Abstract
In this study, uniaxial compression tests and simultaneous acoustic emission (AE) monitoring were carried out on four rocks (yellow sandstone, white sandstone, marble and limestone). The mechanical properties and AE energy evolution characteristics of different rocks were analysed. With the help of critical slowing down (CSD) theory, the AE precursor characteristics of their failure were investigated. It is pointed out that the AE during rock loading has a CSD phenomenon. A sudden change in the variance of one of the CSD indicators can be regarded as a precursor to failure, and it has the advantage of being more accurate and sensitive to failure than the autocorrelation coefficient. The stress level of a rock's failure precursor is closely related to its brittleness characteristics. The higher the brittleness of the rock, the more backward the failure precursor is, and the more difficult the early warning is. The study aims to provide new indicators and references for the monitoring and early warning of rockbursts and other disasters induced by rock fracture in deep underground engineering.
Article highlights
-
1.
Analyzed the mechanical properties and acoustic emission response of different rocks.
-
2.
The critical slowing down theory was introduced to explore the precursor characteristics of acoustic emission for different rock failures.
-
3.
The precursor of rock failure is closely related to its brittleness and energy evolution.
Similar content being viewed by others
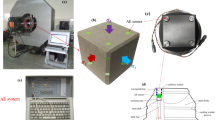
1 Introduction
In deep underground rock engineering, such as tunnelling and coal mining, the rock is often in an extremely high geostress condition. Rock bursts, roof breakage and other disasters induced by rock fracture under high stress conditions seriously affect the safe construction of the project (He et al. 2005; Li et al. 2020, 2023b; Zhao et al. 2021; Ma et al. 2023). Therefore, monitoring and early warning of rock fracture, especially the determination and identification of the precursors of failure, are very important. Accurate identification of failure precursors and timely preventive treatment can reduce casualties and economic losses.
Acoustic emission (AE) is a transient elastic wave emitted locally by a material as a result of a rapid release of energy. It has a wide range of applications in the field of rock mechanics and engineering as a powerful geophysical tool for rock stability monitoring (Hirata et al. 2007; Cai et al. 2007; Přikryl et al. 2003; Lockner 1993; Ohnaka and Mogi 1982). The AE signals during rock fracture can invert the fracture mechanism, location, scale, and deeply reflect the fracture activity and damage degree. Common analysis methods mainly include parametric analysis and waveform analysis. The parametric method mainly analyses the characteristic parameters of AE (e.g., counts, energy, amplitude, etc.) to characterize the progressive damage process of rocks (Moradian et al. 2010; Li et al. 2021a). The RA (ratio of rise time to amplitude) and AF (average frequency) values of the AE can effectively reflect the tension or shear mechanism of the fracture (Ohno and Ohtsu 2021). The waveform method focuses on time–frequency conversion of signals to obtain the frequency characteristics of the signal (Soma et al. 2002). Large-scale ruptures tend to correspond to low-frequency signals, and the opposite is true for small-scale signals (Jiang et al. 2021). The time-series evolution of AE signal characteristics has received much attention from scholars in the hope of identifying precursors of failure. Sudden changes in the timing parameters of the AE, as well as in the frequency, can be regarded as a sign of fracture. A decrease in the b-value of the AE is also considered to be a precursor of a major fracture (Dong et al. 2022). Fractal theory was applied to further reveal structural features of the data for AE and to provide new indicators (Kong et al. 2022). A number of artificial intelligence methods have also been pursued (Li et al. 2023a; Di et al. 2023).
Critical slowing down (CSD) theory describes the phenomenon of distributed fluctuations that promote the generation of a new phase near a critical point before the dynamical system switches from one phase to another. Such distributed fluctuations are usually characterised by increased time, slower recovery and reduced resilience (Zhang et al. 2021). Rock failure and instability are self-organised critical behaviours of a system that is transitioning from a stable to an unstable state. Therefore, it is possible to introduce CSD theory to study AE precursors of rock failure. Some studies have been carried out. For example, Shen et al. (2020) used CSD to study the change characteristics of infrared radiation during loading of water-bearing rocks. The AE signals of water-bearing rocks were analysed with CSD by Li et al. (2021b). Zhou et al. (2023) used CSD to investigate the AE precursors of rock failure after freeze–thaw cycles. However, due to the complexity of the stratigraphy in underground engineering, it is necessary to investigate the failure precursors of different types of rocks and to verify the applicability of the CSD theory to different rocks.
