Abstract
Context
Habitat connectivity is key when designing reserve networks for conservation of species at risk. Acquiring land over time to achieve connectivity for multiple species in a systematic conservation plan can pose a challenge because not all high priority parcels will be acquired, species occurrence data is often limited, and using multiple species models together is complex.
Objectives
We evaluated four possible land acquisition strategies in a such a plan in their ability to meet each of three objectives. The strategies represent different combinations of what are termed ‘Priority 1’, ‘Priority 2’, and ‘Corridor’ lands in the plan. The objectives are to (1) meet conservation target acreages identified in the plan; these are distinct from connectivity goals, (2) maximize structural habitat connectivity, and (3) maximize connectivity for multiple focal species.
Methods
For this case study in Yolo County, California, we compared the efficiency of strategies to meet conservation targets using MARXAN. We compared structural connectivity of MARXAN solutions for each strategy using FRAGSTATS and distance between patches using ArcGIS. We compared focal species connectivity by using ArcGIS to define species-specific least cost networks and then assessing each network’s conformity with MARXAN solutions.
Results
‘Priority 1’ parcels and ‘Corridor’ parcels together provide (1) the most efficient solution for attaining conservation targets, (2) the highest structural connectivity, and (3) high connectivity for the greatest number of focal species.
Conclusions
Because land acquisition patterns are time sensitive and data may be limited, we recommend using spatial prioritization software often and employing several measures of connectivity in decision-making.
Similar content being viewed by others
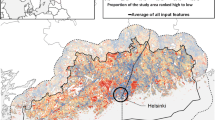
Avoid common mistakes on your manuscript.
Introduction
Connectivity of suitable habitat is a key consideration when planning for species conservation, particularly the long-term survival of a species. A desired conservation outcome for a species would be one in which critical portions of its range have been conserved and there is high connectedness in a reserve system between areas of suitable habitat. Classic works in landscape ecology and conservation highlight the importance of this landscape-level approach to planning for species protection (Noss et al. 1997, Beier and Noss 1998, Crooks and Sanjayan 2006, Wiens 2009). Landscape connectivity has been defined as both structural and functional. Structural connectivity is based on the spatial arrangement of broad structural habitat types or land cover types, such as forests or grasslands, in a landscape while functional connectivity takes species-specific needs into account, such as dispersal behavior (Theobald 2006).
Designing and building reserve networks that achieve connectivity for multiple species can be a challenge for regional conservation planners for two primary reasons. First, acquisition of all priority land parcels identified in a connected reserve system is not guaranteed, due to policies that only allow purchase of property from willing sellers. Second, spatial data representing the distribution of all species of interest is often limited. Plans are forced to rely on suitable habitat as a surrogate for species protection because actual species location or occurrence data is likely incomplete (Noss et al. 1997; Rondinini et al. 2006; Winchell & Doherty 2008). Thus, suitable habitat forms the basis for a species distribution model (SDM) that informs reserve design. Moreover, empirical evidence shows that patterns of species distribution are often constrained by dispersal limitation (Nathan 2001). Yet, even novel approaches that reduce uncertainty in reserve selection by incorporating known species’ dispersal distances in predictive modeling (e.g. Underwood et al. 2010) rely on occurrence records.
Regardless of the level of data supporting a species distribution model (SDM), it can also be a challenge to use multiple SDMs together in reserve design. A search of the literature reveals the complexity involved in using SDMs collectively for this purpose. Some have noted the difficulty in selecting the best SDM for a specific species, let alone an appropriate ensemble modeling method for multiple species (Lin et al. 2018). Others have shown how the vast majority of research on SDMs focuses on methods for creating them rather than their application to decision problems such as reserve selection (Guisan et al. 2013; Mair et al. 2018).
Here we demonstrate the use of limited occurrence data and multiple SDMs in reserve selection decision-making. We evaluate the relative efficiency of four different land acquisition strategies in both meeting conservation targets and attaining connectivity for multiple species within the context of a systematic conservation plan (SCP), the Yolo Habitat Conservation Plan / Natural Community Conservation Plan (hereafter “Yolo HCP/NCCP” or “plan”). The term “systematic conservation planning” comes from the seminal work of the same name published in Nature by Margules and Pressey (2000), who outlined a systematic approach to locating and designing reserves and meeting conservation goals. In California, both Natural Community Conservation Plans (NCCPs) under California’s Endangered Species Act (Fish and Game Code Sect. 2800 et seq.) and federal Habitat Conservation Plans (HCPs) under the Endangered Species Act Sect. 10(a) (1) (B) may be considered systematic conservation plans. Both are intended to establish large reserve networks of permanently protected lands and long-term programs designed to conserve, mitigate for, and manage species legally “covered” by a plan while they allow compatible and appropriate development (Presley 2011). They offer an alternative to traditional approaches to endangered species conservation, which often mitigate or offset impacts to, or “incidental take” of, species on project-by-project basis (McKenney and Kiesecker 2010), a practice that results in uncoordinated, “piecemeal”, and far less effective conservation (Underwood 2010).
