Abstract
Keratinase is a robust enzyme that is produced in the presence of keratin substrates. This enzyme has been recognized for its applications in waste management, leather and detergent industries. Our group has isolated a potential keratinase producing strain of Bacillus aerius NSMk2 from poultry dump soil, and its hide dehairing and stain removal applications have been studied. Considering commercial applicability of keratinase, the present study reports the keratinase production in a stirred tank reactor (5 l). Central composite rotatable design of response surface methodology (RSM) was employed to study the effect of most influencing process variables, i.e., aeration (0.5–1.5 vvm), agitation (150–350 rpm) and incubation period (24–48 h) on keratinase production. The quadratic model predicted 15 experimental runs, and the influence of independent variables and their interaction on keratinase production were interpreted using analysis of variance (ANOVA) and t-test statistics. Coefficient of determination (R2) value close to 1 and Fisher F-value of 3743.77 showed good fit of experimental data to second-order polynomial equation. A reasonable agreement between experimental and predicted values showed the accuracy of deduced model. Applying the desirability function, aeration rate of 1.0 vvm, agitation rate of 276.88 rpm and incubation period of 33.68 h supported maximum keratinase production (318.38 U/ml). Confirmatory experiments were performed to evaluate the accuracy of desirability function. Maximum keratinase activity of 318.11 U/ml close to predicted value (318.38 U/ml) validates the model. The present study provides useful guidelines for large-scale production of keratinase that can be used for various commercial applications.
Article highlights
-
Keratinase production was optimized in a stirred tank reactor by RSM.
-
The influence of aeration, agitation and incubation period on keratinase production was studied.
-
A significant increase in keratinase production was observed at 1.0 vvm aeration and 276.88 rpm agitation after 33.68 h.
Similar content being viewed by others
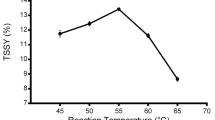
1 Introduction
Chicken feathers are an important by-product of poultry processing industries and account for about 5–7% of the body weight of chicken. It is estimated that several million tons of feathers are generated annually from poultry industries globally [1]. As per the reports of National Center for Manure and Animal Waste Management, USDA, poultry waste if allowed to accumulate in the absence of proper management results in emission of odorous gases such as ammonia, hydrogen sulfide and nitrous oxide and causes Newcastle diseases, air sacculitis and keratoconjunctivitis in humans [2, 3]. Feathers cannot be easily degraded under natural conditions due to the presence of recalcitrant keratin in its structure [4]. Keratin is a bioactive polymer that has a rigid structure consisting of α-helix and β-sheets linked through hydrogen and disulfide bonds. It is the main component of epidermis found in feathers, nail, hair, wool, horn, scales, hooves and external stratum of human skin [5]. A number of methods including physical or chemical treatment of feathers are currently being used. However, widespread use of these methods is limited due to harsh conditions, poor yield and loss of some essential amino acids [6]. Conversion of feathers into value-added products using keratinolytic microbes is of great interest to the researchers. Numerous bacteria, fungi and actinomycetes have been reported to produce keratinases that break down keratin structure to small peptides and amino acids [5]. Bacterial sources, particularly Bacillus sp., have gained much attention for keratinase production because of easy handling, scale-up feasibility and high commercial applicability [4]. Commercially available keratinase preparations, namely Versazyme (Bioresource International, Inc., USA), Valkerase (Bioresource International, Inc., USA) and Prionzyme M (Genecor International California, USA) are all based on KerA from Bacillus licheniformis. Versazyme is the first keratinase product marketed for use in poultry feed by BioResource International, Inc., USA, while Prionzyme M has been used for prion decontamination from medical and dental instruments [1]. Considering the promising applications of keratinases in various biotechnological sectors, these enzymes are expected to take over protease market especially in agroindustry and feed, biomedical, detergent, leather and textile industries in years to come [1, 5].