Therefore, in this paper, four rocks were selected to carry out uniaxial compression experiments, including yellow sandstone, white sandstone, marble and limestone. Moreover, the AE monitoring was also carried out simultaneously. The mechanical properties and AE response characteristics of different rocks were analysed. The CSD theory was introduced to investigate the AE precursors of the failure of different rocks. Finally, the correlation between the brittleness, energy evolution behaviour of the rocks and the failure precursors was explored. The study aims to provide new indicators and references for the monitoring and early warning of rockbursts and other disasters in deep underground engineering.
2 Experimental procedure
2.1 Sample preparation
Four different rocks were used to carry out the study. These were yellow sandstone (YS), white sandstone (WS), marble (M) and limestone (L). Their densities are 1.8 g/cm3, 2.7 g/cm3, 3.1 g/cm3 and 2.4 g/cm3, respectively. They were machined into standard cylindrical samples with a diameter of 50 mm and a height of 100 mm for uniaxial mechanical property testing according to the standards of the International Society of Rock Mechanics. The specimen is physically shown in Fig. 1. X-ray diffraction tests were also carried out to derive their specific mineralogical compositions as shown in Table 1. Figure 2 shows their microstructural image obtained by polarised light microscopy observation.
2.2 Test programme
As shown in Fig. 3, the test system mainly consists of a loading system and an AE monitoring system. The loading system adopted the new SANS microcomputer-controlled electro-hydraulic servo pressure testing machine. The AE acquisition system adopted the 24-channel Micro-II type AE monitoring host of American Physical Acoustics Corporation with a NANO-30 AE probe and a preamplifier. The centre frequency of the AE probe used for the experiment was 150 kHz. During the experiment, the loading rate of the press was set to 500 N/s, and the threshold, amplification and acquisition frequency of the AE signal acquisition were set to 40 dB, 40 dB and 2 × 106/s, respectively. The AE probe was placed close to the surface of the specimen and a lead break test (Dahmene et al. 2016) was carried out, all to ensure that the signals from the rock fracture were captured in their entirety. Turn on the press and AE acquisition simultaneously. Load until the specimen was fully destroyed. Each rock has three replicate specimens. Table 2 gives the numbers and test results of all specimens.
3 Experimental results and analysis
3.1 Mechanical properties
Figure 4 illustrates the stress–strain curves for the different rocks used in the experiment. All specimens underwent approximately four stages of the loading process, including compaction, elastic deformation, crack propagation and failure. The compaction phase had an upward concave curve, due to the closure of the original pores and cracks. This was followed by elastic deformation, when the stress increased linearly with strain. With the increase of stress, the cracks inside the specimen developed and expanded, the damage intensified, and the curve gradually became non-linear. The strain hardening behaviour was exhibited. Loaded to peak stress (i.e., uniaxial compressive strength, UCS), the specimen will fail completely, with a gradual drop in stress.
The mechanical parameters, including UCS and elastic modulus E, of all the rock specimens are statistically presented in Fig. 5. Specifically, the UCS of yellow sandstone, white sandstone, marble and limestone specimens averaged 38 MPa, 70.1 MPa, 51.6 MPa and 45.5 MPa, respectively, whereas the E were 5.8 GPa, 11.3 GPa, 13.3 GPa and 8.6 GPa, respectively.
3.2 AE time-series response
Figure 6 shows the time series variation of the AE energy during the loading of specimens from different rocks. In general, the AE energy variations reflected the deformation as well as the fracture process of the specimens well. In the early stage of loading, there were some fluctuations in the AE energy during the compaction phase due to the random character of the original cracks and pores. During the elastic deformation phase, there was basically no fracture inside the specimen, so the AE was less active and the energy value stayed at a very low level. In the late stage of loading, a large number of cracks developed and expanded as well as penetrated inside the specimen, and the AE response was strong with high energy values. Furthermore, after loading to peak stress, the AE energy also peaked when failure occurred. It seems that the peak AE energy of a rock with high strength is also higher, due to the fact that its failure is more violent. Specifically, the peak AE energy of white sandstone could reach 1800 mV μs, which was significantly higher than other rocks. It also had the highest strength of the four rocks.
