disease resistance in livestock. Livest Sci. 2014;166:190–8.
Bishop SC, Woolliams JA. On the genetic interpretation of disease data. PLoS One. 2010;5:e8940.
Mapholi NO, Maiwashe A, Matika O, Riggio V, Bishop SC, MacNeil MD, et al. Genome-wide association study of tick resistance in south African Nguni cattle. Ticks Tick Borne Dis. 2016;7:487–97.
Escobedo G, Roberts CW, Carrero JC, Morales-Montor J. Parasite regulation by host hormones: an old mechanism of host exploitation? Trends Parasitol. 2005;21:588–93.
Romano MC, Jiménez P, Miranda-Brito C, Valdez RA. Parasites and steroid hormones: corticosteroid and sex steroid synthesis, their role in the parasite physiology and development. Front Neurosci. 2015;9:224.
Escobedo EG. Molecular mechanism involved in the differential effects of sex steroids on the reproduction and infectivity of Taenia crassiceps. J Parasitol. 2004;90:1235–44.
Morrison DD, Vandee Waa E, Bennett JL. Effects of steroids and steroid synthesis inhibitors on fecundity of Schistosoma mansoni in vitro. J Chem Ecol. 1985;12:1901–8.
Fleming MW. Ascaris suum: Role of ecdysteroids in molting. Exp Parasitol. 1985;60:207–10.
Fukao T, Koyasu S. PI3K and negative regulation of TLR signaling. Trends Immunol. 2003;24:358–63.
Koyasu S. The role of PI3K in immune cells. Nat Immunol. 2003;4:313–9.
Li S, Gong P, Tai L, Li X, Wang X, Zhao C, et al. Extracellular vesicles secreted by Neospora caninum are recognized by toll-like receptor 2 and modulate host cell innate immunity through the MAPK signaling pathway. Front Immunol. 2018;9:1633.
Maizels RM, Yazdanbakhsh M. Immune regulation by helminth parasites: cellular and molecular mechanisms. Nat Rev Immunol. 2003;3:733–43.
Allen JE, Maizels RM. Diversity and dialogue in immunity to helminths. Nat Rev Immunol. 2011;11:375–88.
Hewitson JP, Grainger JR, Maizels RM. Helminth immunoregulation: the role of parasite secreted proteins in modulating host immunity. Mol Biochem Parasitol. 2009;167:1–11.
Foster N, Elsheikka HM. The immune response to parasitic helminths of veterinary importance and its potential manipulation for future vaccine control strategies. Parasitol Res. 2012;110:1587–99.
Bricarello PA, Zaros LG, Coutinho LL, Rocha RA, Silva MB, Kooyman FN, et al. Immunological responses and cytokine gene expression analysis to Cooperia punctata infections in resistant and susceptible Nelore cattle. Vet Parasitol. 2008;155:95–103.
Zaros LG, Bricarello PA, Amarante AF, Rocha RA, Kooyman FN, De Vries E, et al. Cytokine gene expression in response to Haemonchus placei infections in Nelore cattle. Vet Parasitol. 2010;171:68–73.
Arujo RN, Padilha T, Zarlenga D, Sonstegard T, Connor EE, Van Tassel C, et al. Use of a candidate gene array to delineate gene expression patterns in cattle selected for resistance or susceptibility to intestinal nematodes. Vet Parasitol. 2009;162:106–15.
Flynn RJ, Mannion C, Golden O, Hacariz O, Mulcahy G. Experimental Fasciola hepatica infection alters responses to tests used for diagnosis of bovine tuberculosis. Infect Immun. 2007;75:1373–81.
Gasbarre LC, Leighton EA, Davies CJ. Influence of host genetics upon antibody responses against gastrointestinal nematode infections in cattle. Vet Parasitol. 1993;46:81–91.
Morris CA, Green RS, Cullen NG, Hickey SM. Genetic and phenotypic relationships among faecal egg count, anti-nematode antibody level and live weight in Angus cattle. Anim Sci. 2003;76:167–74.
Bishop SC, Jackson F, Coop RL, Stear MJ. Genetic parameters for resistance to nematode infections in Texel lambs and their utility in breeding programmes. Anim Sci. 2004;78:185–94.
Woolaston RR, Eady SJ. Australian research on genetic resistance to nematode parasites. In: Gray DG, Woolaston RR, Eaton B, editors. Breeding for resistance to infectious diseases in small ruminants. Canberra: Australian Centre of International Agricultural Research; 1995. p. 1–12.
König S. Genetic background of resistance, tolerance and resilience traits in dairy cattle. 68th Annual Meeting of the European Association for Animal Production, Tallinn, Estonia; 2017.