The utilization of chicken feather waste as fermentation substrate by keratinolytic microbes supports keratinase production and simultaneously offers a feasible microbial technology for efficient waste management [4]. In view of growing trends of keratinases, optimization of processing conditions such as incubation time, aeration and agitation is pivotal for improved and cost-effective keratinase production, because these parameters play a key role in promoting the growth of microorganisms for the secretion of enzymes [7]. Agitation and aeration increase oxygen solubility in medium for better growth of microbes during aerobic fermentation and subsequently influence enzyme production [8]. Fermentation time is another crucial parameter that affects production of industrially important enzymes and is governed by characteristics of culture medium and growth rate. One-variable-at-a-time approach is generally used to screen variables for enzyme production and allows studying the effect of a single variable on microbial processes. However, this approach is unable to detect mutual interactions in multivariable systems. Due to these shortcomings, statistical experimental designs have been recognized as ideal ways for bioprocess optimization studies.
Statistical optimization by response surface methodology is a compendium of statistical and mathematical techniques that have been widely used to study the interaction of multiple variables and their combined influence on enzyme production [9]. The main objective of RSM is to determine optimized operational conditions of the process or to determine a region that satisfies the operating specifications [10]. RSM uses quantitative data from appropriate experiments to determine and simultaneously solve multivariate equations and also reduces the number of experiments eventually. Furthermore, it offers a rapid and unfailing prediction of response, making it a beneficial option for experimental design [10].
Process optimization is generally performed in bench-scale bioreactors and then scaled up for commercial production. The objective of scale-up is to design and build a larger-scale system on the basis of results obtained at smaller scale. In our previous studies, keratinase production has been performed in shake-flask fermentations using minimal salt medium supplemented with chicken feathers as sole source of carbon [11]. Chicken feathers act as inducers for keratinase production and also contain a number of macronutrients and micronutrients that are released in culture medium during their decomposition by keratinolytic microbes [11]. The enzyme has been purified and characterized for physico-chemical properties. The enzyme was found to efficiently remove stains from cotton clothes and showed effective dehairing of goat skin under laboratory conditions [12]. In order to assess the commercial applicability of keratinase from Bacillus aerius NSMk2 in detergent industry and hide dehairing, large-scale production of enzyme is required. So the present study was conducted to develop an economical method using chicken feathers as substrate for keratinase production by Bacillus aerius NSMk2 in a stirred tank reactor. The effect of most influencing process variables (aeration, agitation and incubation time) on keratinase production was studied using central composite rotatable design (CCRD) of response surface methodology (RSM).
2 Materials and methods
2.1 Preparation of substrate
Chicken feathers were procured from local chicken shop in Patiala, Punjab. Feathers were washed extensively and dried to a constant weight. White chicken feathers were milled and used for production of keratinase [11].
2.2 Growth and maintenance of bacterial culture
Bacillus aerius NSMk2 was grown in nutrient broth containing (g/l): peptone 5.0, beef extract 1.5, yeast extract 1.5, sodium chloride 5.0 and pH 7.0. Culture was maintained on nutrient agar slants and stored at 4±1°C, until further use. The subculturing was performed at fortnight intervals.
2.3 Statistical optimization of keratinase production by Bacillus aerius NSMk2
Bacterial culture was grown in minimal salt medium (MSM) containing (g/l): sodium chloride 0.5, dipotassium hydrogen phosphate 0.4, potassium dihydrogen phosphate 0.3, magnesium sulfate 0.1, ammonium chloride 0.5 and crushed feather powder 10.0 as sole carbon source [11]. Culture was grown at 37 °C in a laboratory scale bioreactor (5 l, Bio-Spin-03A, Bio-age, India) fitted with automatic aeration, agitation and temperature control. Exhaust valve was fitted with a condenser to prevent losses by evaporation. Bioreactor agitation rate with a six blade Ruston type impeller was studied in terms of revolutions per min (rpm). Air was sterilized by passing through a hydrophobic membrane filter (0.45 μm, 25 mm, Millipore, USA). Aeration rate was measured by a rotameter in terms of volume of air per volume of medium per min (vvm).
