Abstract
Gastrointestinal nematode (GIN) infections have negative impacts on animal health, welfare and production. Information from molecular studies can highlight the underlying genetic mechanisms that enhance host resistance to GIN. However, such information often lacks for traditionally managed indigenous livestock. Here, we analysed 600 K single nucleotide polymorphism genotypes of GIN infected and non-infected traditionally managed autochthonous Tunisian sheep grazing communal natural pastures. Population structure analysis did not find genetic differentiation that is consistent with infection status. However, by contrasting the infected versus non-infected cohorts using ROH, LR-GWAS, FST and XP-EHH, we identified 35 candidate regions that overlapped between at least two methods. Nineteen regions harboured QTLs for parasite resistance, immune capacity and disease susceptibility and, ten regions harboured QTLs for production (growth) and meat and carcass (fatness and anatomy) traits. The analysis also revealed candidate regions spanning genes enhancing innate immune defence (SLC22A4, SLC22A5, IL-4, IL-13), intestinal wound healing/repair (IL-4, VIL1, CXCR1, CXCR2) and GIN expulsion (IL-4, IL-13). Our results suggest that traditionally managed indigenous sheep have evolved multiple strategies that evoke and enhance GIN resistance and developmental stability. They confirm the importance of obtaining information from indigenous sheep to investigate genomic regions of functional significance in understanding the architecture of GIN resistance.
Similar content being viewed by others
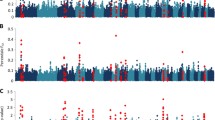
Introduction
Small ruminants (sheep and goats) make immense socio-economic and cultural contributions across the globe. However, gastrointestinal nematode (GIN) infections pose the main constraint to grazing small ruminants1, 2. The control and treatment of GIN infections is estimated to cost tens of billions of dollars worldwide3 and has traditionally relied on chemotherapeutics but, their extended use has resulted in economic losses and raised concerns of environmental health, food safety, and the development of resistance in parasites for all the major groups of anthelminthic drugs1, 4, 5. The evolution of anthelmintic resistance and changes in climate, land-use and farming practices are likely to alter the geographic distribution and infection patterns of parasites and their impacts, calling for the development of sustainable control strategies2, 6.
Evidence for host genetic variability for GIN resistance has been observed in ruminant livestock suggesting selective breeding is a feasible control option7. Indicator traits, such as faecal egg count (FEC), antibody assays, packed cell volume (PCV) and FAMACHA scores have been used to identify resistant and resilient animals8, 9. However, the mechanisms underlying genetic differences in resistance to GIN infections remain poorly understood, with resistance being a physiologically complex trait that develops over time, and indicator traits measured at specific time points may fail to represent all the pathways involved. Most often, quantifying resistance has been through artificial challenge using variable doses of larvae with which the rate, time and specificity of infection are controlled. However, traditional communal grazing management practised in smallholder mixed farming, nomadic and pastoral systems predominate in the (sub)tropics. In these systems, multiple nematode species account for natural infections which occur gradually, and findings from single infection studies may not mirror the patterns of infection encountered in these traditional systems10. There are reports showing between- as well as within-breed variation in resistance to GIN in small ruminants11,12,13. These observations and the genetic fragmentation observed in most breeds, implies that information derived from one breed cannot be extrapolated to another but needs to be validated for individual breeds5.
Advances in genomic technologies offer the opportunity to investigate the nature of genetic variation underlying complex traits14. The investigation and discovery of putative candidate genes, genomic and regulatory variants underpinning GIN resistance may provide a better understanding of the underlying molecular mechanisms and accelerate genetic gains in breeding programs. Genome-wide scans using single nucleotide polymorphisms (SNPs) have identified candidate regions, genes and QTLs putatively associated with GIN resistance on almost all ovine chromosomes11, 15,16,17,18,19. Most of the regions lie within and/or in proximity to MHC II and the interferon family of genes including the multitude of genes activated following interferon exposure20, 21, that are components of the immune response. Other QTLs for GIN resistance implicate other mechanisms, including innate and acquired immune response, gastric mucosal protection and haemostasis pathways18. Here, we utilise genome-wide autosomal genotype data generated with the Illumina Ovine 600 K SNP BeadChip and bioinformatic analysis, to conduct genome-wide screens in the hope of identifying regions and loci, linked to individual animal variability to GIN resistance in traditionally managed autochthonous Tunisian sheep.
Materials and methods
Study cohorts, sample collection, DNA extraction and genotyping
This study required no ethical approvals as all the samples were procured from slaughterhouses and in the presence of a veterinarian. In total, 309 blood samples were collected from indigenous sheep brought for slaughter at eight commercial slaughterhouses (Tunis (Tunis abattoir), Ariana (Ariana abattoir), Bizerte (Bizerte, Mateur and Sajnène abattoirs), Béja (Béja abattoir) and Jendouba (Jendouba and Tabarka abattoirs)) in northern Tunisia where GIN infections present one of the main constraints to small ruminant production22. The blood samples were collected using EDTA vacutainers by puncture of the jugular vein. DNA was extracted from each blood sample using WIZARD Genomic DNA Purification Kit (Promega, Madison, WI, USA) and then stored at − 80 °C. Out of the 309 samples, 101 were selected and assigned to two extreme groups based on GIN infection rates i.e., Non-infected (n = 58) and Infected (n = 43), based on faecal egg counts (FEC). FEC were estimated from 5 grams of stool by concentration floatation followed by the McMaster technique and the eggs per gram calculated to estimate the degree of infection23. The FEC readings ranged from zero (0) to 3800 eggs per gram. All individuals reporting an FEC = 0 were classified as non-infected and those with FEC > 100 were classified as infected.
Information on the grazing pattern and history of anthelmintic use was obtained from animal owners before slaughter. From faecal examinations, eggs of gastro-intestinal helminths (including nematodes and cestodes), Eimeria spp., Trichuris spp. and Nematodirus spp., were identified in the study individuals23 suggesting infections from multiple GIN species. The DNA samples were genotyped with the Illumina 600 K SNP BeadChip (Illumina Inc., San Diego, CA, USA) at GeneSeek Neogen Genomics, Lincoln NE, USA. The BeadChip comprises 606,006 probes that target genome-wide SNPs, among which 577,401 are autosomal, 27,314 are on the X chromosome and 1291 are unassigned.
Data quality control and screening
The 606,006 raw SNP genotypes were processed for quality control with PLINK1.924. The following criteria was used: (1) one individual was randomly selected from one pair of highly related animals with an identity-by-state score (IBS) of greater than 0.99, (2) SNPs with minor allele frequencies (MAF) of no less than 0.01 were retained, (3) individuals and SNPs with call rates lower than 90% and 95%, respectively were discarded and (4) all unmapped SNPs and those on the sex chromosomes were excluded. This generated a dataset of 540,528 autosomal SNPs and 92 samples (Infected = 41; Non-infected = 51). This dataset was subjected to LD pruning using the parameters 50 5 0.5 representing window size, step size and r2 threshold, respectively resulting in 335,070 SNPs that were used for population structure and phylogenetic analysis.
