Abstract
The rising pervasiveness of Artificial Intelligence (AI) has led applied linguists to combine it with language teaching and learning processes. In many cases, such implementation has significantly contributed to the field. The retrospective amount of literature dedicated on the use of AI in language learning (LL) is overwhelming. Thus, the objective of this paper is to map the existing literature on Artificial Intelligence in language learning through bibliometric and content analysis. From the Scopus database, we systematically explored, after keyword refinement, the prevailing literature of AI in LL. After excluding irrelevant articles, we conducted our study with 606 documents published between 2017 and 2023 for further investigation. This review reinforces our understanding by identifying and distilling the relationships between the content, the contributions, and the contributors. The findings of the study show a rising pattern of AI in LL. Along with the metrics of performance analysis, through VOSviewer and R studio (Biblioshiny), our findings uncovered the influential authors, institutions, countries, and the most influential documents in the field. Moreover, we identified 7 clusters and potential areas of related research through keyword analysis. In addition to the bibliographic details, this review aims to elucidate the content of the field. NVivo 14 and Atlas AI were used to perform content analysis to categorize and present the type of AI used in language learning, Language learning factors, and its participants.
Similar content being viewed by others
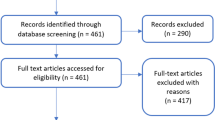
1 Introduction
Artificial Intelligence (AI) holds a pivotal role in rebuilding our society and ‘promising a new era’ in prospective times for its capabilities to act as intelligent beings in various domains including education (Farrokhnia et al. 2023; Górriz et al. 2020). An upsurge, in recent times, on the application of AI in educational sectors, has exhibited notable development, and there has been an equivalent explosion in the number of new AI tools accessible (Chu et al. 2022; Popenici and Kerr 2017). In the field of education, researchers report on the opportunities that AI presents for instructors and learners (Chen et al. 2020). This evolutionary trajectory of AI in education is increasing, showcasing an exponential growth of its explorations across disciplines including language education (Jeon et al. 2023).
The use of AI, in particular with language learning, is appreciated for providing the students with individualized attention, “personalized, interactive, and authentic language learning contexts” in the form of intelligent tools such as Interactive Personal Assistants, web-based systems, virtual reality systems, or chatbots (Lin and Chang 2020; Liang et al. 2021; Wijekumar et al. 2013; Rahman and Tomy 2023; Zhang et al. 2023). Moreover, it allows the teachers/instructors to monitor their students/learners, which reduces their workload and frees the teachers thus allowing them to prioritize the curriculum over repetitive tasks (Pokrivčáková 2019). Integration of AI techniques such as natural language processing (NLP), natural language understanding (NLU), and automatic speech recognition (ASR) allows the use of tools developed through them to be more appropriate in language learning platforms as they comprehend and process human-computer interaction (Lee and Jeon 2022; Shadiev and Liu 2022).
While multiple studies address the research gaps in using AI in language learning, the advancement of AI is at a much faster phase extending the research need perpetually. Gruetzemacher (2022) states that “In the past two years, Language-based AI has advanced by leaps and bounds, changing common notions of what this technology can do”. The technological impediments of the past decades are no longer the present-day concerns. Therefore, the research trends and findings of previous studies slither on every massive leap of AI techniques. For example, in 2017, advancements in word embedding (Peters et al. 2017), and the introduction of Transformer model architecture in the field of NLP outperformed prior recurrent neural network models and offered a novel technique to carry out sequence transduction tasks (Vaswani et al. 2017). Later, the launch of ChatGPT and GPT-4 in 2022 and 2023 offered remarkable conversational capabilities and longer text processing demonstrating keen anticipation for NLPs and the next phase of human-computer interaction (Weitzman 2023). Such advancements of NLP greatly help intelligent systems uncover the unstructured data produced by humans.
The production and reception of natural language consequently expand the use of AI for language learning. Past studies have examined these phenomena of AI in language learning and education. For instance, Liang et al. (2021) performed a bibliographic analysis and systematic review on 71 articles on AILEd (AI in language education) on December 31, 2020. Even though research on language teaching and learning in connection with AI is active, given the dynamic progressive nature of AI techniques, reviewing their implications and applications on language learning, within the designated timeframe, could considerably contribute to the field. Hence, it is essential to state the art of AI in language learning at timely intervals. To review the state-of-art of AI in language learning, a bibliometric analysis was performed. This analysis could segment a “large volume of scientific publications” with the “advancement, availability, and accessibility of bibliometric software and scientific databases”, (Donthu et al. 2021). It could objectively point out the performance and emerging trends in the given field including topics and authors (Verma and Gustafsson 2020). With the study’s primary objective being to review the developments in academic research on AI and language learning between the years 2017 and 2023, the study focuses (1) to analyze the publication trends and growth patterns of AI in language learning (2) to identify the key contributors, collaboration patterns, and influential works in the field (3) to explore the dominant research themes and emerging trends. To operationalize the objectives of the research, they were converted into the following research questions to identify the mentioned publication metrics.
1.1 Research questions
-
RQ1: What are the publication trends and metrics of performance analysis such as Publication, Citation, and both Citation and publication-related metrics?
-
RQ2: Who or Which are the most influential and productive authors, institutions, journals, and countries?