The results of initial screening of process variables identified aeration, agitation and incubation time as significant parameters for keratinase production by Bacillus aerius NSMk2. Therefore, the effect of three independent variables, i.e., agitation (150–350 rpm), aeration (0.50–1.50 vvm) and incubation period (24–48 h) on keratinase production, was studied using CCRD of RSM using Design expert version 12.0.1.0. (Stat-Ease Inc., Minneapolis, USA). Keratinase activity (U/ml) was monitored as a dependent response. Maximum and minimum levels of three process variables are presented in Table 1. Each independent variable was studied at five coded levels, i.e., −1.414, −1.000, 0.000, +1.000, +1.414. CCRD consisting of four factorial points, six axial points and five replicates at the center point resulted in 15 different combinations of experiments. Analysis of variance (ANOVA) was used to determine the statistical significance of regression coefficients, and Fisher’s (F) test was used to determine the second-order polynomial equation:
where Xi, Xi2,… are independent variables which affect predicted response Y., βi, βii, and βij are regression coefficients of the model, β0 is constant coefficient and ɛ is standard error.
Lack of fit was applied to estimate the model terms. Significance of polynomial model equation was determined statistically by coefficient of determination (R2) and adjusted R2. The fitted polynomial equation was then expressed in terms of 3D response surface plots to illustrate relationship between responses and experimental levels of each independent variable. Parity plots were prepared to compare the experimental values with those predicted by the model.
2.4 Validation of model
Validation was performed by conducting experiments predicted by quadratic model under optimal conditions in terms of desirability function. A set of solutions was predicted by model on the basis of desirability range 0 ≤ di ≤ 1, where di is individual desirability function. Value of di = 1, if response is at its goal or target and di = 0, if response is outside an acceptable region [13]. Predicted experiments with high desirability of responses were performed under optimized conditions in a stirred tank reactor. Experimental and predicted values were compared to evaluate the suitability and validation of the model. All experiments were performed thrice to eliminate manual and methodical error.
2.5 Keratinase assay
Broth was decanted, contents were centrifuged (5000 rpm, 4 °C, 10 min) and keratinase activity was assayed in supernatant using crushed feathers as substrate [11]. To 0.2 ml of enzyme, 0.2 g crushed feathers in 1.8 ml Tris HCl buffer (10 mM, pH 8.0) were added and incubated at 45 °C in water bath for 1 h. Reaction was stopped by adding 2 ml of 10% trichloroacetic acid. The contents were centrifuged at 5000 rpm for 15 min to remove precipitated proteins and absorbance of supernatant at 280 nm (A280) was measured using a UV-VIS spectrophotometer (UV1800, Shimadzu, Japan). Concentration of enzyme that increase A280 by a factor of 0.01 as compared to control is considered as one unit of keratinase.
2.6 Statistical analysis
All experiments were conducted in triplicates and the results are expressed as mean ± standard deviation (SD).
3 Results
3.1 Statistical optimization of keratinase production by Bacillus aerius NSMK2 in a stirred tank reactor
In our previous reports, keratinase production has been carried out in shake-flask fermentations [11]. The enzyme was found to show stain removing and hide dehairing potential [12]. So to evaluate its commercial application in leather and detergent industries, large-scale production of enzyme is required. The present study was aimed to investigate the influence of aeration, agitation and incubation period on keratinase production by Bacillus aerius NSMk2 in a stirred tank reactor. Agitation (150–350 rpm), aeration (0.50–1.50 vvm) and incubation period (24–48 h) were selected as independent variables and their influence on keratinase production was studied in 15 experimental runs using CCRD of RSM. The results are shown in Table 2. Under optimized level of variables (aeration 1.0 vvm, agitation 250 rpm and incubation time 36 h), keratinase production was found to be 316.22 U/ml.