Going beyond the requirements of federal HCPs, NCCPs must provide recovery—“methods and procedures within the plan area that are necessary to bring any covered species to the point at which the measures provided pursuant to Chapter 1.5 (commencing with Sect. 2050) [The California Endangered Species Act] are not necessary’” [Sect. 2805(d)]. They and must also provide connectivity—“the establishment of one or more reserves or other measures that provide equivalent conservation of Covered Species within the Plan Area and linkages between them and adjacent habitat areas outside the Plan Area” [Sect. 2820(a)(4)(B)]). In several ways, NCCPs also fit the decision-making framework of SCP as it is defined by Schwartz et al. (2018). First, theoretical foundations for NCCPs are in the fields of landscape ecology and land use planning, what Schwartz et al. term “geospatial planning”. Second, core tools for decision-making include spatial prioritization tools, such as those used in this study. Finally, core tools are applied to designing reserve systems, a key feature of NCCPs.
The Yolo HCP/NCCP (2018) was selected as a case study from among 17 NCCPs approved and being implemented (CDFW 2020) for several reasons. First, it was permitted relatively recently (2019), which means it is early in its implementation phase, before reserve lands have been purchased and when an analysis of potential land acquisition strategies may have the greatest effect on land purchase decisions. Second, although habitat connectivity is required of all NCCPs, this plan faces some unique challenges in not having a large base of public lands within its Conservation Reserve Area upon which to build a connected reserve network. Also, unlike many other NCCPs, the matrix of land cover is not sharply divided between urban or semi-urban parcels and natural habitat but contains agricultural land cover types, which have direct habitat value for some of the covered species and are semi-permeable for others attempting to move through them. Finally, the results are of immediate interest to conservation practitioners. Researchers in conservation have recommended bridging an existing gap between research and practice by sourcing research questions from such practitioners (Knight et al. 2008; Schwartz et al. 2018).
Species distribution models in the Yolo HCP/NCCP are not unlike those of other NCCPs in California in both type and level of data supporting them. In a study of eighteen NCCPs approved or in preparation, Parisi and Greco (2021) found that 17 out of 18 contained mapped occurrences and 15 out of 18 included classified (expert opinion) suitable habitat. The main source of occurrence data for species at risk is the California Natural Diversity Database (CNDDB), a “natural heritage program” data set overseen by NatureServe that has an equivalent in every state (CDFW 2021).
The Yolo HCP/NCCP is also like other systematic conservation plans in identifying top priority lands in the planning phase, before the plan has been approved. Moreover, as an HCP/NCCP plan, it must identify more priority lands than can be acquired because of some uncertainty in the acquisition process. Per existing policy, land purchases must come from willing sellers, so it is likely that some priority parcels will not become available. Similar to other HCP/NCCPs, the Yolo plan has outlined the general shape of a connected reserve system on the landscape and has identified more than one level of priority lands. In this case, Priority 1 lands are clustered around existing public and easement lands and Priority 2 lands clustered around these (Fig. 1). Corridors have also been identified to help achieve structural connectivity. In looking at the amount and pattern of priority lands, one general question arose immediately: Is it possible to meet conservation objectives for species in a plan—i.e. meet target acreages of defined suitable habitat—and still not achieve a highly-connected system over the plan’s permit term? Priority 1 lands alone total 90,170 acres (36,491 ha). At the rate of land acquisition needed to meet the plan’s 24,406-acre (9877 ha) commitment in 50 years, an average of 488 acres (197 ha) per year, it would take an additional 135 years to acquire all of them. There are an additional 136,000 acres (53,007 ha) of Priority 2 lands plus lands within identified corridors (Fig. 6–3 of the plan). Given this, what is the best land acquisition strategy? Our analysis centers around three research questions: (1) What is the most efficient strategy for meeting conservation targets?, (2) What is the most efficient way to maximize structural habitat connectivity in the plan?, and (3) Is there a way to maximize connectivity for multiple focal species in a single plan?
Study area
The Yolo HCP/NCCP plan area covers the entirety of Yolo County, California. However, the parcels eligible for analyses and acquisition reside only in the Conservation Reserve Area, which the plan defines as the valley floor (Fig. 1). Yolo County is located within California’s Great Central Valley (Fig. 1) and is characterized by a Mediterranean-type climate, with cool, wet winters and hot, dry summers. Elevations in the county range from less than 100 feet above mean sea level on the valley floor in the eastern side of the county to approximately 3100 feet above sea level within the mountains forming the county’s northwest corner, the highest elevation being Little Blue Peak at 3123 feet (Fig. 1). Major hydrologic features include Cache Creek in the northern part of the county; Putah Creek, which forms much of the southern edge of the county; and the Sacramento River, which forms much of the county’s eastern border. The valley floor is a matrix of agricultural lands and grasslands, some with seasonal wetlands, surrounding the county’s four incorporated cities – Woodland, West Sacramento, Davis, and Winters. The highest elevations are characterized primarily as oak woodlands while riparian habitat lines the creeks and is found in remnants along the Sacramento River.