-
RQ3: What are the key research themes, frequent and prominent keywords obtained from title, abstract, and keywords through keywords analysis?
-
RQ4: What are the documents and clusters that are connected to a common document’s reference through bibliographic coupling?
-
RQ5: what are the inferences obtained by analyzing the content of all the documents in the study through content analysis? With the study’s primary objective being to review the developments in academic research on AI and language learning between the years 2017 and 2023, the study focuses (1) to analyze the publication trends and growth patterns of AI in language learning (2) to identify the key contributors, bibliographic clusters, and influential works in the field (3) to explore the dominant research themes and emerging trends. To operationalize the objectives of the research, they were converted into research questions to identify the mentioned publication metrics. (RQ1) provides valuable insights into the growth and impact of this interdisciplinary domain. This information can guide researchers, policymakers, and educators in identifying areas of prominence and potential gaps in the literature. (RQ2) allows us to recognize key contributors to the field and potentially foster collaborations. Additionally, (RQ3) helps in analyzing key research themes and prominent keywords to comprehend the evolving discourse and focus of research in this area. Moreover, exploring document connections through bibliographic coupling (RQ4) aids in mapping the intellectual structure of the field. Lastly, extracting inferences through content analysis (RQ5) offers insights into the practical implications of the existing research, potentially informing pedagogical tools used, their frequency of usage, the target learners and also the language learning factors. In sum, addressing these research questions not only contributes to the scholarly understanding of this domain but also has practical implications for educators, researchers, and stakeholders invested in the intersection of AI and language learning.
2 Background of the study
2.1 Artificial intelligence
While multiple researchers have laid out technical definitions to bind AI within a school of thought, Russell and Norvig (2010) categorizes these definitions under two dimensions. First, it has the ability to imitate, think and act humanly and rationally. Second, its connection with the thought process and reasoning along with its behavior. In a broader context, AI involves computing technology that allows machines to mimic human intelligence “in analysis, reasoning, decision making, and self-correction” (Liang et al. 2021; Pokrivčáková 2019). To perform the above-mentioned operations, a wide variety of techniques and methods are used such as “machine learning, adaptive learning, natural language processing, data mining, crowdsourcing, neural networks or an algorithm” (Pokrivčáková 2019).
Despite its complicated mechanisms and progressive developments, IBM (n.d) states that “there is no practical examples of strong AI in use today”. However, as given in Table 1, AI has had many technological breakthroughs from computer vision to advanced natural language processing techniques over a brief period and it is, in many cases, being considered a substitute for human intelligence. At its present rate of growth, AI is presumed to surpass human intelligence. Thus, a review of its applications in various domains is a pressing priority.
2.2 Integration of artificial intelligence in language learning
The incorporation of AI in any field can be in multiple technological forms, tools, or software (Thayyib et al. 2023). Similarly, in language learning and acquisition, a diverse assortment of tools is being integrated with artificial intelligence as it offers language teachers and learners “personalized, interactive and adaptive learning experiences that cater to individual’ needs and preferences” (Rusmiyanto et al. 2023; Pokrivčáková 2019). Research is being carried out to identify appropriate AI-assisted tools to improve each language skill (Rahman et al. 2022) and on the integration of each tool to develop specific areas of language learning and acquisition.
The possibilities of advanced technological input through AI have made Computer Assisted Language Learning (CALL) conventional. Researchers argued that CALL had been limited when proposed with activities directed towards communication and interaction between students such as role plays, discussions, and sharing opinions (Amaral and Meurers 2011). The notion of computers replacing humans in CALL was skeptical as language learning reinforces negotiating meaning and having real-time conversations. Later, the increased possibility of human-like interaction with computers through AI techniques, made Intelligent Computer Assisted Language Learning (ICALL) gain its state as a potential computing technology that reformed CALL through its dynamics in multiple aspects (Segler et al. 2002; Esit 2011; Amaral and Meurers 2011). However, not all AI-based tools are directly associated with ICALL, they are diversified into multiple forms and tools as follows; Adaptive Educational System (Triantafillou et al. 2003), Intelligent Educational System (Cumming et al. 1993) Intelligent Personal Assistant (Rahman and Tomy 2023; Yang et al. 2022) Intelligent Tutoring System (Slavuj et al. 2015), Natural language processing (Nagata 2013), Machine translation tools (Briggs 2018), Chatbots (Jeon 2021; Dokukina and Gumanova 2020), AI writing assistants (Gayed et al. 2022; Godwin-Jones 2022), AI-powered language learning software (Pokrivčáková 2019) and Intelligent Virtual Reality (Ma 2021). These AI-powered language learning tools are used to foster students’ language skills and sub-skills, encourage students’ interaction, reduce the affective factors of language learning and acquisition, push their willingness to communicate, and more (Tai and Chen 2020; Shazly 2021; Liang et al. 2021). Acknowledging its efficient performance in multiple studies, researchers and applied linguists are paying attention to AI-empowered tools and their applications.
