Abstract
Background
The aim of this study was to pool multiple data sets to build a patient-centric, data-informed, natural history model (NHM) for Duchenne muscular dystrophy (DMD) to estimate disease trajectory across patient lifetime under current standard of care in future economic evaluations. The study was conducted as part of Project HERCULES, a multi-stakeholder collaboration to develop tools to support health technology assessments of new treatments for DMD.
Methods
Health states were informed by a review of NHMs for DMD and input from clinicians, patients and caregivers, and defined using common outcomes in clinical trials and real-world practice. The primary source informing the NHM was the Critical Path Institute Duchenne Regulatory Science Consortium (D-RSC) database. This was supplemented with expert input obtained via an elicitation exercise, and a systematic literature review and meta-analysis of mortality data.
Results
The NHM includes ambulatory, transfer and non-ambulatory phases, which capture loss of ambulation, ability to weight bear and upper body and respiratory function, respectively. The NHM estimates patients spend approximately 9.5 years in ambulatory states, 1.5 years in the transfer state and the remainder of their lives in non-ambulatory states. Median predicted survival is 34.8 years (95% CI 34.1–35.8).
Conclusion
The model includes a detailed disease pathway for DMD, including the clinically and economically important transfer state. The NHM may be used to estimate the current trajectory of DMD in economic evaluations of new treatments, facilitating inclusion of a lifetime time horizon, and will help identify areas for further research.
Similar content being viewed by others
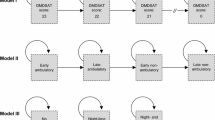
Explore related subjects
Find the latest articles, discoveries, and news in related topics.Avoid common mistakes on your manuscript.
The study identified the transfer state as being important to patients and caregivers. |
The natural history model provides data to inform disease progression under standard of care for economic evaluations of new therapies in Duchenne muscular dystrophy. |
1 Introduction
Duchenne muscular dystrophy (DMD) is a recessive, X-linked inherited neuromuscular disorder that affects 1 in 3500–5000 newborn males worldwide [1,2,3,4] and <1 in 1,000,000 newborn females [5]. It is characterised by a severe deficiency or complete absence of the protein dystrophin, leading to progressive muscle weakness and degeneration [6]. Over the course of the disease, individuals with DMD experience loss of ambulation (LoA; often accompanied by the onset of scoliosis), loss of upper limb and respiratory function and cardiomyopathy.
There are currently no curative treatment options for DMD. Standard-of-care (SoC) therapy for most patients comprises oral corticosteroids (OCS; e.g. prednisolone), which reduce inflammation, slow the progression of muscle weakness, reduce the development of scoliosis and delay respiratory and cardiovascular problems [7].
The therapeutic landscape for DMD is rapidly evolving, with a strong pipeline of emerging treatments including gene therapies, treatments that induce exon skipping and treatments that target molecular pathways [8]. In many countries (such as the UK), manufacturers must demonstrate the cost effectiveness of new treatments to gain reimbursement. Commonly, a cost-utility analysis (CUA) is required, and for a lifelong disease, this involves the estimation of lifetime quality-adjusted life-years (QALYs). To do this, the natural history (NH) of the disease must be modelled over the lifetime of patients.
However, a literature review undertaken by the authors found that there was a paucity of NH data in DMD that captures the full patient pathway (see Supplementary Materials 2 in the electronic supplementary material [ESM]). This limits the ability of cost-effectiveness models to fully capture the potential benefit of new treatments.
This study set out to build a de novo natural history model (NHM) for DMD to inform the trajectory of disease under SoC in current clinical practice across the lifetime of patients to support future economic evaluations of new therapies.
This study included the following six objectives: (1) to identify and define health states; (2) to map available data to the health states; (3) to determine the transition intensities between health states for current SoC; (4) to evaluate the impact of patient characteristics on transition intensities; (5) to predict the proportion of patients in each health state at different points in time; and (6) to quantify the mean time spent in each health state.
The study was conducted as part of Project HERCULES, a multi-stakeholder collaboration to develop tools and evidence to support health technology assessments (HTA) of new treatments for DMD.
2 Methods
Guidance has been published on NH modelling for rare diseases [9,10,11,12]. This details the requirement to construct a statistical analysis plan, pool data from multiple sources, make use of specific patient registry databases where available and have patient and clinical involvement in defining health states and mapping clinical outcomes to these states. This study followed the published guidance and any assumptions made in the construction of the NHM are explained and justified.
2.1 Health States
Health states were based on previously identified health states; health states which make sense to clinicians, patients and caregivers; and health states which can be defined using outcomes commonly collected in clinical trials and real-world practice.
