Abstract
Evapotranspiration (ETo) is an important component of the hydrological cycle and reliable estimates of ETo are essential for assessing crop water requirements and irrigation management. Direct measurement of evapotranspiration is both costly and involves complex and intricate procedures. Hence, empirical models are commonly utilized to estimate ETo using accessible meteorological data. Given that empirical methods operate on various assumptions, it is essential to assess their performance to pinpoint the most suitable methods for ETo calculation based on the availability of input data and the specific climatic conditions of a region. This study aims to evaluate different empirical methods of ETo in the tropical highland Udhagamandalam region of Tamil Nadu, India, utilizing sixty years of meteorological data from 1960–2020. In this study, 8 temperature-based and 10 radiation-based empirical models are evaluated against ETo estimates derived from pan evaporation observation and the FAO Penman–Monteith method (FAO-PM), respectively. Statistical error metrics indicate that both temperature and radiation-based models perform better for the Udhagamandalam region. However, radiation-based models performed better than the temperature based models. This is possibly due to the high humidity of the study region throughout the year. The results suggest that simple temperature and radiation-based models using minimum meteorological information are adequate to estimate ETo and thus find potential application in agricultural water practices, hydrological processes, and irrigation management.
Similar content being viewed by others
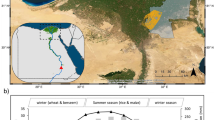
Introduction
Evapotranspiration is an important component of the hydrologic cycle in the land–atmosphere system because it accounts for a sizable portion of the moisture lost from a plant canopy. Evapotranspiration (ET) plays a vital role in both the global hydrological cycle and regional water budgets. It encompasses the combined processes of surface water evaporation, bare soil evaporation, and plant transpiration, representing the movement of water from the Earth's surface and soil to the atmosphere as vapor. This dynamic process influences the distribution and availability of water resources on both at a local and global scale and this has gained utmost importance due to the change in climate1,2. Hence calculating reference crop evapotranspiration is crucial for determining crop water needs and for scheduling irrigation3. Reference evapotranspiration (ETo) quantifies the maximum amount of moisture that is lost to the atmosphere through the combined process of evaporation and plant transpiration for a given atmospheric condition. The goal of determining evapotranspiration is to exclude crop-specific variations from the evapotranspiration process by assuming constant crop conditions. However, the reference crop is not properly defined in this specification, which may pose a challenge in the total elimination of crop components. Several methods are developed for estimation of ETo using direct and indirect approaches. Among these methods, the lysimeter technique is often regarded as the most reliable for estimating crop evapotranspiration (ETc), providing detailed insights into soil water retention and excess irrigation percolation simultaneously. However, the significant expenses and the labor-intensive nature of lysimeter installation and expensive maintenance hinder their widespread adoption in agricultural systems. This limitation restricts the use of lysimeter in the areas within a research site where measurements can be conducted, thereby constraining the spatial coverage of evapotranspiration assessments4. The other methods for estimation of evaporation include pan evaporimeter, water budgeting, empirical models, etc. Among these, the different empirical methods of ETo estimation can be grouped into empirical formulations based on radiation, temperature, mass transfer, and combination theory types. Over time, a variety of empirical methods have been developed to calculate evapotranspiration, but none of them can be deemed flawless due to the wide variations in climatic conditions in different parts of the world. To overcome the ambiguity, the American Society of Civil Engineers (ASCE) and Food and Agriculture Organization (FAO) jointly formulated the Penman–Monteith equation for determining Reference Evapotranspiration by combining all the responsible meteorological parameters /processes5.
Although several models or equations have been reported in the literature for the estimation of ETo, there is uncertainty about which equations are appropriate for a specific climatic situation, and each model needs exact local calibration6. A variety of empirical and semi-empirical approaches have been used in the Indian context for estimating reference evapotranspiration (ETo) based on their performance in other areas of the world7,8.
Muniandy et al. compared 26 empirical models and pan evaporation calculation techniques at the Modern Agriculture Centre in Kluang, Johor, Peninsular Malaysia9. They reported that, when compared to other models, the mass transfer-based Penman model produced the best ETo results. Similar to this, Bourletsikas et al. evaluated ETo using 24 different empirical models10. They discovered that to improve performance in Greece, mass transfer-based models must be calibrated. In addition, 14 distinct empirical ETo equations were investigated by Gao et al. in various climate zones of China. It has been shown that in dry, semi-dry, and humid conditions, respectively, the Priestley–Taylor, Hargreaves, and Makkink approaches performed best11. In northwest China, Celestin et al. compared the 32 empirical ETo equations with the FAO56-PM using temperature, solar radiation, and mass transfer data12. They found that the Mahringer and World Meteorological Organization (WMO) methodologies generated the best results. Djaman et al. reported that the Abtew ETo equation, which is based on solar radiation and a maximum temperature, performed best when compared to the FAO-PM model throughout the three climate zones of Mali13. According to Zakeri et al. the Jensen–Haise approach was the most effective empirical equation in the dry Semnan province14. The climatic conditions in India are highly diverse over different zones and Mandal et al. suggested that the Hargreaves method provides better ETo estimates for different stations in India15.
