Abstract
The lack of soil erosion data and other information about watersheds continues to limit soil erosion modeling. To overcome these limitations, many researchers have turned to machine learning models to analyze and model the complex water erosion processes and integrate them with empirical models. The Beni Haroun dam watershed faces soil erosion due to specific geo-environmental settings and land practices. It poses serious threats to agricultural and natural resource development. For these reasons, this study attempts to identify soil erosion susceptible zones using the Revised Universal Soil Loss Equation (RUSLE) using five key factors (rainfall erosivity, soil erodibility, topography, cover management and conservation practice factor) in GIS environment. Furthermore, we integrated the five RUSLE parameters and the model outputs into two machine learning (ML) algorithms, namely Random Forest (RF) and Random Tree (RT). The proposed models underwent training on 70% of the dataset and were subsequently validated on the remaining 30%. Our results indicated that the most vulnerable to severe soil erosion was concentrated in northwest regions, in contrast to the southeastern regions, which most occupy low erosion and moderate erosion. RUSLE and RT-based RUSLE models yielded nearly identical results in classifying erosion severity, estimating the annual average soil erosion at 17.5 and 17.69 (t ha–1y–1), respectively. In contrast, the Random Forest RF-based RUSLE model presented slightly divergent findings 23.89 (t ha–1y–1). Overall, these findings contribute to the identification of the area’s most vulnerable to soil erosion, providing valuable insights to inform land management and conservation strategies.










Similar content being viewed by others
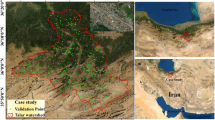
Data availability
The data that support the findings of this study are available on request.
References
Alewell C, Borrelli P, Meusburger K, Panagos P (2019) Using the USLE: chances, challenges and limitations of soil erosion modelling. Int soil Water Conserv Res 7:203–225
Arabameri A, Saha S, Roy J, Chen W, Blaschke T, Tien Bui D (2020) Landslide susceptibility evaluation and management using different machine learning methods in the Gallicash River Watershed, Iran. Remote Sens 12:475
Arabameri A, Seyed Danesh A, Santosh M, Cerda A, Chandra Pal S, Ghorbanzadeh O et al (2022) Flood susceptibility mapping using meta-heuristic algorithms. Geomatics Nat Hazards Risk 13:949–974
Avand M, Mohammadi M, Mirchooli F, Kavian A, Tiefenbacher JP (2023) A New Approach for Smart Soil Erosion modeling: integration of empirical and machine-learning models. Environ Model Assess 28:145–160
Bag R, Mondal I, Dehbozorgi M, Bank SP, Das DN, Bandyopadhyay J et al (2022) Modelling and mapping of soil erosion susceptibility using machine learning in a tropical hot sub-humid environment. J Clean Prod 364:132428
Behera M, Sena DR, Mandal U, Kashyap PS, Dash SS (2020) Integrated GIS-based RUSLE approach for quantification of potential soil erosion under future climate change scenarios. Environ Monit Assess 192:1–18
Borrelli P, Alewell C, Alvarez P, Anache JAA, Baartman J, Ballabio C et al (2021) Soil erosion modelling: a global review and statistical analysis. Sci Total Environ 780:146494
Bou-imajjane L, Belfoul MA, Elkadiri R, Stokes M (2020) Soil erosion assessment in a semi-arid environment: a case study from the Argana Corridor, Morocco. Environ Earth Sci 79:1–14
Breiman L (1996) Bagging predictors. Mach Learn 24:123–140
Chakrabortty R, Pal SC, Sahana M, Mondal A, Dou J, Pham BT et al (2020) Soil erosion potential hotspot zone identification using machine learning and statistical approaches in eastern India. Nat Hazards 104:1259–1294
Chipman JW, Lillesand TM, Schmaltz JE, Leale JE, Nordheim MJ (2004) Mapping lake water clarity with landsat images in Wisconsin, USA. Can J Remote Sens 30:1–7
Chuenchum P, Xu M, Tang W (2019) Estimation of soil erosion and sediment yield in the Lancang–Mekong river using the modified revised universal soil loss equation and GIS techniques. Water 12:135
Ebabu K, Tsunekawa A, Haregeweyn N, Tsubo M, Adgo E, Fenta AA et al (2022) Global analysis of cover management and support practice factors that control soil erosion and conservation. Int Soil Water Conserv Res 10:161–176
Ganasri BP, Ramesh H (2016) Assessment of soil erosion by RUSLE model using remote sensing and GIS-A case study of Nethravathi Basin. Geosci Front 7:953–961