2.1.1 Determining Previously Identified Health States and Outcomes Captured in Previous Clinical Trials
A targeted literature review (TLR) of previous NH studies and functional scales used in DMD was performed in MEDLINE. In the interest of expediency, and as there was no plan to undertake formal quantitative analysis from the findings, a TLR was considered a sufficient and pragmatic solution to providing an overview of published NH data.
The TLR identified existing health state definitions, key milestones in disease progression and the outcomes captured in clinical trials. The results of this review are presented in Supplementary Material 2 (see ESM). Following the review, a set of preliminary health states was determined which could be defined using outcomes commonly collected in clinical trials and real-world practice. These health states depicted the common ‘ambulatory’ model first described by Bushby et al. in the 2010 DMD Guidelines (early/late ambulatory/non-ambulatory) [13].
2.1.2 Expert Input
Health states were presented for stakeholder input to determine whether they reflected clinical experience, and step-changes in patient care and health-related quality of life. Stakeholder input included an advisory board meeting, follow-up questions with two neuromuscular specialists and validation of final health states with a group of clinical experts. The advisory board comprised the two neuromuscular specialists who provided follow-up input, one nurse, one patient advocate and three mothers of individuals with DMD, all from the UK. The advisory board also included three representatives from Duchenne UK and Project HERCULES contributors (including the University of Leicester and Source Health Economics). The final set of health states, developed with stakeholder input, were shared with 20 UK-based clinicians for validation; 14 clinicians did not provide a response, whilst six provided feedback confirming face validity of the states presented.
2.1.3 Final Health State Definitions
Figure 1 presents the final health states, which includes three phases: ambulatory, transfer and non-ambulatory; and permitted transitions.
Health states capture the progression of disease within each phase. This includes the loss of ambulatory function, the loss of the ability to stand and weight bear, and the progressive loss of upper body and respiratory function in non-ambulatory patients.
These health states reflect states that clinicians, patients and caregivers felt captured the natural history of DMD, and were defined using measures used in clinical practice.
The key addition to this model, compared with NHMs previously described in the literature, is the inclusion of the transfer state. This state was identified by patients and caregivers as a key stage in the progression of DMD due to its impact on quality of life, care support requirements and cost. Patients in the transfer state are no longer able to walk but can support their own weight to facilitate transfers, for example between wheelchair and toilet. Once this ability is lost, additional resources are required to transfer patients and the burden on caregivers increases.
Table 1 presents the health state definitions, which were informed by expert clinical input. A degree of pragmatism was required to define the identified health states using available clinical data. In particular, clinical opinion was required to estimate the forced vital capacity percent predicted (FVC%) associated with night-time and full-time ventilation. The rationale for each stage and the definitions used are presented in Supplementary Material 3 (see ESM).
The model splits after state 4, where patients start ventilation and lose hand-to-mouth function (HTMF), as there is no consistent order in which these events occur.
The inclusion of states for, or state definitions including, scoliosis and cardiomyopathy was considered. However, the reporting of data for these diagnoses in the primary data source, the Critical Path Institute (C-Path) Duchenne Regulatory Science Consortium (D-RSC), was poor. In addition, these sequelae do not represent discrete heath states, but are present in patients within several of the health states defined. The onset of scoliosis often occurs at the onset of loss of ambulation [14]. Most patients develop cardiomyopathic features between the ages of 10 and 15 years [15]. As such, future estimation of costs and utilities for economic evaluation using the health states in the NHM should capture these morbidities, for example, by assuming the proportion of patients in each state that may experience them and adjusting quality of life and resource use accordingly.
2.2 Data
2.2.1 Critical Path Institute Duchenne Regulatory Science Consortium
The primary data source informing the NHM is the C-Path D-RSC database. The D-RSC database comprises patient-level multinational clinical data for DMD shared with D-RSC by the original data custodians. The dataset used includes anonymised individual patient data (IPD) from 11 international data sources, including NH studies, placebo arms of clinical trials and registry data (further details are provided in Supplementary Material 4, see ESM). The dataset included the following variables (among others): type of functional test performed, age when the test was performed, test score, race, mutation status and steroid use. Of note, there was not a standard set of common variables collected in clinical studies and registries in DMD; as such, the variables available varied between data sources.
Table 2 presents the number (%) of transitions observed from one state to another in the D-RSC dataset; that is, how many patients were observed in state X at one point in their follow-up and then state Y at the next. Transitions from health state 1 represented the largest percentage of transitions observed (3164 [70.8%]); observations in health states 1 and 2 (early and late ambulatory patients) comprised 81.3% of observed transitions. The greatest number of ‘transitions’ observed was in patients remaining in state 1 (the early ambulatory state; 67.4% of observed transitions).
Of the 4467 observed transitions in the dataset, there were 54 (1.2%) backwards transitions, suggesting an improvement in function. Based on the small number of backwards transitions observed and clinical advice, it was deemed reasonable to assume a progressive NHM in which no backwards transitions can occur. As such, where backwards transitions were observed for an individual in the D-RSC dataset, their health state was assumed to remain unchanged.