4 Critical slowing down based precursor extraction
4.1 Critical slowing down theory
Variance and autocorrelation coefficient (AC) are statistical parameters used to quantitatively characterise the CSD phenomenon (Zhang et al. 2021; Li et al. 2021b; Kong et al. 2015). In this paper, the AE energy parameters reflecting the fracture activity were taken as representative parameters for subsequent analyses in order to reveal the CSD characteristics of the rock loading process. The precursor features of the failure were extracted by characterising the change of its steady state.
The variance, denoted s2, is the characteristic quantity that describes the deviation of the data from the mean value \(\overline{x}\). S is the standard deviation. Specifically, it can be written as:
where xi is the ith datum; n is the number of datums in the sequence.
The autocorrelation coefficient describes the correlation of the same variable at different times. When the lag length of the variable x is j, the autocorrelation coefficient a(j) can be expressed as:
Assuming that there is a forced disturbance in the state variable with period ∆t, the equilibrium regression is approximated as an exponential regression with a recovery rate of γ during the disturbance. The following equation exists in the regression model:
where yn is the deviation of the system variables to equilibrium; βn is a normally distributed random quantity.
When γ and ∆t do not depend on yn, Eq. 4 reduces to the first-order autoregressive model AR(1):
Analyze the AR(1) process through variance:
where Var is the variance; M is the mathematical expectation.
As the system approaches the critical point, the recovery rate for small amplitude perturbations decreases. When the system approaches the critical point, the recovery rate γ tends to 0 and the AC tends to 1. From Eq. 6, the variance tends to infinity, so the increase of variance and AC can be used as a precursor signal that the system is approaching the critical point.
4.2 Window length and lag length
When calculating variance and AC, the appropriate window length and lag length should be determined. The window length refers to a selected sequence containing a specific amount of data, and the lag length refers to the lag from the sequence containing a specific amount of data to a new sequence with the same window length.
Taking the AE energy sequence of YS-1 specimen as an example, the effect of different window lengths on the change of CSD indicator was studied with the lag length fixed at 100. Figure 7 illustrates the results of the calculations. Overall, the window length had essentially no effect on the timing of abrupt changes in variance and AC, only on the magnitude of the rise and fall of the curve. The longer the window length, the smaller the magnitude of the change in the indicators. Then, the effect of the lag length on the indicator change was explored with the window length fixed at 200. Figure 8 illustrates the calculation results. The lag length did not affect the variance. It had some effect on the AC. Usually the larger the lag length, the weaker the data correlation (Li et al. 2021b). It is important to choose the appropriate window length and lag length according to different data lengths.
4.3 Precursor recognition
Here a window length of 200 and a lag length of 100 were chosen to calculate the CSD indicators of the AE energy during loading of different rock specimens. Figure 9 shows the final calculation results. During the early stages of loading, mainly the compaction and elastic deformation phases, the specimen was internally stable. Even if there were small cracks, the system would return to a stable loading state relatively quickly due to the fast recovery rate. The AE signals were weak, and therefore the variance and AC did not change much. In the later stages of loading, a large number of cracks expanded erratically and the specimen gradually lost its ability to return to its original state. The AE response was strong and exhibited the phenomenon of CSD. Variance and AC showed growth. In general, the variance was essentially unchanged in the earlier period and had a high sensitivity to fracture in the later period, where the abrupt change was significant. Although the AC also showed an increasing trend in the later stages, its overall change was choppy and not conducive to the judgement of failure precursors. There were fewer pseudo-signals of variance, so this indicator is recommended as an important reference indicator for rock failure monitoring. In addition, the direct use of the AE energy parameter as a precursor indicator has the problem of difficulty in selecting the critical value in engineering. The CSD theory further exploits the evolutionary and structural characteristics of AE data, which is more valuable for failure warning in engineering. Considering the sudden change point of variance as the precursor point of failure, the precursor time point of each specimen is labelled in Fig. 9. For example, the YS-1 specimen destroyed at 152.8 s, and its precursor point occurred at 131.6 s of loading. The ratio of stress at the precursor point to peak stress was counted for all specimens and the results are shown in Fig. 10. The precursors for marble were the most advanced, averaging at 84% of peak stress. Whereas, the precursors of failure in white sandstone were on average at 98% of the peak stress, making early warning of its failure the most difficult.