Estimation of genetic diversity
Expected (HE) and observed (HO) heterozygosity, effective population size (NE), and patterns of LD decay were investigated for the two cohorts (infected and non-infected). HE and HO were calculated using PLINK v1.9. Pair-wise LD was evaluated using the correlation coefficient (r2) between alleles at two separate SNP loci using PLINK v1.9 with default settings. Following Sved25, NE over generation time was estimated with the equation NEt = (1/4c) (1/r2 − 1), where NEt is the effective population size t generations ago (t = 1/2c); r2 is the LD between pairwise SNPs; and c is the genetic distance in Morgan between pairs of SNPs.
For each cohort, two measures of inbreeding were calculated; (1) SNP based inbreeding coefficient (F) calculated with PLINK v1.9 and (2) runs of homozygosity (ROH) based inbreeding coefficient (FROH). For the latter, ROH streatches were first computed using the “detectRUNS” package in R26. The FROH, was computed as the ratio of the total length of ROH to the length of autosomes (2.45 Gb)27.
Three estimates of ROH were calculated taking into account three genomic distance categories, ROH < 5 Mb, 5 Mb < ROH < 10 Mb, and ROH > 10 Mb, indicative of ancestral (more than ten generations), middle (5–10 generations), and recent (within five generations) inbreeding, respectively28. For the calculation, ROHs were identified for each individual using PLINK v 1.9 with the following parameters: (1) the minimum number of SNPs in a sliding window was 50; (2) the minimum ROH length was set to 1 Mb to eliminate the impact of strong LD; (3) each ROH spanned a minimum of 80 consecutive SNPs; (4) one heterozygous and five missing calls per window were allowed to avoid false negatives that may arise due to occasional genotyping errors or missing genotypes; (5) the minimum SNP density was set at one SNP every 100 kb, and the maximum gap between consecutive SNPs was set to 1 Mb.
Population structure analysis
The 335,070 SNPs were used to infer genetic structure and divergence using three methods. Principal component analysis (PCA) was performed using PLINK v1.9 and the first two PC’s were plotted to visualize individual relationships. The proportion of shared ancestry between individuals was inferred with the unsupervised mode of ADMIXTURE tool v1.3029. This mode does not assume any background information on the number and frequency of alleles in ancestral populations. The ADMIXTURE tool was run with values of K increasing gradually from 1 to 6, to derive cross-validation (CV) errors. The lowest value of the CV error indicates the most likely number of ancestral populations. Five runs were performed for each K. To test for consistency in the ADMIXTURE results, we repeated the ADMIXTURE runs using three datasets of 6,000 SNPs, each drawn at random without replacement from the 335,070 SNPs. The pairwise allele sharing distance (ASD)30 was computed using the program “asd”. A distance matrix of all pairwise ASD dissimilarities, calculated as 1-ASD, was generated and subjected to hierarchical clustering with the Neighbour-Joining algorithm to yield an individual clustering dendrogram. ASD calculation does not require estimates of allele/genotype frequencies making it valid when sample sizes are small. It is also suitable for detecting outliers and is robust to high LD among SNPs.
Detection of selection signatures and association analysis
To investigate the molecular genetic basis underlying natural variation in GIN infection, we investigated genome-wide distributions of ROH in each individual in the infected and non-infected cohorts using PLINK v1.9. For this analysis, we used the 540,528 SNPs that passed the quality thresholds. Using the “detectRUNS”, we counted the number of times a given SNP occurred in the identified ROH in each cohort and presented a Manhattan plot of all the tested SNPs against their autosomal positions. The most frequently observed SNPs in ROHs occurring at the top 25% of the empirical distribution were taken as the most significant loci underlying an ROH under selection. To identify regions of ROH that are likely associated with variations in GIN infection, we compared the ROH regions between the infected and non-infected cohorts and identified the ones that were specific to the non-infected cohort.
We performed the logistic regression (LR) GWAS, FST and XP-EHH analyses to investigate further the genome regions associated with variation in GIN infection. LR-GWAS was performed with PLINK v1.9 using the non-infected cohort as the test sample and the infected cohort as the control. To obtain the 99% confidence intervals for the estimated parameters, the “-ci 0.99” and “-covar” options were invoked and Fisher’s exact test was used to generate the p-values considering location, breed, sex, and age as covariates. The raw p-values were subjected to Bonferroni correction to control the likelihood of any false positive results among the markers identified to be under selection. The corrected p-values were standardized and the − Log 10 (p-value) of 4.25 (the top 0.001) was set as the cut-off threshold to identify candidate regions. The estimations were summarized in 200 Kb window sizes and the genes falling within the candidate regions were identified. The package ’qqman’ in R version 3.5.1 was used to generate the Manhattan plot.
The population differentiation statistic, FST, was used to investigate regions of the genome that have diverged between the two cohorts. The unbiased pairwise FST31 was computed using the HIERFSTAT Package of R32 using a window size of 200 Kb and a window-step size of 100 Kb. Windows with less than five SNPs were excluded from the analysis. The FST values were then standardized by Z transformation following Ahbara et al.33. Windows falling within the top 0.001% of the FST values in each chromosome were considered the putative candidates under divergent selection.
The cross-population extended haplotype homozygosity (XP-EHH)34 was used to compare expected haplotype homozygosity (EHH) and integrated haplotype score (iHS) between the two cohorts to detect selection and its direction. XP-EHH scans SNPs that are homozygous in one population but polymorphic in the other through pairwise comparison of EHH scores. Positive XP-EHH scores indicate selection in the test sample, while negative scores indicate selection in the control. The XP-EHH scores were estimated as:
where IA is the integrated EHH value of the test population and IB is the integrated EHH value of the reference population. Haplotype phasing was inferred for each cohort simultaneously on all SNPs using BEAGLE v3.3.135. The XP-EHH test was performed with the “rehh” package of R36 and the raw XP-EHH scores were standardized to a distribution with zero mean and unit variance. Selection candidates were considered as the regions contained in any of the 200 Kb windows with a significance threshold of p < 0.001; this equates to an XP-EHH value of 4 at the default settings of “rehh” estimation.
Functional annotation of candidate regions
The candidate regions identified by ROH were analysed and the ones that were specific to the non-infected cohort identified. We also analysed the ROH regions of the non-infected cohort, LR-GWAS, FST and XP-EHH candidate regions and the ones that overlapped between at least two approaches were identified and merged using Bedtools v.2.28.037. Genes that were spanned by the ROH (non-infected cohort) and overlapping candidate regions were retrieved using the Biomart/Ensembl (http://www.ensembl.org/biomart) tool based on the Ovine v3.1 reference genome assembly. The set of genes identified in the candidate regions were assessed for biological enrichment gene ontology (GO) and KEGG Pathway (www.kegg.jp/kegg/kegg1.html) terms compared to the full list of Ovis aries autosomal protein-coding genes with the functional annotation tool in DAVID v6.838 using O. aries as the background species. We also mapped the ROH (non-infected) and overlapping candidate regions with those reported in the sheep quantitative trait loci (QTL) database Release 42 (QTLdb; https://www.animalgenome.org/cgi-bin/QTLdb/OA/summary) to identify overlapping QTLs, which may suggest associations with response to parasite infections. To provide further biological interpretation, gene functions were determined from the NCBI database (http://www.ncbi.nlm.nih.gov/gene/) and review of literature.
