Abstract
Multi band, high spatial resolution and information redundancy are the most significant characteristics of hyperspectral image. These remarkable characteristics are mainly caused by the high-dimensional image data. In view of these characteristics, feature extraction of hyperspectral image has naturally become one of the research hotspots in this field. However, it is impossible to fully describe the intrinsic geometric structure of hyperspectral image only by using spectral information. To improve the subsequent forecasting accuracy, spatial information should be mined to further describe the geometric structure of hyperspectral image. Therefore, an effective feature extraction method (SSF_HM) was proposed via using harmonic mean and spectral-spatial filter. This investigation divides the SSF_HM into three steps. First, the pi principal components were extracted by using principal components analysis (PCA) skill and subsequent the pi spatial filtering features were obtained via using area median filter (AMF) method. Then, the original spectral features and extracted spatial filtering features combine to form the fusion feature matrix, and then the scatter matrix \(S_{b}^{HM}\) (based on harmonic mean (HM) spectral-spatial filter inter-class) and scatter matrix \(S_{w}^{HM}\) (based on harmonic mean (HM) spectral-spatial filter intra-class) can be established in the fusion feature space, respectively. Finally, combining the Fisher discriminant analysis model and regularization technique, a new feature extraction method SSF_HM is developed. The proposed SSF_HM method combines spectral information and spatial information. At the same time, the range of feature extraction is expanded from spectral space to spectral-spatial fusion space. The experimental results on three real-world hyperspectral image data sets show the better performance of SSF_HM in comparison with other feature extraction methods in small sample size situation by using maximum likelihood classifier (MLC).












Similar content being viewed by others
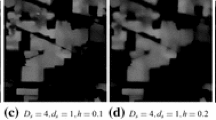
References
Ait-Sahalia Y, Xiu D (2019) Principal component analysis of high-frequency data[J]. J Am Stat Assoc 114(525):287–303
Bi Z, Yu L, Gao H, Zhou P, Yao H (2020) Improved VGG model-based efficient traffic sign recognition for safe driving in 5G scenarios[J]. Int J Mach Learn Cybern. https://doi.org/10.1007/s13042-020-01185-5https://doi.org/10.1007/s13042-020-01185-5
Cai D, He X, Han J (2007) Semi-supervised discriminant analysis[C]. In: IEEE 11th International conference on computer vision, 2007. ICCV 2007. IEEE, 1–7
Chang CI, Ren H (2000) An experiment-based quantitative and comparative analysis of target detection and image classification algorithms for hyperspectral imagery[J]. IEEE Trans Geosci Remote Sens 38(2):1044–1063
Chang CI, Safavi H (2011) Progressive dimensionality reduction by transform for hyperspectral imagery[J]. Pattern Recogn 44(10-11):2760–2773
Chen T, Xu J, Ying H, Chen X, Feng R, Fang X, Gao H, Wu J (2019) Prediction of extubation failure for intensive care unit patients using light gradient boosting machine[J]. IEEE Access 7(1):150960-150968. https://doi.org/10.1109/ACCESS.2019.2946980
Chen J, Ying H, Liu X, Gu J, Feng R, Chen T, Gao H, Wu JA (2020) Transfer learning based super-resolution microscopy for biopsy slice images: the joint methods perspective[J]. IEEE/ACM Trans Comput Biol Bioinf (TCBB). https://doi.org/10.1109/TCBB.2020.2991173
Chen S, Zhang D (2011) Semisupervised dimensionality reduction with pairwise constraints for hyperspectral image classification[J]. IEEE Geosci Remote Sens Lett 8(2):369–373
David L (2002) Hyperspectral image data analysis as a high dimensional signal processing problem[J]. IEEE Signal Processing Mag 19(1):17–28
Elton EJ, Gruber MJ, Brown SJ et al (2011) Modern portfolio theory and investment analysis[M]. Wiley, Hoboken
Fabija n ska A, Sankowski D (2011) Noise adaptive switching median-based filter for impulse noise removal from extremely corrupted images[J]. IET Image Processing 5(5):472–480
Fauvel M, Chanussot J, Benediktsson JA (2009) Kernel principal component analysis for the classification of hyperspectral remote sensing data over urban areas[J]. EURASIP J Adv Signal Process 2009(1):783194
