Abstract
Introduction
The type of methods used in economic evaluations of health technology can lead to results that may influence decisions. Despite the potential impact on decision making, there is very little documentation of methods used in economic evaluation in oncology pertaining to key assumptions and extrapolation methods of survival benefits, especially in terms of survival analysis techniques and methods for extrapolation.
Objectives
The primary objectives of this study were to identify, examine, and describe the methods used in economic evaluations in oncology over a 10-year period, while secondary objectives included examining the use of identified methods across different geographic regions.
Methods
A systematic search of the published oncology literature was conducted to identify economic evaluations of advanced or metastatic cancers published between 2010 and 2019 using the PUBMED, Ovid MEDLINE, and EMBASE databases. A random sample was taken, and information on type of study, data source, modeling techniques, and survival analysis methods were abstracted and descriptively summarized.
Results
A total of 8481 abstracts were identified and 76 economic evaluations were abstracted and assessed. Most identified studies were from North America (38%), East Asia (21%), continental Europe (18%), or the UK (16%), and most commonly focused on lung cancer (18%), colorectal cancer (16%), or breast cancer (13%). A large majority of studies were based on data from randomized controlled trials (82%), utilized a cost-utility approach (82%), and took a public healthcare system perspective (83%). Common model structures included Markov (49%) and partitioned survival (17%). Fitted parametric curves were the most commonly used extrapolation method (89%) for overall survival and most often utilized the Weibull distribution (64%). Secondary assessments showed modest regional variation in the use of identified methods, including the use of fitted parametric curves, testing of the proportional hazards assumption, and validation of results.
Conclusion
A majority of papers in the study sample reported basic characteristics of study type, data source used, modeling techniques, and utilization of survival analysis methods. However, greater detail in reporting extrapolation methods, statistical analyses, and validation of results could be potential improvements, especially across regions, in order to support greater consistency in decision making. Future research could document the diffusion of novel modeling techniques into economic evaluation.
Similar content being viewed by others
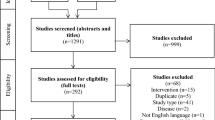
Avoid common mistakes on your manuscript.
Results suggest most published economic evaluations in oncology use a partitioned survival or Markov structure informed by phase III randomized controlled trials. |
The Weibull parametric distribution was most commonly used for both progression-free survival (PFS) and overall survival (OS) extrapolations, for an average of 90 months beyond duration of clinical study. Justification of distribution choice was rare and few studies reported testing the proportional hazards assumption. |
Most identified economic evaluations did not validate the results, suggesting better reporting of survival analysis methods is needed. |
1 Introduction
Economic evaluation in healthcare estimates the value for money of health technologies through assessment of the comparative costs and clinical impacts, the results of which can inform the value of a specified allocation of healthcare resources [1]. To increase transparency and reporting of economic methods of health technologies, several guidelines have been published. For example, the National Institute for Clinical and Care Excellence (NICE) in the UK publishes a series of practice guidelines to aid submissions from manufacturers seeking reimbursement of their health technologies. Similar guidelines have been published by the Canadian Association for Drugs and Technologies in Health (CADTH) in Canada, the 2nd Panel on Cost-Effectiveness in Health and Medicine in the US, the Research Group on Economic Evaluation for Japanese Public Medical Benefits in Japan, and the Health Insurance Review and Assessment Service (HIRA) in South Korea, among many others [2,3,4,5,6,7]. Despite widespread publication and availability of guidelines, documentation of the utilization of specific methods in economic evaluation in oncology remains limited. This is important as the last decade has seen many important methodological advances when conducting economic evaluations of oncologic treatments.
In addition to extrapolation and other survival analysis techniques, other quantitative methods (e.g. statistical testing, crossover adjustment techniques, alternative model structures) have been developed to overcome the limitations of previous methods. For example, clinical studies are finite in length, and in order for the results of clinical studies to be amenable to economic evaluation, it is often necessary to extrapolate clinical outcomes beyond the study duration through survival analysis techniques [2, 5, 6, 8, 9]. In addition, the analytical methods selected for economic evaluation in oncology have been shown to influence survival results [10], and therefore it is important for appropriate methods to be used when evaluating oncology products.
