Abstract
Purpose
Fabry disease (FD) is a rare, X-linked, lysosomal storage disease characterized by great variability in clinical presentation and progressive multisystemic organ damage. Lack of awareness of FD and frequent misdiagnoses cause long diagnostic delays. To address the urgent need for earlier diagnosis, we created an online, risk-assessment scoring tool, the FDrisk, for predicting an individual’s risk for FD and prompting diagnostic testing and clinical evaluation.
Methods
Utilizing electronic health records, data were collected retrospectively from randomly selected, deidentified patients with FD treated at the Emory Lysosomal Storage Disease Center. Deidentified, negative controls were randomly selected from the Fabry Disease Diagnostic Testing and Education project database, a program within the American Association of Kidney Patients Center for Patient Education and Research. Diagnosis of FD was documented by evidence of a pathogenic variant in GLA and/or an abnormal level of leukocyte α-Gal A. Thirty characteristic clinical features of FD were initially identified and subsequently curated into 16 clinical covariates used as predictors for the risk of FD. An overall prediction model and two sex-specific prediction models were built. Two-hundred and sixty samples (130 cases, 130 controls) were used to train the risk prediction models. One-hundred and ninety-seven independent samples (30 cases, 167 controls) were used for testing model performance. Prediction accuracy was evaluated using a threshold of 0.5 to determine a predicted case vs. control.
Results
The overall risk prediction model demonstrated 80% sensitivity, 83.8% specificity, and positive predictive value of 47.1%. The male model demonstrated 75% sensitivity, 95.8% specificity, and positive predictive value of 75%. The female model demonstrated 83.3% sensitivity, 81.3% specificity, and positive predictive value of 45.5%. Patients with risk scores at or above 50% are categorized as “at risk” for FD and should be sent for diagnostic testing.
Conclusion
We have developed a statistical risk prediction model, the FDrisk, a validated, clinician-friendly, online, risk-assessment scoring tool for predicting an individual’s risk for FD and prompting diagnostic testing and clinical evaluation. As an easily accessible, user-friendly scoring tool, we believe implementing the FDrisk will significantly decrease the time to diagnosis and allow earlier initiation of FD-specific therapy.
Similar content being viewed by others
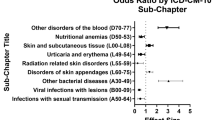
Avoid common mistakes on your manuscript.
Introduction
Fabry disease (FD) (OMIM no. 301500) is a rare, X-linked, lysosomal storage disease caused by pathogenic variants in the GLA gene which result in an absence or severe reduction of the lysosomal enzyme α-galactosidase (α-Gal A, EC 3.2.1.22). This leads to an excess of glycosphingolipids, mainly globotriaosylceramide (Gb3/GL3) and its deacylated form globotriaosylsphingosine (lysoGb3/lysoGL3) [1, 2] which, along with the associated downstream inflammatory mechanisms [3], cause progressive multisystemic organ damage. Of note, despite being an X-linked condition, females are often significantly affected due to a combination of skewed X-chromosome inactivation, clonal expansion, and somatic mosaicism. As such, females experience severity of disease ranging from asymptomatic to severe, resembling that which can be observed in males [4,5,6,7].
FD is categorized into two phenotypes: classic and non-classic (later-onset), each exhibiting a wide phenotypic spectrum. The more severe classic form generally begins in childhood and exhibits a more consistent and insidious pattern of disease progression [5]. Presenting signs and symptoms often include neuropathic pain, heat intolerance, and gastrointestinal issues [8, 9]. Additional signs and symptoms include the following: hypohidrosis progressing to anhidrosis, dermal angiokeratoma (a skin finding commonly found in FD) [5], proteinuria, bradycardia, and cornea verticillata (a characteristic corneal opacity highly suggestive of FD). Without initiation of FD-specific therapy, the disease may progress to chronic renal disease, left ventricular hypertrophy (LVH), arrhythmias, hearing loss, transient ischemic attacks (TIA), stroke, and ultimately premature death [5]. Individuals with the more prevalent nonclassic phenotypes have varying levels of residual α-Gal A activity and therefore experience a more variable disease course with symptoms that typically emerge after childhood and include LVH, arrhythmias, and/or markedly decreased glomerular filtration rate [5, 10]. FD occurs in people of all ages in diverse ethnic, racial, and demographic groups. Estimates of incidence vary depending on the screening methods and populations studied [11]. Initially, it was estimated that classic FD affected 1:117,000 live male births globally [12]; however, the first newborn screening studies conducted in the USA and internationally demonstrated a much higher prevalence of 1:1250 to 1:11,854 with a much higher representation by patients with nonclassic FD [9]. Numerous screening studies of high-risk populations have identified FD in patients with end-stage renal disease [13,14,15,16], cardiac hypertrophy [16,17,18,19], unexplained left ventricular hypertrophy [20, 21], and stroke [22,23,24,25]. It is significant that many screening projects utilized only α-Gal A to identify at-risk patients which reportedly can miss detection of 30–40% of females with FD [5]. In addition to high-risk population screening studies and newborn screening, artificial intelligence (AI) methods are currently being developed to screen for FD [26, 27].