Gao J, Xu L (2015) An efficient method to solve the classification problem for remote sensing image[J]. AEU-Int J Electron Commun 69(1):198–205
Gao J, Xu L (2016) A novel spatial analysis method for remote sensing image classification[J]. Neural Process Lett 43(3):805–821
Gao J, Xu L, Huang F (2016) A spectral-textural kernel-based classification method of remotely sensed images[J]. Neural Comput Applic 27(2):431–446
Gao J, Xu L, Shen J et al (2015) A novel information transferring approach for the classification of remote sensing images[J]. EURASIP J Adv Signal Process 2015(1):38
Gao J, Xu L, Shi A et al (2014) A kernel-based block matrix decomposition approach for the classification of remotely sensed images[J]. Appl Math Comput 228:531–545
Gao W, Zhu Y, Zhang W, Zhang K, Gao H (2019) A hierarchical recurrent approach to predict scene graphs from a visual-attention-oriented perspective[J]. Comput Intell 35:496–516. https://doi.org/10.1111/coin.12202https://doi.org/10.1111/coin.12202
Ghamisi P, Benediktsson JA, Sveinsson JR (2014) Automatic spectral-spatial classification framework based on attribute profiles and supervised feature extraction[J]. IEEE Trans Geosci Remote Sens 52(9):5771–5782
Guo AJX, Zhu F (2019) Spectral-spatial feature extraction and classification by ANN supervised with center loss in hyperspectral imagery[J]. IEEE Trans Geosci Remote Sens 57(3):1755–1767
Guo-Feng F, Li-Ling P, Wei-Chiang H, Sun F Electric Load Forecasting by the SVR Model with Differential Empirical Mode Decomposition and Auto Regression[J]. Neurocomputing, https://doi.org/10.1016/j.neucom.2015.08.051
He X, Cai D, Yan S et al (2005) Neighborhood preserving embedding[C]. In: Tenth IEEE international conference on computer vision, 2005. ICCV 2005. IEEE, 2, 1208–1213
He X, Niyogi P (2004) Locality preserving projections[C]. Advan Neural Inform Process Syst:153–160
Hong W-C (2011) Electric load forecasting by seasonal recurrent SVR (support vector regression) with chaotic artificial bee colony algorithm[J]. Energy 36:5568–5578
Hosseini SA, Ghassemian H (2016) Hyperspectral data feature extraction using rational function curve fitting[J]. Int J Pattern Recogn Artif Intell 30 (01):1650001. https://doi.org/10.1142/S0218001416500014
Hosseini SA, Ghassemian H (2016) Rational function approximation for feature reduction in hyperspectral data[J]. Remote Sensing Letters 7(2):101–110
Ibrahim H, Kong NSP, Ng TF (2008) Simple adaptive median filter for the removal of impulse noise from highly corrupted images[J]. IEEE Trans Consum Electron 54(4):1920–1927
Imani M, Ghassemian H (2015) Feature space discriminant analysis for hyperspectral data feature reduction[J]. ISPRS J Photogramm Remote Sens 102:1–13
Jiang J, Ma J, Chen C et al (2018) SuperPCA: a superpixelwise PCA approach for unsupervised feature extraction of hyperspectral imagery[J]. IEEE Trans Geoscience Remote Sens (99):1–13
Journaux L, Tizon X, Foucherot I et al (2006) Dimensionality reduction techniques: an operational comparison on multispectral satellite images using unsupervised clustering[C]. In: Proceedings of the 7th Nordic, Signal Processing Symposium, 2006. NORSIG 2006. IEEE, 242–245
Kuo BC, Landgrebe DA (2004) Nonparametric weighted feature extraction for classification[J]. IEEE Trans Geosci Remote Sens 42(5):1096–1105
Landgrebe DA (2005) Signal theory methods in multispectral remote sensing [M]. Wiley, Hoboken
Li L, Ge H, Gao J (2017) A spectral-spatial kernel-based method for hyperspectral imagery classification[J]. Adv Space Res 59(4):954–967
Li L, Ge H, Gao J et al (2019) Hyperspectral image feature extraction using maclaurin series function curve Fitting[J]. Neural Process Lett 49 (1):357–374
Li L, Ge H, Gao J et al (2019) A novel geometric mean feature space discriminant analysis method for hyperspectral image feature extraction[J]. Neural Process Lett. https://doi.org/10.1007/s11063-019-10101-0https://doi.org/10.1007/s11063-019-10101-0
Li M-W, Geng J, Hong W-C, Zhang L-D (2019) Periodogram estimation based on LSSVR-CCPSO compensation for forecasting ship motion[J]. Nonlinear Dyn 97:2579–2594