However, only two previous studies have detailed the use of survival analysis in economic evaluations in oncology [11, 12]. The first of these studies examined survival modeling and extrapolation techniques used in oncology submissions to the NICE in the UK before and after publication of the NICE Decision Support Unit’s Technical Support Document (TSD) on survival analysis [13]. The authors extracted data from 20 technology appraisals and reviewed information on model structure, data sources, extrapolation methods, and validation. The authors found that extrapolation techniques in practice have improved since the publication of the guidelines; some form of parametric extrapolation was used in almost all of the NICE submissions except one. However, nearly 30% of the submissions did not identify the source of overall survival (OS) data, and although the authors reported which parametric distributions were tested in the submissions, the specific distribution(s) chosen for extrapolation were not identified. Statistical testing methods used in the submissions were also documented but not disaggregated by type.
A second study from 2019 reviewed 58 NICE technology appraisals and examined the extent to which recommendations made in the NICE Decision Support Unit’s TSD on survival analysis have been followed since its publication [11]. The authors found that while there were increases in validation of the results using data and/or clinical opinion following publication of the TSD, the proportion of submissions that adhered to the TSD recommendations did not change substantively over time. The authors concluded that despite publication of the guidelines, survival analysis conducted as part of NICE technology appraisals remains suboptimal [11]. The study was limited to assessment of survival analysis and did not examine other characteristics of the NICE submissions. While these two UK studies are informative, their generalizability may be limited outside of the UK, and the results are not necessarily reflective of economic models found in the published oncology literature.
2 Objectives
To fill a gap in the literature, the present study aims to identify, examine, and describe the analytical methods used in economic evaluations, including study characteristics and model structure, although a systematic survey of the published oncology cost-effectiveness literature over a 10-year period between 2010 and 2019 using commonly cited English language databases for economic evaluations in oncology. This approach allows a wider range of analytical techniques to be catalogued over a longer period of time than has been presented in previous studies. Secondary objectives of the study include examining the use of identified methods across different geographic regions.
3 Methods
A systematic search of the published oncology literature was conducted to identify economic evaluations of advanced or metastatic cancers published between 2010 and 2019 using the PUBMED, Ovid MEDLINE, and EMBASE databases. The Population, Intervention, Comparison and Outcomes (PICOS) method was followed for determining literature search criteria; identified studies were limited to English-language economic evaluations of advanced or metastatic cancer among adult populations, both treatment(s) and comparator(s) had to be explicitly reported, and outcomes of interest included incremental cost-effectiveness ratios (ICERs) and/or cost-utility effectiveness ratios (ICURs). The literature search was limited to economic evaluations, and duplicates and published abstracts were excluded. All eligibility criteria were defined a priori.
The following keywords were used in the database search queries: ‘cost-effectiveness analysis’, ‘cost-benefit analysis’, ‘cost-utility analysis’, ‘quality-adjusted life-years’, ‘metastasis’, ‘advanced cancer’, ‘advanced neoplasm’, ‘metastatic neoplasm’, ‘economics’, ‘cost’, ‘health economics’, ‘budget’, ‘costing’, ‘price’, ‘pharmacoeconomic’, ‘expenditure’, ‘expenses’, ‘statistical model’, ‘economic model’, ‘probability’, ‘Markov’, ‘Monte Carlo method’, ‘decision theory’, and ‘decision tree’. Refer to the Appendix for specific search strategies for each database.
Initial screening of titles and abstracts for relevance to the study objectives was conducted according to the stated eligibility criteria by the primary author. Full-text articles meeting all of the inclusion criteria and none of the exclusion criteria were reviewed by the primary author, and for each included study, data were extracted (see the electronic supplementary material [ESM] for a complete list of extracted studies) to describe study characteristics (i.e. disease area, patient population, type of cancer, source of clinical data, type of economic evaluation, study perspective, overall conclusions, funding source, validation methods, software used), key assumptions and modeling techniques (model structure, number of modeled health states, time horizon, intervention, comparator, treatment line, discount rates, outcomes of interest, types of analyses, key study results, total costs, base-case ICER, sensitivity analyses, willingness to pay [WTP] threshold, data sources), and extrapolation methods (i.e. statistical techniques used for fitting curves, type of distribution, crossover adjustment, digitization method use).