There were very few transitions observed into or out of state 3 (the new ‘transfer’ state). There were no data pertaining to transitions into state 4 (the first non-ambulatory state) or out of state 4 into states 5 and 6, as no patients were observed in state 4 in the D-RSC dataset. This could be because in order to be assigned to states 3 or 4, a NSAA score (of 1 or 0, respectively) must be recorded, but if the patients are classed by clinicians as non-ambulatory, then the NSAA is unlikely to have been conducted.
2.2.2 Elicitation Exercise
Due to the paucity of data pertaining to health states 3, 4, 5 and 6 in the dataset, an elicitation approach was used to inform transition intensities. This included an initial pilot, in which information was elicited from four clinicians and four caregivers involved in the Project HERCULES collaboration. This was followed by an online survey of Duchenne UK stakeholders, with 20 responses from DMD parents, caregivers and practitioners (separate to the 20 UK-based clinicians who validated the final set of health states).
Using a questionnaire, respondents were asked to describe the average age at which patients enter and exit health states. From the responses received, the mean age and standard deviation (SD) for entering and exiting the states was estimated. These data were then used to simulate IPD from which transition intensities could be estimated [16]. Where some transitions were observed in the D-RSC dataset, the simulated IPD were used to augment rather than replace these data.
Using the elicitation approach, the estimated mean (SD) age at which patients transition into states 3, 4, 5 and 6 was estimated to be 11.8 (4.2), 14.3 (4.5), 19.5 (5.6) and 19.3 (6.4) years, respectively. Please see Supplementary Material 5 for more information and a copy of the survey used.
2.2.3 Mortality
No mortality data were available from the D-RSC dataset. A systematic literature review (SLR) and meta-analysis was therefore performed to identify published mortality data in DMD [17]. Kaplan Meier (KM) curves from 14 studies were digitised and IPD reconstructed via a frequently used algorithm, details of which are presented by Guyot et al. [18]. This is a commonly used technique for obtaining IPD from published studies that makes use of the fact that event times can be determined from the step-function of a KM curve. The digitised mortality data contained 2283 patients and 1050 deaths across the 14 studies. The total follow-up time was 40,274 patient years; the oldest patient in the dataset was aged 44 years. Mortality rates were determined from a parametric survival model assuming a piecewise constant hazard function. This included all 14 studies, and controlled for birth cohort (before 1970, 1970–1990 and after 1990).
Previous studies have observed improvements in survival since 1990 among individuals with DMD who are ventilated [17, 19, 20]. The authors observed this trend in the studies identified, with lower rates of mortality by age estimated in studies published since 1990. Therefore, mortality rates from the birth cohort after 1990 were used in the base-case analysis. The sub-set of patients born after 1990 included 943 patients, of whom 251 had died. Estimated median survival and reported statistics were consistent with the original studies. This validated the IPD reconstruction and estimation of mortality rates which were used in the NHM. Full methods and results of the meta-analysis are reported in Broomfield et al. [17].
2.3 Estimating Transition Intensities
Once the structure of the NHM (health states) had been agreed and data identified, transition intensities were estimated via the following six steps: (1) the mean age of patients observed in each state in the D-RSC dataset was estimated. (2) To estimate the mortality rate for each state, a piecewise constant hazard function was fitted to the mortality data, with cut points determined by the mean age in each state. (3) The initial values of a transition intensity matrix were specified using the estimated mortality rates and setting transition intensities for all transient states to 0.1 (initial transition intensities of 0.01 and 1 were also considered). (4) A multistate model was fitted in R using the msm package using the specified transition intensity matrix and fixing mortality rates at their initial values. (5) A new transition intensity matrix was then defined using the transition intensities estimated in Step 4 and mortality rates estimated in Step 2. (6) Steps 4 and 5 were then repeated using the newly defined transition intensity matrix until the model converged. Convergence was defined as transition rates being equal to 4 decimal places. An exponential distribution (i.e. constant transition intensities) was used to fit the multistate model for transitions up to health states defined by the requirement for full-time ventilation. A piecewise exponential distribution was assumed for transitions from full-time ventilation states to death. Initial consideration of the exponential distribution for all transitions led to an implausibly long length of stay in the full-time ventilation states. This was due to the long tails associated with the exponential distribution and a fixed mortality rate. Use of the piecewise exponential facilitated implementation of an increased rate of mortality after age 30 years in the full-time ventilation states. Age 30 years was selected as a mid-point in the follow up, approximately corresponding to the median survival of patients in the mortality dataset and in the published literature [17, 20].
