Abstract
A greater understanding of ozone damage to the environment and health led to an increased demand for accurate predictions. This study provides two new accurate hybrid models of ozone prediction. The first one (CHIMERE-NARX) is based on a NARX model as a post-processing of the CHIMERE model. In the second (CHIMERE-NARX-DWT), a discrete wavelet transform (DWT) has been added. Our models were built and validated using ozone measurements from the Mediouna station in Casablanca, Morocco, from February 1st to March 27th, 2021. The results highlighted the CHIMERE model limitations, such as wind speed overestimation and insufficient emission data. The first hybrid successfully increased the correlation coefficient from 88 to 93% and reduced RMSE from 23.99 μg/m3 to −3.54 μg/m3, overcoming CHIMERE limitations to some extent, especially during nighttime. A second hybrid addressed the first hybrid limitation, such as using ozone as a single input. This hybrid successfully balanced the weight of NARX at night against the day, increasing the correlation coefficient to 98% and decreasing RMSE to −0.02 μg/m3. This study presents a new generation of post-processing based on deterministic model processes, with the possibility of training them with minimum input data, which can be applied to other models using various pollutants.













Similar content being viewed by others
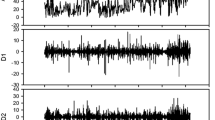
Data availability
The datasets used and/or analyzed during the current study are available from the corresponding author on reasonable request.
Abbreviations
- WHO:
-
World Health Organization
- NOx:
-
Nitrogen oxides
- ML:
-
Machine learning
- CNN:
-
Convolutional neural network
- DA:
-
Data assimilation
- LSTM:
-
Long-short-term memory
- CMAQ:
-
Community multiscale air quality
- ANN:
-
Artificial neural network
- DWT:
-
Discrete wavelet transform
- LM:
-
Levenberg-Marquardt
- RMSE:
-
Root means square error
- CAMS:
-
Copernicus atmosphere monitoring service
- EnKF:
-
Ensemble Kalman filter
- VOC:
-
Volatile organic compounds
- CTM:
-
Chemistry transport models
- NN:
-
Neural network
- MB:
-
Mean bias
- CT:
-
Chi-square test
- NARX:
-
Nonlinear auto-regressive network with exogenous inputs
- NWP:
-
Numerical weather prediction
- FFNNs:
-
Feed-forward neural networks
- WRF:
-
Weather research forecasting
- HPC:
-
High-performance calculator
- LMD:
-
Laboratory of meteorology dynamic
- AQF:
-
Air quality forecasting
- AI:
-
Artificial intelligence
References
Abbasimehr H, Shabani M, Yousefi M (2020) An optimized model using LSTM network for demand forecasting. Comput Ind Eng 143(March):106435. https://doi.org/10.1016/j.cie.2020.106435
Abdallah C, Afif C, El Masri N, Öztürk F, Keleş M, Sartelet K (2018) A first annual assessment of air quality modeling over Lebanon using WRF/Polyphemus. Atmos Pollut Res 9(4):643–654. https://doi.org/10.1016/j.apr.2018.01.003
Adnane et al. (2022) A comparative study between NARX and LSTM models in predicting ozone concentrations: case of Agadir City (Morocco). AI and IoT for Sustainable Development in Emerging Countries, Challenges and Opportunities. https://doi.org/10.1007/978-3-030-90618-4_9
Ajdour A, Leghrib R, Chaoufi J, Chirmata A, Menut L, Mailler S (2019) Towards air quality modeling in Agadir City (Morocco). Materials Today: Proceedings, xxxx. https://doi.org/10.1016/j.matpr.2019.07.438