Results
Genetic diversity estimates
The average estimates of HO, HE, F, FROH and ROH size did not differ significantly (p = 0.05) between the two cohorts (Table 1). However, the non-infected cohort showed marginally higher values of F and ROH size while the infected animals had marginally higher values of HO, HE and FROH. The number and length of ROH estimated for the 0–5 Mb, 5–10 Mb, > 10 Mb genome length categories are shown in Table 2. The most and least frequent ROH length was observed for the 0–5 Mb and > 10 Mb length categories, respectively. The average length of ROH per animal was highest, in the 5–10 Mb length category for non-infected cohort and > 10 Mb length category for the infected cohort. The two cohorts show similar patterns of LD decay over genomic distance although the non-infected cohort showed overall lower LD (r2) (Fig. 1a). In general, the pattern of LD decay shows higher LD at shorter distances which declines rapidly and plateaus off from 0.4 Mb for both cohorts (Fig. 1b). The trend in NE over generation time was the same for both cohorts (Fig. 1c). They are characterised by an increase in NE from 1000 generations ago, which attains maximum value at approximately 330 generations ago, and then declines to the present time. Generally, the non-infected cohort had higher NE across all generations.
Population structure analysis
Population structure and relationship was investigated using PCA (Fig. 2a), ADMIXTURE tool (Fig. 2b,c) and ASD phylogeny (Fig. 2d). The first and second PC’s of the PCA explained, respectively 1.74% and 1.45% of the total genetic variation. The study animals did not differentiate into distinct genetic groups/clusters that correspond to their infection status. Following ADMIXTURE analysis, the lowest CV error was at K = 1 (Fig. 2b) which suggests no genetic differentiation. The ADMIXTURE plot reveals that a similar pattern of genomic composition characterizes the two cohorts at 2 ≤ K ≤ 6 (Fig. 2c). This pattern was replicated when ADMIXTURE was performed using three datasets comprising 6000 SNPs each, drawn at random without replacement from the 335,070 SNPs. The ASD phylogeny also showed no genetic stratification (Fig. 2d).
Population genetic structure and phylogenetic analysis of the two cohorts of autochthonous Tunisian sheep (a) PCA cluster analysis showing PC1 and PC2; (b) Cross-validation plot for admixture analysis; (c) Admixture analysis plot showing the genetic backgrounds present in the study cohorts for 2 ≤ K ≤ 6; (d) ASD phylogenetic tree of individuals of the study population. INF infected cohort; NINF non-infected cohort.
Genome-wide selection signature analysis
The ROH analysis identified 60 ROH regions in the two cohorts (Fig. 3a,b) that spanned 311 genes. The LR-GWAS, FST and XP-EHH identified 346, 32, and 68 regions (Fig. 4a–c), respectively which spanned 673, 152, and 295 genes. These 446 candidate regions overlapped with 645 genes (Supplementary Table S1) of which 71 were found in candidate regions that were identified by at least two methods (Fig. 5).
We considered the ROH analysis as a method that identifies selection signatures within a cohort. We therefore compared the ROH results of infected and non-infected cohorts (Fig. 3a,b). This identified 23 ROH regions that were specific to the non-infected cohort and which spanned 80 putative genes (Table 3) of which 30 remain uncharacterised/unannotated (prefixed with “ENSOARG”). Six of the 23 candidate regions occurred on OAR 1, 2, 3, 6, 10 and 14 and overlapped with FECGEN, LATRICH_2 and NFEC QTLs (Table 3) that are associated with health traits and in particular, parasite resistance.
The LR-GWAS was used to identify candidate regions and possible SNPs associated with GIN infection, while FST, and XP-EHH were also used to investigate selection signatures by contrasting the non-infected and infected cohorts. These three approaches identified 30 candidate regions that overlapped between at least two methods across 17 autosomes (Table 4). When the ROH regions that are specific to the non-infected cohort are considered, the four methods identify 35 candidate regions overlapping between at least two approaches across 19 autosomes and span 121 genes including 11 which remain uncharacterized (Table 4). Of the 35 candidate regions, five that were identified by ROH to be specific to the non-infected cohort overlap with at least one region that was identified by either LR-GWAS, FST and/or XP-EHH and span 13 genes (Table 4) while one region found on OAR 5 (region number 14) was identified by all the four methods (Table 4). This region spans four genes (LOC101104745, PCDHGA1, PCDHGA2, ENSOARG00000000218) and one QTL trait (BIRTH_WT, Body weight (birth)). None of the four genes are associated, directly or indirectly, with endoparasite resistance. Nineteen regions found across OAR 1, 3, 6, 8, 11, 12, 13, 14, 17, 18 and 21 span FECGEN, TFEC_1, HFEC, NFEC, LATRICH_2, IGA, SAOS, WORMCT, PEPSL and CEOSIN QTLs that are linked to response to GIN infection (Table 4). Ten regions overlapped with production (growth) QTL traits (BW, BIRTH_WT, BONE_WT) across OAR 2, 4, 5 and 6, four regions overlapped with meat and carcass traits associated with fatness QTLs (HCWT, FAT_WT, LMYP) across OAR 2 and OAR 10, and anatomy QTLs (BONE_WT) on OAR 24 (Table 4).
Functional enrichment analysis was first tested in the pool of 51 genes, excluding uncharacterized genes, that are present in the 23 ROH candidate regions that were specific to the non-infected cohort (Table 3). A second functional enrichment analysis was performed with the set of 110 genes, excluding 11 uncharacterized ones that were present in the 35 candidate regions that overlapped between at least one ROH, LR-GWAS, FST and XP-EHH regions (Table 4). We found two functional term clusters that were significantly (enrichment score > 1.5) enriched for the genes present in the ROH regions of the non-infected cohort (Table 5). These were associated with “immune system process” and “cytokine receptor interaction”. The second analysis resulted in three significantly (enrichment score > 1.5) enriched clusters. The first cluster was associated with the GO biological terms “Carboxylesterase type B” and “Hydrolase activity”. The “Apical plasma membrane” term, which has a role in intestinal innate immunity, and “Integrin signalling” that functions in immune cells were among the most significant GO biological terms in the second and third clusters, respectively.
Discussion
GIN infections and associated gastroenteritis impact negatively the production efficiency of ruminant livestock, and their management is essential to meet future demand for animal source foods. The observation of large variations in GIN infection suggests variability at the genome level that underpins inter-animal variability in susceptibility39, 40. Rouatbi et al.23 observed inter-individual variation in GIN infection in autochthonous Tunisian sheep leading to the suggestion that it could have a genetic basis. We therefore generated and analysed Ovine 600 K SNP BeadChip genotype data, from 92 samples that comprised GIN infected and uninfected animals under field challenge from Rouatbi et al.23 study. The aim was to investigate signatures of variability in GIN infection and resistance in autochthonous Tunisian sheep that are managed under natural grazing and no history of anthelmintic intervention. We applied four methods to detect genomic regions associated with GIN resistance. Although our study yielded some interesting findings, we acknowledge that studies that use naturally acquired field exposure to deliver a challenge always run the risk of uninfected animals being a combination of truly highly resistant animals, animals that never saw infection and animals that have had infection cleared due to chemical treatment. Additionally, producer provided information is at times not always accurate. These factors may serve to dilute the certainty that the two groups are in fact functionally dissimilar.