Compared to the general population, without treatment, life expectancy for people with FD decreases by 20 and 10 years in males and females respectively [10]. The current therapeutic options for FD vary in availability by country but include the following: intravenous enzyme replacement therapy (ERT) with recombinant α-Gal A [agalsidase alpha (Replagal®, Takeda), agalsidase beta (Fabrazyme®, Sanofi), pegunigalsidase alfa-iwx (Elfabrio®, Protalix Biotherapeutics)], and the oral small molecule pharmacologic chaperone migalastat (Galafold®, Amicus Therapeutics) for those with amenable GLA variants. Several other treatments are in development including the following: second-generation ERT, moss-aGal (Greenovation Biotech/Eleva); substrate reduction therapies, venglustat (Sanofi) and lucerastat (Idorsia Pharmaceuticals); and mRNA and gene therapies, isaralgagene civaparvovec (ST-920, Sangamo) and 4D-310 (4D Molecular Therapeutics).
Like most rare diseases, there is a lack of awareness of FD. Additionally, there is great variability in clinical presentation, with many patients presenting with non-FD-specific renal and cardiovascular diseases. Without properly directed further investigation, patients often are misdiagnosed and endure long diagnostic delays [28].
To address the critical need for earlier diagnosis, we have developed a statistical risk prediction model, the FDrisk, which is a validated, easily accessible, clinician-friendly, online, risk-assessment scoring tool for predicting an individual’s risk for FD and prompting diagnostic testing and clinical evaluation.
Methods
This study received approval from the Emory University Institutional Review Board (IRB no. 00003599). Utilizing electronic health records, data were collected retrospectively from randomly selected, deidentified patients with confirmed FD treated at the Emory Lysosomal Storage Disease Center from 2001 through 2020. As a referral center of excellence for FD, the patients are from beyond the southeast region of the USA. The patients’ original clinical evaluations were undertaken by genetic counselors and medical geneticists with an expertise in FD. The diagnosis of FD was documented by evidence of a pathogenic variant in GLA and/or an abnormal level of leukocyte α-Gal A. Additional evidence of FD included the presence of symptoms consistent with FD and/or a family history of FD. For patients treated with ERT, information was only gathered for the time period previous to receiving treatment.
Based on the opinion of clinical experts in FD, i.e., healthcare providers with clinical and research experience working with more than 100 patients with FD over a decade of time, 30 characteristic clinical features of FD, including relevant demographic information, were initially identified. Subsequently, these features were curated into 16 clinical covariates used as predictors for the risk of FD (Table 1).
Retrospective chart review was conducted to identify FD cases and controls for this study. A total of 130 diagnosed FD cases were used as the FD case cohort for training FD risk prediction models. The FD case cohort ranges in age from 0 to 76 years old (a few patients were diagnosed prenatally or at birth) and is composed of 46 males and 84 females. Another 30 diagnosed FD cases (12 males and 18 females; ages ranging from 0 to 65 years old) were reserved for testing the FD risk prediction models.
Deidentified, FD-negative controls were randomly selected from the Fabry Disease Diagnostic Testing and Education project database, a program within the American Association of Kidney Patients (AAKP) Center for Patient Education and Research. Through this program, no-cost testing is available for individuals who have health issues seen most often in FD and/or have a known family history. Individuals complete a self-response questionnaire indicating the presence or absence of 16 possible FD-related signs/symptoms. In addition, family members with a confirmed diagnosis of FD are identified.
Clinical data for participants in the AAKP program, who are listed in the database for the time period from 2007 to 2015, were retrospectively reviewed. The available database only listed people with a positive family history of FD. A total of 297 controls with complete observations of these 16 clinical features were selected. These include 130 controls (46 males and 84 females matched with the same gender ratio and sample size of the case group, ages ranging from 1 to 81 years old) for model training, and 167 controls (71 males and 96 females, ages ranging from 1 to 96 years old) for model testing.