Ajdour A, Leghrib R, Chaoufi J, Chirmata A (2022a) High spatial resolution effect on ozone pollution modelling: case study of Agadir city (Morocco). Materials Today: Proceedings 52:137–141. https://doi.org/10.1016/j.matpr.2021.11.278
Ajdour A, Leghrib R, Chaoufi J, Chirmata A (2022b) Numerical Method combinations assessment for transport-dominated problems in the CHIMERE model : a case study of Agadir (Morocco). 1(ICPAMS 2021. Lecture Notes in Networks and Systems, vol 476. Springer), 88–100. 10.1007/978-3-031-12416-7_9
Arnold D, Maurer C, Wotawa G, Draxler R, Saito K, Seibert P (2015) Influence of the meteorological input on the atmospheric transport modelling with FLEXPART of radionuclides from the Fukushima Daiichi nuclear accident. J Environ Radioact 139:212–225. https://doi.org/10.1016/j.jenvrad.2014.02.013
Baklanov A, Zhang Y (2020) Advances in air quality modeling and forecasting. Global Transitions 2:261–270. https://doi.org/10.1016/j.glt.2020.11.001
Belis CA, Pernigotti D, Pirovano G, Favez O, Jaffrezo JL, Kuenen J, Denier van Der Gon H, Reizer M, Riffault V, Alleman LY, Almeida M, Amato F, Angyal A, Argyropoulos G, Bande S, Beslic I, Besombes JL, Bove MC, Brotto P et al (2020) Evaluation of receptor and chemical transport models for PM10 source apportionment. Atmos Environ X 5(November 2019):100053. https://doi.org/10.1016/j.aeaoa.2019.100053
Bile A, Tari H, Grinde A, Frasca F, Siani AM, Fazio E (2022) Novel model based on artificial neural networks to predict short-term temperature evolution in museum environment. Sensors 22(2):1–16. https://doi.org/10.3390/s22020615
Blanco-Ward D, Rocha A, Viceto C, Ribeiro AC, Feliciano M, Paoletti E, Miranda AI (2021) Validation of meteorological and ground-level ozone WRF-CHIMERE simulations in a mountainous grapevine growing area for phytotoxic risk assessment. Atmos Environ 259(February):118507. https://doi.org/10.1016/j.atmosenv.2021.118507
Boussaada Z, Curea O, Remaci A, Camblong H, Bellaaj NM (2018) A nonlinear autoregressive exogenous (NARX) neural network model for the prediction of the daily direct solar radiation. Energies 11(3). https://doi.org/10.3390/en11030620
Brands S, Fernández-García G, Tesouro Montecelo M, Gallego Fernández N, Saunders Estévez AD, Carracedo García PE, Neto Venancio A, Melo da Costa P, Costa Tomé P, Otero C, Macho ML, Taboada J (2019) Sensitivity of CHIMERE to changes in model resolution and chemistry over the northwestern Iberian Peninsula. Atmos Chem Phys May:1–22. https://doi.org/10.5194/acp-2019-351
Bullock OR, Alapaty K, Herwehe JA, Kain JS (2015) A dynamically computed convective time scale for the Kain–Fritsch convective parameterization scheme. Mon Weather Rev 143(6):2105–2120. https://doi.org/10.1175/mwr-d-14-00251.1
C EW, Daubechies I (1993) Ten lectures on wavelets. Math Comput 61(204):941. https://doi.org/10.2307/2153268
Cabaneros SM, Calautit JK, Hughes B (2020) Short- and long-term forecasting of ambient air pollution levels using wavelet-based non-linear autoregressive artificial neural networks with exogenous inputs. Intl J Environm Impacts Manag Mitigation Recov 3(2):143–154. https://doi.org/10.2495/ei-v3-n2-143-154
Chai T, Draxler RR (2014) Root mean square error (RMSE) or mean absolute error (MAE)? -Arguments against avoiding RMSE in the literature. Geosci Model Dev 7(3):1247–1250. https://doi.org/10.5194/gmd-7-1247-2014