Although we expected the two cohorts to show differences in genetic diversity and structure, this was not the case; they showed similar levels of genetic diversity and inbreeding and the three clustering algorithms provided corroborating evidence of lack of stratification that was consistent with infection status. The lack of genetic differentiation and structure was also reported between prolific and non-prolific cohorts of Bonga sheep from Ethiopia41 and in the Brazilian Santa Inês breed which shows variability in resistance to GIN infection19. The absence of genetic stratification appears to be a characteristic of sheep from the Maghreb as it has also been observed in sheep populations from Algeria42 and Morocco43 implicating extensive intermixing and cross-mating. There are four possible explanations for the lack of genomic stratification corresponding to infection status. (1) The selection pressure driving the differences in GIN infection is/has not been stringent or long enough to result in differentiation at the genome level; (2) the level of parasite infection may be too low to result in meaningful genomic variation; (3) it may point to a lack of farmer-driven selection that is biased towards the use of uninfected animals for breeding; and/or (4) there could be a high natural selection pressure whereby all animals effectively have the resistance genotype and therefore rather than a distribution, spanning susceptible to resistant, there is a skewed distribution that spans “moderately resistant” to “highly resistant” individuals. One or all of these reasons may explain the lack of genetic differentiation between the two cohorts. The level of genetic diversity in the two cohorts is higher than that observed in commercial breeds, but it falls within the range of values reported in other sheep found in Africa and China44,45,46.
We assessed the average length and number of ROH per individual at three genomic distance categories and the trends in NE over generation time for each cohort. The two cohorts had a high frequency of ROH in the shorter (0–5 Mb) length category reflecting older evolutionary events such as past or ancestral inbreeding. The two cohorts showed similar patterns and trends in LD decay and NE. However, the non-infected cohort had higher values of NE across all generations. This difference is difficult to explain but we presume it may be the result of higher mortalities in the infected cohort. Both cohorts show a gradual increase in NE from 1000 generations ago. This is followed by a drastic decline from around 330 generations ago to the present time suggesting a bottleneck event. A similar trend was observed in Ethiopian and Sudanese local sheep47. Assuming a generation time of three years for traditionally managed local sheep, it can be inferred that the increase in NE started around 3000 years ago. The start of the decline in NE 330 generations back translates to around 990 years ago. Between 240 and 1140 years ago, three favourable climatic periods interspersed with short extreme dry spells were experienced in the continent48. We suggest that the sheep populations most likely thrived when conditions were optimal but shrunk during subsequent droughts. The footprints of these demographic perturbations appear to have been retained in the genomes of the indigenous sheep.
Our analysis revealed 35 candidate regions, spanning 110 genes, that overlapped between at least two out of the four methods we used to detect genomic regions associated with differences in endo-parasite infections. The identification of overlapping candidate regions that are under selection by different approaches suggests plausible evidence for selective influences at the genome49. The convergence of our results points to the reliability of our findings and suggest that they are unlikely to be the outcome of chance events or analytical artefacts.
Both the within (ROH) and between-population (LR-GWAS, FST, XP-EHH) approaches identified genomic regions that could be driving GIN resistance in autochthonous Tunisian sheep. At least two or more methods identified the same candidate regions that colocalized with the FECGEN, TFEC_1, HFEC, NFEC, LATRICH_2, IGA, SAOS, WORMCT, PEPSL and CEOSIN QTLs that have been associated with health traits and in particular parasite resistance, immune capacity and disease susceptibility in sheep showing resistance to GIN50,51,52. This result indicates that some common QTLs underly parasite resistance traits in sheep and support a role for convergent evolution in driving host GIN resistance. For instance, the FECGEN QTL which results in reduced FEC in unmanaged, naturally-parasitized domestic sheep53 and in the primitive Soay sheep20, has been associated with a microsatellite allele (o(IFN)-γ126) found in the first intron of the interferon gamma (IFN)-γ gene and with increased titre of Teladorsagia circumcincta-specific IgA. It has been shown that the effects of the o(IFN)-γ126 allele and IgA on FEC are not independent, and that IgA may mediate the (IFN)-γ effect on FEC20. Furthermore, the IGA QTL could be related to early response to incoming larvae, whereas the FECGEN QTL may be associated with the ability to avoid the development of adult parasites52, 54. The fact that the candidate regions spanned QTLs associated with different QTL traits was expected because the animals analysed in this study are grazed in communal pastures where challenge from multiple GIN parasite species is common and could present different aspects of host-parasite interaction during infection. Indeed, eight nematode species, T. circumcincta, T. trifurcate, Haemonchus contortus, Marshallagia marshalli, Trichostrongylus vitrines, T. axei, Ostertagia lyrate, O. ostertagi and O. occidentalis, were found to colonize the abomasum of the study individuals23.
QTL and GWAS studies suggest that GIN infections can evoke several host responses that enhance innate and acquired immune responses, gastric mucosal protection, haemostasis pathways, delay in parasite development and reduction in number of eggs produced by GIN16. These manifestations depend on the nematode species, parasite exposure, and host-specific factors including age, sex, genetic make-up, hormonal and nutritional status55. The animals analysed in our study are grazed under natural open pastures and are exposed to a wide range of GIN. Our expectation, therefore, was that they would exhibit a large repertoire of host defence mechanisms which may be reflected in the functions of the putative genes present in the candidate regions. We observed two organic cation transporters, SLC22A4 (OCTN1) and SLC22A5 (OCTN2) which are carriers of hydroxyurea, in an overlapping candidate region on OAR 5 (Table 4). Hydroxyurea can inhibit ribonucleotide reductase (RR) and is retained at high concentrations in tissues of various mammalian species including sheep56. Compared to viral or bacterial ribonucleotide reductase, the mammalian RR is less susceptible to inhibition by hydroxyurea56. This action can be critical in inhibiting viral and bacterial replication without affecting mammalian cellular growth. Hydroxyurea can therefore offer a level of immunity against GIN infection as part of the innate immune defence system which may inhibit endoparasites in the gastrointestinal tract of non-infected animals. Some variants present in SLC22A4 and SLC22A5 have also been associated with Inflammatory Bowel and Crohn’s disease’s in humans57, 58.