Liao W, Pizurica A, Scheunders P et al (2013) Semisupervised local discriminant analysis for feature extraction in hyperspectral images[J]. IEEE Trans Geosci Remote Sens 51(1):184–198
Ou D, Tan K, Du Q et al (2019) A novel tri-training technique for the semi-supervised classification of hyperspectral images based on regularized local discriminant embedding feature extraction[J]. Remote Sens 11(6):654
Park CH, Park H (2008) A comparison of generalized linear discriminant analysis algorithms[J]. Pattern Recogn 41(3):1083–1097
Plaza A, Martinez P, Plaza J et al (2005) Dimensionality reduction and classification of hyperspectral image data using sequences of extended morphological transformations[J]. IEEE Trans Geoscience Remote Sens 43(3):466–479
Ren J, Zabalza J, Marshall S et al (2014) Effective feature extraction and data reduction in remote sensing using hyperspectral imaging[J]. IEEE Signal Proc Mag 31(4):149–154
Sugiyama M, Id e T, Nakajima S et al (2010) Semi-supervised local fisher discriminant analysis for dimensionality reduction[J]. Mach Learn 78(1–2):35
Tarabalka Y, Benediktsson JA, Chanussot J (2009) Spectral-spatial classification of hyperspectral imagery based on partitional clustering techniques[J]. IEEE Trans Geosci Remote Sens 47(8):2973–2987
Wang M, Yu J, Niu L et al (2017) Unsupervised feature extraction for hyperspectral images using combined low rank representation and locally linear embedding[C]. In: 2017 IEEE International Conference on Acoustics, Speech and Signal Processing (ICASSP), IEEE, 1428–1431
Wei-Chiang H, Dong Y, Zhang W, Li-Yueh C, Panigrahi BK (2013) Cyclic electric load forecasting by seasonal SVR with chaotic genetic algorithm[J]. Electr Power Energy Syst 44:604–614
Xia J, Du P, He X et al (2013) Hyperspectral remote sensing image classification based on rotation forest[J]. IEEE Geosci Remote Sens Lett 11(1):239–243
Xiao J, Xu H, Gao H, Bian M, Li Y (2020) A weakly supervised semantic segmentation network by aggregating seed cues[J]. ACM Trans Multimed Comput Commun Appl (TOMM). https://doi.org/10.1145/3419842
Zabalza J, Ren J, Yang M et al (2014) Novel folded-PCA for improved feature extraction and data reduction with hyperspectral imaging and SAR in remote sensing[J]. ISPRS J Photogramm Remote Sens 93:112–122
Zhang Z, Ding S, Jia W (2019) A hybrid optimization algorithm based on cuckoo search and differential evolution for solving constrained engineering problems[J]. Eng Appl Artif Intell 85:254–268
Zhang Z, Ding S, Sun Y (2020) A support vector regression model hybridized with chaotic krill herd algorithm and empirical mode decomposition for regression task[J]. Neurocomputing 410:185–201
Zhang Z, Wei-Chiang H (2019) Electric load forecasting by complete ensemble empirical mode decomposition adaptive noise and support vector regression with quantum-based dragonfly algorithm[J]. Nonlinear Dyn 98:1107–1136
Zhang T, Yang J, Zhao D et al (2007) Linear local tangent space alignment and application to face recognition[J]. Neurocomputing 70(7–9):1547–1553
Zhang L, Zhang L, Du B et al (2019) Hyperspectral image unsupervised classification by robust manifold matrix factorization[J]. Inf Sci 485:154–169
Zhou Y, Peng J, Chen CLP (2015) Dimension reduction using spatial and spectral regularized local discriminant embedding for hyperspectral image classification[J]. IEEE Trans Geosci Remote Sens 53:1082–1095
Acknowledgements
This work is partly supported by the Doctoral Research Foundation of Jining Medical University under Grant No.2018JYQD03, and the Doctoral Research Foundation of Jining Medical University for Dr. Li Li, and a Project of Shandong Province Higher Educational Science and Technology Program under Grant No.J18KA217, China.
Author information
Authors and Affiliations
Corresponding author
Additional information
Publisher’s note
Springer Nature remains neutral with regard to jurisdictional claims in published maps and institutional affiliations.
Rights and permissions
About this article
Cite this article
Li, L., Gao, J., Ge, H. et al. An effective feature extraction method via spectral-spatial filter discrimination analysis for hyperspectral image. Multimed Tools Appl 81, 40871–40904 (2022). https://doi.org/10.1007/s11042-022-13121-6
Received:
Revised:
Accepted:
Published:
Issue Date:
DOI: https://doi.org/10.1007/s11042-022-13121-6