Chen F, Kusaka H, Tewari M, Bao JW, Hirakuchi H (2004) Utilizing the coupled WRF/LSM/urban modeling system with detailed urban classification to simulate the urban heat island phenomena over the greater Houston area. Fifth Conference on Urban Environment 9:11. https://ams.confex.com/ams/AFAPURBBIO/techprogram/paper_79765.htm
Cheng FY, Feng CY, Yang ZM, Hsu CH, Chan KW, Lee CY, Chang SC (2021) Evaluation of real-time PM2.5 forecasts with the WRF-CMAQ modeling system and weather-pattern-dependent bias-adjusted PM2.5 forecasts in Taiwan. Atmos Environ, 244(August 2020), 117909. https://doi.org/10.1016/j.atmosenv.2020.117909
Chi Y, Yang P, Ren S, Yang J (2020) Investigation of the community multiscale air quality (CMAQ) model representation of the climate penalty factor (CPF). Sci Total Environ 138954. https://doi.org/10.1016/j.atmosenv.2022.119157
Chianese E, Galletti A, Giunta G, Landi TC, Marcellino L, Montella R, Riccio A (2018) Spatiotemporally resolved ambient particulate matter concentration by fusing observational data and ensemble chemical transport model simulations. Ecol Model 385(August):173–181. https://doi.org/10.1016/j.ecolmodel.2018.07.019
Chirmata A, Leghrib R, Ichou IA (2017) Implementation of the air quality monitoring network at Agadir City in Morocco. J Environ Prot 08(04):540–567. https://doi.org/10.4236/jep.2017.84037
Dai J, Liu Y, Wang P, Fu X, Xia M, Wang T (2020) The impact of sea-salt chloride on ozone through heterogeneous reaction with N2O5 in a coastal region of south China. Atmos Environ 236(May):117604. https://doi.org/10.1016/j.atmosenv.2020.117604
Daubechles I (1989) Orthonormal bases of compactly supported wavelets. Fundamental Papers in Wavelet Theory, XLI(0), 564–651. https://doi.org/10.1515/9781400827268.564
De Meij A, Gzella A, Cuvelier C, Thunis P, Bessagnet B, Vinuesa JF, Menut L, Kelder HM (2009) The impact of MM5 and WRF meteorology over complex terrain on CHIMERE model calculations. Atmos Chem Phys 9(17):6611–6632. https://doi.org/10.5194/acp-9-6611-2009
Do TNN, Ngo XT, Pham VH, Vuong NL, Le HA, Pham CT, Bui QH, Nguyen TNT (2021) Application of WRF-Chem to simulate air quality over Northern Vietnam. Environ Sci Pollut Res 28(10):12067–12081. https://doi.org/10.1007/s11356-020-08913-y
Dudhia J (1989) Numerical study of convection observed during the winter monsoon experiment using a mesoscale two-dimensional model Dudhia_1989_JAtmosSci.pdf. https://doi.org/10.1175/1520-0469(1989)046%3C3077:NSOCOD%3E2.0.CO;2
Dumka UC, Kaskaoutis DG, Verma S, Ningombam SS, Kumar S, Ghosh S (2021) Silver linings in the dark clouds of COVID-19: improvement of air quality over India and Delhi metropolitan area from measurements and WRF-CHIMERE model simulations. Atmos Pollut Res 12(2):225–242. https://doi.org/10.1016/j.apr.2020.11.005
Eslami E, Choi Y, Lops Y, Sayeed A, Khan Salman A (2020) Using wavelet transform and dynamic time warping to identify the limitations of the CNN model as an air quality forecasting system. Geosci Model Dev 13(12):6237–6251. https://doi.org/10.5194/gmd-13-6237-2020
Fairall CW, Bradley EF, Hare JE, Grachev AA, Edson JB (2003) Bulk parameterization of air-sea fluxes: updates and verification for the COARE algorithm. J Clim 16(4):571–591. https://doi.org/10.1175/1520-0442(2003)016<0571:BPOASF>2.0.CO;2