We observed BMPR2 (Bone Morphogenetic Protein Receptor Type 2) in an overlapping candidate region on OAR 2 (Table 4). Ligands of this gene are members of the TGFβ superfamily whose homologues are key players in inducing immunological tolerance59. Elevated expression of TGFβ have been observed in mammalian hosts that mount an effective immune response against GIN59,60,61,62. BMPR2 was found to be highly expressed in mesenteric lymph nodes of cattle that were selected for resistance to intestinal nematodes63 suggesting association with parasite resistance. BMPR2 also occurred in a cluster of genes that were upregulated following Eimeria acervulina infection in chicken and associated with the functional term “Inflammatory Response” in the “Disease and Disorders” category64. Several mechanisms may operate to increase the levels of TGFβ under parasite infection including, host homeostasis to minimize immunopathology under chronic infection; pathogens triggering TGFβ production or activation; or parasite mimicry of the host cytokine to drive the same pathway as the hosts TGFβ59. Examples of all three have been reported in diverse sheep breeds59 and the one that operates in autochthonous Tunisian sheep remains unknown calling for further investigation.
The IL-4 (Interleukin-4) and IL-13 (Interleukin-13) occurred in a candidate region on OAR 5 that was specific to the non-infected cohort. IL-4 plays a crucial role in the differentiation of naive T helper (Th) cells into Th2 effector cells which promote humoral immunity and provide protection against intestinal helminths65. In sheep, Jacobs et al.66 observed that impaired IL-4 signalling promoted the establishment of H. contortus and increased larval burden. An increase in IL-13 in intestinal lymph cells was observed in sheep selected for increased resistance to nematodes67. Two genes RUFY4 (RUN and FYVE Domain Containing Protein 4) and VIL1 (Villin 1) and two IL-8 receptors (CXCR1 and CXCR2) occurred in a strong candidate region on OAR 2 that was also identified by ROH to be specific to the non-infected cohort. RUFY4 is a positive regulator of autophagy and is expressed in a cell-specific manner or under specific immunological conditions associated with IL-4 expression68. VIL1, has been shown to protect gut epithelial cells by decreasing epithelial damage69. It was among the top 30 proteins that were differentially regulated between resistant and susceptible sheep and may play an important role in maintaining the epithelial integrity of abomasal mucosa in response to haemonchosis70. It can therefore be hypothesized that the interaction between RUFY4 and VIL1 with IL-4 may enhance long-term endoparasite resistance in sheep. The two IL-8 receptors were found to be expressed and distributed in normal and morphologically damaged large intestines, suggesting that IL‐8 may play an important role in mediating immune response in gastrointestinal tract, beyond that of potentiating neutrophil recruitment and inflammation71 following intestinal epithelial damage by endoparasites.
Protective immunity against GIN and subsequent parasite expulsion is known to be mediated by Th2 immune response that is orchestrated by the secretion of cytokines such as IL-4, IL-5, IL-10 and IL-13, which promote the recruitment of eosinophils, basophils, mast cells, goblet cell hyperplasia and concurrent mucus and antibody production72,73,74. Nematode expulsion is known to rely on smooth muscle contraction and increased mucus production75. The latter was found to be mediated by intelectin 1 (ITLN), a calcium dependent lectin, that is expressed by Paneth cells in the small intestine of mouse76 and mucus neck cells in the abomasum of sheep77. The expression of ITLN in sheep goblet cells was found to be upregulated by IL-477. High expression of ITLN was also found to be induced during nematode expulsion in Trichinella spiralis and Nocardia brasiliensis in rodents78, 79 and T. circumcincta infections in sheep77, 80. Th2 response has also been described as a mediator for acute wound healing during helminth infection81. We therefore suggest that the upregulation of ITLN by IL-4 may play a role in enhancing GIN resistance in autochthonous Tunisian sheep through parasite expulsion and enhancing wound healing.
Other possible candidate genes that have been associated with GIN resistance and occur in our overlapping candidate regions include FGF2, FAM78B, SPATA5, SPPL2B and FAM96B. FGF2 also known as basic fibroblast growth factor (bFGF and/or FGF-β), is a growth factor and signaling protein that can synergize with IL-17 in the gut to activate the ERK pathway and induce genes for repairing damaged intestinal epithelium82. The gut epithelium is essentially the first line of defence against microbiota and pathogens and therefore, it plays a critical role in enhancing mucosal immunity. FAM78B (Family with Sequence Similarity 78-Member B) was in an overlapping region on OAR 1. In a genome-wide association study of endo-parasite phenotypes, FAM78B was found in a region on bovine chromosome 3 that spanned SNPs that were most strongly associated with antibody response to O. ostertagi40. SPATA5 (Spermatogenesis Associated 5) was among five genes that were included in the most significant functional term cluster linked with immunity-related and cell-proliferation processes in an investigation of genomic regions and genes for gastrointestinal parasite resistance in Djallonke sheep83. SPPL2B (Signal peptide peptidase-like 2B), a member of the signal peptide peptidase-like protease (SPPL) family, localizes to endosomes, lysosomes and plasma membrane and plays a role in enhancing innate and adaptive immunity by cleaving TNFα in activated dendritic cells that triggers IL-12 production84. In humans and chicken, there is strong evidence linking polymorphisms in FAM96B (family with sequence similarity 96 member B) with gastrointestinal and metabolic diseases, and with the development of the digestive system and disorder networks85, 86. These findings suggest that several mechanisms may be employed to trigger and sustain GIN resistance in sheep under traditional grazing and exposure to multiple GIN species.
Our analysis revealed several candidate genomic regions spanning a number of production (growth), and meat and carcass (fatness and anatomy) QTLs. This result was unexpected given our analytical strategy. Kipper et al.87 reported a reduction of 5% and 31% in average daily feed intake and average daily weight gain, respectively in parasitized pigs. Endoparasites tend to limit host nutrient availability by reducing host food intake, digestion, absorption and nutrient assimilation, resulting in nutritional deprivation and destabilization of host growth and development88, 89. To counter against these negative effects, we suggest that natural selection may be acting on the regions spanning growth QTLs to ensure growth and developmental stability of the study populations under GIN challenge. The parallel selection signatures overlapping the growth QTLs may therefore be adaptive strategy that ensures the survival of the study populations.
In conclusion, we assessed the diversity and population structure of two cohorts (infected and non-infected) of autochthonous sheep from Tunisia that were classified based on FEC levels. The two cohorts were characterised by similar levels of genetic diversity and the same genome background suggesting common history and genetic admixture. Four methods of detecting selection signatures identified regions of the genome that were most likely associated with GIN resistance suggesting that the animals have established a certain level of immunity under natural challenge. The functions of the putative genes and the overlapped QTLs suggest multiple strategies, including host immune response, intestinal epithelium damage repair, mucus production and parasite expulsion, play a role in GIN resistance in sheep. This may be due in part to the fact that GIN resistance is the net outcome of many physiological and immunological pathways, and thus resistance in animals could be owing to variation at a large number of loci. Furthermore, natural selection is also acting concurrently on regions spanning growth related QTLs to ensure developmental stability under GIN challenge. Although the data used in our study is relatively small, we believe this does not compromise the integrity of our findings as it is compensated for by the high density of the marker loci used. We have also limited our discussion to candidate regions that were specific to the non-infected cohort and those that overlap between at least two analytical approaches. This research confirms the importance of obtaining data from local sheep populations managed in their production environments to gather information on genomic regions of functional significance in GIN resistance.
