Abstract
Emerging economies, while exhibiting higher growth rates compared to developed countries, are susceptible to external shocks, leading to financial fragility. Traditional analysis methods often fall short in accuracy and timeliness. This research introduces a novel approach utilizing Back-Propagation Neural Network (BPNN) to predict financial fragility in emerging markets, focusing on the BRICS countries. By considering twelve impactful factors and employing Principal Component Analysis (PCA), five key influencers are identified. The BPNN model is iteratively optimized to achieve superior quality. Historical data validation attests to the model’s effectiveness. The study identifies five critical factors influencing financial fragility: GDP growth rate, inflation rate, monetary policy, interest rates, and bank’s capital-asset ratio. Among these, GDP growth rate emerges as a significant determinant. Positive growth is correlated with financial stability, while a slowdown or negative growth signals elevated risks. Emerging markets are particularly vulnerable to global economic fluctuations due to their reliance on exports and foreign capital. Additionally, weaker financial systems amplify their susceptibility to shocks.The research underscores the importance of building robust financial sectors, replenishing funding buffers, and proactively managing distressed assets in emerging market economies. The proposed BPNN model provides a powerful tool for risk prediction, though it requires strong indicator data support. While computational intensity and interpretability remain challenges, the benefits of BPNNs outweigh these limitations. Effective communication and information exchange across countries and markets are crucial for maintaining stability in emerging market finance. This study contributes valuable insights into the prediction of financial fragility in emerging markets, offering a comprehensive framework for policymakers and financial practitioners to navigate the challenges and opportunities presented by these dynamic economies.






Similar content being viewed by others
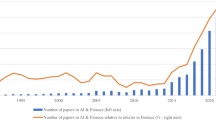
Data Availability
Data will be made available on request.
References
Anderloni, L., Bacchiocchi, E., & Vandone, D. (2012). Household financial vulnerability: An empirical analysis. Research in Economics, 66(3), 284–296.
Ariza-Garzón, M. J., Arroyo, J., Caparrini, A., & Segovia-Vargas, M. J. (2020). Explainability of a machine learning granting scoring model in peer-to-peer lending. Ieee Access, 8, 64873–64890.
Bhatore, S., Mohan, L., & Reddy, Y. R. (2020). Machine learning techniques for credit risk evaluation: A systematic literature review. Journal of Banking and Financial Technology, 4, 111–138.
Blazhenets, G., Ma, Y., Sörensen, A., Rücker, G., Schiller, F., Eidelberg, D., ... & Meyer, P. T. (2019). Principal components analysis of brain metabolism predicts development of Alzheimer dementia. Journal of Nuclear Medicine, 60(6), 837–843.
Bodley, P., & Mengzhe, C. (2020). Monetary Policy and Financial Vulnerability. China Finance, 2020(6), 14–17.
Bussmann, N., Giudici, P., Marinelli, D., & Papenbrock, J. (2021). Explainable machine learning in credit risk management. Computational Economics, 57, 203–216.
Chang, H., & Tiancai, X. (2018). Construction of China’s financial system vulnerability index system and decomposition of risk factors. Shanghai Finance, 2018(10), 12–22.
Chhatwani, M., & Mishra, S. K. (2021). Does financial literacy reduce financial fragility during COVID-19? The moderation effect of psychological, economic and social factors. International Journal of Bank Marketing, 39(7), 1114–1133.
Chong, B. U., & Kim, H. (2019). Capital structure volatility, financial vulnerability, and stock returns: Evidence from Korean firms. Finance Research Letters, 30, 318–326.
De Lucia, C., Pazienza, P., & Bartlett, M. (2020). Does good ESG lead to better financial performances by firms? Machine learning and logistic regression models of public enterprises in Europe. Sustainability, 12(13), 5317.
Demertzis, M., Domínguez-Jiménez, M., & Lusardi, A. (2020). The financial fragility of European households in the time of COVID-19 (No. 2020/15). Bruegel Policy Contribution.
Hu, H., Tang, L., Zhang, S., & Wang, H. (2018). Predicting the direction of stock markets using optimized neural networks with Google Trends. Neurocomputing, 285, 188–195.
Imerman, M. B., & Fabozzi, F. J. (2020). Cashing in on innovation: A taxonomy of FinTech. Journal of Asset Management, 21, 167–177.
Ke, Y. (2023). Cross market contagion of global systemic financial risks under the impact of economic policy uncertainty: A study based on TVP-FAVAR and TVP-VAR models. Statistical Research, 40(7), 70–84.
Li, C., Jin, K., Zhong, Z., Zhou, P., & Tang, K. (2021). Financial risk early warning model of listed companies under rough set theory using BPNN. Journal of Global Information Management (JGIM), 30(7), 1–18.
Li, X., Wang, J., & Yang, C. (2023). Risk prediction in financial management of listed companies based on optimized BP neural network under digital economy. Neural Computing and Applications, 35(3), 2045–2058.