2.3 Previous reviews on AI in language learning and education
Like given in Table 2, previous review papers have analyzed the trends and research foci of artificial intelligence and AI-powered tools in language learning and education. Review papers have focused on specified AI-based tools like Intelligent tutoring systems, Voice based virtual agents and speech recognition chatbots (Xu et al. 2019; Katsarou et al. 2023; Jeon et al. 2023) and have worked on reviewing the integration of intelligent tools such as ChatGPT and conversational AI, and approaches like ICALL in language learning (Kohnke et al. 2023; Weng and Chiu 2023; Ji et al. 2022). Likewise, researchers have reviewed the role of AI in language learning and language education(Huang et al. 2021; Liang et al. 2021; Fang et al. 2023; Ali 2020; Sharadgah and Sa’di 2022; Yang et al. 2022). Although studies have focused on AI and its applications, reviews on AI, in many cases, have focused either on “Artificial Intelligence” or on other applications such as “Intelligent Tutoring Systems” but not on both. Studies analyzing both aspects are notably limited. Thus, this paper aims to perform an analysis of both aspects which includes AI and AI-powered tools and approaches. Additionally, following the introduction of advanced NLP models in 2022, there has been a notable absence of dedicated reviews concerning the role of AI in language learning. Thus, this study aims to analyze the trends and impact of AI in language learning up to the year 2023. This includes the most advanced ChatGPT and other NLP-powered intelligent agents. Along with bibliometric analysis, through content analysis we also investigated its connectives with language learning factors and the target learners through the mode of instruction.
3 Methodology
3.1 Defining aims and scope
It is essential to set clear objectives and parameters before moving on to the process of bibliometric analysis Belmonte et al. (2020); Donthu et al. (2021). The authors sought to analyze the conceptual framework and reflect on influential research contributors and their collaboration in the research area. Any area of research with more than 500 papers “deserves a bibliometric analysis” (Hou and Yu 2023; Donthu et al. 2021). The scope of the study, in accordance with the regular standards, will analyze more than 500 papers. Along with bibliometric analysis, a content analysis will be performed to identify the key applications and their participants in the research area.
3.2 Data source
The authors opted for the Scopus database to carry out the bibliometric and content analysis, in line with other prior bibliometric research (Thayyib et al. 2023; Ahmed et al. 2022; Goodell et al. 2021). Scopus is one of the largest databases of scholarly works with more than 84 million records and 1.8 billion cited references (Home https://hai.stanford.edu/). With its advanced search capabilities and wide coverage, it holds the records of research works being published even in developing countries. It offers adequate bibliometric details such as citation information, bibliographic information, abstracts, keywords, funding details, and other information including references. In addition, Scopus provides these data in multiple formats to feed into software that is used to systematically analyze documents.
3.3 Data collection and refinement
The authors retrieved the data used for analysis from the Scopus database on June 22, 2023. The period range was limited between the years of 2017 and 2023 to obtain scholarly works that mostly reflect advanced AI techniques in language learning. With reference to previous relevant bibliometric and systematic reviews, the search keywords were finalized (Hou and Yu 2023; Liang et al. 2021; Popenici and Kerr 2017; Chu et al. 2022; Jeon et al. 2023; Tan et al. 2022). To further extend the scope of the study, ChatGPT was also included. However, we excluded “machine learning”, “deep learning” and “deep neural networks”. While undoubtedly, these components are important in the broader field of AI, these terms tend to yield a substantial number of papers related to computer language learning and programming languages, which are distinct from our primary focus on second language learning.
The chosen keywords were influenced by the prominent pedagogical viewpoint within second language learning. NLP techniques, conversational systems, and interactive chatbots are frequently employed in this context to facilitate meaningful interactions between the AI and the learner. Considering these factors, the following keywords were used to search relevant articles in the database ( TITLE-ABS-KEY ( "Chatbot*" OR "conversational agent" OR "pedagogical agent" OR "conversational system" OR "dialogue system" OR "spoken dialogue system" OR "intelligent personal assistant" OR "ICALL" OR "intelligent computer assisted language learning" OR "artificial intelligence" OR "intelligent tutoring system" OR "ChatGPT" OR "ChatGPT-4" OR "natural language processing" OR "NLP" ) AND TITLE-ABS-KEY ( "Language learning" OR "language teaching" OR "language acquisition" OR "second language learning" OR "foreign language learning" ) ). A total of 1870 results were obtained from the keyword search. The publication selection procedure is given in Fig. 1 Page et al. (2021), and the inclusion and exclusion criteria are in Table 3. On the exclusion of articles based on the criteria, only 606 documents were processed for bibliometric and content analysis.
3.4 Technical tools and procedure of data analysis
Bibliometric analysis and content analysis were performed to gain an overall understanding of the research on AI in language learning. The study employed bibliometric analysis to identify the publication trends, leading authors, institutions, prominent journals, collaboration patterns, citation analysis, geographic distribution, keywords co-occurrence analysis, co-authorship analysis, and co-citation analysis. The authors used VOSviewer, Publish or Perish software, and Scopus to visualize and extract results from the retrieved data with the objective to carry out bibliometric analysis.