Schukken YH, Grommers FJ, Van de Geer D, Erb HN, Brand A. Risk factors for clinical mastitis in herds with a low bulk milk somatic cell count. 2. Risk factors for Escherichia coli and Staphylococcus aureus. J Dairy Sci. 1991;74:826–32.
Howell A, Baylis M, Smith R, Pinchbeck G, Williams D. Epidemiology and impact of Fasciola hepatica exposure in high-yielding dairy herds. Prev Vet Med. 2015;121:41–8.
Turner LB, Harrison BE, Bunch RJ, Porto Neto LR, Li Y, Barendse W. A genome-wide association study of tick burden and milk composition in cattle. Anim Prod Sci. 2010;50:235–45.
Acknowledgements
The authors wish to thank the farmers for their cooperation in this study. We gratefully thank the H. Wilhelm Schaumann foundation for the provision of a PhD grant to KM.
Funding
The authors acknowledge the financial support for this project provided by transnational funding bodies, being partners of the FP7 ERA-net project, CORE Organic Plus, and the cofund from the European Commission.
Availability of data and materials
All the data supporting the results of this article are included within the article. The raw phenotypic and genotypic data is stored in the databases of the IT-company vit Verden and in the cluster SKYLLA from Giessen University (https://www.uni-giessen.de/fbz/svc/hrz/svc/server/hpc). All data can be provided by request.
Author information
Authors and Affiliations
Contributions
SK Initiation of the genetic research design and coordination of the study. ChS Initiation and organization of the laboratory analyses. CaS Coordination of selective genotyping and implementation of data analyses. KM Collection and assessment of parasitological data and collection of blood samples for genotyping and data analyses. KB and TY Support with statistical analyses. PK and GB Provision of cow genotypes and genome imputations. KM and CaS drafted the manuscript. KM and SK wrote the final paper. All authors participated in the data interpretation and read and approved the final manuscript.
Corresponding author
Ethics declarations
Ethics approval and consent to participate
Cow phenotypes reflect the traits from official milk recording schemes plus the collection of fecal samples. Farmers agreed to participate in this study, and the respective breeding organizations and milk recording organizations provided the data (i.e. the traits from official milk recording schemes, genomic data and pedigree data). The reference sequence data for imputation were obtained from the 1000 bull genome project [47].
Consent for publication
Not applicable.
Competing interests
The authors declare that they have no competing interests.
Publisher’s Note
Springer Nature remains neutral with regard to jurisdictional claims in published maps and institutional affiliations.
Additional files
Additional file 1:
Table S1. Chromosome-wide significance thresholds for the endoparasite traits rFEC-GIN, rFEC-FH and rFLC-DV including the number of SNP markers after quality control (QC) and the effective number of independent SNP markers based on linkage disequilibrium (LD). (DOCX 16 kb)
Additional file 2:
Figure S1. Principal component analysis of the 148 DSN cattle for (A) the mean values of FEC-GIN, (B) the mean values of FEC-FH, (C) the mean values of FLC-DV and (D) the three different farms. Plot of the first two principal components (PC1 and PC2) of each individual cow based on SNP information to evaluate the extent of the population structure. (PDF 31 kb)
Additional file 3:
Table S2. List of all SNP markers associated with the residuals of gastrointestinal nematodes (rFEC-GIN) identified in Black Pied dairy cattle by genome-wide analysis. (DOCX 19 kb)
Additional file 4:
Table S3. List of all SNP markers associated with the residuals of Fasciola hepatica (rFEC-FH) identified in Black Pied dairy cattle by genome-wide analysis. (DOCX 15 kb)
Additional file 5:
Table S4. List of all SNP markers associated with the residuals of Dictyocaulus viviparus (rFLC-DV) identified in Black Pied dairy cattle by genome-wide analysis. (DOCX 38 kb)
Rights and permissions
Open Access This article is distributed under the terms of the Creative Commons Attribution 4.0 International License (http://creativecommons.org/licenses/by/4.0/), which permits unrestricted use, distribution, and reproduction in any medium, provided you give appropriate credit to the original author(s) and the source, provide a link to the Creative Commons license, and indicate if changes were made. The Creative Commons Public Domain Dedication waiver (http://creativecommons.org/publicdomain/zero/1.0/) applies to the data made available in this article, unless otherwise stated.
About this article
Cite this article
May, K., Scheper, C., Brügemann, K. et al. Genome-wide associations and functional gene analyses for endoparasite resistance in an endangered population of native German Black Pied cattle. BMC Genomics 20, 277 (2019). https://doi.org/10.1186/s12864-019-5659-4
Received:
Accepted:
Published:
DOI: https://doi.org/10.1186/s12864-019-5659-4