Analytical methods identified in each included study were extracted and documented in the extraction sheet, and similarities and differences were descriptively assessed. Economic evaluations sponsored by industry have been observed to utilize longer time horizons than those conducted by health technology assessment (HTA) agencies [14]. Previous observations have also suggested that industry-sponsored studies are more likely than academic-sponsored studies to report favorable conclusions of cost effectiveness [15]. In addition, since novel approaches have been suggested to model immunotherapy (IO) [16, 17], a comparison of the model structure between IO and non-IO drugs was conducted. Chi-square tests were used to probe relationships between categorical variables in order to substantiate these previous observations. Identified studies were also grouped according to geography in order to capture potential variation across regions. Statistical testing was performed in Microsoft Excel 2019 (Microsoft Corporation, Redmond, WA, USA).
A large number of studies was anticipated to be identified through the search strategies. Based on the number of studies reported in previous publications, it was determined that a sample size approximately equal to that reported by Benedict and Muszbek [12] (n = 58), and three times larger than the sample size of n = 20 studies reported by Bell Gorrod et al. [11], would be an appropriate and representative snapshot of the large number of studies captured in the literature search. It was initially assumed that a 20% random sample of all eligible studies over the 2010–2019 timeframe, conducted in Microsoft Excel using a combination of RAND, INDEX, and MATCH functions, would yield at least 60 studies. Prior to knowing the exact number of studies that would meet the inclusion criteria, the random sample size of 20% was to be increased to reach a total of 60 studies if necessary. This desired sample of 60 studies was based on previous studies on methods (Benedict and Muszbek [N = 58] and Bell Gorrod et al. [N = 20] [11, 12]). To validate the representativeness of our sample, we took another random sample of 20% of the studies and compared the two samples in terms of type of economic evaluation and model structure.
4 Results
4.1 Results of a Systematic Survey
A total of 8481 abstracts were identified through the literature search and 1671 duplicates were removed (Fig. 1); 5907 studies were excluded in level 1 screening (according to the PICOS criteria), and the remaining 903 full-text studies were assessed using a predefined eligibility form. Of these studies, 538 were removed through level 2 screening (according to the PICOS criteria) and a total of 378 met the eligibility criteria (Fig. 1). The 20% random sampling of the 378 studies meeting the inclusion criteria resulted in 76 studies being included in the abstraction set (see the ESM for a complete list of the included studies).
4.2 Study Characteristics
A detailed description of the study characteristics is presented in Table 1. Briefly, close to half of the included studies originated from North America (38%), and the most commonly assessed cancer types were lung (18%), colorectal (16%), and breast cancers (15%). Approximately half of the studies were published within the last 4 years. A majority (82%) of identified papers were based on clinical data from phase III randomized controlled trials, while another 16% utilized observational data from real-world evidence studies. The remaining 3% of studies were based on data from random-effects network meta-analyses, including one study employing a network of five trials [18] and the other comprising a network of 16 studies [19]. The real-world studies were overwhelmingly retrospective in nature (92%), comprised of predominantly database analyses (67%) or based on registry data (25%). Several of the real-world studies (17%) conducted propensity score matching to balance prognostic factors between treatment arms, and 33% utilized Cox models.
Funding sources of the identified studies were relatively well-balanced between industry sponsorship (33%), public grants (32%), and no declaration of funding (28%). Cross-referencing the time horizon selected by study authors with the source of funding revealed that industry-sponsored studies were more likely to use longer time horizons. Of the 32 included studies that used a time horizon of 10 + years (including lifetime), 47% were industry-sponsored, whereas 16% were funded through public grants. Conversely, for the studies using shorter time horizons of ≤ 5 years, funding sources were more evenly distributed, with 39% sponsored by industry and 39% funded through public grants; however, no statistically significant relationship was identified between funding sources and time horizon (Chi-square test: p = 0.2939).
4.3 Key Assumptions and Modeling Techniques from Identified Studies
Over three-quarters of all included studies (82%) were cost-utility analyses and 83% were conducted from a public healthcare system perspective. The most common model structure was the Markov model (47%) followed by the partitioned survival model (17%). More than half (57%) of all included studies concluded that the intervention under investigation was cost effective and this proportion was higher for industry-sponsored studies (76%; Chi-square test: p = 0.0054). Details are presented in Table 2.