Feng R, Zheng HJ, Zhang AR, Huang C, Gao H, Ma YC (2019) Unveiling tropospheric ozone by the traditional atmospheric model and machine learning, and their comparison:a case study in hangzhou, China. Environ Pollut 252:366–378. https://doi.org/10.1016/j.envpol.2019.05.101
Ferreyra MFG, Curci G, Lanfri M (2016) First implementation of the WRF-CHIMERE-EDGAR modeling system over Argentina. IEEE J Select Top Appl Earth Observ Remote Sens 9(12):5304–5314. https://doi.org/10.1109/JSTARS.2016.2588502
Gama C, Ribeiro I, Lange AC, Vogel A, Ascenso A, Seixas V, Elbern H, Borrego C, Friese E, Monteiro A (2019) Performance assessment of CHIMERE and EURAD-IM’ dust modules. Atmos Pollut Res 10(4):1336–1346. https://doi.org/10.1016/j.apr.2019.03.005
Gaubert B, Coman A, Foret G, Meleux F, Ung A, Rouil L, Ionescu A, Candau Y, Beekmann M (2014) Regional scale ozone data assimilation using an ensemble Kalman filter and the CHIMERE chemical transport model. Geosci Model Dev 7(1):283–302. https://doi.org/10.5194/gmd-7-283-2014
Gavete L, Vivanco MG, Molina P, Gavete ML, Urena F, Benito JJ (2012) Implementation in CHIMERE of a conservative solver for the advection equation-cmmse10. J Comput Appl Math 236(12):3026–3033. https://doi.org/10.1016/j.cam.2011.04.003
Ge S, Zhang J, Wang S, Xu Q, Ho T (2019) New insight of ozone pollution impact from flare emissions of chemical plant start-up operations. Environ Pollut 245:873–882. https://doi.org/10.1016/j.envpol.2018.11.048
Guenther AB, Jiang X, Heald CL, Sakulyanontvittaya T, Duhl T, Emmons LK, Wang X (2012) The model of emissions of gases and aerosols from nature version 2.1 (MEGAN2.1): an extended and updated framework for modeling biogenic emissions. Geosci Model Dev 5(6):1471–1492. https://doi.org/10.5194/gmd-5-1471-2012
Gulia S, Shiva Nagendra SM, Khare M, Khanna I (2015) Urban air quality management-a review. Atmos Pollut Res 6(2):286–304. https://doi.org/10.5094/APR.2015.033
Hagan MT, Menhaj MB (1994) Training feedforward networks with the Marquardt algorithm. IEEE Trans Neural Netw 5(6):989–993. https://doi.org/10.1109/72.329697
Han X, Cao T (2022) Urbanization level , industrial structure adjustment and spatial effect of urban haze pollution : evidence from China ’ s Yangtze River Delta urban agglomeration. Atmos Pollut Res 13(6):101427. https://doi.org/10.1016/j.apr.2022.101427
Hong SY, Dudhia J, Chen SH (2004) A revised approach to ice microphysical processes for the bulk parameterization of clouds and precipitation. Mon Weather Rev 132(1):103–120. https://doi.org/10.1175/1520-0493(2004)132<0103:ARATIM>2.0.CO;2
HPC (2014) Recensement General de la population et de l’habitat -Volume 2. Population (English Edition), 2. https://www.hcp.ma/reg-casablanca/attachment/673642/
Jenkins G, Gueye M (2022) Annual and early summer variability in WRF-CHEM simulated West African PM10 during 1960–2016. Atmos Environ 273(December 2021):118957. https://doi.org/10.1016/j.atmosenv.2022.118957
Jiménez PA, Dudhia J (2012) Improving the representation of resolved and unresolved topographic effects on surface wind in the wrf model. J Appl Meteorol Climatol 51(2):300–316. https://doi.org/10.1175/JAMC-D-11-084.1
Khodayari A, Vitt F, Phoenix D, Wuebbles DJ (2018) The impact of NOx emissions from lightning on the production of aviation-induced ozone. Atmos Environ 187(May):410–416. https://doi.org/10.1016/j.atmosenv.2018.05.057
