Abstract
Segmentation of organs and lesions could be employed for the express purpose of dosimetry in nuclear medicine, assisted image interpretations, and mass image processing studies. Deep leaning created liver and liver lesion segmentation on clinical 3D MRI data has not been fully addressed in previous experiments. To this end, the required data were collected from 128 patients, including their T1w and T2w MRI images, and ground truth labels of the liver and liver lesions were generated. The collection of 110 T1w-T2w MRI image sets was divided, with 94 designated for training and 16 for validation. Furthermore, 18 more datasets were separately allocated for use as hold-out test datasets. The T1w and T2w MRI images were preprocessed into a two-channel format so that they were used as inputs to the deep learning model based on the Isensee 2017 network. To calculate the final Dice coefficient of the network performance on test datasets, the binary average of T1w and T2w predicted images was used. The deep learning model could segment all 18 test cases, with an average Dice coefficient of 88% for the liver and 53% for the liver tumor. Liver segmentation was carried out with rather a high accuracy; this could be achieved for liver dosimetry during systemic or selective radiation therapies as well as for attenuation correction in PET/MRI scanners. Nevertheless, the delineation of liver lesions was not optimal; therefore, tumor detection was not practical by the proposed method on clinical data.






Similar content being viewed by others
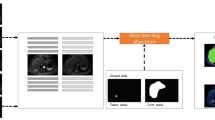
References
Tang X et al (2020) Whole liver segmentation based on deep learning and manual adjustment for clinical use in SIRT. Eur J Nucl Med Mol Imaging 47:2742–2752
Rahmani R et al (2019) Improved diagnostic accuracy for myocardial perfusion imaging using artificial neural networks on different input variables including clinical and quantification data. Revista Española De Medicina Nuclear E Imagen Molecular (English Edition) 38(5):275–279
Eftekhari M et al (2018) Automated interpretation of myocardial perfusion images with multilayer perceptron network as a decision support system. J Med Imaging Health Inf 8(9):1844–1849
Gholipour C et al (2009) Prediction of conversion of laparoscopic cholecystectomy to open surgery with artificial neural networks. BMC Surg 9(1):1–6
Zhen S-h et al (2020) Deep learning for accurate diagnosis of liver tumor based on magnetic resonance imaging and clinical data. Front Oncol 10:680
Hu P et al (2016) Automatic 3D liver segmentation based on deep learning and globally optimized surface evolution. Phys Med Biol 61(24):8676
Dou Q et al (2016) 3D deeply supervised network for automatic liver segmentation from CT volumes. In: Ourselin S et al (eds) Medical Image Computing and Computer-Assisted Intervention–MICCAI 2016: 19th International Conference, Athens, Greece, October 17–21, 2016, Proceedings, Part II 19. Springer
Jansen MJ et al (2019) Liver segmentation and metastases detection in MR images using convolutional neural networks. J Med Imaging 6(4):044003–044003
Jansen MJ, Kuijf HJ, Pluim JP (2019) Optimal input configuration of dynamic contrast enhanced MRI in convolutional neural networks for liver segmentation. In: Medical imaging 2019: image Processing. International Society for Optics and Photonics
Masoumi H et al (2012) Automatic liver segmentation in MRI images using an iterative watershed algorithm and artificial neural network. Biomed Signal Process Control 7(5):429–437
Wang K et al (2019) Automated CT and MRI liver segmentation and biometry using a generalized convolutional neural network. Radiology: Artif Intell 1(2):180022
Tustison NJ et al (2010) N4ITK: improved N3 bias correction. IEEE Trans Med Imaging 29(6):1310–1320
Ahmad M et al (2019) Convolutional-neural-network-based feature extraction for liver segmentation from CT images. In: Eleventh International Conference on Digital Image Processing (ICDIP 2019). SPIE
Ahmad M et al (2018) Deep-stacked auto encoder for liver segmentation. In: Wang Y et al (eds) Advances in Image and Graphics Technologies: 12th Chinese conference, IGTA 2017, Beijing, China, June 30–July 1, 2017, Revised Selected Papers 12. Springer
Ahmad M et al (2019) Deep belief network modeling for automatic liver segmentation. IEEE Access 7:20585–20595
Ahmad M et al (2022) A lightweight convolutional neural network model for liver segmentation in medical diagnosis. Computational Intelligence and Neuroscience, 2022
Isensee F et al (2018) Brain tumor segmentation and radiomics survival prediction: Contribution to the BRATS 2017 challenge. In: Crimi A et al (eds) Brainlesion: Glioma, Multiple Sclerosis, Stroke and Traumatic Brain Injuries: Third International Workshop, BrainLes 2017, Held in Conjunction with MICCAI 2017, Quebec City, QC, Canada, September 14, 2017, Revised Selected Papers 3. Springer
Drozdzal M et al (2016) The importance of skip connections in biomedical image segmentation. In: Carneiro G et al (eds) Deep Learning and Data Labeling for Medical Applications. DLMIA LABELS 2016. Springer
Christ PF et al (2017) Automatic liver and tumor segmentation of CT and MRI volumes using cascaded fully convolutional neural networks. arXiv Preprint arXiv:1702.05970,
Pratondo A, Chui C-K, Ong S-H (2017) Integrating machine learning with region-based active contour models in medical image segmentation. J Vis Commun Image Represent 43:1–9
Häme Y (2008) Liver tumor segmentation using implicit surface evolution. Midas J: p. 1–10
Fallahpoor M et al (2022) Generalizability assessment of COVID-19 3D CT data for deep learning-based disease detection. Comput Biol Med 145:105464
Han X (2017) Automatic liver lesion segmentation using a deep convolutional neural network method. arXiv preprint arXiv:1704.07239,
Vorontsov E et al (2018) Liver lesion segmentation informed by joint liver segmentation. In: 2018 IEEE 15th International Symposium on Biomedical Imaging (ISBI 2018). IEEE
Acknowledgements
This study was supported by the Elite Research Grant Committee, with an award number (958298) from the National Institute for Medical Research Development (NIMAD), Tehran, Iran.
The authors keep the memory of their colleague, Dr. Habibollah Dashti, who passed away in his fight against cancer before reading the final version of the manuscript. We as many of his patients remember his expertise and essence of humanity in liver surgery and practice.
Author information
Authors and Affiliations
Corresponding author
Ethics declarations
Compliance with Ethical Standards
This article does not contain any studies on human participants or animals performed by any of the authors. The study was performed on the previously acquired images after the anonymization of the data.
Conflict of interest
The authors declare no conflicting interest.
Additional information
Publisher’s Note
Springer Nature remains neutral with regard to jurisdictional claims in published maps and institutional affiliations.
Rights and permissions
Springer Nature or its licensor (e.g. a society or other partner) holds exclusive rights to this article under a publishing agreement with the author(s) or other rightsholder(s); author self-archiving of the accepted manuscript version of this article is solely governed by the terms of such publishing agreement and applicable law.
About this article
Cite this article
Fallahpoor, M., Nguyen, D., Montahaei, E. et al. Segmentation of liver and liver lesions using deep learning. Phys Eng Sci Med (2024). https://doi.org/10.1007/s13246-024-01390-4
Received:
Accepted:
Published:
DOI: https://doi.org/10.1007/s13246-024-01390-4