Variance analysis (ANOVA) was studied to investigate the significant levels of process parameters and their interactions for optimal keratinase production. ANOVA is a statistical tool to analyze multiple datasets and identify the parameter having most significant effect on the response [14]. Fisher ‘F’ value, Prob > F, degree of freedom (df) and lack of fit assist in the determination of significant interactions between independent variables and their influence on the desired response. F-value is the ratio of mean squares due to regression and mean squares due to error. Larger F-value and low p-value indicate greater significance of model terms [14]. High F-value (3743.77) for keratinase production validates the significance of the designed quadratic model (Table 3). Prob > F is the p-value for the designed model. If the value of prob > F is smaller than 0.05, the model terms are said to be relevant [15]. The resulting F-values for all individual, interaction and square terms of variables are high and their p-values are less than 0.05, which implies the significance of each variable (Table 3). On the basis of significance of all terms, following polynomial equation was generated in coded level:
In the above equation, A stands for aeration, B stands for agitation and C stands for incubation time. The positive sign of coefficients in Eq. (2) indicates synergistic effect and negative sign indicates their antagonistic effect.
Fitness of model was also analyzed using degree of freedom (df) that appears due to replicates, and the model with computed value of df between 3 and 4 is said to be significant. In the present model, df 4 for pure error indicates greater reliability of experiments with respect to predicted values. Lack of fit measures inability of the model to represent data at points that are excluded in regression [14]. A lack of fit value (p = 0.1321) observed in this study was insignificant which implies that the model equation could predict keratinase production at any interaction values of the variables. Goodness of fit can be ensured by determination coefficient (R2), predicted R2 and adjusted R2. The multiple correlation coefficients (R2) for keratinase production were found to be 0.99 that implies the model equation could explain 99% of the total variation and therefore suggests greater reliability of the model (Table 4). Predicted R2 value (0.9840) was in reasonable agreement with the adjusted R2 value (0.9996), suggesting a good adjustment between the observed and predicted values.
Adequate precision is signal-to-noise ratio and is used to test the adequacy of designed model. Its value should be greater than four and in the present study, adequate precision of 169.956 shows that the model has strong signal to be used for optimization [15]. Coefficient of variation (CV%) is standardized and dimensionless ratio of standard deviation to mean and is used to measure dispersion of probability or frequency distribution. Smaller CV% indicate greater reliability of model [15]. A relatively lower CV value (0.46%) recorded from this study indicates consistency of generated model for keratinase production. To study the distribution of experimental values with respect to predicted values, parity plot was prepared (Fig. 1). The figure clearly illustrates that the data points on the plot were reasonably distributed near the straight line, indicating an optimal fit between experimental and actual values, and that the underlying assumptions of above analysis are appropriate. The results also suggested that the selected quadratic model could adequately predict response variables for the experimental data.
3.2 Interactions between independent variables for keratinase production
The three-dimensional (3D) response surface plots were drawn to illustrate individual influence of process variables and their interaction on keratinase production by Bacillus aerius NSMK2 (Fig. 2a–c). Each 3D plot presented the effect of two variables, while the third one was held at middle level. Elliptical 3D response surface graphs confirmed significant interaction among process variables. Figure 2a represents a significant interaction between aeration and agitation. Varying aeration and agitation rates, a significant influence on keratinase production was observed. Increase in agitation up to 250 rpm and aeration rate up to 1.0 vvm enhanced keratinase production. Further increase in agitation and aeration rate repressed enzyme production. A similar interaction between incubation time and aeration was observed (Fig. 2b). However, keratinase production was almost constant, when incubation time and agitation rate were increased at a fixed aeration rate (Fig. 2c). Circular contour implied that mutual interaction between incubation time and agitation rate was insignificant.