The authors, through content analysis, opted to identify the overview of the types of AI tools used in language learning, the language skills it is being tested against, and the educational levels of the participants of the study. After identifying the keywords through text-based content analysis on VOSviewer, a conceptual content analysis was performed, adhering to the deductive coding approach following the structural coding method through code categorization (Krippendorff 2018). The coding scheme for content analysis was done with NVivo, which assists in “classifying, sorting and modelling qualitative data” (Bazeley 2019). The schemes of the documents were initially classified through auto-coding in NVivo. In addition, manual classification was done independently by two research scholars to examine the auto-coded results. The auto-coding was fed into Atlas AI to identify the connections between the variables of the study.
4 Findings
4.1 Publication trends and performance analysis of AI in language learning
The screened data had 606 documents published between the years 2017 and 2023. It included 230 research articles, 29 book chapters, 330 conference papers, and 17 reviews. Among these documents, 39 were articles-in-press. Most of these documents were closed access, only 185 articles were open access among which 117 were research articles, 61 were conference papers, and 7 were reviews. However, along with the increase in the total number of documents published over time, open-access documents got doubled between 2017 and 2023. As shown in Fig. 2, there is a noticeable growth in the total number of documents published from 2017 to 2023 in the subject area. From 2017 to 2022, there has been a gradual increase in the number of documents that were published, indicating a growth of 189.8 \(\%\). The data presented for 2023 is not complete as it was collected during the middle of the year (June 22, 2023). However, the reported number of 72 documents signifies promising growth. While the number of research publications has increased, the number of citations has decreased over time as presented in Fig. 2.
“Performance analysis examines the contribution of research constituents to a given field” (Donthu et al. 2021). With total publication and total citation details, the analysis has other metrics to be evaluated including ‘scientific actors’ like h-index and i-index (Cobo et al. 2011). Table 4 indicates the overall performance of AI in language learning through selected metrics from Donthu et al. (2021).
Donthu et al. (2021) states that it is a “standard practice” to present the background or profile of the retrieved documents. Thus, the study further elaborates on the contributions of the (1) authors, (2) institutions, (3) sources, and (4) countries that are highly influential in the field of AI in language learning. The following formulas were used to calculate the metrics identified in Table 4: PAY = (TP \(\div\) NAY), ACP = TC \(\div\) TP, PCP = (NCP / TP) * 100, and CCP = (TC \(\div\) NCP). NCA was calculated by identifying (The total number of authors - Duplicates) in Microsoft Excel. NAY is the total number of years that the research constituent records the publications and NCP was identified by filtering the publications with citation in Microsoft Excel. The h-index and I- Index were calculated through Publish or Perish software by the RIS format derived from Scopus (Table 4).
4.2 Top authors, sources, countries, and institutions
We used VOSviewer and R studio (Biblioshiny) to identify and cross-validate individual influential authors through co-citation analysis. As given in Table 5, the authors identified are Meurers d.; Fryer I.K.; Hwang g.j.; Dizon. G.; Chen x.; Zou d.; Strik. H; Cucchiarini c.; Thompson A.; and Xie h. In Table 6, the top ten cited sources with a minimum of 5 documents in the field are listed. The sources are Computer Assisted Language Learning, Interactive Learning Environments, Lecture Notes in Computer Science (including subseries Lecture Notes in Artificial Intelligence and Lecture Notes in Bioinformatics), Educational Technology and Society, Procedia Computer Science, ACM International Conference Proceeding Series, Journal of Physics: Conference Series, Proceedings of the 13th Workshop on Innovative Use of NLP for Building Educational Applications, BEA 2018 at the 2018 Conference of the North American Chapter of the Association for Computational Linguistics: Human Language Technologies, NAACL-HTL 2018, International Journal of Emerging Technologies in Learning, Applied Sciences (Switzerland). The document type of sources are Journals, Book series, and Conference proceedings. Table 7 includes documents and citations of the top ten organizations with high impact in the field of AI in language learning between the years 2017 and 2023. The University of Sydney, Himeji Dokkyō University, National Taiwan Normal University, University of Piraeus, Georgia State University, National Taiwan University of Science and Technology, University of Tübingen, The Education University of Hong Kong, Lingnan University and the University of Cambridge are the most influential organisations.
Figure 3 presents the top ten influential countries in the field along with their total number of documents produced and citations achieved. We found out that the United States, Japan, China, Hong Kong, Taiwan, Canada, Germany, Australia, the United Kingdom, and Indonesia are the most productive and influential countries. VOSviewer was used to identify these countries by evaluating the citations against countries. The minimum number of documents per country was set to 10 to identify countries that are both productive and influential.
4.3 Keywords analysis
The co-occurrences of keywords analysis was made through VOSviewer to identify the keywords that authors use frequently. With full counting, the co-occurrence of the author keywords has opted with keyword occurrences of \(>5\). Of the 3252 keywords, only 184 (5.66\(\%\)) met the threshold with 4069 links and a total link strength of 10134. The overall strength of the co-occurrence connections between each of the 184 keywords was calculated. By doing so, Fig. 4 was generated.