The applicability of any evapotranspiration model is limited by the availability of input data. Therefore, there is a strong need to identify and understand the most suited empirical models for specific areas to obtain ETo estimates from limited data sets. Various studies attempted to make an approximation to the ETo for different climatic conditions using a limited amount of information14. The main objective of this study is to evaluate various empirical approaches (18 models) to identify a suitable model which best fits the climatic conditions of Udhagamandalam in the Nilgiri district of Tamil Nadu by comparing all the 18 empirical models against ETo estimates derived from pan evaporation observation and the FAO Penman–Monteith method (FAO-PM), respectively.
Materials and methods
Study area
The study area Udhagamandalam is located on Western Ghats between 11°24′36″ N latitude and 76°41′15″ E longitude with an average altitude of 2218 m in the Nilgiri district of Tamil Nadu, India (Fig. 1). Udhagamandalam has a cold climatic topography with a tropical highland climate. Despite located in the tropics, Udhagamandalam experiences pleasant weather year-round, in contrast to most of South India. On the other hand, the nights in January and February are monotonously cold17. The average monthly weather parameters over 60 years (1960–2020) are represented in Table 1. The average maximum temperature varies from 17.6 °C in July to 22.1 °C in April, whereas the average minimum temperature ranges from 7.3 °C in January to 12.6 °C in May. The average annual rainfall is 1250 mm and a major amount (74%) of rainfall occurs during the southwest monsoon season (June to September). The data of meteorological parameters like maximum and minimum temperature, rainfall, wind speed, relative humidity, pan evaporation and sunshine hours were collected from the meteorological observatory at the Indian Institute of Soil and Water Conservation, Udhagamandalam. The average daily temperature is obtained by summing the maximum and minimum temperatures during a 24-h period and dividing them by two.
Map of study area. (Figure created by the 3rd author—Using Administrative Boundary of India and its districts using ARC GIS Software Version-10.1-https://www.arcgis.com).
The study considered 18 empirical models based on 2 categories, i.e., Temperature based and Radiation based. The pan observed reference evapotranspiration which was obtained by multiplying pan evaporation with the pan coefficient value and FAO-PM based reference evapotranspiration are considered as base models for comparison.
where, ETo is the reference crop evapotranspiration (mm/day), Epan is the measured class A pan evaporation (mm/day) and Kpan is the pan coefficient.
Various studies have reported a high correlation between Epan and ETo when evaporation pans are maintained properly18,19. Different equations for estimating Kpan values have been proposed by various scholars in recent decades20,21,22,23,24,25. In this study, the Allen and Pruitt26 method was used to calculate Kpan value and selection of the Allen and Pruitt method for calculating the Kpan value is justified based on its empirical validation by few of the earlier authors20,21,22,23,24,25, simplicity, adaptability, consideration of local conditions, and its accuracy. These factors make it a reliable and widely accepted approach for estimating pan coefficients and adjusting reference evapotranspiration to local conditions using pan evaporation data.
Empirical methods of ETo calculation
Several ways are available to measured evapotranspiration using direct and indirect methods. Evapotranspiration can be measured in field condition directly using a weighing lysimeter, pan evaporimeter and water budgeting technique. Even though these methods give accurate data, due to their high cost of installation and maintenance, indirect methods are preferred in most of the conditions. The empirical methods are based on observed meteorological parameters and involve statistical fit coefficients and are generally adequate for a specific climatic or region condition. However, because these methodologies and models were developed for specific climatic zones and have various input data requirements and assumptions, they yield different values26. Previous research on numerous scales also revealed that alternative methodologies might produce outcomes that are noticeably different which makes it difficult to choose the right procedure for the intended application27.
In this study, a total of 18 empirical models are selected which are broadly classified as temperature-based and radiation-based models (Table 2). The temperature-based methods consider maximum, minimum and mean temperatures and relative humidity as major input factors. On the other hand, the radiation-based models consider temperature, solar radiation and net radiation as input parameters. FAO56-Penman–Monteith method is the universally accepted method for the calculation of evapotranspiration over various geographical regions as it is physically based and integrates physiological characteristics and aerodynamic variables45,46. It is a combination method based on temperature, radiation, relative humidity, wind speed, and saturation vapor pressure values. The equation is as follows.
Where ETo = grass reference evaporation (mm day−1), = slope of saturation vapor pressure curve (kPa C−1), Rn = net radiation (MJ m−2 day−1), G = soil heat flux density (MJ m−2 day−1), which was taken as zero for daily estimates, T = daily mean air temperature (C) at 2 m, U2 = mean wind speed at 2 m (m s−1), es = saturation vapor pressure (kPa), ea = actual vapor pressure (kPa) and = psychometric constant (0.0677 kPa C−1). Co = conversion parameter (= 0.0023).