3.3 Validation of model
The optimal conditions to maximize keratinase production were validated through numerical optimization of Design Expert Software using the desirability function. Numerical optimization was performed with the same range of aeration, agitation and incubation time which was used in CCRD experiments for optimal keratinase production. This approach involves the scale of desirability function ranging between d = 0, which suggests that the response is completely unacceptable, and d = 1, which suggests that the response is exactly the target value. The value of d increases between 0 and 1 as the desirability of corresponding response increases [13]. The most appropriate alternative optimization results offered by the system for each parameter according to selected parameters and desirability value 1.0 are shown in Table 5. Among all the three solutions suggested by the model, optimal conditions were found to be aeration 1.0 vvm, agitation 276.88 rpm and incubation time 33.68 h (Fig. 3). Maximum keratinase production predicted by the model was 318.38 U/ml. The predicted optimal conditions were checked experimentally by running three experiments under the same conditions, and keratinase production of 318.11 U/ml was observed. Good agreement between replicated results and predicted values strongly supported high reliability and validation of model for keratinase production.
3.4 Discussion
Keratinases are extracellular inducible proteases that hydrolyze crystalline and cross-linked keratin structures to produce a keratin hydrolysate which consists mainly of soluble proteins, peptides and amino acids [16]. Keratinase production can be significantly enhanced by modifying production medium and process parameters. Bacillus aerius NSMk2 has previously been identified as potential keratinase producer and medium constituents supporting maximum keratinase yield have been optimized [11]. Our previous studies reported potential of Bacillus aerius NSMk2 keratinase in detergent and dehairing applications [12]. In order to develop suitable technology for possible commercialization, large-scale production of keratinase is desired. Present investigation focused primarily on improvement of keratinase production by Bacillus aerius NSMk2 in a stirred tank reactor using response surface methodology (RSM). RSM has been widely accepted as a statistical tool to quantify the influence of factors on one or more responses [9]. Of the experimental design techniques used for process analysis and modeling, greater efficiency can be gained using central composite rotatable design (CCRD), where design points are arranged to maximize the data range, while reducing the number of experimental runs required. Commonly, this multifactor design is used to obtain an optimal response model in microbiology such as enzyme production in cell cultures by varying medium or culture conditions [10]. Therefore, CCRD was used to design the experiments in the present study.
Aeration and agitation are one of the critical biotic factors affecting microbial growth and fermentation process. Aeration (1.0 vvm) and agitation (250 rpm) showed a strong influence on keratinase production (Fig. 2a). Similar results have been reported for keratinase secretion from Meiothermus sp. [17], Arthrobacter sp. [18], Bacillus licheniformis [19] and Bacillus cereus [20]. In aerobic fermentation, oxygen is a limiting factor because of its low solubility and low volumetric mass transfer in bioreactors. Thus, rate of oxygen transfer is very essential for efficient microbial metabolic activities. In the present study, an increase in keratinase production was observed with increase in aeration rate from 0.3 to 1.0 vvm, beyond which keratinase yield was found to decrease. At high aeration rate in bioreactor, air flow along the shaft increases and impeller tends to get flooded. An impeller when surrounded by air column is no longer in contact with liquid resulting in poor mixing, reduced air dispersion and negligible oxygen transfer [21]. High aeration may also change physiological conditions such as foam formation that further affect viability of microbes. Excessive oxygen levels may also have detrimental effect on cells due to oxygen toxicity [8]. Lakshmi et al. [22] reported maximum keratinase production by Bacillus sp. under high aeration of 3 vvm, while maximum keratinase production from Bacillus subtilis has been reported at 1 vvm [23].
Efficient mass transfer is always associated with proper agitation in submerged fermentations. Agitation facilitates uniform distribution of nutrients to the cells, facilitates heat transfer, promotes oxygen transfer uniformly in fermentation broth and maintains cells in suspension that is prerequisite for high biomass production, which in turn enhances enzyme production [8]. A high agitation rate not only increases power consumption, but also creates shear stress and forms vortex which influences viability of shear sensitive microbes, enzyme stability and results in poor mass transfer [21]. The size of bubbles in bioreactor is largely dependent on turbulence generated by an impeller. Low agitation speed fails to generate sufficient turbulence and hold up air bubbles and consequently influences volumetric mass transfer due to high medium viscosity [8, 15]. Low oxygen solution rate subsequently results in stunted bacterial growth and low metabolite production [24]. Similarly, lower keratinase production due to poor mixing as well as lower dissolved oxygen has been reported from Bacillus sp. [25]. Laba et al. [26] and Mamangey et al. [27] reported keratinase production by Kocuria rhizophila and Azotobacter chroococcum at 180 rpm. Optimal keratinase production by Actinomadura keratinilytica [28], Bacillus amyloliquefaciens [29] and Chryseobacterium sediminis [30] has been reported at 200 rpm, while optimal keratinase production from Bacillus licheniformis has been reported at 250 rpm [31].