In approximately 9% of studies, methods for crossover adjustments were reported to have been used. Of these seven studies, some of which reported more than one crossover adjustment method, the most commonly cited methods were Cox regression with crossover as a time-dependent covariate (29%), rank-preserving structural failure time (29%), and inverse probability of censoring weights (29%); however, three of the seven studies (43%) did not report the specific crossover adjustment method used.
4.4 Extrapolation Methods
Forty-nine percent of studies reported extrapolation of survival endpoints and 19% of these studies reconstructed Kaplan–Meier curves using digitization techniques (Table 3). When reported, the average number of months of extrapolation beyond the clinical study duration was approximately 90 months. Among the 49% of studies that extrapolated results, 89% reported extrapolation using fitted parametric curves. Hybrid models combining both Kaplan–Meier trial data and extrapolated data were relatively rare (5%), and only two studies (5%) used solely the hazard ratio method to extrapolate over time (both based on patient-level observational data). Other non-common methods used for extrapolation included use of a simple average monthly transition probability applied across years (3%), and transition probabilities calibrated to minimize mean squared differences between trial survival endpoints and model-generated curves (3%). Less than 20% of the extrapolated studies reported testing the proportional hazards assumption to justify their extrapolation. Half of these used the log-cumulative hazards plot to assess proportional hazards, while the other half did not report the method of assessment used.
Overall, 28 studies (37%) reported which distributions were used for extrapolation, and among these 28 studies, the Weibull distribution was the most commonly used parametric distribution for both treatment and comparator model arms in modeling progression-free survival (PFS; 39%, 36%) and OS (64%, 61%), followed by the log normal distribution for PFS (18%, 21%) and the log-logistic for OS (14%, 14%). Distribution selection for PFS was reported in 21 studies, 27 studies reported distribution selection for OS, and the Akaike Information Criterion (AIC) was the most commonly reported method for identifying best statistical fit (32%). The majority of identified studies did not validate the results of their analyses and extrapolations; only 21% of the identified studies performed a validation procedure, and validation was more commonly performed in studies published in later years compared with earlier years. The most common validation techniques reported were clinical experts (44%), comparison with previous studies (31%), comparison with real-world evidence (13%), and creation of a separate validation model (13%).
4.5 Additional Analyses
The greatest number of identified studies were from North American countries (38%), followed by countries in East Asia (21%), continental Europe (18%), and the UK (16%). A majority of the North American studies (59%), European studies (64%), and UK studies (58%) found the treatment under investigation to be cost effective at the WTP threshold cited by the authors, while this proportion was 50% for studies from East Asia. Among studies that reported conducting validation exercises, some small geographic variation was noted: 11% of studies from North America reported validation, compared with 6% of studies from East Asia, 5% of studies from the UK, and 4% of studies from continental Europe. In addition, a much higher proportion of studies from the UK (100%) and North America (93%) reported use of probabilistic sensitivity analysis than studies from other geographic regions (continental Europe: 71%; East Asia: 69%). Comparable trends were observed for utilization of deterministic sensitivity analysis, scenario analyses, and use of cost-effectiveness acceptability curves. Sensitivity analyses were also reported in some studies from South America, Australia, and Africa, but the number of studies included for each of these regions was too low to draw clear inferences or conclusions.
Some regional variation was also observed when analyzing survival extrapolation methods across jurisdictions. The use of fitted parametric curves for extrapolation was frequently reported in studies from the UK (83%), about twice as often as reported in studies from other geographic regions (East Asia: 44%; North America: 38%; continental Europe: 29%). Additional variation between regions included the number of studies reporting adjustment for crossover, which was higher in the UK (25%) than in other regions (0–7%), and testing of the proportional hazards assumption, which was consistently rare across most regions (between 7% and 17%) but was not reported at all in studies from East Asia. Other methods were broadly similar across regions. Results of the analysis comparing IO (n = 19) and non-IO (n = 57) economic evaluations indicated that while approximately half of both IO (58%) and non-IO studies (46%) used Markov models, a greater proportion of IO-focused studies used partitioned survival models (32%) compared with non-IO studies (12%).