5 Discussions
In fact, the failure of a loaded rock is a strain energy-driven state instability (Pan and Lu 2018; Wang et al. 2022). During loading, part of the work done by the environment is stored in the rock as elastic strain energy, and the rest is dissipated due to fracture and irreversible deformation. The calculation formulas for the total energy U, elastic energy Ue, and dissipated energy Ud input from the outside is as follows (Li et al. 2021a, b; Ma et al. 2020):
Their evolution is schematically illustrated in Fig. 11. The response of AE as a radiated signal reflecting the release of local strain energy implies the intensification of damage and fracture within the specimen, which is essentially an energy dissipation behaviour. Figure 12 shows the evolution of the variance of AE versus dissipation energy for different rock specimens. The evolution of variance index is synchronous with the change of dissipated energy.
Rocks with more significant pre-peak energy dissipation tend to have more advanced precursors. For example, the marble in the test had a more significant strain-hardening behaviour before peak stress. A significant amount of strain energy was dissipated during the crack propagation phase prior to final failure. AE also responded significantly, so its precursor was more advanced. In contrast, white sandstone had less pre-peak energy dissipation and its precursor point was very late. The ratio of pre-peak energy dissipation actually reflects the brittleness characteristics of the rock material (Wang et al. 2022). Specifically, it can be evaluated by a classical brittleness index BI (Hucka and Das 1974):
where Uep is the strain energy stored within the specimen at peak stress, and Up is the total strain energy input at peak stress. Higher BI means more brittle rock. The brittleness index BI was calculated for all the specimens and its relationship with the stress level of the precursor was explored. The results are shown in Fig. 13. There is a significant positive correlation between the brittleness index and the stress level of the precursor, which can be well described by a linear function. In general, brittle rocks have less dissipated energy before the peak and their failure is sudden and therefore more difficult to be warned. The brittleness of rock materials is an inherent material property that depends on their material composition, cementation, and microstructure.
Moreover, this is closely related to the proneness dynamic hazards such as rockbursts. It is suggested that reducing the brittleness of rocks by means of some material modification, such as water immersion (Li et al. 2021a, b; Li et al. 2023c) or heat treatment (Xu et al. 2022), may reduce the difficulty of warning of rock failure and may reduce the risk of rock bursts.
6 Conclusions
In this paper, uniaxial compression tests were carried out for four types of rocks, including yellow sandstone, white sandstone, marble and limestone. The acoustic emission (AE) signals during the loading process were also monitored. The mechanical properties of different rocks and the time-series changes of AE energy parameters were analysed. The critical slowing down (CSD) theory was introduced to investigate the AE precursors of rock failure. The main conclusions are as follows:
-
1.
AE energy during rock loading has a CSD phenomenon. At the late stage of loading, the CSD indicators variance and autocorrelation coefficient (AC) grow. The larger the window length, the smaller the fluctuation of variance curve and AC curve. The lag length has no effect on the variance, but it affects the rise and fall of the AC curve.
-
2.
The direct use of AE energy as a monitoring indicator has the drawback of difficult selection of warning values, while the CSD indicator further exploits the evolutionary and structural characteristics of the data, which is of broad engineering significance. The change in variance is more accurate compared to the AC and is more sensitive to fracture. Abrupt changes in variance are suggested as precursors of rock failure.
-
3.
The more brittle the rock, the more sudden the failure and the more difficult it is to warn of the failure. The brittleness of the rock has a positive correlation with the stress level at the failure precursor point. This is related to the law of energy dissipation during loading. It is recommended that rock brittleness be attenuated by means of material modification in order to reduce the risk of rockburst and the difficulty of AE warning.