Data availability
The data used in here is available from the communicating author upon reasonable request.
References
Papadopolous, E. Anthelmintic resistance in sheep nematodes. Small Rumin. Res. 76, 99–103 (2008).
Miller, C. M. et al. The production cost of anthelmintic resistance in lambs. Vet. Parasitol. 186, 376–381 (2012).
Roeber, F., Jex, A. R. & Gasser, R. B. Impact of gastrointestinal parasitic nematodes of sheep, and the role of advanced molecular tools for exploring epidemiology and drug resistance—An Australian perspective. Parasites Vectors 6, 153. https://doi.org/10.1186/1756-3305-6-153 (2013).
Papadopolous, E., Gallidis, E. & Ptochos, S. Anthelmintic resistance in sheep in Europe: A selected review. Vet. Parasitol. 189, 85–88 (2012).
Zvinorova, P. I. et al. Breeding for resistance to gastrointestinal nematodes—The potential in low-input/output small ruminant production systems. Vet. Parasitol. 225, 19–28 (2016).
Charlier, J., van der Voort, M., Kenyon, F., Skuce, P. & Vercruysse, J. Chasing helminths and their economic impact on farmed ruminants. Trends Parasitol. 30, 361–367 (2014).
Kemper, K. E. et al. The distribution of SNP marker effects for faecal worm egg count in sheep, and the feasibility of using these markers to predict genetic merit for resistance to worm infections. Genet. Res. 93, 203–219 (2011).
Burke, J. M. & Miller, J. E. Use of FAMACHA system to evaluate gastrointestinal nematode resistance/resilience in offspring of stud rams. Vet. Parasitol. 153, 85–92 (2008).
Shaw, R. J., Morris, C. A., Wheeler, M., Tate, M. & Sutherland, I. A. Salivary IgA: A suitable measure of immunity to gastrointestinal nematodes in sheep. Vet. Parasitol. 186, 109–117 (2012).
Munoz-Guzman, M. A., Cuellar-Ordaz, J. A., Valdivia-Anda, A. G., Buendia-Jimenez, J. A. & Alba-Hurtado, F. Correlation of parasitological and immunological parameters in sheep with high and low resistance to haemonchosis. Can. J. Anim. Sci. 86, 363–371 (2006).
McRae, K. M. et al. Response to Teladorsagia circumcincta infection in Scottish Blackface lambs with divergent phenotypes for nematode resistance. Vet. Parasitol. 206, 200–207 (2014).
McBean, D. et al. Faecal egg counts and immune markers in a line of Scottish Cashmere goats selected for resistance to gastrointestinal nematode parasite infection. Vet. Parasitol. 229, 1–8 (2016).
Aboshady, H. M., Stear, M. J., Johansson, A., Jonas, E. & Bambou, J. C. Immunoglobulins as biomarkers for gastrointestinal nematodes resistance in small ruminants: A systematic review. Sci. Rep. 10, 7765. https://doi.org/10.1038/s41598-020-64775-x (2020).
Andersson, L. & Georges, M. Domestic-animal genomics: Deciphering the genetics of complex traits. Nat. Rev. Genet. 5, 202–212 (2004).
Pickering, N. K., Auvray, B., Dodds, K. G. & McEwan, J. C. Genomic prediction and genome-wide association study for dagginess and host internal parasite resistance in New Zealand sheep. BMC Genom. 16, 958. https://doi.org/10.1186/s12864-015-2148-2 (2015).
Benavides, M. V. et al. Identification of novel loci associated with gastrointestinal parasite resistance in a red Maasai × Dorper backcross population. PLoS ONE 10, 1–20. https://doi.org/10.1371/journal.pone.0122797 (2015).
Atlija, M., Arranz, J.-J., Martinez-Valladares, M. & Gutiérrez-Gil, B. Detection and replication of QTL underlying resistance to gastrointestinal nematodes in adult sheep using the ovine 50K SNP array. Genet. Sel. Evol. 48, 4. https://doi.org/10.1186/s12711-016-0182-4 (2016).
Benavides, M. V., Sonstegard, T. S. & Van Tasssell, C. Genomic regions associated with sheep resistance to gastrointestinal nematodes. Trends Parasitol. 32, 470–480 (2016).
Berton, M. P. Genomic regions and pathways associated with gastrointestinal parasites resistance in Santa Inês breed adapted to tropical climate. J. Anim. Sci. Biotechnol. 8, 73. https://doi.org/10.1186/s40104-017-0190-4 (2017).
Coltman, D. W., Wilson, K., Pilkington, J. G., Stear, M. J. & Pemberton, J. M. A microsatellite polymorphism in the gamma interferon gene is associated with resistance to gastrointestinal nematodes in a naturally-parasitized population of Soay sheep. Parasitology 122, 571–582 (2001).
Janßen, M., Weimann, C., Gauly, M. & Erhardt, G. Associations between infections with Haemonchus contortus and genetic markers on ovine chromosome 20. In Proc. Of the 7th World Congr. Genet. Appl. to Livest. Prod. Session 13 Disease resistance, Communication N° 13-11, August 19-23 Montpellier, France (2002).
Akkari, H., Gharbi, M. & Darghouth, M. A. Dynamics of infestation of tracers lambs by gastrointestinal helminths under a traditional management system in the North of Tunisia. Parasite 19, 407–415 (2012).
Rouatbi, M. et al. Individual variability among autochthonous sheep in Northern Tunisia to infection by abomasum nematodes and Babesia/Theileria parasites. Vet. Med. Sci. https://doi.org/10.1002/vms3.310 (2020).
Purcell, S. et al. PLINK: A tool set for whole-genome association and population-based linkage analyses. Am. J. Hum. Genet. 81, 559–575 (2007).
Sved, J. A. Linkage disequilibrium and homozygosity of chromosome segments in finite populations. Theor. Popul. Biol. 2, 125–141 (1971).
Biscarini, F., Cozzi, P., Gaspa, G. & Marras, G. detectRUNS: Detect runs of homozygosity and runs of heterozygosity in diploid genomes. CRAN (The Comprehensive R Archive Network) (2019).
McQuillan, R. et al. Runs of homozygosity in European populations. Am. J. Hum. Genet. 83, 359–372 (2008).
Mastrangelo, S. et al. Runs of homozygosity reveal genome-wide autozygosity in Italian sheep breeds. Anim. Genet. 49, 71–81 (2018).
Alexander, D. H., Novembre, J. & Lange, K. Fast model-based estimation of ancestry in unrelated individuals. Genome Res. 19, 1655–1664 (2009).
Gao, X. & Martin, E. R. Using allele sharing distance for detecting human population stratification. Hum. Heredity 68, 182–191 (2009).
Weir, B. S. & Cockerham, C. C. Estimating F-statistics for the analysis of population structure. Evolution 38, 1358–1370 (1984).
Goudet, J. Hierfstat, a package for R to compute and test hierarchical F-statistics. Mol. Ecol. Notes. 5, 184–186 (2005).
Ahbara, A. et al. Genome-wide variation, candidate regions and genes associated with fat deposition and tail morphology in Ethiopian indigenous sheep. Front. Genet. 9, 699. https://doi.org/10.3389/fgene.2018.00699 (2019).