In order to harmonize clinical features of the FD case and control groups, the more specifically described clinical features collected for the FD case cohort were grouped into the16 broader pre-established AAKP clinical categories. For example, for the AAKP clinical category “cardiac disease,” it was necessary to combine the following individual cardiac features found in the FD case group: “arrhythmia,” “bradycardia,” “conduction abnormality,” “left ventricular hypertrophy,” and “cardiac valvulopathy” (Table 1). When identical clinical variables were not available, data was collected as specifically as possible. Note that several variables were identical, e.g., “tinnitus.”
Statistical methods
Training and testing samples were randomly selected from the whole cohort. In the training cohort, we first randomly selected 130 cases from all cases in our cohort and randomly selected the same number of controls (130) as the cases. The training cohort with 260 samples (168 females, 92 males, equal case/control ratios for females and males) was used to train the risk prediction models for FD [29]. A total of 15 clinical covariates (except the sex variable) were considered to fit the sex-specific risk prediction models, and a total of 16 clinical covariates were considered to fit the risk prediction model for all samples. A small percentage of missing values were observed in the FD cases and were imputed to the median values of FD cases of the corresponding covariate. The missing rates for FD cases were < 4% for clinical variables except proteinuria (17%) and cornea (36%).
Because all members of the control cohort had a positive family history of FD while not all members of the FD case cohort had a positive family history, including the family history as a predictive variable in model training would have led to a negative effect size, which would have suggested that a sample with a positive family history of FD has less risk than one without. To avoid such bias caused by training data curation, the covariate of family history of FD was excluded from our model training with plans to annotate the final tool with a note that all patients with a family history of FD should be considered “at risk.”
Logistic regression models with elastic-net penalty [30] were trained for FD cases vs. controls, respectively, for all samples, female samples, and male samples in the training cohort (n = 260). The elastic-net penalty is a weighted combination of L1 (lasso) [31] and L2 (ridge) [32] penalty with one parameter alpha denoting the proportion of L1 (lasso) penalty and another parameter lambda denoting the shared penalty magnitude. The L1 (lasso) penalty helped select predictive covariates, while the L2 (ridge) penalty helped handle correlated covariates. Both parameters were tuned by 10-fold cross validation [33] where the values leading to the highest classification accuracy by 10-fold cross-validations were used to train the risk prediction models.
A test cohort with 197 independent samples (30 cases and 167 controls, 114 females, 83 males) was used to test the model performance. Probabilities of having FD were predicted per sample by these fitted logistic models for test samples. Predicted probabilities were obtained for all test samples by using the risk prediction model fitted using all samples. Additionally, another set of predicted probabilities of female test samples was obtained by using the risk prediction model fitted using only female training samples, while another set of predicted probabilities of male test samples was obtained by using only male training samples.
The prediction performance was first evaluated by using the prediction area under curve (AUC) values of the receiver operating characteristic (ROC) curves [34]. The AUC value indicates the probability that a randomly selected true case will have higher risk probability than a randomly selected true control. A threshold of 0.5 was selected to determine a predictive “case” for those with predicted probability ≥ 0.5 and a predictive “control” for those with predicted probability < 0.5. By applying this threshold, the prediction accuracy of the overall risk prediction model and two sex-specific risk prediction models was evaluated in independent test samples using the overall prediction accuracy, sensitivity, and specificity. Test results were further stratified by age.
Results
All models performed very well, with ROC curves and AUC values calculated to determine the performance of each model: full analysis set (FAS) 0.919 (Fig. 1), male model 0.968, and female model 0.91 (Fig. 2).
Here, although high AUC values were partly due to a large proportion of controls in the test cohort, the prediction model with test AUC > 0.9 is still considered to be very accurate. Higher AUC values, generally ranging between 0.5 and 1, indicate higher prediction accuracy by the corresponding risk prediction model.
A risk prediction threshold of ≥ 0.50, which was chosen to determine if a patient is at risk for FD, was shown to provide the best balance between sensitivity, specificity, and positive predictive value in the test results (Fig. 3 and Fig. 4).