The 184 keywords were classified into seven clusters. Table 8 details the seven clusters. Cluster 1 consists of 49 items, like language learning (f = 193, Total link strength = 1026), Natural language processing systems (f = 132, Total link strength = 817), Computational linguistics (f = 46, Total link strength = 262), Second language acquisition (f = 44, Total link strength = 207), and Deep Learning (f = 32, Total link strength = 231). Cluster 2 comprised 43 items, including Artificial Intelligence (f = 211, Total link strength = 1051), Students (f = 112, Total link strength = 784), Teaching (f = 94, Total link strength = 664), Engineering Education (f = 49, Total link strength = 397), and Education computing (f = 39, Total link strength = 317). Cluster 3 has 43 items and the most occurred keywords in this cluster are E-learning (f = 78, Total link strength = 556), linguistics (f = 39, Total link strength = 283), Education (f = 27, Total link strength = 175), Chatbot (f = 26, Total link strength = 92), and English languages (f = 24, Total link strength = 183). Cluster 4 consists of 16 items, including Learning systems (f = 125, Total link strength = 896), Foreign language (f = 36, Total link strength = 238), Computer Assisted Language Learning (f = 28, Total link strength = 195), Intelligent computer-assisted language learning (f = 16, Total link strength = 61), and Error correction (f = 10, Total link strength = 62). 14 items were found in cluster 5, like Computer aided instruction (f = 109, Total link strength = 824), Intelligent tutoring systems (f = 25, Total link strength = 178), Foreign language learning (f = 24, Total link strength = 142), Educational technology (f = 13, Total link strength = 79), and Tutoring system (f = 10, Total link strength = 85). Cluster 6 comprises 11 items including Speech recognition (f = 24, Total link strength = 181), Automatic speech recognition (f = 8, Total link strength = 70), Machine translations (f = 9, Total link strength = 62), Computer-aided language translation (f = 7, Total link strength = 57), and Deep neural networks (f = 7, Total link strength = 48). In Cluster 7, 8 items were found including Teacher (f = 27, Total link strength = 212), Learning platform (f = 8, Total link strength = 48), Decision making (f = 6, Total link strength = 44), Statistical tests (f = 5, Total link strength = 35), and online learning (f = 5, Total link strength = 32).
4.4 Bibliographic coupling
Bibliographic coupling was done to identify literature that is connected through a common document’s reference. The network visualization of the documents as illustrated in Fig. 5 details the interconnections between the documents through 5 clusters. To perform this visualisation, full counting was opted with the unit of analysis as Bibliometric coupling with documents. In order to narrow down the influential works, the minimum number of citations per document was set to 20. Of the 606 documents, only 40 met the threshold. Among the filtered 40 documents, only 21 items in the network had the largest set of connected items.The most influential authors of AI in language learning are listed in Table 9.
The most influential documents identified through Bibliometric coupling are “Stimulating and sustaining interest in a Language Course: An experimental comparison of Chatbot and Human task partners” (Fryer et al. 2017), “Technology and the Future of language teaching” (Kessler 2018), “Chatbot learning partners: Connecting learning experiences, interest, and competence” (Fryer et al. 2019), and “Using Intelligent Personal Assistants for Second Language Learning: A Case Study of Alexa” Dizon (2017), and “Chatbots for language learning-Are they really useful? A systematic review of chatbot-supported language learning” (Huang et al. 2021).
4.5 Content analysis
The title and the abstract fields of all the documents were fed into VOSviewer to trace out the most occurred words. With a minimum number of occurrences per term set to 10, we identified 382 terms. For each of the 382 items, a relevance score was calculated by default in VOSviewer, and only 60\(\%\) of the most relevant terms were opted for further analysis. Upon filtration, we created a map based on textual data with 299 items under 6 clusters. Among these 299 items from VOSviewer, we excluded terms that do not add up to any contextual meaning such as research gap, participants, perception, English language teaching, methodology, experiment results, observation, sample, control group, experimental group, the current study, post-test, and questionnaire. A total number of 254 were excluded on such pretest. A total number of 45 terms were manually coded through NVivo 14 into three different schemes or parent codes namely (1) Artificial Intelligence Tools and Techniques (2) Participants (3) Language Learning Factors.
AI tools and techniques gave us an overview on the type of AI-based technology that is implemented in the studies, the participants’ parent code had the different age groups of learners upon whom the experiments had been conducted, and the Language Learning Factors parents code had the factors with which the AI techniques were tested against. Figure 6 illustrates the 45 items under three different clusters. The child codes are organized under parent codes. The terms used most frequently under AI tools and techniques are as follows with their occurrence in references: AI Chatbots (f = 521), ChatGPT (f = 22), Conversational Agent (f = 217), Automatic Speech Recognition (f = 216), Intelligent Personal Assistants (f = 216), Google Assistant (f = 46), Amazon Alexa (f = 24), Agent (f = 209), Virtual Reality (f = 171), Natural Language Processing (f = 170), CALL (f = 152), ICALL (f = 79), Machine Translation (f = 134), Web (f = 108), Application (f = 94), Intelligent Tutoring System (f = 60), Robot (f = 45), Error Correction (f = 43), Gengobot (f = 40), MALL (f = 39), Mobile Devices (f = 15), Mobile Learning (f = 12), Mobile Applications (f = 8), ICT (f = 29), and Gamification (f = 28). The terms most frequently used under the parent code Language Learning Factors are Writing (f = 184), Speaking (f = 153), Vocabulary (f = 148), Listening (f = 106), Grammar (f = 92), Proficiency (f = 81), Accuracy (f = 79), Pronunciation (f = 71), Fluency (f = 66), Motivation (f = 64), Sentence pattern (f = 61), Comprehension (f = 57), Anxiety (f = 32), and Formulaic sequence (f = 22). Third, the terms most frequently used under the parent code of participants are University students (f = 116), Children (f = 100), College students (f = 85), Language teachers (f = 65), Higher education (f = 63), and School students (f = 18).