Sabeti, P. et al. Genome-wide detection and characterization of positive selection in human populations. Nature 449, 913–918 (2007).
Browning, S. R. & Browning, B. L. Rapid and accurate haplotype phasing and missing-data inference for whole-genome association studies by use of localized haplotype clustering. Am. J. Hum. Genet. 81, 1084–1097 (2007).
Gautier, M. & Vitalis, R. rehh: An R package to detect footprints of selection in genome-wide SNP data from haplotype structure. Bioinformatics 28, 1176–1177 (2012).
Quinlan, A. R. & Hall, I. M. BEDTools: A flexible suite of utilities for comparing genomic features. Bioinformatics 26, 841–842 (2010).
Huang, D. W., Sherman, B. T. & Lempicki, R. A. Systematic and integrative analysis of large gene lists using DAVID bioinformatics resources. Nat. Protoc. 4, 44–57 (2008).
Gasbarre, L. C., Leighton, E. A. & Sonstegard, T. Role of the bovine immune system and genome in resistance to gastrointestinal nematodes. Vet. Parasitol. 98, 51–64 (2001).
Twomey, A. J. et al. Genome-wide association study of endo-parasite phenotypes using imputed whole-genome sequence data in dairy and beef cattle. Genet. Sel. Evol. 51, 15. https://doi.org/10.1186/s12711-019-0457-7 (2019).
Dolebo, A. T. et al. Genome-wide scans identify known and novel regions associated with prolificacy and reproduction traits in a sub-Saharan African indigenous sheep (Ovis aries). Mamm. Genome 30, 339–352. https://doi.org/10.1007/s00335-019-09820-5 (2019).
Gaouar, S. B. S. et al. Genome-wide analysis highlights genetic dilution in Algerian sheep. Heredity 118, 293–301 (2017).
Belabdi, I. et al. Genetic homogenization of indigenous sheep breeds in Northwest Africa. Sci. Rep. 9, 7920. https://doi.org/10.1038/s41598-019-44137-y (2019).
Edea, Z., Dessie, T., Dadi, H., Do, K.-T. & Kim, K.-S. Genetic diversity and population structure of Ethiopian sheep populations revealed by high-density SNP markers. Front. Genet. 8, 218. https://doi.org/10.3389/fgene.2017.00218 (2017).
Kim, E.-S. et al. Multiple genomic signatures of selection in goats and sheep indigenous to a hot arid environment. Heredity 116, 255–264 (2016).
Cheng, J. et al. Population structure, genetic diversity, and selective signature of Chaka sheep revealed by whole genome sequencing. BMC Genom. 21, 520. https://doi.org/10.1186/s12864-020-06925-z (2020).
Ahbara, A.M. Autosomal genome-wide analysis of diversity, adaptation and morphological traits in African indigenous sheep. PhD Thesis, University of Nottingham, UK pp. 183 (2019).
Verschuren, D. Decadal and century-scale climate variability in tropical Africa during the past 2000 years. In Past Climate Variability Through Europe and Africa (eds Battarbee, R. W. et al.) 139–158 (Kluwer Academic Publishers, 2007).
Qanbari, S. & Simianer, H. Mapping signatures of positive selection in the genome of livestock. Livest. Sci. 166, 133–143 (2014).
Balic, A., Bowles, V. M., Liu, Y. S. & Meeusen, E. N. Local immune responses in sensitized sheep following challenge infection with Teladorsagia circumcincta. Parasite Immunol. 25, 375–381 (2003).
Marshall, K. et al. Genetic mapping of quantitative trait loci for resistance to Haemonchus contortus in sheep. Anim. Genet. 40, 262–272 (2009).
Gutiérrez-Gil, B. et al. Quantitative trait loci for resistance to trichostrongylid infection in Spanish Churra sheep. Genet. Sel. Evol. 41, 46. https://doi.org/10.1186/1297-9686-41-46 (2009).
Crawford, A.M. & McEwen, J.C. Identification of Animals Resistant to Nematode Parasite Infection. New Zealand Provisional Patent 330201. New Zealand (1998).
Davies, G. et al. Quantitative trait loci associated with parasitic infection in Scottish blackface sheep. Heredity 96, 252–258 (2006).
Vercruysse, J. et al. Control of helminth ruminant infections by 2030. Parasitology 145, 1655–1664 (2018).
Fraser, D. I. et al. Widespread natural occurrence of hydroxyurea in animals. PLoS ONE 10, 11. https://doi.org/10.1371/journal.pone.0142890 (2015).
Leung, E. et al. Polymorphisms in the organic cation transporter genes SLC22A4 and SLC22A5 and Crohn’s disease in a New Zealand Caucasian cohort. Immunol. Cell Biol. 84, 233–236 (2006).
McCann, M. J. et al. The effect of turmeric (Curcuma longa) extract on the functionality of the solute carrier protein 22 A4 (SLC22A4) and interleukin-10 (IL-10) variants associated with inflammatory bowel disease. Nutrients 6, 4178–4190 (2014).
Johnston, C. J. C., Smyth, D. J., Dresser, D. W. & Maizels, R. M. TGF-Beta in tolerance, development and regulation of immunity. Cell. Immunol. 299, 14–22 (2016).
Leng, Q., Bentwich, Z. & Borkow, G. Increased TGF-beta, Cbl-b and CTLA-4 levels and immunosuppression in association with chronic immune activation. Int. Immunol. 18, 637–644 (2006).
Turner, J. D. et al. Intensity of intestinal infection with multiple worm species is related to regulatory cytokine output and immune hyporesponsiveness. J. Infect. Dis. 197, 1204–1212 (2008).
Elias, D., Britton, S., Aseffa, A., Engers, H. & Akuffo, H. Poor immunogenicity of BCG in helminth infected population is associated with increased in vitro TGF-β production. Vaccine 26, 3897–3902 (2008).
Araujo, R. N. et al. Use of candidate gene array to delineate gene expression patterns in cattle selected for resistance to susceptibility to intestinal nematodes. Vet. Parasitol. 162, 106–115 (2009).
Kim, D. K. et al. Immune effects of dietary anethole on Eimeria acervuline infection. Poult. Sci. 92, 2625–2634 (2013).
Li-Weber, M. & Krammer, P. H. Regulation of IL4 gene expression by T cells and therapeutic perspectives. Nat. Rev. Immunol. 3, 534–543 (2003).
Jacobs, J. R., Greiner, S. P. & Bowdridge, S. A. Impaired interleukin-4 signalling promotes establishment of Haemonchus contortus in sheep. Parasite Immunol. 40, e12597. https://doi.org/10.1111/pim.12597 (2018).
Pernthaner, A., Cole, S. A., Morrison, L. & Hein, W. R. Increased expression of interleukin-5 (IL-5), IL-13, and tumor necrosis factor alpha genes in intestinal lymph cells of sheep selected for enhanced resistance to nematodes during infection with Trichostrongylus colubriformis. Infect. Immun. 73, 2175–2183 (2005).