That is, a risk score of 50% or greater is categorized as “at risk” for FD. In the test cohort, the overall risk prediction model demonstrated 80% sensitivity, 83.8% specificity, and a positive predictive value of 47.1%; the male-specific risk prediction model demonstrated 75% sensitivity, 95.8% specificity, and a positive predictive value of 75%; and the female-specific risk predication model demonstrated 83.3% sensitivity, 81.3% specificity, and positive predictive value of 45.5%. Stratification of the test cohort by age ≥ 18 years old (n = 136) or < 18 years old (n = 61) with the FAS model showed a greater AUC of 0.964, a greater specificity of 94.1%, and a greater accuracy of 91.8% in the < 18-year-old age group compared with an AUC of 0.912, specificity of 79.3%, and accuracy of 79.4% in the ≥ 18-year-old age group. Comparable sensitivity of 80% was shown for both age groups. The finding of greater AUC, specificity, and accuracy in the < 18-year-old age group is consistent with higher likelihood that pediatric patients with the characteristic features of FD have the disease.
The estimated coefficients of predictive clinical variables were determined for the overall FAS model, as well as for the male- and female-specific models (Fig. 5).
The magnitude of the coefficients reflects the magnitude of influence of the predicted risk probability by the corresponding clinical variables, i.e., a variable’s effect size. Positive coefficients mean that the present or larger values of the corresponding clinical variables will increase the risk probability, while negative coefficients indicate that the present or larger values of the corresponding clinical variables will decrease the risk probability. All clinical variables with non-zero coefficient values would contribute to the predicted risk probability. A clinical variable with a high positive coefficient, such as cornea verticillata, reflects the disease-specific manifestations of FD. The clinical variables with coefficients of smaller magnitudes are not necessarily significantly associated with FD but would be more concerning when seen at an earlier age or in combination with features that suggest a diagnosis.
Discussion
Clinicians in both general practice and many nongenetic focused sub-specialties are likely to be caring for people with undiagnosed FD. However, lack of awareness of FD, difficulty recognizing the clustering of pathologies, vague subjective complaints, and the lack of a detailed family history can cause long diagnostic delays for patients with FD and their affected family members. Cascade testing after diagnosing a patient with FD is critical since, on average, each patient with FD has at least five family members who also have FD [35]. Additionally, it needs to be recognized that females can be severely affected by FD, and that the number of females with FD has been profoundly underestimated partly due to limited testing of only α-Gal A without follow-up molecular testing of the GLA gene [5].
With availability of effective treatment and support options, increasing understanding of FD and the significant impact on quality of life [36], it has become even more imperative that people with FD are identified earlier. To address this need, we developed a statistical risk-prediction model, the FDrisk, which is a validated, easily accessible, and clinician-friendly, online, risk-assessment scoring tool for predicting an individual’s risk for FD and prompting diagnostic testing and clinical evaluation (Fig. 6).
As a result of a collaborative effort, the FDrisk tool can be accessed at FDrisk.org which is hosted and maintained by the ThinkGenetic Foundation, Inc. (thinkgenetic.org), a nonprofit organization dedicated to creating practical, informational content on genetic diseases, and useful resources. On the web page, the FDrisk appears as a real-time graphic display of the risk score which is generated by answering 16 clinical questions requiring a yes/no response. The risk score is clearly expressed as a percentage of predicted risk probability and is marked on the graph with a vertical red line. Anyone generating a risk score of 50% or greater should be sent for clinical evaluation and diagnostic testing, which is available through sponsored testing programs and standard laboratories. Although the most important risk factor of family history was unable to be included in the analysis, a notation on the tool reminds clinicians that anyone with a family history of FD is considered to be at high risk for FD, no matter the risk score, and should be sent for diagnostic testing.
In addition to the FDrisk, screening tools using AI methodology are currently in development and have great future potential to identify undiagnosed patients with FD. One such tool, the OM1 Patient Finder™ (OM1 Inc., Boston, MA, USA), uses deidentified longitudinal health history data and predictive analytics to identify patients most likely to qualify for, and participate in, specific trials [37]. This includes patients who may have relevant but undiagnosed disease. Using data, e.g., medication history, prescription information, laboratory results, symptoms and signs, procedures, and diagnoses extracted from patient-level health care claims and electronic medical records Jefferies et al. [26], examined the performance of the OM1 Patient Finder in identifying patients with FD by looking at the phenotypic patterns of a study population of 1,004,978 patients which included 4978 patients with confirmed FD. They concluded that the tool has “a very strong analytic performance” in identifying patients with FD, and that it may contribute to increasing the diagnosis of FD.
Michalski et al. [27] developed a decision-support scoring system for FD, using natural language processing (NLP), to evaluate the electronic health records of 19,385 patients, including 13 with FD from a multi-hospital Polish healthcare system. Based on physician opinion, 13 curated clinical features of FD were chosen and scored. The FD risk score was determined by the sum of the scores for each clinical feature. A patient with a risk score of ≥ 4 was further evaluated. One patient in the control group with a score of eight as well as one patient from the study group with a score of three were ultimately diagnosed with FD. Although the scoring system achieved an AUC of 0.998, there were several limitations cited by the authors which impacted the accuracy and discrimination power in identifying patients with FD. The authors concluded that further development and testing with a larger and more diverse patient population are needed.