Then, a code-occurrence analysis was conducted to present a two-field plot to depict the relationship between the participants and the AI tools and techniques used and between language learning factors and AI tools and techniques. In order to do that, we fed the parent and child codes into ATLAS AI to generate the visualizations in Fig. 7 and to identify the link strength between the parent and its sub-codes. The plot showcases the co-occurrence patterns between the two variables. The dense clusters illustrated in Fig. 7b indicate strong link strength such as the link between Teachers and chatbots and between chatbots and grammar in Fig. 7a. Though it depicts the association between the tested variables, not all the variables that were fed were displayed due to their weak association such as ChatGPT in Fig. 7a and formulaic sequences in Fig. 7b.
5 Discussion
RQ1: What are the publication trends and metrics of performance analysis such as Publication, Citation, and both Citation and Publication-related metrics?
RQ1 is devoted to identifying the research trend of AI in language learning. The annual total publication and citation records could provide an overview of the future of the research area and its potential. The findings of publication trends given in Fig. 2 depict a gradual growth in terms of production (publication) till 2022. As the data was collected on June 22, 2023, the production rate is still incomplete. However, a promising amount of literature has been produced within the first half of the year. In contrast to the rising publication rates, a decrease in citation records could be observed. The decrease in citations may have been attributed to the research focus shift. Over time, researchers have been exploring new AI-based technologies that have not gained much attention. Researchers have shifted focus from generic terms such as ”Artificial Intelligence” to specific tools such as IPA and ChatGPT. In both cases, the need for researchers to cite other specific applications and tools is low. The plausible reason for the reduction in citations could be the saturation of the field. The growth may have reached a point where new papers are not cited as frequently as old papers which are considered to be foundational works. We further analyzed various other metrics of publication and citation to gain insights into AI in LL. The findings shed light on productivity, its impact, and the rate of collaboration in the field. With the total number of included publications, sole-authored publications, and co-authored publications, we evaluated the level of collaboration among the researchers. The results revealed that the ( CI = 0.76) indicated a strong culture of collaboration among the researchers. The productivity of AI in language learning (TP = 606; PAY = 87) is on par with other renowned bibliometric or systematic reviews on pedagogic techniques in language learning like Virtual tools with (TP = 104), Mobile assisted vocabulary learning with (TP = 687), synchronous computer-mediated communication with (TP = 1292), and Augmented Reality with (TP = 1275) despite excluding documents published before 2017 (Botero-Gomez et al. 2023; Daǧdeler 2023; Hou and Yu 2023; Min and Yu 2023).
The research output of AI in LL has received a total of 3194 citations, with an average of 5.27 citations per document and an ACY of 456.28, indicating that the academic community has a positive reception of the produced documents. Other citation metrics such as CCP and PCP evaluate the amount and the impact of the influential works of the field. Notably, more than 60\(\%\) of the documents had citation records with an average CCP of 8.72, suggesting a high percentage of influential papers in the field. We further examined the research impact indices to reflect the overall impact of authors in the field. The (h-index = 27, G = index = 41, and I10 index = 85) for our dataset suggests that the scholars in our field have had a significant impact.
RQ2: Who or Which are the most influential and productive authors, institutions, journals, and countries?
The second research question aims at identifying the top authors, institutions, countries, and journals. The results of the analysis provided valuable insights into the contributors to the field. Our study revealed prolific authors who have made a major contribution to the field of AI in LL. The identified authors have contributed 30 documents altogether with a strong collaboration pattern between each other and other authors of the field. Only 10 \(\%\) of the 30 documents were sole-authored publications, and 90\(\%\) being collaborative contributions. Moreover, it was found that 30\(\%\) of the documents produced by these authors demonstrated collaboration among themselves.
The authors focused mostly on discussing the general trends and problems surrounding AI (Huang et al. 2023; Chen et al. 2021; Liang et al. 2021) and its tools such as Chatbots (Zhang et al. 2023; Huang et al. 2021; Fryer et al. 2019), ChatGPT (Kohnke et al. 2023), IPAs like Alexa and Google Assistant (Dizon et al. 2022; Dizon 2021), ICALL (Chen et al. 2022; Ruiz et al. 2019), Grammarly (Dizon 2021), Natural Language Processing (Ziegler et al. 2017), Speech (Litman et al. 2018) and digital technologies (Liu et al. 2023; Kienberger et al. 2022). While influential authors play an indelible mark in any field, equally remarkable is the role of academic sources who support the research fields. In the field of AI in LL, the most influential sources are journals (5) followed by conference proceedings (4) and book series (1). The journals have produced (\({\bar{x}}\) = 8.6, SD = 3.36, Min = 5, Max = 13) documents with the impact measured through citation of (\({\bar{x}}\) = 93.2, SD = 50.74, Min = 30, Max = 166) between 2017 and 2023. The renowned journals of the field publish articles about AI in LL under the categories of language and linguistics, computer science applications, and education which indicates that the research field is multidisciplinary and not bound to any particular school of thought.