Li, Y., Ni, P., & Chang, V. (2020). Application of deep reinforcement learning in stock trading strategies and stock forecasting. Computing, 102(6), 1305–1322.
Ma, C., Wu, J., Sun, H., Zhou, X., & Sun, X. (2023). Enhancing user experience in digital payments: A hybrid approach using SEM and neural networks. Finance Research Letters, 58, 104376.
Mayovets, Y., Vdovenko, N., Shevchuk, H., Zos-Kior, M., & Hnatenko, I. (2021). Simulation modeling of the financial risk of bankruptcy of agricultural enterprises in the context of COVID-19. Journal of Hygienic Engineering and Design, 36, 192–198.
Ofori-Okyere, I., Edghiem, F., & Kumah, S. P. (2023). Marketing inclusive banking services to financially vulnerable consumers: A service design approach. Journal of Services Marketing, 37(2), 232–247.
Safi, A., Chen, Y., Wahab, S., Ali, S., Yi, X., & Imran, M. (2021). Financial instability and consumption-based carbon emission in E-7 countries: The role of trade and economic growth. Sustainable Production and Consumption, 27, 383–391.
Samantaray, S., & Sahoo, A. (2020). Prediction of runoff using BPNN, FFBPNN, CFBPNN algorithm in arid watershed: A case study. International Journal of Knowledge-Based and Intelligent Engineering Systems, 24(3), 243–251.
Vučinić, M. (2020). Fintech and financial stability potential influence of FinTech on financial stability, risks and benefits. Journal of Central Banking Theory and Practice, 9(2), 43–66.
Warmenhoven, J., Cobley, S., Draper, C., Harrison, A., Bargary, N., & Smith, R. (2019). Considerations for the use of functional principal components analysis in sports biomechanics: Examples from on-water rowing. Sports Biomechanics, 18(3), 317–341.
Xian, H., Yang, Y., & Ting, H. (2019). Is the significant reversal of international capital flows a negative effect on the economic growth of emerging market countries – The adaptability of global capital outflow control. Studies of International Finance, 2019(7), 3–13.
Xiaofen, T., & Yuan, L. (2018). Debt of non-financial enterprises in emerging market countries: Current situation, causes, risks, and countermeasures. International Economic Review, 2018(5), 61–77.
Yang, G., & Guanglian, C. (2018). Analyzing financial cooperation between China and Central Asia from the perspective of financial vulnerability. New Finance, 2018(3), 17–21.
Yang, W., Zhao, Y., Wang, D., Wu, H., Lin, A., & He, L. (2020). Using principal components analysis and IDW interpolation to determine spatial and temporal changes of surface water quality of Xin’anjiang River in Huangshan, China. International Journal of Environmental Research and Public Health, 17(8), 2942.
Yang, X., Zhang, J., & Wu, Y. (2019). Grey evaluation of housing policy’s partition effect: Empirical test based on panel data from 35 large and medium-sized cities in China. Journal of Grey System, 31(1).
Yongqin, W., & Ding, Q. (2020). How financial innovation affects emerging market finance and economy: Concurrent discussion on China’s financial reform. The Journal of World Economy, 43(7), 146–169.
Zhang, H., & Luo, Y. (2022). Enterprise financial risk early warning using BP neural network under internet of things and rough set theory. Journal of Interconnection Networks, 22(03), 2145019.
Zhang, J., Qin, Z., Yin, H., Ou, L., & Zhang, K. (2019). A feature-hybrid malware variants detection using CNN based opcode embedding and BPNN based API embedding. Computers & Security, 84, 376–392.
Author information
Authors and Affiliations
Contributions
Among the authors, XS and PY contributed equally as first authors. FY and ZQ responsible for the language translation of this research. SY and XW are all corresponding authors who contribute equally to the article. All authors read and approved the final manuscript.
Corresponding authors
Ethics declarations
Ethics Approval
All experiments conducted in this study adhere to ethical requirements.
Competing Interest
The authors declare no competing interests.
Additional information
Publisher's Note
Springer Nature remains neutral with regard to jurisdictional claims in published maps and institutional affiliations.
This article is part of the Topical Collection on Innovation Management in Asia
Rights and permissions
Springer Nature or its licensor (e.g. a society or other partner) holds exclusive rights to this article under a publishing agreement with the author(s) or other rightsholder(s); author self-archiving of the accepted manuscript version of this article is solely governed by the terms of such publishing agreement and applicable law.
About this article
Cite this article
Sun, X., Yuan, P., Yao, F. et al. Financial Fragility in Emerging Markets: Examining the Innovative Applications of Machine Learning Design Methods. J Knowl Econ (2024). https://doi.org/10.1007/s13132-023-01731-w
Received:
Accepted:
Published:
DOI: https://doi.org/10.1007/s13132-023-01731-w