When studying rare diseases, it is often a challenge to curate cohorts with hundreds of samples. However, in this retrospective chart review, a relatively large number of patients with confirmed FD and relevant clinical data were identified [38]. Nonetheless. there are a number of limitations given our available data. First, in order to match clinical features for the analysis, several detailed individual features in the case cohort were grouped to fit into some of the more broadly defined pre-established categories of clinical features in the AAKP control cohort.
Second, due to limitations in the AAKP database, all the patients in the control cohort had a positive family history of FD. Since not all patients in the case cohort had positive family history of FD, family history was excluded in the final analysis to avoid bias caused by training data curation. Including family history would have falsely indicated that those with a positive family history have less risk of having FD. Nonetheless, as instructed in the tool, anyone with a family history of FD needs to be tested no matter the resulting risk score. It is important to note that all three prediction models (full analysis set, male and female) performed very well as determined using ROC curves and AUC values. The resulting AUC values, greater than 0.9 for all three models, indicate a high prediction accuracy by these risk prediction models.
The third limitation, ascertainment bias, is present since both the case and control cohorts were collected from single centers.
Future directions are to pilot the FDrisk in clinics such as cardiology, nephrology, and neurology to determine its clinical efficacy and promote awareness of both FD and this tool. Depending on these results, a “next-generation” tool may be generated utilizing an expanded training data set which would include case and control databases with additional equally matched clinical variables, including family history.
At this time, the FDrisk, as an open and easily accessible, user-friendly tool, can generate a risk score in the clinic using information directly obtained from the patient. With its ease of use, and availability online, investigators may choose to study the clinical efficacy of the FDrisk in their own populations. Eventually, the FDrisk tool could be used in the screening of a large number of patients by applying it to data captured from the electronic medical records of selected groups in concert with an AI-based model.
Conclusion
We believe that the implementation of this FDrisk tool will have a positive impact on people with FD and their families by increasing the number of people screened for FD, decreasing the frequency of missed and misdiagnoses, and leading to earlier and correct diagnosis. Once identified, patients can be appropriately treated by clinicians experienced in the care of individuals with FD along with a team of multidisciplinary specialists who can provide appropriate drug treatment, organ-specific management, treatment of variable adjuvant conditions, genetic counseling, and psychosocial support. Initiating earlier disease-specific therapy could slow disease progression and development of complications [39, 40] and ultimately make a meaningful difference in the lives of people living with FD.
Availability of data and materials
The data sets analyzed during the current study are not publicly available because they are confidential patient records, protected by the Health Insurance Portability and Accountability Act, but the data may be available from the corresponding author on reasonable request.
References
Brady RO, Gal AE, Bradley RM, Martensson E, Warshaw AL, Laster L. Enzymatic defect in Fabry’s disease. Ceramidetrihexosidase deficiency. N Engl J Med. 1967;276:1163–7. https://doi.org/10.1056/NEJM196705252762101.
Kint JA. Fabry’s disease: alpha-galactosidase deficiency. Science. 1970;167:1268e9. https://doi.org/10.1126/science.167.3922.1268.
Rozenfeld P, Feriozzi S. Contribution of inflammatory pathways to Fabry disease pathogenesis. Mol Genet Metab. 2017;122(3):19–27. https://doi.org/10.1016/j.ymgme.2017.09.004.
Najafian B, Silvestroni A, Sokolovskiy A, Tøndel C, Svarstad E, et al. A novel unbiased method reveals progressive podocyte globotriaosylceramide accumulation and loss with age in females with Fabry disease. Kidney Int. 2022;102(1):173–82. https://doi.org/10.1016/j.kint.2022.03.023.
Ortiz A, Germain DP, Desnick RJ, Politei J, Mauer M, Burlina A, et al. Fabry disease revisited: management and treatment recommendations for adult patients. Mol Genet Metab. 2018;123(4):416–27. https://doi.org/10.1016/j.ymgme.2018.02.014.
Street NJ, Yi MS, Bailey LA, Hopkin RJ. Comparison of health-related quality of life between heterozygous women with Fabry disease, a healthy control population, and patients with other chronic disease. Genet Med. 2006 Jun;8(6):346–53. https://doi.org/10.1097/01.gim.0000223545.63012.5a. PMID: 16778596.