In accordance with the knowledge gained from influential journals, organizations that publish influential works include not only the Department of English Language Education but also interdisciplinary departments such as Institute of Technology, Institutes of Digital Learning and Education, Department of Mathematics and Information Technology, Department of informatics, Computer Science Department, Institutes of Automated Language Teaching and Assessment, and Institute and Department of Computer Science and Technology. Thus, maintaining a highly multidisciplinary approach in the field. On the other hand, when we looked at the most productive and impactful countries in the field, we found that the majority of 156 documents on AI came from China, and the United States has got the highest number of citations of 778 with 78 documents. The results of our study are consistent with other bibliometric studies that link AI with other sectors, such as Big Data Analytics (Thayyib et al. 2023), Food Safety (Liu et al. 2023), and Smart Buildings (Luo 2022), although a similar study on the role of AI in language education claimed that Taiwan, the US, and the United Kingdom had secluded the top most spots. The prior analysis by Liang et al. (2021) examined documents between 1889 and 2020, whereas our study looked at documents published between 2017 and 2023, which may have led to a difference in our results. Thus, the overall analysis of RQ2 provides insights into the most influential authors, institutions, sources, and countries which can guide researchers to understand the factors that contribute to their success.
RQ3: What are the key research themes, frequent and prominent keywords obtained from title, abstract, and keywords through keyword analysis?
RQ3 illustrated extensively used keywords of AI in LL. The authors merely listed the keywords that were automatically retrieved and clustered by VOSviewer. The list contains highly occurred keywords with strong TLS and occurrences. Results reveal that “Natural language processing systems” is the most occurred technical keyword apart from “Artificial Intelligence”. However, contextual meaning or research inferences could not be obtained through the use of NLP in language learning as most AI-based systems used in language learning and acquisition platforms uses tools that are incorporated with NLP (Meurers 2012; Zilio et al. 2017). But hints for future studies could be obtained through keywords that have lower TLS and occurrences. The identified keywords with weaker connections could be focused by the researchers if found to be potential areas of research.
RQ4: What are the documents and clusters that are connected to a common document’s reference through bibliographic coupling?
In RQ4, documents that were often cited by other authors of the same field were identified through bibliographic coupling. The top documents identified through bibliometric coupling were published in the year 2017 followed by 2018 and 2020. According to Dogan et al. (2023), a significant amount of literature was produced on AI in education in 2018. Our study, which aligns closely with Dogan’s findings, also observed a similar pattern, with a high number of influential works published in 2017, followed by another peak in 2018. The use of AI, Chatbots, and Alexa are discussed in most documents (Fryer et al. 2017; Dizon 2017; Huang et al. 2021). These documents are seen, in most cases, to be foundational works, which could be the cause of the declining citation patterns as discussed in RQ1. The inferences obtained through bibliometric coupling identify the key papers and shed light on the research landscape.
RQ5: what are the inferences obtained by analyzing the content of all the documents in the study through content analysis?
RQ5 aimed at quantitatively contextualizing the content by coding the documents into clusters and therefore deducing inferences. Figure 8 illustrates the types of AI used in language learning, the language learning factors, and its participants based on hierarchy compared by a number of coding references. According to the model given, a large number of studies have been conducted with AI-embedded Chatbots. In line with the aforementioned statement, Jeon et al. (2023) conducted a systematic review of chatbots acknowledging their widespread application. Like Chatbots, other AI tools like CALL, Conversational agents, Virtual Reality, NLP tools, and IPAs are prevalent in the field. On the other hand, writing is the language learning factor that is mostly preferred with AI applications in language studies followed by speaking, vocabulary, proficiency, accuracy, pronunciation, listening, and fluency.
The participants that are most sought after for implying AI are university students followed by children, college students, language teachers, and students of higher education. In addition to figuring out the dominant components within the variables, we examined the interconnections among them to establish previously established research areas and research gaps. Chatbot was experimented extensively with teachers, children, and university students. Figure 7b depicts the relationship between AI tools and the participants. Even though a high amount of interconnections could be observed with different language learning factors and AI tools, the interconnections between AI tools and the levels of participants are weak. Future studies could work on experimenting with AI tools with different participant levels. Despite the fact that there is a lot of literature on writing skills, many AI tools have been tested with speaking skills. Researchers could contribute to the field by working on weaker connections. For instance, students in colleges, universities, and schools might be exposed to different AI tools. The same could be done for fluency and anxiety, which are core areas of research with weaker connectives.
6 Conclusion
This study used bibliometric and content analysis to analyze the research trends, patterns, key contributors and content in the field of AI in LL. It summarises the bibliometric information of the field along with prominent authors, institutions, sources, and countries. A rise in publication trends has been identified. Researchers who integrate AI into language learning use a variety of tools, leading to the formation of new fields within the field and new branches within AI-based language learning. This, in turn, is speculated to be a major reason for the decline in citation trends. However, the constructive viewpoint regarding this aspect is that the researchers, utilizing diverse AI-based tools, are expected to contribute significantly to the field. Affirming this, documents that were published during 2017 and 2018 are identified, through bibliographic coupling, to be ‘often cited’ papers indicating their mark as foundational works. On analysing the bibliographic and textual data on multiple aspects, we yielded the following results:
-
1.