2.4 Sensitivity Analysis
To explore areas of uncertainty, a set of scenario analyses were performed (Table 3). The NHM was generated with the transfer state (NHM A) and without (NHM B) in a scenario analysis to assess uncertainty associated with transitions into and out of the newly identified transfer state, where data were informed by the elicitation exercise.
3 Results
3.1 Base Case Results
NHM A (transfer state included) converged after two iterations. The model was insensitive to the initial transition rates estimated; using starting values of 0.01 or 1 for all transitions led to the same results in the same number of iterations. Transition intensity matrices are presented in Supplementary Material 6 (see ESM).
Figure 2 presents state membership over time, assuming patients enter the model in state 1 aged 5 years. Historically, studies had reported an average age of diagnosis of approximately 5 years [21, 22] and at the time of this study there were limited data in the D-RSC data set for children younger than 5 years of age. The NHM therefore assumes that patients younger than 5 years have yet to transition from the early ambulatory state, which clinical opinion suggested was a reasonable assumption.
Table 4 presents the predicted mean time spent in each health state and median survival. It was estimated that, on average, patients spent approximately 9.5 years in the ambulatory states, 1.5 years in the transfer state and the remainder of their lives in the non-ambulatory states. Predicted median survival was 34.8 years.
Table 5 shows the mean age of patients in each health state predicted by the NHM.
Table 6 shows the mean time in state and median survival for subpopulations including steroid users, and two subgroups of patients defined according to mutation. Patient subgroups defined according to mutation comprised patients with mutations Dup, Skip 51, Skip 53, Small mutation, Stop, any other deletion (mutation = 1) and patients with mutations Del 3-7, Skip 44, Skip 45 (mutation = 2). These populations have previously been identified and used by C-Path [23]; mutations Del 3-7, Skip 44 and Skip 45 have been reported to be associated with a milder phenotype/disease course compared with mutations skippable by exon 51 and 53 [24,25,26]. Mutations considered in the analysis were based on available data and are not exhaustive.
Confidence intervals are available in Supplementary Material 7 (see ESM).
Median survival in steroid users was very similar to median survival in the total population, and the mean time in states was similar. This was an expected result as approximately 80% of patients in the D-RSC dataset were steroid users (760/954). We attempted to fit the model to all non-steroid users, and also to fit the model to the whole dataset with steroid use (ever/never) as a covariate, but neither of these models converged.
Median survival for patients coded as Mutation = 1 was the same as the total population, and mean time in states was similar as this population comprised over 90% of patients in the D-RSC dataset (891/954).
Estimates for patients coded as Mutation = 2 were based on relatively few observations (63/954) and so should be interpreted with caution. Estimates of median survival and mean time in states differ to the total population estimates, with the higher median survival based on the long length of stay of a few patients in health state 5.
3.2 Sensitivity Analyses
Median survival and mean time in each health state for scenario analyses are shown in Table 7; confidence intervals for the mean time in state can be found in Supplementary Material 7 (see ESM).
Scenario analyses predicted a median survival of between 29.6 and 30.6 years. Predicted median survival for NHM A and B were similar (29.8 vs 29.7 years, respectively). However, it was estimated that patients spent approximately 1 year longer in the ambulatory states in NHM B than in NHM A (10.6 vs 9.5 years, respectively).
Use of all mortality rates (i.e. including mortality estimates published before 1990) decreased median survival by approximately 4 years. Directionally, this was as expected as previous studies have observed improvements in survival since 1990 (31–33).
Of the scenarios evaluating variations in the data and use of data from the elicitation exercise, changing the number of patients generated in the simulated IPD had the greatest impact on median survival. This is because the elicitation exercise predicted slightly higher mean age in states 5 and 6 than the observed data. As a result, the more patients simulated based on the results of the elicitation exercise (n = 100), the higher the median survival, and the fewer patients simulated (n = 20), the lower the median survival.
It was not possible to implement a piecewise exponential distribution for all transitions or for all mortality transitions as the models did not converge.
4 Discussion
This study provides a set of transition intensities to inform the natural history of DMD in future economic evaluations of new treatments, where previously there has been a paucity of data to inform health state transitions over the lifetime of patients. These data can be used to estimate transition probabilities for cost-effectiveness models with different cycle lengths.
The NHM has been developed with input from patients, caregivers and clinicians, which means that the health states reflect clinically important changes in patient care and health-related quality of life. Notably, it includes the transfer state, which has not been included in previous NHMs.