Statistical evaluation of models
The empirical model performance can be evaluated using several statistical error metrics. But there is not a single statistic that encompasses all relevant factors. For this reason, it is helpful to take into account a variety of performance data and to comprehend the kind of knowledge or insight they may offer. The open-air package of R software47 was used to assess the performance of models. The open air is an R package chiefly developed for the analysis of air pollution measurement data but it is versatile and widely used in the atmospheric and climate sciences47. The ranking of model was done with modstat function by considering statistical performance indicators like Fraction of predictions within a factor or two (FAC2), Mean Bias (MB), Mean Gross Error (MGE), Normalized Mean Bias (NMB), Normalized Mean Gross Error (NMGE), Root Mean Square Error (RMSE), correlation coefficient r and Index of Agreement (IOA). The details of the statistical error metrics are given below. In the following definitions, Oi represents the ith observed value and Mi represents the ith modelled value for a total of n observations.
Fraction of predictions within a Factor or two, (FAC2)
The fraction of modelled values within a factor of two of the observed values are the fraction of model predictions that satisfy:
Mean bias (MB)
The mean bias gives a clear indicator of whether model estimates/predicted values are on average over or underestimated. MB expressed in the same units as the variables under consideration47.
Mean gross error (MGE)
This gives mean gross error or simply the mean error without reference to overestimation or underestimation. MGE is expressed in the same units as the input values47.
Normalized mean bias (NMB)
Whether the modeled mean underestimates or overestimates the observed mean is indicated by the sign of NMB. Additionally, the NMB magnitude reveals the degree of under or overestimation48.
Normalized mean gross error (NMGE)
The normalized mean gross error further ignores whether a prediction is an over or underestimated.
Root mean square error (RMSE)
This give the measure of how close modelled values are to predicted values. It ranges from 0 to ∞ and lower the values better the performance
Correlation coefficient (r)
Correlation is a measure of the degree of association between two variables. The Pearson's correlation49 r is given by
Index of agreement (IOA)
The Index of Agreement, IOA compares model estimates or predictions (Pi; I = 1, 2,…, n) with matched pairs of reliable observations (Oi; I = 1, 2,…, n). It spans between −1 and + 1 with values approaching + 1 representing better model performance50.
Taylor diagram
One of the more effective tools for assessing model performance is the Taylor Diagram. The graphic demonstrates the simultaneous variation in three complementing model performance statistics. The correlation coefficient R, standard deviation (sigma), and the (centred) root-mean-square error are these statistics. Due to their relationships with one another, which may be illustrated by the Law of Cosines, these three statistics can be plotted on a single (2D) graph51.
Results and discussion
In the study area, the maximum ETo (3.4 mm day−1) was observed during March and minimum ETo (1.7 mm day−1) was recorded in July. Among the four seasons, maximum ETo was recorded during summer (3.1 mm day−1) and minimum during monsoon (1.9 mm day−1) as per the water vapour pressure variation in different seasons. These results indicate high water requirements during summer followed by winter and monsoon seasons in the study area. The high-water requirements in summer and winter are due to high air temperatures and low atmospheric humidity in summer and stronger winds in winter which increase the ETo by increased evaporation rate and strong aerodynamic effects respectively. An interesting observation is that the ETo during the monsoon period (June–October) is relatively low compared to other seasons and this period has high humidity (70–90%) due to the study region experiencing strong moist laden southwesterly monsoon winds in this season. The relation between evapotranspiration and meteorological parameters like maximum temperature, minimum temperature, relative humidity, wind speed and solar radiation were plotted in Figs. 2, 3, 4, 5 and 6.
The relationship between ETo and meteorological parameters is fundamental in understanding various aspects of environmental processes, including water availability, ecosystem functioning, and climate dynamics. Mean temperature typically has a direct influence on ETo rates as it enhances the evaporation. As temperatures rise, the energy available for driving the process of evaporation increases. This means that higher temperatures generally lead to higher rates of evapotranspiration. This relationship is evident in regions experiencing warmer climates, where ET tends to be more pronounced, and these Figs. 2, 3, 4, 5 and 6 also confirm the same. Figure 3 shows ETo in different months are inversely correlated with minimum temperatures which is again attributable to temperature effect. It is observed that among the parameters maximum temperature and solar radiation showed a positive correlation with ETo, and the other parameters like minimum temperature, relative humidity showed a negative correlation with ETo. The correlation values (r)obtained for maximum temperature and solar radiation are 0.89 and 0.93, and in the case of minimum temperature and relative humidity are −0.73 and −0.92, respectively. Regression analysis with these parameters confirmed the same. The moisture abundance in monsoon months also can be seen from relatively high RH in June–October in the study region (Fig. 4). In the case of wind speed, it showed negative relation with evapotranspiration throughout the year except during monsoon, when it showed the increase in wind led to decrease (Fig. 5) in evapotranspiration. The decrease of ETo due to stronger winds during monsoon period is due to increased moisture transport by the stronger monsoon winds (Fig. 5) to the study region which reduces the rate of ETo from plants.
The reference evapotranspiration ETo was calculated using 18 empirical models, which were further grouped into two categories, i.e., Temperature based and radiation-based methods and discussed below.