Finally, to investigate the representativeness of the 20% random sample, a second 20% random sample was taken. Comparing the types of models in the second random sample with the original random sample, the proportion of studies reporting the primary analysis as cost utility, cost effectiveness, or ‘other’ were 84%, 13%, and 2% in the second random sample, and 82%, 17%, and 1% in the original random sample, respectively. A high degree of concordance between the two random samples was also observed for the proportions of studies reporting common model structures: Markov models (58% vs. 49%), partitioned survival (17% vs. 17%), decision tree (8% vs. 7%), ‘other’ (7% vs. 5%), and not reported (9% vs. 9%). A small degree of variation was seen for less commonly used model structures: combination (0% vs. 7%), discrete event simulation (0% vs. 3%), and microsimulation (1% vs. 4%).
5 Discussion
5.1 Summary of Main Results
This 20% random sample of published economic evaluations over the past decade has shown that many advances in economic evaluation methods have diffused into common usage. These methods included deterministic and probabilistic sensitivity analysis, extrapolation of outcomes beyond the duration of clinical trials, utilization of cost-effectiveness acceptability curves, both Markov and partitioned survival model structures, and the cost-utility analytical framework. Less frequently or inconsistently utilized methods included testing of the proportional hazards assumption (for those studies in which it would have been appropriate to do so), assessing statistical fit of survival extrapolations, and validating study results. Looking at the study sample across geographic regions, heterogeneity was observed in the use and reporting of procedures for validating results, statistical curve fitting techniques, testing of proportional hazards assumption, and adjustment for crossover. While new methods may be developed over time, uniform uptake across regions is not guaranteed, even when supported by the publication of economic evaluation guidelines.
5.2 Explanation of Findings and Comparison with Other Studies
Previously cited reviews of economic evaluations in oncology have examined data over a very limited period or have been focused on a specific jurisdiction [11, 12]. An additional study from late 2019 examined modeling approaches in 100 NICE technology appraisals and 124 published studies, finding that the state transition model (41.0%, 82.3%) and partitioned survival model (54.0%, 12.1%) were the most commonly utilized model structures in NICE submissions and published oncology literature, respectively [20]. However, this study was limited to a 5-year period and investigated model structure exclusively and did not review, for example, the model assumptions regarding PFS or survival extrapolations. To the authors’ current knowledge, the present analysis provides the first examination of published English-language economic evaluations in oncology across a 10-year period, focused on multiple modeling methods across multiple jurisdictions and cataloguing trends in methods uptake across geographies.
Comparing the study characteristics observed in the present study based on published economic evaluations in oncology between 2010 and 2019 with the results of previously conducted studies reveals a number of similarities. First, Markov models and partitioned survival models appear to be the most commonly utilized model structures across most geographic regions, which suggests that these methods have been broadly accepted and integrated into economic evaluation processes. Comparisons between UK studies included in the present analysis and previous studies (also UK studies) demonstrates further similarities in terms of average model duration (time horizon), use of fitted parametric curves and extrapolation techniques, the use of procedures to validate results, and testing of the proportional hazards assumption. For example, the use of fitted parametric survival curves was found to be similar between previous publications and the UK subset from the present study (76% [12], 91% [11], and 83%, respectively).
Insights beyond those presented in previous studies include results presented across more than a single geography. For example, while the use of partitioned survival models has been extensively observed by Benedict and Muszbek (61%) [12], Bullement and colleagues (54%) [20], and UK studies included in the present analysis (42%), this model structure was seldomly observed in studies from North America (17%), continental Europe (7%), and East Asia (6%). Studies from jurisdictions outside the UK tended to favor the use of Markov models (24%, 43%, and 56%, respectively). In contrast to the results from previous UK studies, fitted parametric survival curves were also much less frequently reported in studies from East Asian countries (44%), North America (38%), and continental Europe (29%). These observations suggest that there may be important differences in uptake of economic evaluation methods across geographic regions, and these could potentially lead to differences in decision making.
5.3 Limitations
The present study is associated with a number of limitations. Search parameters were limited to articles published in English, exhibiting a bias towards studies from countries that have English as a first language. Second, not all economic evaluations in oncology require or report extrapolation of survival endpoints, and thus the total number of studies from which inferences may be drawn around survival outcomes may be limited. In addition, while the 20% random sample taken was assumed to be representative of the entire 378 identified studies that met the inclusion criteria, there is no guarantee that the studies not included in the random sample would provide similar or corroborative results, although this may be an intuitively plausible conjecture. To address this limitation, a second 20% random sample was taken in which the analysis type and model structure characteristics were found to be similar to the proportions observed in the original 20% random sample, providing some reassurance regarding its representativeness. It was also assumed that if study authors did not mention extrapolation, it was assumed that study results were not extrapolated. In some cases, absence of this evidence may not be evidence of its absence, although the number of studies to which this applies might be expected to be small. Comparisons between regions were also limited by the relatively small number of studies per group.