Methods
Methods and data sources for this study are summarized in Table 1. To determine efficient solutions for meeting conservation targets, we employed a widely used semi-optimization software package called MARXAN (Ball et al. 2011) to compare four possible land acquisition strategies (Fig. 2), all within the Conservation Reserve Area depicted in Fig. 6–5 of the plan. MARXAN is a spatial prioritization software tool that uses an algorithm to evaluate the effectiveness of different combinations of planning units in achieving conservation goals. It selects planning units that, together, minimize both cost and boundary length, while meeting a set of conservation targets. Cost is defined by the user and can be both economic and ecological. Scenario A considers all parcels within the Conservation Reserve Area in selecting reserves. Scenario B includes only parcels identified as “higher priority” in Fig. 6–6 of the plan (Priority 1). Scenario C is comprised of parcels identified as “higher priority” and “lower priority” (Priority 1 and 2, respectively). Scenario D includes both “higher priority” (Priority 1) parcels and parcels inside the ecological corridors shown in Figure ES-2 and Fig. 6–3 of the plan. All input data for identifying conservation targets (Table 2) was vector-based geographic information system (GIS) data available from the Yolo Habitat Conservancy (2020b) and all data preparation for MARXAN was done in ArcGIS Desktop 10.7.1 (ESRI Inc.© 2019).
a b Four land acquisition scenarios analyzed in MARXAN. Parcels eligible for selection in each MARXAN scenario are within the Conservation Reserve Area and marked by diagonal hatching. c d Four land acquisition scenarios analyzed in MARXAN. Parcels eligible for selection in each MARXAN scenario are within the Conservation Reserve Area and marked by hatching
We chose land ownership parcels as a planning or reserve selection unit for MARXAN, considering this the most realistic way to identify reserve network solutions, as land will be acquired in whole parcels. The alternative would be to create a regularly-spaced planar tessellation surface, composed of hexagons for example. For parcel boundaries in Scenario A, we downloaded publicly available tax assessor data from Yolo County (2020) and, using the ArcGIS extraction analysis tool ‘Clip,’ we clipped it to the Conservation Reserve Area boundary. Priority 1 and 2 lands layers for scenarios B, C, and D were available from the Yolo Habitat Conservancy (2020a) as selected parcels. For the ecological corridor portion of Scenario D, we intersected the downloaded parcel data with a boundary layer we created to represent ecological corridors. To create this boundary layer, we selected ecological corridor polygons (planning units 7, 9, 17 and 18) from the Planning Units layer depicted in Figure ES-2 and Fig. 6–3 of the plan (Yolo Habitat Conservancy 2020b). The plan also includes a corridor for the Sacramento River. To create this polygon, we buffered a GIS line feature of the river by 0.25 miles or 1320 feet on each side, giving it initially the same width as the Cache Creek Corridor, and then clipped the buffered feature to exclude that portion of the river in neighboring Sacramento County. Once the ecological corridor layer and parcel layer were intersected, any polygon slivers without an identifying assessor’s parcel number, often those labeled “road” or “river”, were deleted.
To prepare biological data for MARXAN, we first identified the total conservation commitment acreage (24,406 acres, 9877 ha) from the Yolo HCP/NCCP within the conservation reserve area and then each unique conservation target (Table 1, based on Table 6–2 [a] of the plan). A target may be a single element, such as a natural community type, or a combination of elements, such as a natural community type that is also modeled habitat for a species or also in a particular location in the planning area. The source for natural community types was land cover data mapped in Figs. 2–5 through 2–10 of the plan. For species modeled habitat, we used the data depicted in the species maps in Appendix A: Covered Species Accounts. Location data are the planning units shown in Figure ES-2 of the plan. Where a conservation target represented the intersection of two elements, such as acres of a natural community type that are also modeled habitat for a species, the two element layers were intersected and the polygons from the resulting layer dissolved into one. The dissolved polygon was then intersected with the parcel layers representing the four scenarios (Fig. 2) and the acreage by parcel of a conservation target calculated for each scenario. English units have been used here for both analysis and reporting of results as these are the sole units utilized in the plan. International (SI) units are reported parenthetically.