The definitions of included health states required an element of pragmatism; night-time ventilation was defined based on an FVC 30–50%; whilst in clinical practice not all patients with FVC 30–50% are night-time ventilated. Similarly, not all patients with FVC < 30% are full-time ventilated. Assumptions around ventilation status have also been made in previous studies in DMD. In Iff et al., the late ambulatory stage (defined using clinical tests) included the assumption of FVC ≤ 50%, with patients in this state described as losing pulmonary capabilities and requiring regular assisted ventilation [27]. The model described here makes similar assumptions around ventilation status but provides additional granularity, which will facilitate the application of utilities and costs to differing levels of ventilatory support in a cost-effectiveness analysis. In Landfeldt et al., a cost-effectiveness model based on ventilation status assumed that patients would require night-time and day- and night-time ventilation support at a mean age of 21 and 28 years, respectively [28]. The advantage of our approach in this case is that the requirement for ventilatory support is based on data for FVC rather than an assumption.
The NHM highlights current data gaps and data collection requirements for future clinical studies and disease registries. A key weakness of the NHM results from the paucity of data currently available to inform some transitions. In some cases, it was not possible to include data from registries where the data owners had yet to complete their own analyses. In other cases, there was simply a paucity of data in the D-RSC dataset.
Historically, clinical trials have not included endpoints which identify patients moving into and out of the newly identified transfer state. In order to observe patients in state 4, for example, a score of zero on the North Star Ambulatory Assessment (NSAA item 1: stand) is required. However, non-ambulatory patients are unlikely to be assessed on this scale and therefore no score is recorded.
Studies have tended to focus on younger patients in earlier health states, since this is where treatments have been targeted. A previous study suggests several possible reasons for this [29]. Firstly, younger patients have more functional abilities to preserve and therefore may be better subjects to demonstrate the success of new treatments in clinical trials. Secondly, if patients on OCS are included in clinical trials, the positive effect of OCS might mask the effect of the treatment being tested. As the studies available for this analysis focused on younger patients, this meant there were fewer observations to inform transition intensities in later health states. In addition, short study follow-up meant there were no mortality data.
Steps taken to overcome the paucity of data in this NHM have their limitations. The use of the elicitation exercise to inform transitions into and out of the transfer state is associated with uncertainty. Mortality rates, based on the current mean age of patients in each state obtained via the SLR and meta-analysis, may overestimate future health state mortality as new treatments slow progression. As such, data pertaining to transitions into and out of the transfer state, and mortality data, could be improved in future iterations of the NHM.
The recently developed Transition Assessment North Star (TANS) has been designed with a focus on transfer ability and trunk function, providing a score for the NSAA based on the easier items [24]. The John Walton Muscular Dystrophy Research Centre at Newcastle University is currently collecting data internationally using this instrument. The TANS may therefore prove useful for future data collection to inform transitions into and out of the transfer state. In terms of mortality data, a study is currently underway by the authors of this study to analyse mortality data from the Clinical Practice Research Datalink (CPRD) in the UK.
Sparse data also meant that the exponential distribution was used to fit the multistate model from states 1 to 8A/8B, as other models, for example the Weibull, were less likely to converge. Whilst the exponential distribution is easily interpreted, its use results in long tails in the extrapolations of health state occupancy, which can lead to implausibly long time periods being estimated in later health states. Initial analysis assuming an exponential distribution for all transitions led to a clinically implausible mean length of stay in state 8A/8B, with nearly 25% of patients estimated to survive beyond the age of 50. Therefore, a piecewise exponential was assumed for transitions out of state 8A/8B to death, which resulted in more clinically plausible predictions of the mean length of stay in state 8A/8B. In future iterations of the NHM, it would be valuable to also consider relaxation of the use of an exponential distribution for earlier transitions.
Given the limitations associated with data availability, it is useful to consider the validity of the NHM with reference to its congruence with the source data and previously published studies.
With the exception of states 8A and 8B, the predicted mean ages are lower in the NHM than the ages obtained when combining the D-RSC and elicitation exercise datasets. This is due to the presence of mortality in the NHM, that is, patients can leave states in the NHM in two ways, either by moving to the next state or dying, whereas D-RSC does not contain mortality data. This means patients spend less time in each state and the mean age in each state is lower. Mean ages in states 8A/8B are still slightly older in the NHM than the D-RSC dataset. This is because there is a slight pooling of patients in this state as a result of using the exponential distributions prior to state 8A/8B.
This study predicts a mean time of approximately 9.5 years in ambulatory states and 1.5 years in the transfer state; a total of approximately 11 years prior to moving into non-ambulatory health states. In 2017, McDonald et al., reported median age at loss of ambulation of 11.62 years (95% CI 11.09–12.28) in a prospective cohort study of patients with at least 1 year of OCS use at death or last known visit [30]. The 1.5 years spent in the transfer state was consistent with clinical/expert opinion derived from the elicitation exercise; however, this result would benefit from external validation.
The predicted median survival in this study (34.8 years) falls within the confidence interval for median survival estimated by Landfeldt et al. (2020), in which median survival with ventilatory support was 31.8 years (95% CI 29.3–36.2) [20] and slightly exceeds the confidence interval by Broomfield et al. (28.1 years; 95% CI 25.1–30.3) [17]. The higher estimate of survival is likely due to the fact that the C-Path patients used to populate the NHM are largely US-based and represent a more recent population than the global population in the study by Broomfield et al. [17].