Wang RY, Lelis A, Mirocha J, Wilcox WR. Heterozygous Fabry women are not just carriers, but have a significant burden of disease and impaired quality of life. Genet Med. 2007;9:34–45. https://doi.org/10.1097/gim.0b013e31802d8321.
Hopkin RJ, Jefferies JL, Laney DA, Lawson VH, Mauer M, Taylor MR, et al. Fabry pediatric expert panel. The management and treatment of children with Fabry disease: a United States-based perspective. Mol Genet Metab. 2016;117(2):104–13. https://doi.org/10.1016/j.ymgme.2015.10.007.
Laney DA, Peck DS, Atherton AM, Manwaring LP, Christensen KM, Shankar SP, et al. Fabry disease in infancy and early childhood: a systematic literature review. Genet Med. 2015;17(5):323–30. https://doi.org/10.1038/gim.2014.120.
Germain DP, Fouilhoux A, Decramer S, Tardieu M, Pillet P, Fila M, et al. Consensus recommendations for diagnosis, management and treatment of Fabry disease in paediatric patients. Clin Genet. 2019b;96(2):107–17. https://doi.org/10.1111/cge.13546.
Doheny D, Srinivasan R, Pagant S, Chen B, Yasuda M, Desnick RJ. Fabry disease: prevalence of affected males and heterozygotes with pathogenic GLA mutations identified by screening renal, cardiac and stroke clinics, 1995-2017. J Med Genet. 2018;55(4):261–8. https://doi.org/10.1136/jmedgenet-2017-105080.
Meikle PJ, Hopwood JJ, Clague AE, Carey WF. Prevalence of lysosomal storage disorders. JAMA. 1999;281(3):249–54. https://doi.org/10.1001/jama.281.3.249.
Ichinose M, Nakayama M, Ohashi T, Utsunomiya Y, Kobayashi M, Eto Y. Significance of screening for Fabry disease among male dialysis patients. Clin Exp Nephrol. 2005;9(3):228–32. https://doi.org/10.1007/s10157-005-0369-4.
Kotanko P, Kramar R, Devrnja D, Paschke E, Voigtländer T, Auinger M, et al. Results of a nationwide screening for Anderson-Fabry disease among dialysis patients. J Am Soc Nephrol. 2004;15(5):1323–9. https://doi.org/10.1097/01.asn.0000124671.61963. 1e. Erratum in: J Am Soc Nephrol. 2004 Aug;15(8):1a. Erratum in: J Am Soc Nephrol. 2004 Sep;15(9):A4. PMID: 15100373.
Moiseev S, Fomin V, Savostyanov K, Pushkov A, Moiseev A, Svistunov A, et al. The prevalence and clinical features of Fabry disease in hemodialysis patients: Russian nationwide Fabry dialysis screening program. Nephron. 2019;141(4):249–55. https://doi.org/10.1159/000495886.
Nakao S, Takenaka T, Maeda M, Kodama C, Tanaka A, Tahara M, et al. An atypical variant of Fabry’s disease in men with left ventricular hypertrophy. N Engl J Med. 1995;333(5):288–93. https://doi.org/10.1056/NEJM199508033330504.
Chimenti C, Pieroni M, Morgante E, Antuzzi D, Russo A, Russo MA, et al. Prevalence of Fabry disease in female patients with late-onset hypertrophic cardiomyopathy. Circulation. 2004;110(9):1047–53. https://doi.org/10.1161/01.CIR.0000139847.74101.03.
Monserrat L, Gimeno-Blanes JR, Marín F, Hermida-Prieto M, García-Honrubia A, Pérez I, et al. Prevalence of Fabry disease in a cohort of 508 unrelated patients with hypertrophic cardiomyopathy. J Am Coll Cardiol. 2007;50(25):2399–403. https://doi.org/10.1016/j.jacc.2007.06.062.
Sachdev B, Takenaka T, Teraguchi H, Tei C, Lee P, McKenna WJ, et al. Prevalence of Anderson-Fabry disease in male patients with late onset hypertrophic cardiomyopathy. Circulation. 2002;105(12):1407–11. https://doi.org/10.1161/01.cir.0000012626.81324.38. PMID: 11914245.
Ackerman MJ, Landstrom AP. Detection of subclinical Fabry disease in patients presenting with hypertrophic cardiomyopathy. J Am Coll Cardiol. 2007;50(25):2404–5. https://doi.org/10.1016/j.jacc.2007.09.022.