Khomsi K, Najmi H, Amghar H, Chelhaoui Y, Souhaili Z (2021) COVID-19 national lockdown in Morocco: impacts on air quality and public health. One Health 11:100200. https://doi.org/10.1016/j.onehlt.2020.100200
Khomsi K, Chelhaoui Y, Alilou S, Souri R, Najmi H, Souhaili Z (2022) Concurrent heat waves and extreme ozone (O3) episodes: combined atmospheric patterns and impact on human health. Int J Environ Res Public Health 19(5). https://doi.org/10.3390/ijerph19052770
Li J, Yu S, Chen X, Zhang Y, Li M, Li Z, Song Z, Liu W, Li P, Xie M, Xing J (2022) Evaluation of the WRF-CMAQ model performances on air quality in china with the impacts of the observation nudging on meteorology. Aerosol Air Qual Res 22(4):220023. https://doi.org/10.4209/aaqr.220023
Lightstone SD, Moshary F, Gross B (2017) Comparing CMAQ forecasts with a neural network forecast model for PM2.5 in New York. Atmosphere 8(9). https://doi.org/10.3390/atmos8090161
Lu H, Xie M, Liu X, Liu B, Jiang M, Gao Y, Zhao X (2021) Adjusting prediction of ozone concentration based on CMAQ model and machine learning methods in Sichuan-Chongqing region, China. Atmos Pollut Res 12(6):101066. https://doi.org/10.1016/j.apr.2021.101066
Luecken DJ, Yarwood G, Hutzell WT (2019) Multipollutant modeling of ozone, reactive nitrogen and HAPs across the continental US with CMAQ-CB6. Atmos Environ 201(December 2018):62–72. https://doi.org/10.1016/j.atmosenv.2018.11.060
Ma C, Wang T, Jiang Z, Wu H, Zhao M, Zhuang B, Li S, Xie M, Li M, Liu J, Wu R (2020) Importance of bias correction in data assimilation of multiple observations over eastern China using WRF-Chem/DART. J Geophys Res-Atmos 125(1):1–22. https://doi.org/10.1029/2019JD031465
Mailler S, Menut L, Khvorostyanov D, Valari M, Couvidat F, Siour G, Turquety S, Briant R, Tuccella P, Bessagnet B, Colette A, Létinois L, Markakis K, Meleux F (2017) CHIMERE-2017: from urban to hemispheric chemistry-transport modeling. Geosci Model Dev 10(6):2397–2423. https://doi.org/10.5194/gmd-10-2397-2017
Mallat SG (1989) A theory for multiresolution signal decomposition: the wavelet representation. Fund Papers Wavelet Theory II(7):494–513. https://doi.org/10.1515/9781400827268.494
Mazzeo A, Burrow M, Quinn A, Marais EA, Singh A, Ng D, Gatari MJ, Pope FD (2022a) Evaluation of the WRF and CHIMERE models for the simulation of PM 2 . 5 in large East African urban conurbations. 10677–10701
Mazzeo A, Huneeus N, Ordoñez C, Orfanoz-Cheuquelaf A, Menut L, Mailler S, Valari M, Denier van der Gon H, Gallardo L, Muñoz R, Donoso R, Galleguillos M, Osses M, Tolvett S (2018) Impact of residential combustion and transport emissions on air pollution in Santiago during winter. Atmos Environ 190(December 2017):195–208. https://doi.org/10.1016/j.atmosenv.2018.06.043
Mazzeo A, Zhong J, Hood C, Smith S, Stocker J, Cai X, Bloss WJ (2022b) Modelling the impact of national vs. local emission reduction on PM2.5 in the West Midlands, UK using WRF-CMAQ. Atmosphere 13(3):1–15. https://doi.org/10.3390/atmos13030377
McCabe LC (2019) Atmospheric pollution. In Industrial and Engineering Chemistry (3rd ed., Vol. 43, Issue 9). Elsevier Inc. https://doi.org/10.1021/i650501a715
Menut L, Bessagnet B, Khvorostyanov D, Beekmann M, Blond N, Colette A, Coll I, Curci G, Foret G, Hodzic A, Mailler S, Meleux F, Monge J-L, Pison I, Siour G, Turquety S, Valari M, Vautard R, Vivanco MG (2013) CHIMERE 2013: a model for regional atmospheric composition modelling. Geosci Model Dev 6(4):981–1028. https://doi.org/10.5194/gmd-6-981-2013