A recent publication by Iff et al. [27] estimated the mean age of patients with DMD in different health states using claims and electronic medical records in the United States. Patients were stratified into the common ambulatory stages: early/late ambulatory/non-ambulatory. Health state definitions had some similarities with the definitions in the NHM presented here. Definitions for early and late ambulatory states were similar to states 1 and 2 in the NHM, respectively. The early non-ambulatory state overlaps with states 4 and 5 in the NHM, with health state definitions including FVC > 50%. Finally, the late ambulatory state overlaps with states 6, 7A/7B and 8A/8B in the NHM, with health state definitions including FVC% < 50% and ≤ 50% in the NHM and Iff et al., respectively. However, there were also some differences, with Iff et al. not including a transfer state and including (for example) age criteria for earlier states and scoliosis for later states.
Estimated mean age in state was lower in the NHM than was estimated by Iff et al. for states 1–5 and early ambulatory to early non-ambulatory, respectively. The estimated mean ages were 5.5 versus 7.4 years in state 1/the early ambulatory state, 7.8 versus 13.1 years in state 2/late ambulatory state and 11.9 to 15.2 years in states 4 and 5 versus 18.1 years in the early non-ambulatory state. There is overlap, however, in the late non-ambulatory states, with a mean age of 23.2 years in the late ambulatory state reported by Iff et al. versus 18.4–26.1 years in states 6 to 8A/8B in the NHM. The slight discrepancies may be due to the differing state definitions, with the NHM in this study offering a more granular disease progression structure.
Further validation of the NHM is certainly warranted as are further iterations as new data become available, for example, as diagnosis of DMD is made earlier than the starting age of 5 years assumed in this model.
Another key next step is to describe the methods for implementing the NHM within a future cost-effectiveness model, including the estimation of transition probabilities for different model cycle lengths, implementing a treatment effect and methods for explicitly including the sequelae not included in the NHM (i.e. scoliosis and cardiomyopathy) if required. The implementation of the NHM within a cost-effectiveness model will be the subject of a future publication.
Ultimately, if future clinical trials capture endpoints based on the definitions of each health state in the NHM, treatment effects for all observed transitions can be applied to the NHM within a cost-effectiveness model. In the meantime, an intervention might be assumed to impact only transitions for which trial data are available, and in the absence of data to inform other transitions, the value of collecting information might be estimated.
5 Conclusion
The NHM developed includes a detailed disease pathway for DMD, which has only been possible due to the integration of multiple data sources augmented by an unprecedented level of stakeholder input. The model provides quantitative insights into the lifetime trajectory of DMD under SoC and will support the development of future economic evaluations, with the potential to capture broader disease effects and adopting a lifetime time horizon. The identification of the transfer state also highlights a priority area for further data collection.
Change history
03 July 2024
A Correction to this paper has been published: https://doi.org/10.1007/s41669-024-00506-6
References
Crisafulli S, Sultana J, Fontana A, Salvo F, Messina S, Trifiro G. Global epidemiology of Duchenne muscular dystrophy: an updated systematic review and meta-analysis. Orphanet J Rare Dis. 2020;15(1):141.
San Martín PP, Solis F, Cavada CG. Survival of patients with Duchenne muscular dystrophy. Rev Chil Pediatr. 2018;89(4):477.
van den Bergen JC, Ginjaar HB, van Essen AJ, Pangalila R, de Groot IJ, Wijkstra PJ, et al. Forty-five years of duchenne muscular dystrophy in The Netherlands. J Neuromuscul Dis. 2014;1(1):99–109.
Wang M, Birnkrant DJ, Super DM, Jacobs IB, Bahler RC. Progressive left ventricular dysfunction and long-term outcomes in patients with Duchenne muscular dystrophy receiving cardiopulmonary therapies. Open Heart. 2018;5(1): e000783.
Duan D, Goemans N, Takeda S, Mercuri E, Aartsma-Rus A. Duchenne muscular dystrophy. Nat Rev Dis Primers. 2021;7(1):13.
Falzarano MS, Scotton C, Passarelli C, Ferlini A. Duchenne muscular dystrophy: from diagnosis to therapy. Molecules. 2015;20(10):18168–84.
Matthews E, Brassington R, Kuntzer T, Jichi F, Manzur AY. Corticosteroids for the treatment of Duchenne muscular dystrophy. Cochrane Database Syst Rev. 2016;2016(5):CD003725.
Markati T, Oskoui M, Farrar MA, Duong T, Goemans N, Servais L. Emerging therapies for Duchenne muscular dystrophy. Lancet Neurol. 2022;21(9):814–29.