The data representing conservation targets and their values by planning unit (parcel) were then reformatted into a tab-delimited Planning Unit versus Conservation Feature, one of several input files required by MARXAN. In the Planning Unit input file, we set the cost for each parcel as its calculated acreage. Authors of the MARXAN good practices handbook (Ardron et al. 2010) are aware that parcel cost information is not always available, which is the case in this study, and note that reserve area can be used as a surrogate for cost based on the assumption that the larger the reserve size the more costly it will be to implement and manage, although this is not always the case. The status was set at “0” for parcels potentially selected as new reserves, allowing these parcels to be in the initial (or seed) reserve system. The status of existing reserves was set at “2”, forcing them into selection as the initial part of a reserve system, although their conservation values do not appear in any input files because they do not contribute to new conservation targets.
Because a compact and connected reserve system with minimal edge is a desired outcome for this plan, an optional ‘boundary length’ input file and ‘boundary length modifier’ (BLM) were used in this analysis. According to Ardron et al. (2010), a compact reserve system is one with a low edge to area ratio. Reducing the edge to area ratio of a reserve network results in multiple benefits including a smaller number of reserves, lower management and transaction costs, and potentially more viable populations and ecological processes. A BLM improves the compactness of reserve system solutions by accounting for a connectivity cost between reserves based on the effective length of their shared boundaries. The BLM value is set by the MARXAN user and represents a tradeoff between reserve cost and the desire for compactness. Boundary length between parcels included in each scenario was calculated using the ‘Polygon Neighbors’ tool in ArcGIS and the results were reformatted for input to MARXAN. The BLM was determined using a method from Stewart and Possingham (2005) as cited in Game and Grantham (2008). Total reserve system boundary length was plotted against total cost in area for repeated MARXAN runs, at 100 iterations each, with different BLM values. BLM values of 0.0, 0.04, 0.05, 0.07, 0.075, 0.08, 0.1, 0.5, and 1.0 were tested. The selected BLM value of 0.08 was at the inflection point in which both total boundary length and total cost were at a minimum.
For each scenario, we conducted 10 MARXAN runs of 100 repetitions each and recorded the MARXAN “best” single solution in a run as well as the “summed” solution, which includes the selection frequency of each parcel calculated across all repetitions in that run. Using both solutions is recommended as a best practice for interpreting and refining MARXAN outputs as well as communicating them spatially (Ardron et al. 2010). We did not find it necessary to include very high numbers of runs and repetitions because, according to Ardron et al. (2010), the feasible solutions generated were all within a few percent of each other in the objective function – a measure of efficiency that is a function of planning unit costs, boundary costs, and penalties – and thus the best solutions generated among them were also all likely to be the most optimal.
We calculated average cost in acres and planning units of the “best” single solutions among the 10 runs. As a way of identifying the “best of the best” parcels for each scenario, or a best summed solution, we also averaged selection frequency values for each parcel across the 10 runs and, because data were unevenly distributed, classified the averaged values using natural breaks. We used this natural break to select a cut-off point for the class of parcels selected most often. For example, in Scenario A, which includes all parcels in the Conservation Reserve Area, the parcels retained as part of the best summed solution were selected at least 40% of the time. Conservation targets not met in each scenario were taken from MARXAN output files that report these data.
To determine efficient solutions for maximizing structural connectivity, we compared connectivity of the MARXAN solutions for each scenario using FRAGSTATS (McGarigal et al. 2013) and the ‘Average Nearest Neighbor’ tool in ArcGIS. Analyses were performed on both the summed solution for each scenario, featuring the most frequently selected parcels, and a randomly selected single “best” solution from among the 10 runs in MARXAN. We chose to analyze single best as well as summed solutions, reasoning that a summed solution with only the most frequently selected parcels from many repetitions is likely to be larger and more contiguous than any one single solution and may show a different level of structural connectivity.
To prepare data for FRAGSTATS, we first converted parcel polygons from each solution layer from vector to raster format in ArcGIS, with a cell (pixel) size of 30 feet (900 square feet). Slivers – residual single pixels or strings of raster cells a single pixel wide – were eliminated. To analyze patch shape and spatial connectedness within each of the eight solution layers, we selected Perimeter-Area Ratio (PARA) and Contiguity Index (CONTIG) from among the patch shape metrics in FRAGSTATS using an eight-cell neighbor rule and averaged the resulting values across all patches. Both PARA and CONTIG may be considered measures of shape complexity. FRAGSTATS identifies unique patches of contiguous pixels as a part of calculating these statistics. PARA equals the ratio of a patch perimeter to its area and emphasizes elongation (Ardron et al. 2010). It can point to longer, narrower habitat patches that may be subject to negative edge effects. CONTIG assesses the spatial connectedness, or contiguity, of cells within a grid-cell patch to provide an index on patch boundary configuration (LaGro 1991). It may point to patches that are in the process of fragmenting. CONTIG convolves a 3 × 3 pixel template as a moving window and assigns values to pixels based upon class (patch type of interest versus background) and spatial relationship to surrounding pixels (horizontal and vertical weighted higher than diagonal). The value of each pixel in the output image, computed when at the center of the moving template, is a function of the number and location of pixels, of the same class, within the nine-cell image neighborhood. Thus, large contiguous patches result in higher values (McGarigal 2015). The average contiguity value is divided by the sum of template values minus one to yield an index value between 0 and 1.