Incubation time is another important parameter for metabolite production. Keratinase is an inducible enzyme and it accumulates in the medium in the presence of a suitable inducer. The influence of incubation time can be seen from experimental run number 10 and 13 (Table 2). These runs have similar aeration and agitation rate but different incubation time. Enzyme levels keep on accumulating in the medium as cells divide and maximum keratinase production was observed (318.38 U/ml) after 33.68 h of incubation. This shows that enzyme is a primary metabolite, being produced by growing cells using chicken feathers as substrate. Thereafter, continuous reduction in keratinase production was observed which may be attributed to the exhaustion of nutrients, attainment of stationary phase by bacterial culture, and accumulation of by-products in medium such as toxins, inhibitors and proteases or catabolite repression of enzymes [18]. During the fermentation period, rheological properties of broth may change significantly due to increase in biomass and changes in morphological structure of keratin substrate [32]. Keratinase has been reported to degrade keratinous biomass faster with the simultaneous release of proteins, peptides and amino acids [16]. These secondary metabolites increase viscosity of liquid medium that subsequently influences mass transfer and reduces enzyme production [8]. The majority of studies reported optimal keratinase production within 36–48 h [19, 20].
3.5 Conclusion
Keratinases have multifarious biotechnological applications. To drive the myroids of applications, the use of techniques to optimize enzyme yield and lowering of production cost would be a continuous trend. The influence of process parameters on enzyme production which could not be studied in shake-flask fermentations could be easily investigated in a bioreactor. Central composite rotatable design of RSM comprising of aeration 1.0 vvm, agitation 276.88 rpm and incubation period 33.68 h supported maximum keratinase production (318.38 U/ml) by Bacillus aerius NSMk2. Coefficient of determination (R2) of 0.9999 and very low probability value (p-value <0.0001) imply the significance of the model. Predicted keratinase production agreed with the observed keratinase yield, suggesting the precision and reliability of the model for keratinase optimization studies and further demonstrates the employability of the predicted optimum fermentation conditions for cost-effective keratinase production for various industrial applications.
References
Qiu J, Wilkens C, Barrett K, Meyer AS (2020) Microbial enzymes catalyzing keratin degradation: classification, structure, function. Biotechnol Adv 44:107607. https://doi.org/10.1016/j.biotechadv.2020.107607
Thyagarajan D, Barathi M, Sakthivadivu R (2014) Scope of poultry waste utilization. J Agric Veter Sci 6:29–35
Tamreihao K, Mukherjee S, Khunjamayum R et al (2018) Feather degradation by keratinolytic bacteria and biofertilizing potential for sustainable agricultural production. J Basic Microbiol 59:1–10. https://doi.org/10.1016/j.bcab.2017.04.010
Callegaro K, Brandelli A, Daroit DJ (2019) Beyond plucking: feather processing into valuable into protein hydrolysate. Waste Manag 95:399–415. https://doi.org/10.1016/j.wasman.2019.06.040
Sharma R, Devi S (2018) Versatility and commercial status of microbial keratinases: a review. Rev Environ Sci Biotechnol 17:19–45. https://doi.org/10.1007/s11157-017-9454-x
Bhari R, Kaur M, Singh RS (2021) Chicken feather waste hydrolysate as a superior biofertilizer in agroindustry. Curr Microbiol. https://doi.org/10.1007/s00284-021-02491-z