Rare Diseases: Natural History Studies for Drug Development—FDA Draft Guidance for Industry (2019). Available at: https://www.fda.gov/regulatory-information/search-fda-guidance-documents/rare-diseases-natural-history-studies-drug-development. Accessed 11 May 2023
Best Practices for Development and Application of Disease Progression Models—FDA Workshop (2021). Available at: https://www.fda.gov/drugs/news-events-human-drugs/best-practices-development-and-application-disease-progression-models-11192021. Accessed 11 May 2023.
Jewell NP. Natural history of diseases: statistical designs and issues. Clin Pharmacol Ther. 2016;100(4):353–61.
Liu J, Barrett JS, Leonardi ET, Lee L, Roychoudhury S, Chen Y, et al. Natural history and real-world data in rare diseases: applications, limitations, and future perspectives. J Clin Pharmacol. 2022;62(Suppl 2):S38–55.
Bushby K, Finkel R, Birnkrant DJ, Case LE, Clemens PR, Cripe L, et al. Diagnosis and management of Duchenne muscular dystrophy, part 1: diagnosis, and pharmacological and psychosocial management. Lancet Neurol. 2010;9(1):77–93.
Choi YA, Shin HI, Shin HI. Scoliosis in Duchenne muscular dystrophy children is fully reducible in the initial stage, and becomes structural over time. BMC Musculoskelet Disord. 2019;20(1):277.
McNally EM. Cardiomyopathy in muscular dystrophy: when to treat? JAMA Cardiol. 2017;2(2):199.
Papadimitropoulou K, Stijnen T, Riley RD, Dekkers OM, le Cessie S. Meta-analysis of continuous outcomes: using pseudo IPD created from aggregate data to adjust for baseline imbalance and assess treatment-by-baseline modification. Res Synth Methods. 2020;11(6):780–94.
Broomfield J, Hill M, Guglieri M, Crowther M, Abrams K. Life expectancy in duchenne muscular dystrophy: reproduced individual patient data meta-analysis. Neurology. 2021;97(23):e2304–14.
Guyot P, Ades AE, Ouwens MJ, Welton NJ. Enhanced secondary analysis of survival data: reconstructing the data from published Kaplan-Meier survival curves. BMC Med Res Methodol. 2012;1(12):9.
Eagle M, Baudouin SV, Chandler C, Giddings DR, Bullock R, Bushby K. Survival in Duchenne muscular dystrophy: improvements in life expectancy since 1967 and the impact of home nocturnal ventilation. Neuromuscul Disord. 2002;12(10):926–9.
Landfeldt E, Thompson R, Sejersen T, McMillan HJ, Kirschner J, Lochmüller H. Life expectancy at birth in Duchenne muscular dystrophy: a systematic review and meta-analysis. Eur J Epidemiol. 2020;35(7):643–53.
Mohamed K, Appleton R, Nicolaides P. Delayed diagnosis of Duchenne muscular dystrophy. Eur J Paediatr Neurol. 2000;4(5):219–23.
Ciafaloni E, Fox DJ, Pandya S, Westfield CP, Puzhankara S, Romitti PA, et al. Delayed diagnosis in duchenne muscular dystrophy: data from the Muscular Dystrophy Surveillance, Tracking, and Research Network (MD STARnet). J Pediatr. 2009;155(3):380–5.
Conrado DJ, Larkindale J, Berg A, Hill M, Burton J, Abrams KR, et al. Towards regulatory endorsement of drug development tools to promote the application of model-informed drug development in Duchenne muscular dystrophy. J Pharmacokinet Pharmacodyn. 2019;46(5):441–55.
Ricotti V, Ridout DA, Pane M, Main M, Mayhew A, Mercuri E, et al. The NorthStar Ambulatory Assessment in Duchenne muscular dystrophy: considerations for the design of clinical trials. J Neurol Neurosurg Psychiatry. 2016;87(2):149–55.
Wang RT, Barthelemy F, Martin AS, Douine ED, Eskin A, Lucas A, et al. DMD genotype correlations from the Duchenne Registry: endogenous exon skipping is a factor in prolonged ambulation for individuals with a defined mutation subtype. Hum Mutat. 2018;39(9):1193–202.
Bello L, Morgenroth LP, Gordish-Dressman H, Hoffman EP, McDonald CM, Cirak S, et al. DMD genotypes and loss of ambulation in the CINRG Duchenne Natural History Study. Neurology. 2016;87(4):401–9.
Iff J, Zhong Y, Gupta D, Paul X, Tuttle E, Henricson E, et al. Disease progression stages and burden in patients with Duchenne muscular dystrophy using administrative claims supplemented by electronic medical records. Adv Ther. 2022;39:2906–19.