Sadasivan C, Chow JTY, Sheng B, Chan DKH, Fan Y, Choi PCL, et al. Screening for Fabry disease in patients with unexplained left ventricular hypertrophy. PLoS One. 2020;15(9):e0239675. https://doi.org/10.1371/journal.pone.0239675.
Brouns R, Thijs V, Eyskens F, van den Broeck BS, van Broeckhoven C, et al. Belgian Fabry study prevalence of Fabry disease in a cohort of 1000 young patients with cerebrovascular disease. Stroke. 2010;41(5):863–8. https://doi.org/10.1161/STROKEAHA.110.579409.
Linthorst GE, Bouwman MG, Wijburg FA, Aerts JM, Poorthuis BJ, Hollak CE. Screening for Fabry disease in high-risk populations: a systematic review. J Med Genet. 2010;47(4):217–22. https://doi.org/10.1136/jmg.2009.072116.
Rolfs A, Böttcher T, Zschiesche M, Morris P, Winchester B, Bauer P, et al. Prevalence of Fabry disease in patients with cryptogenic stroke: a prospective study. Lancet. 2005;366(9499):1794–6. https://doi.org/10.1016/S0140-6736(05)67635-0. Erratum in: Lancet. 2006 Dec 23;368(9554):2210. PMID: 16298216.
Wozniak MA, Kittner SJ, Tuhrim S. Frequency of unrecognized Fabry disease among young European-American and African-American men with first ischemic stroke. Stroke. 2010;41(1):78–81. https://doi.org/10.1161/STROKEAHA.109.558320.
Jefferies JL, Spencer AK, Heather AL, et al. A new approach to identifying patients with elevated risk for Fabry disease using a machine learning algorithm. Orphanet Journal of Rare Diseases. 2021;16:518. https://doi.org/10.1186/s13023-021-02150-3.
Michalski AA, Lis K, Stankiewicz J, Kloska SM, Sycz A, Dudziński M, et al. Supporting the diagnosis of Fabry disease using a natural language processing-based approach. J Clin Med. 2023;12(10):3599. https://doi.org/10.3390/jcm12103599.
Reisin R, Perrin A, García-Pavía P. Time delays in the diagnosis and treatment of Fabry disease. Int J Clin Pract. 2017;71(1) https://doi.org/10.1111/ijcp.12914.
Hastie T, Tibshirani R, Friedman JS. The Elements of Statistical Learning. 2 ed. New York: Springer-Verlag; 2009.
Zou H, Hastie T. Regularization and variable selection via the elastic net. Journal of the Royal Statistical Society: Series B (Statistical Methodology). 2005;67(2):301–20. https://doi.org/10.1111/j.1467-9868.2005.00503.x.
Tibshirani R. Regression shrinkage and selection via the lasso. J R Stat Soc Ser B Methodol. 1996;58(1):267–88. https://www.jstor.org/stable/2346178.
Hoerl AE, Kennard RW. Ridge regression: biased estimation for nonorthogonal problems. Technometrics. 2000;42(1):80–6. https://doi.org/10.2307/1271436.
Stone M. Cross-validatory choice and assessment of statistical predictions. J R Stat Soc Ser B Methodol. 1974;36(2):111–33. https://www.jstor.org/stable/2984809.
Linden A. Measuring diagnostic and predictive accuracy in disease management: an introduction to receiver operating characteristic (ROC) analysis. J Eval Clin Pract. 2006;12(2):132–9. https://doi.org/10.1111/j.1365-2753.2005.00598.x.
Laney DA, Fernhoff PM. Diagnosis of Fabry disease via analysis of family history. J Genet Couns. 2008;17(1):79–83. https://doi.org/10.1007/s10897-007-9128-x.
Morand O, Johnson J, Walter J, Atkinson L, Kline G, Frey A, et al. Symptoms and quality of life in patients with Fabry disease: results from an International Patient Survey. Adv Ther. 2019;36(10):2866–80. https://doi.org/10.1007/s12325-019-01061-x.
Laney DA, Dronen J, Jacob D, Rosen A, Lobel C, Yang JJ, et al. Development and implementation of an automated severity scoring system to identify patients at possible increased risk for ten lysosomal disorders. Mol Genet Metab. 2022;135:S70–1. https://doi.org/10.1016/j.ymgme.2021.11.178.
Hee SW, Willis A, Tudur Smith C, Day S, Miller F, Madan J, et al. Does the low prevalence affect the sample size of interventional clinical trials of rare diseases? An analysis of data from the aggregate analysis of clinicaltrials.gov. Orphanet J Rare Dis. 2017;12(1):44. https://doi.org/10.1186/s13023-017-0597-1.