Terawaki, S., Camosseto, V., Pierre, P. & Gatti, E. RUFY4: Immunity piggybacking on autophagy?. Autophagy 12, 598–600 (2016).
Arijs, I. et al. Mucosal gene expression of antimicrobial peptides in inflammatory bowel disease before and after first infliximab treatment. PLoS ONE 4, 7984. https://doi.org/10.1371/journal.pone.0007984 (2009).
Nagaraj, S. H. et al. Proteomic analysis of the abomasal mucosal response following infection by the nematode, Haemonchus contortus, in genetically resistant and susceptible sheep. J. Proteom. 75, 2141–2152 (2012).
Williams, E. J. et al. Distribution of the interleukin-8 receptors, CXCR1 and CXCR2, in inflamed gut tissue. J. Pathol 192, 533–539 (2000).
Woodbury, R. G. et al. Mucosal mast cells are functionally active during spontaneous expulsion of intestinal nematode infections in rat. Nature 312, 450–452 (1984).
Finkelman, F. D. et al. Interleukin-4-and interleukin-13-mediated host protection against intestinal nematode parasites. Immunol. Rev. 201, 139–155 (2004).
McRae, K. M., McEwan, J. C., Dodds, K. G. & Gemmell, N. J. Signatures of selection in sheep bred for resistance or susceptibility to gastrointestinal nematodes. BMC Genom. 15, 637. https://doi.org/10.1186/1471-2164-15-637 (2014).
Khan, W. I. & Collins, S. M. Immune-mediated alteration in gut physiology and its role in host defence in nematode infection. Parasite Immunol. 26, 319–326 (2004).
Komiya, T., Tanigawa, Y. & Hirohashi, S. Cloning of the novel gene intelectin, which is expressed in intestinal paneth cells in mice. Biochem. Biophys. Res. Commun. 251, 759–762 (1998).
French, A. T. et al. The expression of intelectin in sheep goblet cells and upregulation by interleukin-4. Vet. Immunol. Immunopathol. 120, 41–46 (2007).
Pemberton, A. D. et al. Innate BALB/c enteric epithelial responses to Trichinella spiralis: Inducible expression of a novel goblet cell lectin, intelectin-2, and its natural deletion in C57BL/10 mice. J. Immunol. 173, 1894–1901 (2004).
Voehringer, D. et al. Nippostrongylus brasiliensis: identification of intelectin-1 and -2 as Stat6-dependent genes expressed in lung and intestine during infection. Exp. Parasitol. 116, 458–466 (2007).
Athanasiadou, S. et al. Proteomic approach to identify candidate effector molecules during the in vitro immune exclusion of infective Teladorsagia circumcincta in the abomasum of sheep. Vet. Res. 39, 58. https://doi.org/10.1051/vetres:2008035 (2008).
Chen, F. et al. An essential role for TH2-type responses in limiting acute tissue damage during experimental helminth infection. Nat. Med. 18, 260. https://doi.org/10.1038/nm.2628 (2012).
Song, X. et al. Growth factor FGF2 cooperates with interleukin-17 to repair intestinal epithelial damage. Immunity 43, 488–501 (2015).
Álvarez, I. et al. Identification of genomic regions and candidate genes of functional importance for gastrointestinal parasite resistance traits in Djallonké sheep of Burkina Faso. Arch. Anim. Breed 62, 313–323 (2019).
Friedmann, E. et al. SPPL2a and SPPL2b promote intramembrane proteolysis of TNFα in activated dendritic cells to trigger IL-12 production. Nat. Cell Biol. 8, 843–848 (2006).
Stehling, O. et al. Human CIA2A (FAM96A) and CIA2B (FAM96B) integrate maturation of different subsets of cytosolic-nuclear iron-sulfur proteins and iron homeostasis. Cell Metab. 18, 187–198 (2013).
Boulton, K. et al. Phenotypic and genetic variation in the response of chickens to Eimeria tenella induced coccidiosis. Genet. Sel. Evol. 50, 63. https://doi.org/10.1186/s12711-018-0433-7 (2018).
Kipper, M., Andretta, I., Monteiro, S. G., Lovatto, P. A. & Lehnen, C. R. Meta-analysis of the effects of endoparasites on pig performance. Vet. Parasitol. 181, 316–320 (2011).
Goater, C. P., Semlitsch, R. D. & Bernasconi, M. V. Effects of body size and parasite infection on the locomotory performance of juvenile toads, Bufo bufo. Oikos 66, 129–136 (1993).
Hansen, J. & Perry, B. The Epidemiology, Diagnosis, and Control of Helminth Parasites of Ruminants 4th edn. (International Laboratory for Research on Animal Diseases, 1994).
Acknowledgements
We would like to thank all the farmers whose animals were sampled. This study was supported in part by the CGIAR Research Program for Livestock (Livestock CRP) under the Genetics Flagship. We acknowledge financial support from the donors to the CGIAR. The work was partialy supported by the Laboratoire d'Épidémiologie des Infections Enzootiques des Herbivores en Tunisie: Application à la Lutte (Ministère de l'Enseignement Supérieur et de la Recherche Scientifique, Tunisia; LR16AGR01).
Author information
Authors and Affiliations
Contributions
G.M., M.R., A.H., B.R. and J.M.M. conceived the study. R.M. carried out the field work including sampling under supervision of G.M. A.M.A. analysed the data under supervision from J.M.M. A.M.A. and J.M.M. wrote the manuscript. All authors read and approved the manuscript.
Corresponding author
Ethics declarations
Competing interests
The authors declare no competing interests.
Additional information
Publisher's note
Springer Nature remains neutral with regard to jurisdictional claims in published maps and institutional affiliations.
Supplementary Information
Rights and permissions
Open Access This article is licensed under a Creative Commons Attribution 4.0 International License, which permits use, sharing, adaptation, distribution and reproduction in any medium or format, as long as you give appropriate credit to the original author(s) and the source, provide a link to the Creative Commons licence, and indicate if changes were made. The images or other third party material in this article are included in the article's Creative Commons licence, unless indicated otherwise in a credit line to the material. If material is not included in the article's Creative Commons licence and your intended use is not permitted by statutory regulation or exceeds the permitted use, you will need to obtain permission directly from the copyright holder. To view a copy of this licence, visit http://creativecommons.org/licenses/by/4.0/.
About this article
Cite this article
Ahbara, A.M., Rouatbi, M., Gharbi, M. et al. Genome-wide insights on gastrointestinal nematode resistance in autochthonous Tunisian sheep. Sci Rep 11, 9250 (2021). https://doi.org/10.1038/s41598-021-88501-3
Received:
Accepted:
Published:
DOI: https://doi.org/10.1038/s41598-021-88501-3
- Springer Nature Limited
This article is cited by
-
Genomic heterozygosity is associated with parasite abundance, but the effects are not mediated by host condition
Evolutionary Ecology (2023)
-
Interaction networks and pathway analysis of genetic resistance to gastrointestinal nematodes in sheep
Tropical Animal Health and Production (2023)
-
Genome variation in tick infestation and cryptic divergence in Tunisian indigenous sheep
BMC Genomics (2022)