Beg QS, Sahai V, Gupta R (2003) Statistical media optimization and alkaline protease production from Bacillus mojavensis in bioreactor. Process Biochem 39:203–209. https://doi.org/10.1016/S0032-9592(03)00064-5
Zhou Y, Han LR, He HW et al (2018) Effects of agitation, aeration and temperature on production of a novel glycoprotein GP-1 by Streptomyces kanasenisi ZX01 and scale-up based on volumetric oxygen transfer coefficient. Molecules 23:125. https://doi.org/10.3390/molecules23010125
Gafar AA, Khayat EB, Ahmad SA et al (2020) Response surface methodology for the optimization of keratinase production in culture medium containing feathers by Bacillus sp. UPM-AAG1. Catalysts 10:1–18. https://doi.org/10.3390/catal10080848
Obeng DP, Morrell S, Napier-Munn TJ (2005) Application of central composite rotatable design to modeling the effect of some operating variables on the performance of three-product cyclone. Int J Miner Process 76:181–192. https://doi.org/10.1016/j.minpro.2005.01.002
Bhari R, Kaur M, Singh RS et al (2018) Bioconversion of chicken feathers by Bacillus aerius NSMk2: a potential approach in poultry waste management. Bioresour Technol Rep 3:224–230. https://doi.org/10.1016/j.biteb.2018.07.015
Bhari R, Kaur M, Singh RS (2019) Thermostable and halotolerant keratinase from Bacillus aerius NSMk2 with remarkable dehairing and laundary applications. J Basic Microbiol 59:555–568. https://doi.org/10.1002/jobm.201900001
Derringer G, Suich R (1980) Simultaneous optimization of several response variables. J Qual Technol 12:214–219. https://doi.org/10.1080/00224065.1980.11980968
Brereton RG (2018) ANOVA tables and statistical significance of models. J Chemom 33:1–5. https://doi.org/10.1002/cem.3019
Greenland S, Stephan JS, Kenneth JR et al (2016) Statistical tests, P values, confidence intervals, and power: a guide to misinterpretations. Eur J Epidemiol 31:337–350. https://doi.org/10.1007/2Fs10654-016-0149-3
Bhari R, Kaur M, Singh RS (2020) Nutritional enhancement of chicken feather waste hydrolysate by Bacillus aerius NSMk2. Indian J Microbiol 60:518–525. https://doi.org/10.1007/s12088-020-00897-0
Kuo JM, Yang JI, Chen WM et al (2012) Purification and characterization of a thermostable keratinase from Meiothermus sp. I40. Int Biodeterior Biodegrad 70:111–116. https://doi.org/10.1016/j.ibiod.2012.02.006
Barman NC, Zohora FT, Das KC et al (2017) Production, partial optimization and characterization of keratinase enzyme by Arthrobacter sp. NFH5 isolated from soil samples. AMB Express 7:1–8. https://doi.org/10.1186/s13568-017-0462-6
Abdel-Fattah AM, El-Gamal MS, Ismail SA et al (2018) Biodegradation of feather waste by keratinase produced from newly isolated Bacillus licheniformis ALW1. J Genet Eng Biotechnol 16:311–318. https://doi.org/10.1016/j.jgeb.2018.05.005
Akhter M, Marzan LW, Akter Y et al (2020) Microbial bioremediation of feather waste for keratinase production: an outstanding solution for leather dehairing in tanneries. Microbiol Insights 13:1–12. https://doi.org/10.1177/2F1178636120913280
Karimi A, Golbabaei F, Mehrnia MR et al (2013) Oxygen mass transfer in a stirred tank bioreactor using different impeller configurations for environmental purposes. Iran J Environ Health Sci Eng 10:6. https://doi.org/10.1186/1735-2746-10-6
Lakshmi PJ, Chitturi MCK, Lakshmi W (2013) Efficient degradation of feathers by keratinase producing Bacillus sp. Int J Microbiol 2013:608321. https://doi.org/10.1155/2013/608321
Macedo AJ, da Silva WOB, Gava R et al (2005) Novel keratinase from Bacillus subtilis S14 exhibiting remarkable dehairing capabilities. Appl Environ Microbiol 71:594–596. https://doi.org/10.1128/AEM.71.1.594-596.2005