Availability of data and materials
The related data used to support the findings of this study are included within the article.
References
Cai M, Morioka H, Kaiser PK, Tasaka Y, Kurose H, Minami M, Maejima T (2007) Back-analysis of rock mass strength parameters using AE monitoring data. Int J Rock Mech Min Sci 44:538–549
Dahmene F, Yaacoubi S, El Mountassir M, Bendaoud N, Langlois C, Bardoux O (2016) On the modal acoustic emission testing of composite structure. Compos Struct 140:446–452
Di YY, Wang EY, Huang T (2023) Identification method for microseismic, acoustic emission and electromagnetic radiation interference signals of rock burst based on deep neural networks. Int J Rock Mech Min Sci 170:105541
Dong LJ, Zhang LY, Liu HN, Du K, Liu XL (2022) Acoustic emission b value characteristics of granite under true triaxial stress. Mathematics 10:451
He MC, Xie HP, Peng SP, Jiang YD (2005) Study on rock mechanics in deep mining engineering. Chin J Rock Mech Eng 24(16):2803–2813
Hirata A, Kameoka Y, Hirano T (2007) Safety management based on detection of possible rock bursts by AE monitoring during tunnel excavation. Rock Mech Rock Eng 40:563–576
Hucka V, Das B (1974) Brittleness determination of rocks by different methods. Int J Rock Mech Min Sci Geomech Abstr 11:389–392
Jiang RC, Dai F, Liu Y, Li A, Feng P (2021) Frequency characteristics of acoustic emissions induced by crack propagation in rock tensile fracture. Rock Mech Rock Eng 54:2053–2065
Kong XG, Wang EY, Hu SB, Li ZH, Liu XF, Fang BF, Zhan TQ (2015) Critical slowing down on acoustic emission characteristics of coal containing methane. J Nat Gas Sci Eng 24:156–165
Kong B, Zhuang ZD, Zhang XY, Jia S, Lu W, Zhang XY, Zhang WR (2022) A study on fractal characteristics of acoustic emission under multiple heating and loading damage conditions. J Appl Geophys 197:104532
Li XL, Cao ZY, Xu YL (2020) Characteristics and trends of coal mine safety development. Energy Sources Part A Recov Util Environ Eff. https://doi.org/10.1080/15567036.2020.1852339
Li HR, Qiao YF, Shen RX, He MC, Cheng T, Xiao YM, Tang J (2021a) Effect of water on mechanical behavior and acoustic emission response of sandstone during loading process: phenomenon and mechanism. Eng Geol 294:106386
Li HR, Shen RX, Qiao YF, He MC (2021b) Acoustic emission signal characteristics and its critical slowing down phenomenon during the loading process of water-bearing sandstone. J Appl Geophys 194:104458
Li BL, Wang EY, Li ZH, Cao X, Liu XF, Zhang M (2023a) Automatic recognition of effective and interference signals based on machine learning: a case study of acoustic emission and electromagnetic radiation. Int J Rock Mech Min Sci 170:105505
Li HR, He MC, Qiao YF, Cheng T, Xiao YM, Gu ZJ (2023b) Mode I fracture properties and energy partitioning of sandstone under coupled static-dynamic loading: implications for rockburst. Theoret Appl Fract Mech 127:104025
Li HR, Qiao YF, He MC, Shen RX, Gu ZJ, Cheng T, Xiao YM, Tang J (2023c) Effect of water saturation on dynamic behavior of sandstone after wetting-drying cycles. Eng Geol 319:107105
Lockner D (1993) The role of acoustic emission in the study of rock fracture. Int J Rock Mech Min Sci Geomech Abstr 30:883–899
Ma Q, Tan YL, Liu XS, Gu QH, Li XB (2020) Effect of coal thicknesses on energy evolution characteristics of roof rock-coal-floor rock sandwich composite structure and its damage constitutive model. Compos B 198:108086
Ma Q, Liu XL, Tan YL et al (2023) Numerical study of mechanical properties and microcrack evolution of double-layer composite rock specimens with fissures under uniaxial compression. Eng Fract Mech 289(2):109403
Moradian ZA, Ballivy G, Rivard P, Gravel C, Rousseau B (2010) Evaluating damage during shear tests of rock joints using acoustic emissions. Int J Rock Mech Min Sci 47:590–598
Ohnaka M, Mogi K (1982) Frequency characteristics of acoustic emission in rocks under uniaxial compression and its relation to the fracturing process to failure. J Geophys Res 87:3873–3884