Comparison of pan observed and FAO-PM based reference evapotranspiration (ETo)
The study compared ETo estimates derived from pan evaporation observation and the FAO Penman–Monteith method (FAO-PM), respectively, which is the internationally accepted method of ETo calculation. The FAO-PM-based ETo ranges from 2.4 to 4.1 mm day−1 which is close to the pan observed ETo value, which is 1.7 to 3.4 mm day−1 (Table 3, Fig. 7). The FAO-PM method slightly overestimated the pan observed values of ETo.
Comparison of pan observed and empirical model-based reference evapotranspiration (ETo)
The temperature-based ETo is presented in Table 3 and depicted in a box plot (Fig. 8), along with ETo obtained from pan evaporation observation evapotranspiration. Among the eight temperature-based models assessed, the Papadakis model exhibited an underestimation of ETo ranging from 0.5 to 2 mm day−1, while the Trajkovic method showed an overestimation of ETo ranging from 5.7 to 8.1 mm day−1. This discrepancy in the Papadakis model can be attributed to its reliance solely on water vapor pressure deficit, while the overestimation in the Trajkovic method results from its consideration of both radiation and temperature without factoring in the role of atmospheric humidity. The vapor pressure deficit-based relation of ETo is effective primarily under dry conditions but may prove inadequate under humid conditions.
The Ivanov model reported the widest range of evapotranspiration with the lowest value as 1.1 mm day−1 and highest as 4.1 mm day−1, whereas Blaney Criddle model has the lowest ETo range from 3.7 to 4.5 mm day−1. The Berti model which is based on both net radiation and maximum, minimum and mean temperatures gave an ETo value (2.3 to 3.3 mm day−1) close to the pan observed value. The Schendel (2.7 to 4.3 mm day−1), Hargreaves–Samani (2.7 to 3.9 mm day−1) and Kharrufa (2.6 to 3.8 mm day−1) methods also gave a similar range of ETo.
In the case of radiation-based ETo methods (Table 3, Fig. 9), the Abtew method showed the lowest ETo values (1.8 to 3.5 mm day−1) which were close to observed values (1.7 to 3.4 mm day−1). Tabari (2.3 to 3.5 mm day−1), Caprio (2.3 to 4.1 mm day−1) and Turc (2.4 to 3.9 mm day−1) methods also showed a similar range of ETo. The range of ETo was nearly similar in Irmark (Rs) (2.6 to 3.9 mm day−1), Jensen–Haise (2.5 to 4.4 mm day−1) and Jones (2.6 to 4.4 mm day−1) methods of calculation. The highest range of ETo was observed in Irmark (Rn) (3.4 to 4.5 mm day−1), Makkink (3.5 to 5.8 mm day−1) and Priestley Taylor (4.8 to 6.7 mm day−1) methods.
Comparison of FAO-PM and empirical models based reference evapotranspiration (ETo)
The temperature-based ETo calculation was compared with the FAO-PM method and plotted in Fig. 10. It was observed that Papadakis method underestimated the FAO-PM values, whereas Trajkovic and Blaney Criddle's methods overestimated the same. The comparison of radiation-based models and FAO-PM was depicted in Fig. 11. The Makkink and Priestley–Taylor models overestimated the ETo, whereas all other models were close to the FAO-PM values.
Statistical performance of FAO-PM model with pan observed ETo
The comparison of FAO-PM based ETo with with ETo obtained from pan evaporation observation, showed good performance in terms of all the statistical indices considered (Table 4). The high values of FAC2 (1) and r (0.95), and low values of MB (0.66), MGE (0.66), NMB (0.27) and NMGE (0.27) indicate good performance of the FAO-PM model. Study of Vishwakarma et al.52 compared ETo obtained from pan evaporation observation and with ETo from FAO PM method for humid and subtropical conditions of Ludhiana district of Punjab, India and observed better performance of the FAO-PM method with good RMSE value of 1.13 and r value of 0.96. The radiation-based models also consider temperature variables compared to the temperature-based models as ETo depends on Rs (available energy for evaporation) as well as the temperature of the air.
Statistical performance of empirical models with Pan observed ETo
The statistical analysis of temperature-based models is presented in Table 4. It is observed that all the models gave FAC2 value of 1 except Papadakis (0.42), Blaney Criddle (0.67) and Trajkovic (0.0), which indicate better performance of most of the models. Overall, the Berti model was found to be the best performing model with MB = 0.22, MGE = 0.29, NMB = 0.09, NMGE = 0.12, RMSE = 0.35, r = 0.9 and IOA = 0.71. The Ivanov model also gave better results with FAC2, MB, MGE, NMB, NMGE, RMSE, r and IOA values respectively. The poor performed models were Blaney Criddle and Trajkovic, where Blaney Criddle gave FAC2 value of 0.67, MB of 1.69, MGE of 1.69, NMB of 0.69, NMGE of 0.69, RMSE of 1.8, r value of −0.12 and IOA value of −0.41. The negative ‘r’ value in Blaney Criddle method indicates a negative linear relationship with observed values. In the case of the Trajkovic method the FAC2, MB, MGE, NMB, NMGE, RMSE, r and IOA values are 0.00, 4.17, 4.17, 1.7, 1.7, 4.19, 0.85 and −0.76 respectively. The negative values of MB and NMB IN Ivanov (MB = −0.09, NMB = −0.04) and Papadakis (MB = −1.36, NMB = −0.55) indicate underestimation of ETo values in these methods. The Taylor diagram indicating the performance of the models is presented in the Fig. 12. The relative performance of different tested models is as follows Beri > Ivanov > Hargreves > Kharrufa > Schendel > Papadakis > Blaney Criddle > Trajkovic.