As a measure of patch distribution, we used the ‘Average Nearest Neighbor’ tool in ArcGIS on the vector versions of each solution layer. This tool reports the mean distance between polygon centroids.
To assess connectivity for selected focal species, we used the ‘Cost Connectivity’ tool in ArcGIS. We chose the most dispersal-limited covered species in each of two major landscape matrices within the plan – burrowing owl (Athene cunicularia) and California tiger salamander (Ambystoma californiense), within the agricultural landscape, which includes grasslands and some seasonal wetlands such as vernal pools, and western pond turtle (Emys marmorata) and valley elderberry longhorn beetle (Desmocerus californicus dimorphus) in the riparian/wetland landscape. In a report of independent science advisors for the Yolo HCP/NCCP (Spencer et al. 2006), the authors recommend the use of focal species to help achieve the biological goals of the plan, and one such way to categorize species is by a combination of functional category such as dispersal limitation and major community type.
The ‘Cost Connectivity’ tool focuses on defining the optimum network of least-cost paths between regions, defined as core habitat patches for the purposes of this study. For each species, we considered a core habitat patch to be any patch of suitable habitat with a recent occurrence of a species (Source: CDFW 2021) or any patch of suitable habitat within public or easement land, considering this habitat to be already protected. Recent occurrences for a species and suitable habitat are the data depicted in its species map in Appendix A: Covered Species Accounts of the plan. The goal was not to define a single movement path for each species to disperse through from its point of occurrence, but to look at what the pattern of dispersal might look like through the most highly suitable habitat and see how well it conforms with the four scenarios or land acquisition strategies of this study. So as not to limit the analysis to within-plan boundaries that would be artificial to species, we chose habitat patches rather than parcel boundaries as cores and extended the initial scope of the analysis to public lands beyond the Conservation Reserve Area.
After converting each composite layer of patches for a species from vector to raster format, we used the ‘Region Group’ tool in ArcGIS to create an input regions layer for the ‘Cost Connectivity’ tool. To create an input cost surface layer for the same tool, we assigned cost values to polygons in the land cover data layer or individual species distribution model (Yolo Habitat Conservancy 2020b) per the rules in Table 3 and then rasterized and merged the input data sets. The highest costs are for the least permeable forms of land cover and the lowest costs for the most highly suitable habitat for a species.
We then assessed conformity of the least cost network solution for each species (Fig. 3) with the best summed solution for each of the four scenarios (Fig. 4). Each least cost network was first clipped to the Conservation Reserve Area so it could be compared directly with the selected parcels for each best summed solution, which are limited to this portion of the plan area. Percent of least cost network segments intersecting each best summed solution was determined using the ‘Select by Location’ function in ArcGIS and calculating the percentage of path segments selected. We then determined the median path cost value of selected path segments. The distribution of path cost values was often highly skewed or bimodal; thus, we selected median rather than mean as a preferred measure of central tendency.
Results
Results regarding the most efficient strategy for meeting conservation targets are presented in Table 4. Readers will note that efficiency is measured in numbers of parcels needed rather than acreages of targeted natural communities or species modeled habitat. This is because, practicably, land will be acquired in whole parcels. Parcels are not likely to be subdivided for sale unless considered for land development and, even if divided, not likely by natural community or habitat boundaries. Scenario A, which considers all parcels in the Conservation Reserve Area, hits the highest number of conservation targets (20/21), but is also the least efficient in doing so, with an average of 2241 planning units to meet these targets. Scenario B, which considers only Priority 1 parcels, hits the fewest number of conservation targets (15/21), but is also the most efficient in doing so, with an average of 404 planning units. The best combination of attaining conservation targets (18/21) and doing so with efficiency (an average of 422 planning units) appears to be Scenario D, which considers Priority 1 lands and corridors. Scenario C, which considers Priority 1 and 2 parcels is also relatively effective and efficient with 19/21 conservation targets hit and an average of 513 parcels required. The relative patterns of both effectiveness and efficiency hold up across all four scenarios when considering both MARXAN single best solutions and MARXAN summed solutions.