Germain DP, Arad M, Burlina A, Elliott PM, Falissard B, Feldt-Rasmussen U, et al. The effect of enzyme replacement therapy on clinical outcomes in female patients with Fabry disease - a systematic literature review by a European panel of experts. Mol Genet Metab. 2019a;126(3):224–35. https://doi.org/10.1016/j.ymgme.2018.09.007.
McCafferty EH, Scott LJ. Migalastat: a review in Fabry disease. Drugs. 2019;79:543–54. https://doi.org/10.1007/s40265-019-01090-4.
Acknowledgements
The authors greatly thank Robin Vinson for extensive administrative assistance. We also recognize all of the hardworking medical professionals and scientists striving to improve medical knowledge and outcomes in Fabry disease as well as the courageous patients and families who allow us the privilege of working with them.
Funding
J. Y., received a grant from NIH/NIGMS R35GM138313. ThinkGenetic Foundation, Inc. is a recipient of an unrestricted educational grant from Chiesi Global Rare Diseases, a portion of which was used for development of the online FD-risk scoring tool. The American Association of Kidney Patients’ (AAKP) Center for Patient Education and Research is funded via grants from Sanofi and Amicus Therapeutics.
Author information
Authors and Affiliations
Contributions
CJL, inspection of the manuscript, conception and design, analysis and interpretation of the data, drafting the article, and revising it critically for important intellectual content. DAL, inspection of the manuscript, conception and design, analysis and interpretation of the data, drafting the article, and revising it critically for important intellectual content. JY, inspection of the manuscript, conception and design, analysis and interpretation of the data, drafting the article, and revising it critically for important intellectual content. DJ, inspection of the manuscript, analysis and interpretation of data, and revising it critically for important intellectual content. AR, inspection of the manuscript, analysis and interpretation of the data, and revising it critically for important intellectual content. CZO, inspection of the manuscript; conception and design, analysis, and interpretation of the data; and revising it critically for important intellectual content. DC, inspection of the manuscript, analysis and interpretation of data, and revising it critically for important intellectual content. JD, inspection of the manuscript, analysis and interpretation of data, and revising it critically for important intellectual content.
Corresponding author
Ethics declarations
Ethics approval and consent to participate
This study received approval from the Emory University Institutional Review Board (IRB no. 00003599). Approval for consent was waived.
Consent for publication
Not applicable.
Competing interests
C. J. L. declares no competing interests. D. A. L., consults with Chiesi, Spark Therapeutics, Sanofi-Genzyme, Takeda, Protalix, and Amicus Therapeutics and Founder of ThinkGenetic, Inc. J. Y. declares no competing interests. D. J., CEO of ThinkGenetic, Inc.; director of ThinkGenetic Foundation, Inc., and stock options from ThinkGenetic, Inc. A. R., employee of ThinkGenetic Foundation, Inc. C. Z. O., volunteer advisor to ThinkGenetic, Inc.; received a monthly consulting fee between April 2020 and April 2021 from the Takeda Pharmaceuticals Medical Communications; received a stock grant in 2017 from ThinkGenetic, Inc. D. C.; and executive director of the American Association of Kidney Patients. J. D., owns stock options as an employee of ThinkGenetic, Inc.; board member of ThinkGenetic Foundation, Inc.; chair of the Sanofi Genzyme North American Fabry Registry Board; and stock options ThinkGenetic, Inc.
Additional information
Publisher’s Note
Springer Nature remains neutral with regard to jurisdictional claims in published maps and institutional affiliations.
Rights and permissions
Open Access This article is licensed under a Creative Commons Attribution 4.0 International License, which permits use, sharing, adaptation, distribution and reproduction in any medium or format, as long as you give appropriate credit to the original author(s) and the source, provide a link to the Creative Commons licence, and indicate if changes were made. The images or other third party material in this article are included in the article's Creative Commons licence, unless indicated otherwise in a credit line to the material. If material is not included in the article's Creative Commons licence and your intended use is not permitted by statutory regulation or exceeds the permitted use, you will need to obtain permission directly from the copyright holder. To view a copy of this licence, visit http://creativecommons.org/licenses/by/4.0/.
About this article
Cite this article
Lobel, C.J., Laney, D.A., Yang, J. et al. FDrisk: development of a validated risk assessment tool for Fabry disease utilizing electronic health record data. J Rare Dis 3, 2 (2024). https://doi.org/10.1007/s44162-023-00026-7
Received:
Accepted:
Published:
DOI: https://doi.org/10.1007/s44162-023-00026-7