In case of radiation-based models (Table 4), The Abtew and Tabari methods performed better with respect to pan observed ETo. In Abtew method gave FAC2 = 1, MB = 0.10, MGE = 0.12, NMB = 0.04, NMGE = 0.05, RMSE = 0.16, r = 0.97 and IOA = 0.88 values, which displays better performance of the model. For the Tabari model also the statistical performance was better with FAC2 = 1, MB = 0.42, MGE = 0.42, NMB = 0.17, NMGE = 0.17, RMSE = 0.48, r = 0.93 and IOA = 0.58 values. Even though the Makkink method yield high value of r (0.98), the other statistical indicators like FAC2 (0.67), MB (2.12), MGE (2.12) NMB (0.86), NMGE (0.86), RMSE (2.13) and IOA (−0.53) gave poor values, resulted in the worst performance of the model. The Priestley Taylor method gave the highest value of MB (3.10) and NMB (1.26) indicating highest over estimation. The overall performance of the model was worst with poor values of FAC2 (0.25), MGE (3.10), NMGE (1.26), RMSE (3.12), r (0.85) and IOA (−0.68). The performance of the models as given by the Taylor diagram is shown in Fig. 13. Among all the radiation-based models, Abtew performed best, followed by Jones, Tabari, Caprio, Turc, Irmark (Rs), Jensen–Haise, Irmark (Rn), Makkink and Priestley Taylor.
Statistical performance of empirical models with FAO-PM ETo
The statistical performance of temperature-based models with respect to the FAO-PM method differs slightly from what was observed with respect to pan observed ETo (Table 5). The Hargreaves model recorded best performance with FAC2 = 1, MB = 0.07, MGE = 0.13, NMB = 0.02, RMSE = 0.17, r = 0.97 and IOA = 0.87. The superior performance of the Hargreaves–Samani model was reported by Bogawski and Bednorz53 under the central European condition. They reported that when only temperature or pan evaporation data were available, the calibrated Hargreaves–Samani method provided the most accurate results (RE = 0.275). The better performance of Hargreaves ETo model was also reported by Mandal et al.15 for different stations in India. The Schendel method also produced similar results with FAC2, MB, MGE, NMB, NMGE, RMSE, r and IOA values as 1, 0.13, 0.15, 0.04, 0.05, 0.18, 0.98 and 0.85 respectively. The Trajkovic (FAC2 = 0.08, MB = 3.50, MGE = 3.5, NMB = 1.12, NMGE = 1.12, RMSE = 3.52, r = 0.95 and IOA = −0.72) and Papadakis (FAC2 = 0.00, MB = −2.02, MGE = 2.02, NMB = −0.65, NMGE = 0.65, RMSE = 2.04, r = 0.89 and IOA = −0.52) performed worst among the models. The negative MB and NMB values of Berti, Ivanov and Papadakis models indicates the underestimation of ETo in these models, when compared to FAO-PM method. The overall performance of temperature-based models are in the order of Hargreaves–Samani > Schendel > Berti > Kharrufa > Ivanov > Blaney- Criddle > Papadakis > Trajkovic. The performance of the models is depicted in Taylor diagram Fig. 14.
In the case of radiation based models the Turc method yielded better performance with least bias and error values (MB = −0.02, NMB = −0.01, MGE = 0.06, NMGE = 0.02, RMSE = 0.07) (Table 4). Highest values of FAC2 (1), r (1.00) and IOA (0.94) are also observed in this model. According to Tabari54, the Turc model produced the best ETo values for the cold humid climatic condition of Iran. The model was first created for humid environments, which is most likely why it performs best in humid climates. Additionally, Trajkovic and Kolakovic55 discovered that this model may deliver accurate ETo estimations in humid climates. The second-best method was found to be Caprio with FAC2, MB, MGE, NMB, NMGE, RMSE, r and IOA values as 1, −0.06, 0.11, −0.02, 0.07, 1.00 and 0.94 respectively. The negative values of MB and NMB in Turc, Caprio, Tabari and Abtew are denoting the under estimation of ETo values in these models. The poor performance was observed in Priestley–Taylor (MB = 2.43, MGE = 2.43, NMB = 0.78, NMGE = 0.78, RMSE = 2.44, r = 0.96, IOA = −0.60) and Makkink (MB = 1.45, MGE = 1.45, NMB = 0.47, NMGE = 0.47, RMSE = 1.47, r = 0.97, IOA = −0.34) model. Tabari reported the worst performance of the Priestley Taylor model in the most humid regions of Iran16 (Table 5). From the Taylor diagram (Fig. 15) the performance of different radiation-based models is Turc, followed by Caprio, Irmark (Rs), Jensen–Haise, Tabari, Jones, Abtew, Irmark (Rn), Makkink and Priestley–Taylor.