Menut L, Mailler S, Siour G, Bessagnet B, Turquety S, Rea G, Briant R, Mallet M, Sciare J, Formenti P, Meleux F (2015) Ozone and aerosol tropospheric concentrations variability analyzed using the ADRIMED measurements and the WRF and CHIMERE models. Atmos Chem Phys 15(11):6159–6182. https://doi.org/10.5194/acp-15-6159-2015
Mlawer EJ, Taubman SJ, Brown PD, Iacono MJ, Clough SA (1997) Radiative transfer for inhomogeneous atmospheres: RRTM, a validated correlated-k model for the longwave. J Geophys Res-Atmos 102(D14):16663–16682. https://doi.org/10.1029/97jd00237
Mok KM, Miranda AI, Yuen KV, Hoi KI, Monteiro A, Ribeiro I (2017) Selection of bias correction models for improving the daily PM10 forecasts of WRF-EURAD in Porto, Portugal. Atmos Pollut Res 8(4):628–639. https://doi.org/10.1016/j.apr.2016.12.010
Onwukwe C, Jackson PL (2021) Gridded bias correction of modeled PM2.5 for exposure assessment, and estimation of background concentrations over a coastal valley region of northwestern British Columbia, Canada. J Air Waste Manag Assoc 71(2):156–169. https://doi.org/10.1080/10962247.2020.1844342
Pak U, Kim C, Ryu U, Sok K, Pak S (2018) A hybrid model based on convolutional neural networks and long short-term memory for ozone concentration prediction. Air Qual Atmos Health 11(8):883–895. https://doi.org/10.1007/s11869-018-0585-1
Pal, R. (2017). Validation methodologies. Predictive Modeling of Drug Sensitivity, 83–107. https://doi.org/10.1016/b978-0-12-805274-7.00004-x
Pan S, Choi Y, Jeon W, Roy A, Westenbarger DA, Kim HC (2017) Impact of high-resolution sea surface temperature, emission spikes and wind on simulated surface ozone in Houston, Texas during a high ozone episode. Atmos Environ 152:362–376. https://doi.org/10.1016/j.atmosenv.2016.12.030
Park S-Y, Dash UK, Yu J, Yumimoto K, Uno I, Song CH (2022) Implementation of an ensemble Kalman filter in the community multiscale air quality model (CMAQ Model v5.1) for data assimilation of ground-level PM2.5. 2, 2773–2790. https://doi.org/10.5194/gmd-2021-302
Permadi DA, Oanh NTK, Vautard R (2018) Integrated emission inventory and modeling to assess distribution of particulate matter mass and black carbon composition in Southeast Asia. Atmos Chem Phys 18(4):2725–2747. https://doi.org/10.5194/acp-18-2725-2018
Pernigotti D, Georgieva E, Thunis P, Bessagnet B (2012) Impact of meteorology on air quality modeling over the Po valley in northern Italy. Atmos Environ 51:303–310. https://doi.org/10.1016/j.atmosenv.2011.12.059
Petkova EP, Jack DW, Volavka-Close NH, Kinney PL (2013) Particulate matter pollution in African cities. Air Qual Atmos Health 6(3):603–614. https://doi.org/10.1007/s11869-013-0199-6
Pino-Cortés E, Carrasco S, Acosta J, de Almeida Albuquerque TT, Pedruzzi R, Díaz-Robles LA (2022) An evaluation of the photochemical air quality modeling using CMAQ in the industrial area of Quintero-Puchuncavi-Concon, Chile. Atmos Pollut Res 13(3). https://doi.org/10.1016/j.apr.2022.101336
Puigferrat A, de Pouplana I, Amato F, Oñate E (2021) Numerical prediction of the distribution of black carbon in a street canyon using a semi-Lagrangian finite element formulation. Build Environ 199(January):107910. https://doi.org/10.1016/j.buildenv.2021.107910