This study was focused on published literature exclusively, and thus there is a potential for publication bias since oncology models built for reimbursement submissions were not included in the study. The study results also do not directly capture the impact of the evolution of methods on oncology models submitted to HTA agencies or the subsequent reimbursement recommendations made based on those models. Conference abstracts were also excluded, implying the potential for not having captured some of the most up-to-date economic evaluation methods in use, and this may in turn affect the external validity of this study. However, since the content of conference abstracts is necessarily limited and may differ from the content included in full publications, excluding them in the present study may be justifiable for the purposes of comparison with published economic evaluations.
5.4 Future Directions
As economic evaluation becomes increasingly embedded in decision making, a subsequent increase in the aggregate number of published studies can be expected. This increase will provide opportunities to re-evaluate the uptake of methods in light of the development of new guidelines, survival analysis techniques, and other methods. While our study found that Markov and partitioned survival models were the most common structures used among our sample of studies published between 2010 and 2019, future research could focus on the use of novel modeling techniques such as discrete event simulation [20,21,22,23,24], multistate modeling [20, 25, 26], and mixture cure models [27, 28], which are more frequently used to overcome specific limitations inherent in more rudimentary analytical approaches. Discrete event simulation, for example, is typically used when the implementation of a defined model structure is not manageable as a cohort-based state transition model, or when baseline heterogeneity, continuous disease markers, time-varying event rates, and the influence of prior events on subsequent event rates are of relevance to decision making [22]. Recent advances in the development of anticancer therapies have led to the advent of therapeutics that may be curative for certain patients, leading to recommendations of using mixture cure modeling [29]. Since we might expect to see more frequent use of newer modeling methods [20], and given that these novel methods have not (yet) been incorporated into current guidelines, it could be helpful and informative for future research efforts to track and document the diffusion of these newer methods into use over time, both in the published oncology literature and in technology appraisals from health technology assessment bodies.
6 Conclusion
This review of published economic evaluations in oncology has shown that over the past decade a majority of the identified papers reported basic characteristics of study type, data source used, modeling techniques, and utilization of survival analysis methods. However, greater detail in reporting extrapolation methods, statistical analyses, and validation of results could be potential improvements. Regional variation observed in the use of these methods warrants further examination in order to support greater consistency in decision making. Future research efforts could be dedicated towards documenting the diffusion of novel modeling techniques into economic evaluation.
References
Richardson J, Schlander M. Health technology assessment (HTA) and economic evaluation: efficiency or fairness first. J Market Access Health Policy. 2019;7(1):1557981. https://doi.org/10.1080/20016689.2018.1557981.
Sanders GD, Neumann PJ, Basu A, Brock DW, Feeny D, Krahn M, et al. Recommendations for conduct, methodological practices, and reporting of cost-effectiveness analyses: second panel on cost-effectiveness in health and medicine. JAMA. 2016;316(10):1093–103. https://doi.org/10.1001/jama.2016.12195.
Bae S, Lee S, Bae EY, Jang S. Korean guidelines for pharmacoeconomic evaluation (second and updated version): consensus and compromise. Pharmacoeconomics. 2013;31(4):257–67. https://doi.org/10.1007/s40273-012-0021-6.
Research group on economic evaluation for Japanese public medical benefits. Guideline for Economic Evaluation of Healthcare Technologies in Japan [in Japanese]. 2013. https://jglobal.jst.go.jp/en/detail?JGLOBAL_ID=201402257860290440. Accessed 16 June 2019
National Institute for Health and Care Excellence. Guide to the Methods of Technology Appraisal 2013. London: National Institute for Health and Care Excellence (NICE); 4 Apr 2013. Process and Methods Guides No. 9. PMID: 27905712. https://www.nice.org.uk/process/pmg9/resources/guide-to-the-methods-of-technology-appraisal-2013-pdf-2007975843781Accessed 9 Feb 2020
Drummond MF, Schwartz JS, Jonsson B, Luce BR, Neumann PJ, Siebert U, et al. Key principles for the improved conduct of health technology assessments for resource allocation decisions. Int J Technol Assess Health Care. 2008;24(3):244–58. https://doi.org/10.1017/s0266462308080343 (discussion 362-8).