The comparison study of various models against pan observations and the FAO PM methods across all seasons, showed that radiation-based models exhibited superior performance over temperature-based ones. This superiority can be attributed to the significant influence of Rs (solar radiation) in driving ETo in tropical humid climates41,56. Rs plays a pivotal role in redistributing surface energy into various forms (latent, sensible, and ground heat fluxes), thereby facilitating moisture transport through evaporation/evapotranspiration from vegetated surfaces. The reliance of radiation-based models on net radiation to estimate ETo values35,40,57 underscores the crucial role of net radiation in governing surface energy balance. Consequently, radiation-based models offer reliable estimates of ETo values. However, it is noteworthy that both radiation and temperature-based empirical models demonstrated suitability for the study region, which experiences high altitudes and maintains moderate to high humidity levels throughout the year. The effectiveness of both types of models in this region can be attributed to the limited influence of atmospheric moisture in regulating ETo56,57,58.
Conclusion
The present study evaluated the performance of different empirical ETo models for the temperate hilly region of Udhagamandalam in South India based on long-term meteorological data. From a detailed comparison of different empirical ETo models with pan observed and FAO PM methods considering the entire year covering all the seasons, it is found that the radiation-based models performed better than the temperature based models. Overall, the radiation-based models outperformed the other empirical models due to the more significant role of solar radiation (Rs) in driving ETo in tropical humid climates. This shows that Rs is the controlling factor in the partitioning of surface energy to different forms (latent, sensible, and ground heat fluxes) which leads to transport of moisture through evaporation/evapotranspiration from vegetated surface. These results confirm that the radiation-based models can reliably estimate ETo values possibly due to net radiation largely determining the evaporation requirements as it effectively controls the surface energy balance. However, in the case of non-availability of radiation data, temperature based empirical models can also be used for the study region. The findings from the study suggests that simple temperature based empirical models can be used for estimation of ETo and that will help in devising suitable irrigation management decisions such as crop water requirement, irrigation scheduling and related agricultural water management practices.
Data availability
All data generated or analysed during this study are included in this published article. The datasets (Raw data) used and/or analyzed during the current study available from the corresponding author on request.
References
Abeysiriwardana, H. D., Muttil, N. & Rathnayake, U. A comparative study of potential evapotranspiration estimation by three methods with FAO Penman–Monteith method across Sri Lanka. Hydrology 9, 206. https://doi.org/10.3390/hydrology9110206 (2022).
Senatilleke, U., Abeysiriwardana, H., Makubura, R. K., Anwar, F. & Rathnayake, U. Estimation of potential evapotranspiration across Sri Lanka using a distributed dual-source evapotranspiration model under data scarcity. Adv. Meteorol. 2022, 1–14. https://doi.org/10.1155/2022/6819539 (2022).
Rasul, G. Water requirement of wheat crop in Pakistan. J. Energy Appl. Sci. 12, 1–9 (1993).
Ghiat, I., Mackey, H. R. & Al-Ansari, T. A review of evapotranspiration measurement models, techniques and methods for open and closed agricultural field applications. Water 13, 2523 (2021).
Poyen, E. F. B., Ghosh, A. K. & Palash Kundu, P. Review on different evapotranspiration empirical equations. IJAEMS. 2, 239382 (2016).
Dehghanisanij, H., Yamamoto, T. & Rasiah, V. Assessment of evapotranspiration estimation models for use in semi-arid environments. Agric. Water Manag. 64, 91–106 (2004).
Kumar, M., Raghuwanshi, N. S., Singh, R., Wallender, W. W. & Pruitt, W. O. Estimating evapotranspiration using artificial neural network. J. Irrig. Drain. Eng. 128, 224–233 (2002).
Trajkovic, S., Todorovic, B. & Stankovic, M. Forecasting of reference evapotranspiration by artificial neural networks. J. Irrig. Drain. Eng. 129, 454–457 (2003).
Muniandy, J. M., Yusop, Z. & Askari, M. Evaluation of reference evapotranspiration models and determination of crop coefficient for Momordica charantia and Capsicum annuum. Agric. Water Manag. 169, 77–89 (2016).
Bourletsikas, A., Argyrokastritis, I. & Proutsos, N. Comparative evaluation of 24 reference evapotranspiration equations applied on an evergreen-broadleaved forest. Hydrol. Res. 49, 1028–1041 (2018).
Gao, Z., He, J., Dong, K. & Li, X. Trends in reference evapotranspiration and their causative factors in the West Liao River basin, China. Agric. For. Meteorol. 232, 106–117 (2017).
Celestin, S., Qi, F., Li, R., Yu, T. & Cheng, W. Evaluation of 32 simple equations against the Penman–Monteith method to estimate the reference evapotranspiration in the Hexi Corridor, Northwest China. Water 12, 2772 (2020).
Djaman, K., Koudahe, K., Akinbile, C. O. & Irmak, S. Evaluation of eleven reference evapotranspiration models in semiarid conditions. J. Water Resour. Prot. 9, 1469–1490 (2017).