Gaubi I, Chaabani A, Ben Mammou A, Hamza MH (2017) A GIS-based soil erosion prediction using the revised Universal Soil loss equation (RUSLE) (Lebna watershed, Cap Bon, Tunisia). Nat Hazards 86:219–239
Ghosal K, Bhattacharya SD (2020) A review of RUSLE Model. J Indian Soc Remote Sens 48:689–707
Haan CT, Barfield BJ, Hayes JC (1994) Design hydrology and sedimentology for small catchments. Elsevier
Islami FA, Tarigan SD, Wahjunie ED, Dasanto BD (2022) Accuracy assessment of land use change analysis using Google Earth in Sadar Watershed Mojokerto Regency. IOP Conference Series: Earth and Environmental Science 950: 012091
Jasinski MF (1990) Sensitivity of the normalized difference vegetation index to subpixel canopy cover, soil albedo, and pixel scale. Remote Sens Environ 32:169–187
Jayawardena S, Dharshika T, Herath R (2017) Observed climate trends, future climate change projections and possible impacts for Sri Lanka‘. Neela Haritha’the Clim Change Magazine Sri Lanka 2:144–151
Kateb Z, Bouchelkia H, Benmansour A, Belarbi F (2020) Sediment transport modeling by the SWAT model using two scenarios in the watershed of Beni Haroun dam in Algeria. Arab J Geosci 13:1–17
Kebede YS, Endalamaw NT, Sinshaw BG, Atinkut HB (2021) Modeling soil erosion using RUSLE and GIS at watershed level in the upper beles, Ethiopia. Environ Challenges 2:100009
Li P, Tariq A, Li Q, Ghaffar B, Farhan M, Jamil A et al (2023) Soil erosion assessment by RUSLE model using remote sensing and GIS in an arid zone. Int J Digit Earth 16:3105–3124
Luvai A, Obiero J, Omuto C (2022) Soil loss assessment using the revised universal soil loss equation (RUSLE) model. Applied and Environmental Soil Science 2022
Marouf N, Remini B (2019) Impact study of Beni-Haroun dam on the environmental and socio-economic elements in Kébir-Rhumel basin, Algeria. Journal of Water and Land Development
Mazour M, Roose E (2002) Influence de la couverture végétale sur le ruissellement et l’érosion des sols sur parcelles d’érosion dans les bassins versants du Nord-Ouest De l’Algérie. Bull Réseau Eros 21:320–330
McCool DK, Foster GR, Mutchler CK, Meyer LD (1989) Revised slope length factor for the Universal Soil loss equation. Trans ASAE 32:1571–1576
Mishra VN, Rai PK (2016) A remote sensing aided multi-layer perceptron-Markov chain analysis for land use and land cover change prediction in Patna district (Bihar), India. Arab J Geosci 9:249
Moisa MB, Negash DA, Merga BB, Gemeda DO (2021) Impact of land-use and land-cover change on soil erosion using the RUSLE model and the geographic information system: a case of Temeji watershed, Western Ethiopia. J Water Clim Change 12:3404–3420
Moore ID, Burch GJ (1986) Physical basis of the length-slope factor in the universal soil loss equation. Soil Sci Soc Am J 50:1294–1298
Nhu V-H, Shahabi H, Nohani E, Shirzadi A, Al-Ansari N, Bahrami S et al (2020) Daily water level prediction of Zrebar lake (Iran): a comparison between M5P, random forest, random tree and reduced error pruning trees algorithms. ISPRS Int J Geo-Information 9:479
Parveen R, Kumar U (2012) Integrated approach of universal soil loss equation (USLE) and geographical information system (GIS) for soil loss risk assessment in Upper South Koel Basin, Jharkhand
Pham BT, Luu C, Van Phong T, Nguyen HD, Van Le H, Tran TQ et al (2021) Flood risk assessment using hybrid artificial intelligence models integrated with multi-criteria decision analysis in Quang Nam Province, Vietnam. J Hydrol 592:125815
Pham QB, Afan HA, Mohammadi B, Ahmed AN, Linh NTT, Vo ND et al (2020) Hybrid model to improve the river streamflow forecasting utilizing multi-layer perceptron-based intelligent water drop optimization algorithm. Soft Comput 24:18039–18056
Phinzi K, Ngetar NS, Ebhuoma O (2021) Soil erosion risk assessment in the Umzintlava catchment (T32E), Eastern Cape, South Africa, using RUSLE and random forest algorithm. S Afr Geogr J 103:139–162
Rango A, Arnoldus HMJ (1987) Aménagement des bassins versants. Cahiers techniques de la FAO 36
Ranzi R, Le TH, Rulli MC (2012) A RUSLE approach to model suspended sediment load in the Lo river (Vietnam): effects of reservoirs and land use changes. J Hydrol 422:17–29
Razavi-Termeh SV, Sadeghi-Niaraki A, Choi S-M (2020) Gully erosion susceptibility mapping using artificial intelligence and statistical models. Geomatics Nat Hazards Risk 11:821–844
Renard KG (1997) Predicting soil erosion by water: a guide to conservation planning with the revised Universal Soil loss equation (RUSLE). United States Government Printing,