China Center for Health Economic Research. China guidelines for pharmacoeconomic evaluations [in Chinese]. 2011. https://tools.ispor.org/PEguidelines/source/China-Guidelines-for-Pharmacoeconomic-Evaluations_2011_Chinese.pdf
Briggs ACK, Sculpher M. Decision modelling for health economic evaluation. New York: Oxford University Press; 2006.
Canadian Association for Drugs and Technologies in Health. Guidelines for the economic evaluation of health technologies: Canada. 4th ed. 2018. https://www.cadth.ca/about-cadth/how-we-do-it/methods-and-guidelines/guidelines-for-the-economic-evaluation-of-health-technologies-canada. Accessed 29 June 2019
Wan X, Peng L, Li Y. A review and comparison of methods for recreating individual patient data from published Kaplan–Meier survival curves for economic evaluations: a simulation study. PLoS ONE. 2015;10(3):e0121353. https://doi.org/10.1371/journal.pone.0121353.
Bell Gorrod H, Kearns B, Stevens J, Thokala P, Labeit A, Latimer N, et al. A review of survival analysis methods used in NICE technology appraisals of cancer treatments: consistency, limitations, and areas for improvement. Med Decis Mak Int J Soc Med Decis Mak. 2019;39(8):899–909. https://doi.org/10.1177/0272989x19881967.
Benedict A, Muszbek N. Survival modelling in UK oncology technology appraisals since the publication of good practice guidelines. The Evidence Forum; 2018.
Latimer N. NICE DSU Technical Support Document 14: undertaking survival analysis for economic evaluations alongside clinical trials—extrapolation with patient-level data. 2011. http://nicedsu.org.uk/wp-content/uploads/2016/03/NICE-DSU-TSD-Survival-analysis.updated-March-2013.v2.pdf. Accessed 20 Sep 2019
Masucci L, Beca J, Sabharwal M, Hoch JS. Methodological issues in economic evaluations submitted to the Pan-Canadian oncology drug review (pCODR). Pharmacoecon Open. 2017;1(4):255–63. https://doi.org/10.1007/s41669-017-0018-3.
Valachis A, Polyzos NP, Nearchou A, Lind P, Mauri D. Financial relationships in economic analyses of targeted therapies in oncology. J Clin Oncol. 2012;30(12):1316–20. https://doi.org/10.1200/jco.2011.38.6078.
Gibson EJ, Begum N, Koblbauer I, Dranitsaris G, Liew D, McEwan P, Tahami Monfared AA, Yuan Y, Juarez-Garcia A, Tyas D, Lees M. Modeling the economic outcomes of immuno-oncology drugs: alternative model frameworks to capture clinical outcomes. Clinicoecon Outcomes Res. 2018;10:139–54.
Gibson EJ, Begum N, Koblbauer I, Dranitsaris G, Liew D, McEwan P, et al. Cohort versus patient level simulation for the economic evaluation of single versus combination immuno-oncology therapies in metastatic melanoma. J Med Econ. 2019;22(6):531–44.
Lu S, Ye M, Ding L, Tan F, Fu J, Wu B. Cost-effectiveness of gefitinib, icotinib, and pemetrexed-based chemotherapy as first-line treatments for advanced non-small cell lung cancer in China. Oncotarget. 2017;8(6):9996–10006. https://doi.org/10.18632/oncotarget.14310.
Coyle D, Ko YJ, Coyle K, Saluja R, Shah K, Lien K, et al. Cost-effectiveness analysis of systemic therapies in advanced pancreatic cancer in the Canadian Health Care System. Value Health. 2017;20(4):586–92. https://doi.org/10.1016/j.jval.2016.11.002.
Bullement A, Cranmer HL, Shields GE. A review of recent decision-analytic models used to evaluate the economic value of cancer treatments. Appl Health Econ Health Policy. 2019;17(6):771–80. https://doi.org/10.1007/s40258-019-00513-3.
Pan F, Reifsnider O, Zheng Y, Proskorovsky I, Li T, He J, et al. Modeling clinical outcomes in prostate cancer: application and validation of the discrete event simulation approach. Value Health. 2018;21(4):416–22. https://doi.org/10.1016/j.jval.2017.09.022.