Results regarding the most efficient way to maximize structural habitat connectivity in the plan are presented in Table 5, along with their adherence to some classic principles of reserve design posited by Diamond (1975) and Noss et al. (1997). MARXAN best summed solutions for each scenario are displayed in Fig. 4. Actual values of both FRAGSTATS and ArcGIS Average Nearest Neighbor measures are less important than comparative or relative values. Scenario A with all parcels results in habitat patches that are closest together on average, but they are many and small, with the highest perimeter-area ratio and the lowest within-patch contiguity. Scenario B, with Priority 1 lands only, and Scenario D, with Priority 1 lands and corridors, both result in fewer, larger habitat patches with low perimeter-area ratio and high within-patch contiguity, but also the greatest average distance between patches. Scenario C, with Priority 1 and 2 lands, – results in habitat patches closer together than Scenarios B or C, with a moderate perimeter-area ratio and relatively high within-patch contiguity.
Results of our analysis of connectivity for selected focal species are illustrated in Fig. 3 and presented in Table 6. The least cost pattern of movement for the burrowing owl had the highest conformance with Scenario D, Priority 1 parcels and corridors but the lowest average cost consistent with Scenario A, all parcels. For the California tiger salamander, the least cost network had the highest conformance and the lowest average cost with Scenario D, Priority 1 parcels and corridors. The western pond turtle network had the highest conformance with Scenario B, Priority 1 and 2 Parcels, with the same average cost across all scenarios. Finally, the valley elderberry longhorn beetle network had the highest conformance with Scenario A, all parcels, and the lowest average cost with Scenario C, Priority 1 and 2 parcels, and Scenario D, Priority 1 parcels and corridors.
Results of each measure were then ranked across all four scenarios, with one representing the highest rank and four the lowest rank for any given measure. We summed the ranked scores for each scenario and assigned an overall ranking based on the summed value, with the lowest summed value representing the highest overall rank (Table 7). Scenario D, with Priority 1 lands and corridors shows the highest overall ranking, followed by Scenario B, with Priority 1 lands only; Scenario C, with Priority 1 and 2 lands; and, Scenario A, with all parcels.
Discussion
With ever-increasing landscape fragmentation due to human population expansion resulting in critical needs for conservation and limited resources with which to accomplish those actions, it is increasingly important that the potential outcomes of systematic conservation strategies be assessed for their efficacy. This case study presents several potential conservation outcomes over a 50-year build-out time period for a county that has gone through an extensive effort at systematic conservation planning with a goal of habitat connectivity for multiple species. Also, data may be limited in a multi-species systematic conservation plan, yet strategic decisions must still be made to maximize efficiency and effectiveness when assembling a connected reserve system over time. One goal here was to consider several measures that may serve as inputs to such decision making.
For the Yolo HCP/NCCP it would seem most effective to focus on Priority 1 lands and corridors, although additional lands must still be acquired to fully meet all conservation targets. Priority 1 and 2 lands together may not prove as effective, particularly because the advantage of Priority 2 lands lies in their adjacency to Priority 1 lands. If they are selected as an alternative to Priority 1 lands, the result may be less compactness and connectivity than Priority 1 lands would achieve alone or in conjunction with corridors. The Priority 1 lands with corridors strategy also holds up well for individual focal species, likely because many of the existing public lands are in the grassland/agriculture landscape matrix and many of the corridor lands are in both this matrix or in the riparian/wetland matrix along streams. We recommend using spatial prioritization software and employing several measures of connectivity in decision-making, and doing so either in the planning phase of a systematic conservation plan or early in the process of acquiring land for an approved plan, before reserve lands have been purchased and when an analysis of potential land acquisition strategies may have the greatest effect on land purchase decisions.
Results here may be limited in that they illustrate best-case scenarios for connectivity under specific, idealized criteria for a total conservation target commitment of 24,406 acres (9877 ha) in Yolo County. No single strategy for parcel acquisition is likely to be exactly realized due to a policy for the plan which dictates that no parcel may be purchased without a willing seller. Thus, some parcels may be unavailable for acquisition and may preclude ever achieving a fully connected reserve system in Yolo County unless the permit term for the plan is extended well beyond 50 years. It is also worth noting that few Priority 1 lands exist on Putah Creek, an important riparian corridovr that forms the southern boundary of the plan west of the county’s southeastern panhandle, so many parcels here were ignored by the optimization scenarios. If land parcels along Putah Creek are acquired in the future for recreational or other purposes it could provide the basis for greater acquisitions by the Yolo HCP/NCCP plan to enhance connectivity in this riparian corridor. For these reasons, we recommend that MARXAN be re-run after each parcel is acquired in real time to re-assess connectivity opportunities, as results are highly sensitive to initial conditions.
Although NCCPs such as the Yolo HCP/NCCP are required to plan for habitat connectivity for covered species, the temporal dimension of land acquisition illustrates why maximizing connectivity for multiple focal species in a single plan is an ongoing process. Unexpected future land title or easement donations with high conservation value can alter the course of implementing the plan and create opportunities in new areas at the expense of other areas. Conversely, unexpected urban development in areas adjacent to the plan or even, in the case of the Yolo HCP/NCCP, a shift within the agricultural matrix from herbaceous and semi-permeable crops to orchards or vineyards with lower connectivity value, can alter future acquisition priorities based on a lowering of conservation value.