Ranganathan A (2004) The Levenberg-Marquardt algorithm 3 LM as a blend of gradient descent and Gauss-Newton itera. Internet Httpexcelsior Cs Ucsb Educoursescs290ipdfL MA Pdf, 142(June), 1–5. http://twiki.cis.rit.edu/twiki/pub/Main/AdvancedDipTeamB/the-levenberg-marquardt-algorithm.pdf
Rangel-Alvarado R, Pal D, Ariya P (2022) PM2.5 decadal data in cold vs. mild climate airports: COVID-19 era and a call for sustainable air quality policy. Environ Sci Pollut Res:58133–58148. https://doi.org/10.1007/s11356-022-19708-8
Ratola N, Jiménez-Guerrero P (2017) Modelling benzo[a]pyrene in air and vegetation for different land uses and assessment of increased health risk in the Iberian Peninsula. Environ Sci Pollut Res 24(13):11901–11910. https://doi.org/10.1007/s11356-015-5394-6
Sayeed A, Choi Y, Eslami E, Jung J, Lops Y, Salman AK, Lee JB, Park HJ, Choi MH (2021) A novel CMAQ-CNN hybrid model to forecast hourly surface-ozone concentrations 14 days in advance. Sci Rep 11(1):1–8. https://doi.org/10.1038/s41598-021-90446-6
Sayeed A, Choi Y, Eslami E, Lops Y (2019) Using a deep convolutional neural network to predict 2017 ozone concentrations, 24 hours in advance. Neural Netw. https://doi.org/10.1016/j.neunet.2019.09.033
Sayeed A, Eslami E, Lops Y, Choi Y (2022) CMAQ-CNN: a new-generation of post-processing techniques for chemical transport models using deep neural networks. Atmos Environ 273(December 2021):118961. https://doi.org/10.1016/j.atmosenv.2022.118961
Schober P, Schwarte LA (2018) Correlation coefficients: appropriate use and interpretation. Anesth Analg 126(5):1763–1768. https://doi.org/10.1213/ANE.0000000000002864
Schuch D, de Freitas ED, Espinosa SI, Martins LD, Carvalho VSB, Ramin BF, Silva JS, Martins JA, de Fatima Andrade M (2019) A two decades study on ozone variability and trend over the main urban areas of the São Paulo state, Brazil. Environ Sci Pollut Res 26(31):31699–31716. https://doi.org/10.1007/s11356-019-06200-z
Sherstinsky A (2020) Fundamentals of recurrent neural network (RNN) and long short-term memory (LSTM) network. Physica D: Nonlinear Phenomena 404:132306. https://doi.org/10.1016/j.physd.2019.132306
Siddiqua A, Hahladakis JN, Ahmed W, Attiya KA, Al. (2022) An overview of the environmental pollution and health effects associated with waste landfilling and open dumping. Environ Sci Pollut Res 58514–58536. https://doi.org/10.1007/s11356-022-21578-z
Srivastava N, Blond N (2022) Impact of meteorological parameterization schemes on CTM model simulations. Atmos Environ 268(November 2020):118832. https://doi.org/10.1016/j.atmosenv.2021.118832
Stoffel M, Bamer F, Markert B (2018) Artificial neural networks and intelligent finite elements in non-linear structural mechanics. Thin-Walled Struct 131(June):102–106. https://doi.org/10.1016/j.tws.2018.06.035
Tsokov S, Lazarova M, Aleksieva-petrova A (2022) A hybrid spatiotemporal deep model based on CNN and LSTM for air pollution prediction
Tuccella P, Menut L, Briant R, Deroubaix A, Khvorostyanov D, Mailler S, Siour G, Turquety S (2019) Implementation of aerosol-cloud interaction within WRF-CHIMERE online coupled model: evaluation and investigation of the indirect radiative effect from anthropogenic emission reduction on the Benelux Union. Atmosphere 10(1). https://doi.org/10.3390/atmos10010020