Karnon J, Haji A, Afzali H. When to use discrete event simulation (DES) for the economic evaluation of health technologies? A review and critique of the costs and benefits of DES. Pharmacoeconomics. 2014;32(6):547–58. https://doi.org/10.1007/s40273-014-0147-9.
Wang HI, Roman E, Crouch S, Aas E, Burton C, Patmore R, et al. A generic model for follicular lymphoma: predicting cost, life expectancy, and quality-adjusted-life-year using UK population-based observational data. Value Health. 2018;21(10):1176–85. https://doi.org/10.1016/j.jval.2018.03.007.
Tappenden P, Chilcott J, Brennan A, Squires H, Stevenson M. Whole disease modeling to inform resource allocation decisions in cancer: a methodological framework. Value Health. 2012;15(8):1127–36. https://doi.org/10.1016/j.jval.2012.07.008.
Williams C, Lewsey JD, Mackay DF, Briggs AH. Estimation of survival probabilities for use in cost-effectiveness analyses: a comparison of a multi-state modeling survival analysis approach with partitioned survival and Markov decision-analytic modeling. Med Decis Mak. 2016;37(4):427–39. https://doi.org/10.1177/0272989X16670617.
Williams C, Lewsey J, Briggs A, Mackay D. Cost-effectiveness analysis in R using a multi-state modeling survival analysis framework: a tutorial. Med Decis Mak. 2017;37(4):340–52. https://doi.org/10.1177/0272989X16651869.
Othus M, Bansal A, Koepl L, Wagner S, Ramsey S. Accounting for cured patients in cost-effectiveness analysis. Value Health. 2017;20(4):705–9. https://doi.org/10.1016/j.jval.2016.04.011.
Ishak J, Villalobos CF, Proskorovsky I. Projecting survival with cure mixture models: when are the data mature enough for reliable analysis? Value in Health. 2018;21:S53. https://doi.org/10.1016/j.jval.2018.04.443.
Whittington M. Value assessment methods and pricing recommendations for potential cures: a technical brief. 2019. https://icer-review.org/wp-content/uploads/2019/08/Valuing-a-Cure-Technical-Brief.pdf. Accessed 1 July 2020
Author information
Authors and Affiliations
Corresponding author
Ethics declarations
Funding
No funding was received for this work.
Conflict of interest
Graeme Ball is an employee of Gilead Sciences, Inc. (Canada). Jean-Eric Tarride, Mitch Levine, and Lehana Thabane have no conflicts of interest that are directly relevant to the content of this article.
Availability of data and material
The authors declare that all data used in this study are derived from the publicly available published oncology literature. The Excel spreadsheets used for the analysis can be provided upon reasonable request.
Code availability
Not applicable
Author contributions
GB collected and analyzed the data, and drafted the manuscript. JET contributed to the design of the study, and the analysis and interpretation of the data. JET, ML, and LT conceptualized the study and contributed to the revision of the manuscript. All authors gave final approval for the version to be published.
Electronic supplementary material
Below is the link to the electronic supplementary material.
Appendices
Appendix
Search strategies
Ovid Medline


Embase

Rights and permissions
Open Access This article is licensed under a Creative Commons Attribution-NonCommercial 4.0 International License, which permits any non-commercial use, sharing, adaptation, distribution and reproduction in any medium or format, as long as you give appropriate credit to the original author(s) and the source, provide a link to the Creative Commons licence, and indicate if changes were made. The images or other third party material in this article are included in the article's Creative Commons licence, unless indicated otherwise in a credit line to the material. If material is not included in the article's Creative Commons licence and your intended use is not permitted by statutory regulation or exceeds the permitted use, you will need to obtain permission directly from the copyright holder. To view a copy of this licence, visit http://creativecommons.org/licenses/by-nc/4.0/.
About this article
Cite this article
Ball, G., Levine, M., Thabane, L. et al. Onwards and Upwards: A Systematic Survey of Economic Evaluation Methods in Oncology. PharmacoEconomics Open 5, 397–410 (2021). https://doi.org/10.1007/s41669-021-00263-w
Accepted:
Published:
Issue Date:
DOI: https://doi.org/10.1007/s41669-021-00263-w