Changes in data availability also point to the need for ongoing assessments of efficiency and connectivity. Additional species occurrence data and even finer-grained vegetation mapping, particularly along fragmented riparian corridors, may become available over time. Occurrence data can confirm or even alter expert-driven ratings of habitat suitability for a species, the basis of both the species models developed for the Yolo HCP/NCCP and the cost surface layers developed here for least cost path modeling. Finer-grained vegetation mapping can lead to more precise dispersal patterns predicted by least cost corridor modeling for species such as the Valley Elderberry Longhorn Beetle (Desmocerus californicus dimorphus), who are associated with a single host plant species found within a natural community or habitat type. More actual measures of parcel cost other than reserve size used as a surrogate may also become available. Authors of the MARXAN best practices manual (Ardron et al. 2010) acknowledge that reserve size does not necessarily correlate with cost yet, at present, these authors have no other practical metrics of cost available to them.
This Yolo County case study shows that the efficacy of systematic conservation strategies can vary significantly depending on how priority lands are considered together in achieving conservation targets and maximizing structural and functional connectivity; how the pattern of acquisition can change over time; and, when and in what format new data becomes available. Conservation planners need to be cognizant of all these factors in developing and implementing future systematic conservation plans, including federal HCPs and California NCCPs for species at risk.
Data availability
The datasets generated during the current study are available from the corresponding author on reasonable request. Source data layers used in the analysis were made available to the authors by the Yolo Habitat Conservancy (2020a, 2020b), were publicly available on-line from Yolo County (2020), or were made available by the California Department of Fish and Wildlife through a subscription (CDFW 2021).
References
Ardron, J.A., Possingham, H.P., and C.J. Klein. 2010 Marxan good practices handbook. Pacific Marine Analysis and Research Association, Victoria
Ball, I., H. Possingham and M. Watts. 2011. Marxan version 2.4.3. marine reserve design via annealing. https://marxansolutions.org/software/
Beier P, Noss RF (1998) Do habitat corridors provide connectivity? Conserv Biol 12:1241–1252
California Department of Fish and Wildlife (CDFW) (2020) Special Animals and Special Plants lists. https://wildlife.ca.gov/Data/CNDDB/Plants-and-Animals
California Department of Fish and Wildlife (CDFW) (2021) California Natural Diversity Database. https://wildlife.ca.gov/Data/CNDDB
Diamond JM (1975) The island dilemma: lessons of modern biogeographic studies for the design of natural preserves. Biol Cons 7:129–146
Game ET, Grantham HS (2008) Marxan user manual: for Marxan version 1.8.10. University of Queensland, St. Lucia, Queensland, Australia, and Pacific Marine Analysis and Research Association, Vancouver, British Columbia, Canada. https://pacmara.org/marxan-user-manual-for-marxan-version-1-8-10
Guisan A, Tingley R, Baumgartner JB, Naujokaitis-Lewis I, Sutcliffe PR, Tulloch AIT, Regan TJ, Brotons L, McDonald-Madden E, Mantyka-Pringle C, Martin TG, Rhodes JR, Maggini R, Setterfield SA, Elith J, Schwartz MW, Wintle BA, Broennimann O, Austin M, Ferrier S, Kearney MR, Possingham HP, Buckley YM (2013) Predicting species distributions for conservation decisions. Ecol Lett 16:1424–1435.
Knight AT, Cowling RM, Rouget M, Balmford A, Lombard AT, Campbell BM (2008) Knowing but not doing: selecting priority conservation and the research-implementation gap. Conserv Biol 22:610–617.
LaGro J Jr (1991) Assessing patch shape in landscape mosaics. Photogrammetric Eng Remote Sens 57:285–293
Lin Y, Lin W, Anthony J, Ding T, Mihoub J, Henle K, Schmeller DS (2018) Assessing uncertainty and performance of ensemble conservation planning strategies. Landsc Urban Plan 169:57–69.
Mair L, Mill AC, Robertson PA, Rushton SP, Shirley MDF, Rodriguez JP, McGowan PJK (2018) The contribution of scientific research to conservation planning. Biol Cons 223:82–96.