Ulfah S, Awalludin SA, Wahidin W (2018) Advection-diffusion model for the simulation of air pollution distribution from a point source emission. J Phys Conf Ser 948(1). https://doi.org/10.1088/1742-6596/948/1/012067
Wang, H. W., Li, X. B., Wang, D., Zhao, J., He, H. di, & Peng, Z. R. (2020). Regional prediction of ground-level ozone using a hybrid sequence-to-sequence deep learning approach. J Clean Prod, 253, 119841. https://doi.org/10.1016/j.jclepro.2019.119841
Wang J, Li J, Wang X, Wang J, Huang M (2021) Air quality prediction using CT-LSTM. Neural Comput & Applic 33(10):4779–4792. https://doi.org/10.1007/s00521-020-05535-w
WHO. (2020). Air pollution. https://www.who.int/health-topics/air-pollution#tab=tab_1
Yang J, Ma J (2019) Feed-forward neural network training using sparse representation. Expert Syst Appl 116:255–264. https://doi.org/10.1016/j.eswa.2018.08.038
Zhan Y, Luo Y, Deng X, Grieneisen ML, Zhang M, Di B (2018) Spatiotemporal prediction of daily ambient ozone levels across China using random forest for human exposure assessment. Environ Pollut 233:464–473. https://doi.org/10.1016/j.envpol.2017.10.029
Zhou G, Xu J, Xie Y, Chang L, Gao W, Gu Y, Zhou J (2017) Numerical air quality forecasting over eastern China: an operational application of WRF-Chem. Atmos Environ 153:94–108. https://doi.org/10.1016/j.atmosenv.2017.01.020
Acknowledgments
The Faculty of Science University IBN ZOHR supported this research in all its stages. We would like to thank warmly Ministry of Interior, especially the Department of Energy and Environment of the Souss Massa region, for their collaboration. We are grateful to the Dynamic Meteorology Laboratory (LMD) for its valuable assistance. The calculations for this simulation were done using the national HPC managed by the National Center of Scientific and Technological Research (CNRST) in Morocco. The authors are grateful to the staff of “HPC” in particular Ms. Bouchra RAHIM, Scientific Computing team leader, for her availability and assistance.
Author information
Authors and Affiliations
Contributions
Amine Ajdour, Anas Adnane, Brahim Ydir, Dris Ben hmamou: conceptualization, methodology, validation, writing, original draft, writing—review and editing. Kenza KHOMSI, Hassan AMGHAR, Youssef CHELHAOUI: data acquisition and validation, review and editing. Jamal CHAOUFI, Radouane LEGHRIB: direction, methodology, investigation—review and editing.
Corresponding author
Ethics declarations
Conflict of interest
The authors declare no competing interests.
Additional information
Responsible Editor: Marcus Schulz
Publisher’s note
Springer Nature remains neutral with regard to jurisdictional claims in published maps and institutional affiliations.
Rights and permissions
Springer Nature or its licensor holds exclusive rights to this article under a publishing agreement with the author(s) or other rightsholder(s); author self-archiving of the accepted manuscript version of this article is solely governed by the terms of such publishing agreement and applicable law.
About this article
Cite this article
Ajdour, A., Adnane, A., Ydir, B. et al. A new hybrid models based on the neural network and discrete wavelet transform to identify the CHIMERE model limitation. Environ Sci Pollut Res 30, 13141–13161 (2023). https://doi.org/10.1007/s11356-022-23084-8
Received:
Accepted:
Published:
Issue Date:
DOI: https://doi.org/10.1007/s11356-022-23084-8