Santharam L, Easwaran SN, Mohanakrishnan AS et al (2019) Effect of aeration and agitation on yeast inulinase production: a biocalorimetric investigation. Bioprocess Biosys Eng 42:1009–1021. https://doi.org/10.1007/s00449-019-02101-0
Pissuwan D, Suntornsuk W (2001) Production of keratinase by Bacillus sp. FK 28 isolated in Thailand. Nat Sci 35:171–178
Laba W, Zarowska B, Chorazyk D et al (2018) New keratinolytic bacteria in valorization of chicken waste. AMB Express 8:9–15. https://doi.org/10.1186/s13568-018-0538-y
Mamangey J, Suryanto D, Munir E et al (2020) Keratinase activity of a newly keratinolytic bacteria, Azotobacter chroococcum B4. J Pure Appl Microbiol 14:1203–1211
Habbeche A, Saoudi B, Jaouadi B et al (2014) Purification and biochemical characterization of a detergent-stable keratinase from a newly thermophilic actinomycete Actinomadura keratinilytica Cpt29 isolated from poultry compost. J Biosci Bioeng 117:413–421. https://doi.org/10.1016/j.jbiosc.2013.09.006
Hamiche S, Mechri S, Khelouia L et al (2019) Purification and biochemical characterization of two keratinases from Bacillus amyloliquefaciens S13 isolated from marine brown alga Zonaria tourneforti with potential keratin-biodegradation and hide-unhairing activities. Int J Biol Macromol 122:758–769. https://doi.org/10.1016/j.ijbiomac.2018.10.174
Kshetri P, Roy SS, Sharma SK et al (2019) Transforming chicken feather waste into feather protein hydrolysate using a newly isolated a multifaceted keratinolytic bacterium Chryseobacterium sediminis RCM-SSR-7. Waste Biomass Valorization 10:1–11. https://doi.org/10.1007/s12649-017-0037-4
Ramnani P, Singh R, Gupta R (2005) Keratinolytic potential of Bacillus licheniformis RG1: structural and biochemical mechanism of feather degradation. Can J Microbiol 51:191–196. https://doi.org/10.1139/w04-123
Gupta S, Singh R (2014) Hydrolyzing proficiency of keratinases in feather degradation. Indian J Microbiol 54:466–470. https://doi.org/10.1007/s12088-014-0477-5
Acknowledgements
The financial assistance received from Science and Engineering Research Board (SERB), Government of India, New Delhi, in the form of a major research project (ECR/2015/000156) is duly acknowledged.
Author information
Authors and Affiliations
Corresponding author
Ethics declarations
Conflict of interest
The authors declare no conflict of interest.
Additional information
Publisher's Note
Springer Nature remains neutral with regard to jurisdictional claims in published maps and institutional affiliations.
Rights and permissions
Open Access This article is licensed under a Creative Commons Attribution 4.0 International License, which permits use, sharing, adaptation, distribution and reproduction in any medium or format, as long as you give appropriate credit to the original author(s) and the source, provide a link to the Creative Commons licence, and indicate if changes were made. The images or other third party material in this article are included in the article's Creative Commons licence, unless indicated otherwise in a credit line to the material. If material is not included in the article's Creative Commons licence and your intended use is not permitted by statutory regulation or exceeds the permitted use, you will need to obtain permission directly from the copyright holder. To view a copy of this licence, visit http://creativecommons.org/licenses/by/4.0/.
About this article
Cite this article
Bhari, R., Kaur, M. & Singh, R.S. Optimization and validation of keratinase production by Bacillus aerius NSMk2 in a stirred tank reactor using response surface methodology. SN Appl. Sci. 3, 641 (2021). https://doi.org/10.1007/s42452-021-04629-x
Received:
Accepted:
Published:
DOI: https://doi.org/10.1007/s42452-021-04629-x