Landfeldt E, Alfredsson L, Straub V, Lochmuller H, Bushby K, Lindgren P. Economic evaluation in Duchenne muscular dystrophy: model frameworks for cost-effectiveness analysis. Pharmacoeconomics. 2017;35(2):249–58.
Merlini L, Sabatelli P. Improving clinical trial design for Duchenne muscular dystrophy. BMC Neurol. 2015;26(15):153.
McDonald CM, Henricson EK, Abresch RT, Duong T, Joyce NC, Hu F, et al. Long-term effects of glucocorticoids on function, quality of life, and survival in patients with Duchenne muscular dystrophy: a prospective cohort study. Lancet. 2018;391(10119):451–61.
Acknowledgements
The authors would like to acknowledge the clinicians, caregivers, patients and industry stakeholders of the Project HERCULES steering committee for their invaluable input into this study, Anna Mayhew from The John Walton Muscular Dystrophy Research Centre at Newcastle for information on the Transition Assessment North Star (TANS) instrument, Chris Jackson at the MRC Biostatistics Unit of the University of Cambridge for his support on the use of the msm R package and Prof. Laura Bojke at the Centre for Health Economics at the University of York for advice regarding the pilot elicitation exercise. The authors would also like to thank study team members from our participating CINRG sites, details of whom can be found in Supplementary Material 1. Finally, the authors would like to thank Dr Jennifer Ferris and Dr Dominic Partridge of Source Health Economics for medical writing and editorial support.
Author information
Authors and Affiliations
Consortia
Corresponding author
Ethics declarations
Funding
This study was funded by Duchenne UK.
Conflict of interest
Micki Hill has worked for GSK since April 2022. Keith Abrams is a partner and director of Visible Analytics Limited, a health technology assessment consultancy company; a National Institute for Health and Care Research (NIHR) Senior Investigator Emeritus (NF-SI-0512-10159); and a member of the National Institute for Health and Care Excellence (NICE) Diagnostics Advisory Committee. Jane Larkindale has worked for PepGen Inc. since April 2022. There are no other conflicts of interest for authors contributing to this manuscript.
Availability of data and materials
D-RSC data access applications can be made via https://c-path.org/programs/d-rsc/overview/database-access/. Access to the mortality data set and the simulated individual patient data obtained from the elicitation exercise in this study are available on request from jb781@leicester.ac.uk.
Ethics approval
All studies within the D-RSC dataset are fully de-identified and compliant to the terms and conditions specified by the data owners, with more information at https://c-path.org/programs/d-rsc/overview/. Further analysis of the D-RSC dataset and methods of the elicitation exercise were reviewed and approved by the University of Leicester ethics committee (ref number: 32556-jb781-Ls:healthsciences).
Consent to participate
All participants were provided with an information sheet and required to provide informed consent before completing the elicitation exercise.
Consent for publication
Participants in the online elicitation exercise were presented with a comprehensive information sheet, and by taking part, gave consent for the results of the exercise to be published anonymously.
Code availability
A multi-state model was fitted in R using the msm package, allowing for piecewise-constant transition intensities, with details of the analysis described in the main body of text.
Author contributions
EC and FC conceived the study; EC acquired the funding; KRA and MH designed the study; JG provided project administration; JL supervised the conduct of the study and data collection; JL and MH managed the data, including quality control; KRA, MH, MG, JB and MJC provided statistical advice on study design and analysed the data; KRA, JB, JM-C and FW drafted the manuscript; FC, JH and MG conducted the writing review; and JB is responsible for the whole paper.
Additional information
The original online version of this article was revised: In the orginally published article, many of the supplementary materials were missing from the Supplementary Information file. A new file has now been uploaded containing all supplementary materials.
Supplementary Information
Below is the link to the electronic supplementary material.
Rights and permissions
Open Access This article is licensed under a Creative Commons Attribution-NonCommercial 4.0 International License, which permits any non-commercial use, sharing, adaptation, distribution and reproduction in any medium or format, as long as you give appropriate credit to the original author(s) and the source, provide a link to the Creative Commons licence, and indicate if changes were made. The images or other third party material in this article are included in the article's Creative Commons licence, unless indicated otherwise in a credit line to the material. If material is not included in the article's Creative Commons licence and your intended use is not permitted by statutory regulation or exceeds the permitted use, you will need to obtain permission directly from the copyright holder. To view a copy of this licence, visit http://creativecommons.org/licenses/by-nc/4.0/.
About this article
Cite this article
Broomfield, J., Hill, M., Chandler, F. et al. Developing a Natural History Model for Duchenne Muscular Dystrophy. PharmacoEconomics Open 8, 79–89 (2024). https://doi.org/10.1007/s41669-023-00450-x
Accepted:
Published:
Issue Date:
DOI: https://doi.org/10.1007/s41669-023-00450-x