Zakeri, M. S., Mousavi, S. F., Farzin, S. & Sanikhani, H. Modeling of reference crop evapotranspiration in wet and dry climates using data-mining methods and empirical equations. J. Soft Comput. Civ. Eng. 6, 1–28 (2022).
Mandal, D. K., Mandal, C. & Srinvas, C. V. Comparison of Penman and Hargreaves Potential Evapotranspiration estimation procedures under different climatic conditions in India. Geogr. Rev. India. 53, 26–28 (1991).
Tabari, H., Grismer, M. E. & Trajkovic, S. Comparative analysis of 31 reference evapotranspiration methods under humid conditions. Irrig. Sci. 31, 107–117 (2013).
Santha, S. & Devi, M. B. Annual rainfall prediction in Nilgiris using fuzzy logic. Eur. J. Mol. Clin. Med. 7, 2020 (2020).
Pruitt, W. O. Empirical method of estimating evapotranspiration using primarily evaporation pans. In Proceedings of the evapotranspiration and its role in water resources management (ed. Jensen, M. E.) 57–61 (ASAE, St Joseph, 1966).
Doorenbos, J. & Pruitt, W. O. Guidelines for predicting crop water requirements. FAO Irrig. Drain. Pap. 24, 1–179 (1977).
Cuenca, R. H. Irrigation system design (Prentice Hall, 1989).
Allen, R. G. & Pruitt, W. O. FAO-24 reference evapotranspiration factors. J. Irrig. Drain. Eng. 117, 758–773 (1991).
Snyder, R. L. Equation for evaporation pan to evapotranspiration conversions. J. Irrig. Drain. Eng. 118, 977–980 (1992).
Pereira, A. R., Nova, N. A. V., Pereira, A. S. & Barbieri, V. A model for the class A pan coefficient. Agric. For. Meteorol. 76, 75–82 (1995).
Orang, M. Potential accuracy of the popular non-linear regression equations for estimating pan coefficient values in the original and FAO-24 tables. Unpublished Report (California Department of Water Resources, Sacramento, 1998).
Raghuwanshi, N. S. & Wallender, W. W. Converting from pan evaporation to evapotranspiration. J. Irrig. Drain. Eng. 124, 275–277 (1998).
Grismer, M. E., Orang, M., Snyder, R. & Matyac, R. Pan evaporation to reference evapotranspiration conversion methods. J. Irrig. Drain. Eng. 128, 180–184 (2002).
Alizadeh, A., Kamali, G., Khanjani, M. J. & Rahnavard, M. R. Evaluation of evapotranspiration estimation methods in arid regions of Iran. Iran. J. Geog. Res. 19, 97–105 (2004).
Hargreaves, G. H. & Samani, Z. A. Reference crop evapotranspiration from temperature. Appl. Eng. Agric. 1, 96–99 (1985).
Schendel, U. Vegetationswasserverbrauch und -wasserbedarf (Habilitation, 1967).
Kharrufa, N. S. Simplified equation for evapotranspiration in arid regions. Beitr. Hydrol. Sonderh. 5, 39–47 (1985).
Trajkovic, S. Hargreaves versus Penman–Monteith under humid conditions. J. Irrig. Drain. Eng. 133, 38–42 (2007).
Berti, A. et al. Assessing reference evapotranspiration by the Hargreaves method in northeastern Italy. Agric. Water Manag. 140, 20–25 (2014).
Singh, V. P. Hydrologic Systems, Vol. II, Watershed Modelling (Prentice-Hall, 1989).
Papadakis, J. Potential evapotranspiration. Avenue Cordoba 4564, Buenos Aires. pp.54 (1965)
Cunha, F. F. D., Magalhães, F. F., Castro, M. A. D. & Souza, E. J. D. Performance of estimative models for daily reference evapotranspiration in the city of Cassilândia, Brazil. Eng. Agríc. 37, 173–184 (2017).
Makkink, G. F. Testing the Penman formula by means of lysimeters. J. Inst. Water Eng. 11, 277–288 (1957).
Jensen, M. E. & Haise, H. R. Estimation of evapotranspiration from solar radiation. J. Irrig. Drain. Div. 89, 15–41 (1963).
Irmark, S., Irmark, A., Allen, R. G. & Jones, J. W. Solar and net radiation-based equations to estimate reference evapotranspiration in humid climates. J. Irrig. Drain. Eng. 129, 336–347 (2003).
Irmark, S. & Haman, D. Z. Evapotranspiration: Potential or Reference? ABE 343/AE256, 6/2003. EDIS. 2003 (2003).
Caprio, J. The solar thermal unit concept in problems related to plant development ant potential evapotranspiration. In Phenology and Seasonality Modelling (ed. Lieth, H.) 353–364 (Springer, 1974).
Jones J. W & Ritchie J. T. In: Hoffman GJ, Howell TA, Solomon KH (eds) ASAE monograph no.9. ASAE, St. Joseph, 63–89 (1990).
Turc, L. Estimation of irrigation water requirements, potential evapotranspiration: a simple climatic formula evolved up to date. Ann. Agron. 12, 13–49 (1961).