Renard KG, Foster GR, Weesies GA, Porter JP (1991) RUSLE: revised universal soil loss equation. J Soil Water Conserv 46:30–33
Sader SA, Winne JC (1992) RGB-NDVI colour composites for visualizing forest change dynamics. Int J Remote Sens 13:3055–3067
Sahli Y, Mokhtari E, Merzouk B, Laignel B, Vial C, Madani K (2019) Mapping surface water erosion potential in the Soummam watershed in Northeast Algeria with RUSLE model. J Mt Sci 16:1606–1615
Schonlau M, Zou RY (2020) The random forest algorithm for statistical learning. Stata J 20:3–29
Senanayake S, Pradhan B, Alamri A, Park H-J (2022) A new application of deep neural network (LSTM) and RUSLE models in soil erosion prediction. Sci Total Environ 845:157220
Shaikh S, Palanisamy M, Mohideen ARS (2020) Estimate the annual soil loss in Kummattipatti Nadi watershed using rusle model through geospatial technology. Geodesy Cartography 46:75–82
Song W, Wu C (2021) Introduction to advancements of GIS in the new IT era. Ann GIS 27:1–4
Sun Z, Sandoval L, Crystal-Ornelas R, Mousavi SM, Wang J, Lin C et al (2022) A review of earth artificial intelligence. Comput Geosci 159:105034
sxdsdsfd
Tahmasebi P, Kamrava S, Bai T, Sahimi M (2020) Machine learning in geo-and environmental sciences: from small to large scale. Adv Water Resour 142:103619
Toumi A, Remini B (2018) Perte De La capacité De Stockage D’eau Au barrage de Beni Haroun. Algérie SAGREN 2:80–97
Tripathy KP, Mishra AK (2023) Deep learning in Hydrology and Water resources disciplines: concepts, methods, applications, and research directions. J Hydrol : 130458
Tsegaye L, Bharti R (2021) Soil erosion and sediment yield assessment using RUSLE and GIS-based approach in Anjeb watershed, Northwest Ethiopia. SN Appl Sci 3:582
Van der Knijff JM, Jones RJA, Montanarella L (2000) Soil erosion risk: assessment in Europe. European Soil Bureau, European Commission Brussels
Wischmeier WH, Smith DD (1978) Predicting rainfall erosion losses: a guide to conservation planning. Department of Agriculture, Science and Education Administration
Wright MN, Wager S, Probst P (2020) Ranger: A fast implementation of random forests. R package version 0.12 1
Zeghmar A, Marouf N, Mokhtari E (2022) Assessment of soil erosion using the GIS-based erosion potential method in the Kebir Rhumel Watershed, Northeast Algeria. Journal of Water and Land Development
Zeghmar A, Marouf N, Mokhtari EH (2023) Assessment of soil erosion risk and simulation of the hydro-sedimentary balance using GIS, remote sensing and hydrological models. PhD Thesis, Larbi Ben M’hidi Oum El Bouaghi University (in French)
Zeyneb T, Nadir M, Boualem R (2022) Modeling of suspended sediment concentrations by artificial neural network and adaptive neuro fuzzy interference system method–study of five largest basins in Eastern Algeria. Water Pract Technol 17:1058–1081
Zhang XJ, Wang ZL (2017) Interrill soil erosion processes on steep slopes. J Hydrol 548:652–664
Funding
This research received no specific grant from any funding agency in the public, commercial, or not-for-profit sectors.
Author information
Authors and Affiliations
Contributions
Conceptualization, Zeghmar.Amer., Mokhtari Elhadj., Marouf Nadir.; Data acquisition, Zeghmar.Amer., Mokhtari Elhadj., Marouf Nadir.; Methodology, Zeghmar.Amer., Mokhtari Elhadj., Marouf Nadir.; Writing-original draft preparation, Zeghmar.Amer., Mokhtari Elhadj., Marouf Nadir.; Writing-review and editing, Zeghmar.Amer., Mokhtari Elhadj., Marouf Nadir.
Corresponding author
Ethics declarations
Competing interests
The authors declare no competing interests.
Conflict of interest
On behalf of all authors, the corresponding author declares no potential conflict of interest.
Additional information
Communicated by H. Babaie.
Publisher’s Note
Springer Nature remains neutral with regard to jurisdictional claims in published maps and institutional affiliations.
Rights and permissions
Springer Nature or its licensor (e.g. a society or other partner) holds exclusive rights to this article under a publishing agreement with the author(s) or other rightsholder(s); author self-archiving of the accepted manuscript version of this article is solely governed by the terms of such publishing agreement and applicable law.
About this article
Cite this article
Zeghmar, A., Mokhtari, E. & Marouf, N. A machine learning approach for RUSLE-based soil erosion modeling in Beni Haroun dam Watershed, Northeast Algeria. Earth Sci Inform (2024). https://doi.org/10.1007/s12145-024-01305-7
Received:
Accepted:
Published:
DOI: https://doi.org/10.1007/s12145-024-01305-7