Ohno K, Ohtsu M (2021) Crack classification in concrete based on acoustic emission. Constr Build Mater 24:2339–2346
Pan XH, Lu Q (2018) A quantitative strain energy indicator for predicting the failure of laboratory-scale rock samples: application to shale rock. Rock Mech Rock Eng 51:2689–2707
Přikryl R, Lokajíček T, Li C, Rudajev V (2003) Acoustic emission characteristics and failure of uniaxially stressed granitic rocks: the effect of rock fabric. Rock Mech Rock Eng 36:255–270
Shen RX, Li HR, Wang EY, Chen TQ, Li TX, Tian H, Hou ZH (2020) Infrared radiation characteristics and fracture precursor information extraction of loaded sandstone samples with varying moisture contents. Int J Rock Mech Min Sci 130:104344
Soma N, Niitsuma H, Baria R (2002) Evaluation of subsurface structure at Soultz hot dry rock site by the AE reflection method in time-frequency domain. Pure Appl Geophys 159:543–562
Wang W, Wang Y, Chai B, Du J, Xing LX, Xia ZH (2022) An energy-based method to determine rock brittleness by considering rock damage. Rock Mech Rock Eng 55:1585–1597
Xu L, Gong FQ, Liu ZX (2022) Experiments on rockburst proneness of pre-heated granite at different temperatures: Insights from energy storage, dissipation and surplus. J Rock Mech Geotech Eng 14:1343–1355
Zhang ZH, Li YC, Hu LH, Tang CA, Zheng HC (2021) Predicting rock failure with the critical slowing down theory. Eng Geol 280:105960
Zhao ZH, Sun W, Chen SJ et al (2021) Determination of critical criterion of tensile-shear failure in Brazilian disc based on theoretical analysis and meso-macro numerical simulation. Comput Geotech 134:104096
Zhou ZL, Ullah B, Rui YC, Cai X, Lu JY (2023) Predicting the failure of different rocks subjected to freeze-thaw weathering using critical slowing down theory on acoustic emission characteristics. Eng Geol 316:107059
Funding
This work was financially supported by the National Natural Science Foundation of China (51974305) and Fundamental Research Funds for the Central Universities (2022XSCX32).
Author information
Authors and Affiliations
Contributions
JCO: Conceptualization, Methodology, Writing-original draft. EYW: Project administration. XYW: Investigation, Writing-Reviewing and Editing.
Corresponding author
Ethics declarations
Ethics approval and consent to participate
Not applicable.
Consent for publication
The authors agree with the publication of the manuscript.
Competing interests
The authors declare that we have no known competing financial interests or personal relationships that could have appeared to influence the work reported in this paper.
Additional information
Publisher's Note
Springer Nature remains neutral with regard to jurisdictional claims in published maps and institutional affiliations.
Rights and permissions
Open Access This article is licensed under a Creative Commons Attribution 4.0 International License, which permits use, sharing, adaptation, distribution and reproduction in any medium or format, as long as you give appropriate credit to the original author(s) and the source, provide a link to the Creative Commons licence, and indicate if changes were made. The images or other third party material in this article are included in the article's Creative Commons licence, unless indicated otherwise in a credit line to the material. If material is not included in the article's Creative Commons licence and your intended use is not permitted by statutory regulation or exceeds the permitted use, you will need to obtain permission directly from the copyright holder. To view a copy of this licence, visit http://creativecommons.org/licenses/by/4.0/.
About this article
Cite this article
Ou, J., Wang, E. & Wang, X. Failure precursor characteristics of different types of rocks under load: insights from critical slowing down of acoustic emission. Geomech. Geophys. Geo-energ. Geo-resour. 9, 161 (2023). https://doi.org/10.1007/s40948-023-00712-2
Received:
Accepted:
Published:
DOI: https://doi.org/10.1007/s40948-023-00712-2