Margules CR, Pressey RL (2000) Systematic conservation planning. Nature 405:243–253
McGarigal, K., S. A. Cushman, and E. Ene. 2013. FRAGSTATS version 4.2.1: spatial pattern analysis program for categorical and continuous maps. http://www.umass.edu/landeco/research/fragstats/fragstats.html
McGarigal, K. 2015. FRAGSTATS help. LandEco Consulting. University of Massachusetts, Amherst. https://www.umass.edu/landeco/research/fragstats/documents/fragstats.help.4.2.pdf
McKenney BA, Kiesecker JM (2010) Policy development for biodiversity offsets: a review of offset frameworks. Environ Manag 45:165–176
Nathan R (2001) Dispersal biogeography. In: Levin SA (ed) Encyclopedia of biodiversity. Academic Press, San Diego
Noss RF, O’Connell MA, Murphy DD (1997) The science of conservation planning: habitat conservation under the Endangered Species Act. Island Press, Washington D.C
Parisi, M. D. and S. E. Greco. 2021. The explicit integration of species conceptual models and species distribution models as a best practice for systematic conservation planning in California. California Fish and Wildlife Special CESA
Presley GL (2011) California’s natural community conservation program: Saving species habitat amid rising development. In: Arha K, Thompson BH (editors). The endangered species act and federalism: Effective conservation through greater state commitment. Routledge, New York, New York. https://doi.org/10.4324/9781936331857
Rondinini C, Wilson KA, Boitani L, Grantham H, Possingham HP (2006) Tradeoffs of different types of species occurrence data for use in systematic conservation planning. Ecol Lett 9:1136–1145.
Schwartz MW, Cook CN, Pressey RL, Pullin AS, Runge MC, Salafsky N, Sutherland WJ, Williamson MA (2018) Decision support frameworks and tools for conservation. Conserv Lett 11:1–12
Spencer, W. (lead advisor/facilitator), R. Noss, J. Marty, M. Schwartz, E. Soderstrom, P. Bloom, G. Wylie, and S. Gregory (contributor). 2006. Report of independent science advisors for Yolo County Natural Community Conservation Plan/Habitat Conservation Plan (NCCP/HCP). https://wildlife.ca.gov/Conservation/Planning/NCCP/Scientific-Input
Stewart RR, Possingham HP (2005) Efficiency, costs and trade-offs in marine reserve system design. Environ Model Assess 10:203–213.
Theobald DM (2006) Exploring the functional connectivity of landscapes using landscape networks. In: Crooks KR, Sanjayan M, M (eds) Connectivity conservation. Cambridge University Press, Cambridge
Underwood JG (2010) Combining landscape-level conservation planning and biodiversity offset programs: a case study. Environ Manage 47:121–129.
Underwood JG, D’Agrosa C, Gerber LR (2010) Identifying conservation areas on the basis of alternative distribution data sets. Conserv Biol 24:162–170
Wiens JA (2009) Landscape ecology as a foundation for sustainable conservation. Landscape Ecol 24:1053–1065.
Winchell CS, Doherty PF Jr (2008) Using California gnatcatcher to test underlying models in Habitat Conservation Plans. J Wildl Manag 72:1322–1327.
Yolo County (2020) Yolo County tax parcels open data. GIS data. https://yodata-yolo.opendata.arcgis.com/
Yolo Habitat Conservancy (2020a) PriorityLands.gdb. GIS data.
Yolo Habitat Conservancy (2020b) YoloHCPNCCPbase.gdb. GIS data.
Yolo Habitat Conservation Plan/Natural Community Conservation Plan (2018) https://www.yolohabitatconservancy.org/documents
Acknowledgements
Thank you to Chris Alford of the Yolo Habitat Conservancy for expert assistance in providing spatial data for this analysis.
Funding
The authors declare that no funds, grants, or other support were received during the preparation of this manuscript.
Author information
Authors and Affiliations
Contributions
All authors contributed to the study conception and design. Data analysis was performed by MDP, in consultation with PRH and SEG. The first draft of the manuscript was written by MDP and all authors commented on this and subsequent drafts. All authors read and approved the final manuscript.
Corresponding author
Ethics declarations
Conflict of interest
The authors have no competing financial interests to the process or outcome of the study.
Additional information
Publisher's Note
Springer Nature remains neutral with regard to jurisdictional claims in published maps and institutional affiliations.
Rights and permissions
Open Access This article is licensed under a Creative Commons Attribution 4.0 International License, which permits use, sharing, adaptation, distribution and reproduction in any medium or format, as long as you give appropriate credit to the original author(s) and the source, provide a link to the Creative Commons licence, and indicate if changes were made. The images or other third party material in this article are included in the article's Creative Commons licence, unless indicated otherwise in a credit line to the material. If material is not included in the article's Creative Commons licence and your intended use is not permitted by statutory regulation or exceeds the permitted use, you will need to obtain permission directly from the copyright holder. To view a copy of this licence, visit http://creativecommons.org/licenses/by/4.0/.
About this article
Cite this article
Parisi, M.D., Huber, P.R. & Greco, S.E. Assessing conservation outcomes and maximizing habitat connectivity for multiple species in systematic conservation plans: a case study in Yolo County, California. Landsc Ecol 38, 1621–1642 (2023). https://doi.org/10.1007/s10980-023-01664-4
Received:
Accepted:
Published:
Issue Date:
DOI: https://doi.org/10.1007/s10980-023-01664-4