Priestley, C. H. B. & Taylor, R. J. On the assessment of surface heat flux and evaporation using large scale parameters. Mon. Weather Rev. 100, 81–92 (1972).
Abtew, W. Evapotranspiration measurement and modeling for three wetland systems in South Florida. Water Resour. Bull. 32, 465–473 (1996).
Allen, R. G., Pereira, L. S., Raes, D. & Smith, M. Crop evapotranspiration-Guidelines for computing crop water requirements-FAO Irrigation and drainage paper 56. FAO Rome 300, D05109 (1998).
Chu, R. et al. Changes in reference evapotranspiration and its contributing factors in Jiangsu, a major economic and agricultural province of eastern China. Water 9, 486 (2017).
Carslaw, D. C. & Ropkins, K. Openair—An R package for air quality data analysis. Environ. Model Softw. 27, 52–61 (2012).
Gustafson, W. I. Jr. & Yu, S. Generalized approach for using unbiased symmetric metrics with negative values: Normalized mean bias factor and normalized mean absolute error factor. Atmos. Sci. Lett. 13, 262–267 (2012).
Pearson, K. VII. Mathematical contributions to the theory of evolution—III. Regression, heredity, and panmixia. Philos. Trans. R. Soc. Lond. Ser. A Contain. Pap. Math. Phys. Character. 187, 253–318 (1896).
Willmott, C. J., Robeson, S. M. & Matsuura, K. A refined index of model performance. Int. J. Climatol. 32, 2088–2094 (2012).
Taylor, K. Summarizing multiple aspects of model performance in a single diagram. J. Geophys. Res. 106, 7183–7192 (2001).
Vishwakarma, D. K. et al. Methods to estimate evapotranspiration in humid and subtropical climate conditions. Agric. Water Manag. 261, 107378 (2022).
Bogawski, P. & Bednorz, E. Comparison and validation of selected evapotranspiration models for conditions in Poland (Central Europe). Water Resour. Manag. 28, 5021–5038 (2014).
Tabari, H. Evaluation of reference crop evapotranspiration equations in various climates. Water Resour. Manag. 24, 2311–2337 (2010).
Trajkovic, S. & Kolakovic, S. Evaluation of reference evapotranspiration equations under humid conditions. Water Resour. Manag. 23, 3057–3067 (2009).
Irmak, S., Payero, J. O., Martin, D. L., Irmak, A. & Howell, T. A. Sensitivity analyses and sensitivity coefficients of standardized daily ASCE-Penman–Monteith equation. J. Irrig. Drain. Eng. 132, 564–578 (2006).
Dar, E. A., Yousuf, A. & Brar, A. S. Comparison and evaluation of selected evapotranspiration models for Ludhiana district of Punjab. J. Agrometeorol. 19, 274–276 (2017).
Muhammad, M. K. I. et al. Evaluation of empirical reference evapotranspiration models using compromise programming: A case study of Peninsular Malaysia. Sustainability. 11, 4267 (2019).
Acknowledgements
The authors would like to thank the respective head of the institute for providing the necessary support and encouragement for smooth completion of this study. Authors wish to express their gratitude to the anonymous referees whose insightful comments and constructive criticism helped us to improve the earlier version of our manuscript. Research funding support from the NRSC-ISRO (under NHP and NICES), Government of India is gratefully acknowledged.
Author information
Authors and Affiliations
Contributions
Conceptualization, supervision, methodology and formal analysis—U.S., P.R. and F.S. and Writing—U.S., P.R. and F.S. original draft preparation—S.M.; Data curation and analysis—F.S. and U.S. Project administration, investigation—P.R., M.A., P.M., M.M., S.K.A., A.R.S.; Writing—review and editing. C.V.S., K.K., V.K., M.J. All authors have read and agreed to the published version of the manuscript.
Corresponding authors
Ethics declarations
Competing interests
The authors declare no competing interests.
Additional information
Publisher's note
Springer Nature remains neutral with regard to jurisdictional claims in published maps and institutional affiliations.
Rights and permissions
Open Access This article is licensed under a Creative Commons Attribution 4.0 International License, which permits use, sharing, adaptation, distribution and reproduction in any medium or format, as long as you give appropriate credit to the original author(s) and the source, provide a link to the Creative Commons licence, and indicate if changes were made. The images or other third party material in this article are included in the article's Creative Commons licence, unless indicated otherwise in a credit line to the material. If material is not included in the article's Creative Commons licence and your intended use is not permitted by statutory regulation or exceeds the permitted use, you will need to obtain permission directly from the copyright holder. To view a copy of this licence, visit http://creativecommons.org/licenses/by/4.0/.
About this article
Cite this article
Raja, P., Sona, F., Surendran, U. et al. Performance evaluation of different empirical models for reference evapotranspiration estimation over Udhagamandalm, The Nilgiris, India. Sci Rep 14, 12429 (2024). https://doi.org/10.1038/s41598-024-60952-4
Received:
Accepted:
Published:
DOI: https://doi.org/10.1038/s41598-024-60952-4
- Springer Nature Limited