Between 2017 and 2022, there is a considerable increase in the number of publications on AI in language learning of 189.8\(\%\) demonstrating a promising growth in the field with 60.3\(\%\) of the documents with citations of (\(\ge 1\)).
-
2.
The field exhibits a significant number of co-authored publications, totalling to 466, in contrast to the relatively lower count of sole-authored publications, which stands at only 140.
-
3.
As the field is emerging, a lot of new tools and technologies are being incorporated into the field. Resulting in a high number of citations for the works published in 2017 and 2018. The articles published during this period are often cited and considered as foundational works.
-
4.
Our findings have identified the United States, China, and Japan, sequentially, as the most influential countries in publishing research related to AI in language learning.
-
5.
On analysing the author’s keywords, we identified that there is an upsurge in the following in areas of study in connection with AI in language learning: natural language processing, computational linguistics, deep learning, speech recognition, machine translation, and deep neural networks. Among these keywords, “Natural language processing” is the most used keyword indicating the presence of Large Language Models of AI being frequently opted in language studies.
-
6.
Fryer et al. (2017) and Kessler (2018), which examine the usage of chatbots in language learning and the impact of technology including AI in language learning, consequently, were shown to be the most influential texts. The first document talks about the usage of Chatbots in language learning setup, and the second document discusses the extensive use of technology in language learning. This conclusion, through bibliographic coupling, is consistent with the outcomes of the content analysis.
-
7.
Through content analysis, we identified the most occurring textual terms used in the retrieved data from Scopus. We identified that the most occurring AI tool was Chatbots followed by Chatgpt, Conversational Agents, Automatic Speech Recognition and Intelligent Personal Assistants. We also identified the most researched language learning factor with AI which is Writing followed by Speaking, Vocabulary, Listening and Grammar. The most targeted participants are University Students followed by children, college students and language teachers.
6.1 Implications and contributions
In light of the rapid pace of technological advancements, several reviews are limited to incorporating the latest NLP tools, such as ChatGPT and Intelligent Personal Assistants, into their analyses. While prior bibliometric analyses have provided us with a comprehensive understanding of the bibliometric landscape concerning AI in language learning, the dynamic nature of AI necessitates an investigation inclusive of the recently launched tools, particularly in light of the technological developments emerging post-2019, with ChatGPT serving as a prime illustration thereof. Surprisingly, no prior bibliometric analysis has embraced these cutting-edge NLP tools and techniques. Furthermore, there exists a conspicuous absence of content analysis within the domain of AI in language learning. Hence, our study aims to bridge these critical gaps by providing a thorough examination of the wide range of tools and techniques utilized in AI for language learning, their respective frequencies, and the target participant groups they have been applied to. The outcomes of our research will enable future researchers to identify research gaps through content analysis by providing them with a comprehensive understanding of bibliometric information. The frequency of research among the three factors of content analysis will also serve as a vital resource for pinpointing areas that needs research attention.
6.2 Limitation of the study and recommendation for future studies
Through addressing the limitation of the study, we would want to suggest areas for further research. First, the study is limited to the Scopus database and between the years 2017 and 2023. Despite Scopus being an academically promising database for language studies, documents published on the Web of Science, ERIC, ScienceDirect and Google Scholar could be paid due attention to extend the coverage. The conclusive decision of both the databases shall reflect well the research field. Thus, the findings of the bibliometric and content analysis are limited to the Scopus database. Though some documents are indexed in more than one database, the inclusion of any of the databases could alter the findings of the bibliometric findings. Second, Even though we included most AI tools and techniques including ChatGPT and IPAs, not every tool was included in this study. keywords refinement can be done to identify more papers addressing other AI tools in the field. For instance, keywords identified through this study such as “Deep learning”, “machine learning”, “deep neural network”, “Machine translation” and “computer-aided instruction” could be included in future studies to extend the scope of the field. This study acknowledges the assumption that the identified keywords and content categories faithfully reflect the diversity and intricacies of AI tools employed in language learning. However, it is important to recognise that this approach may inadvertently overlook emerging trends or unconventional terminologies within this rapidly evolving field. Future research endeavors should remain attuned to these evolving nuances in the realm of AI tools for language learning. A different approach to review shall also be considered. This study has applied quantitative analysis to examine the research scope, similarly, studies could opt for qualitative analysis to extract valuable insights. Systematic reviews can be done on other prominent AI tools identified through content analysis. Given these limitations, the findings of the study can be beneficial for researchers in the field of AI in LL, since the study outlines both the research focus and the research gaps.
References
Ahmed S, Alshater MM, Ammari AE, Hammami H (2022) Artificial intelligence and machine learning in finance: a bibliometric review. Res Int Bus Financ 61:101646. https://doi.org/10.1016/j.ribaf.2022.101646
Ali Z (2020) Artificial intelligence (AI): a review of its uses in language teaching and learning. IOP Conf Ser 769(1):012043. https://doi.org/10.1088/1757-899x/769/1/012043
Amaral L, Meurers D (2011) On using intelligent computer-assisted language learning in real-life foreign language teaching and learning. ReCALL 23(1):4–24. https://doi.org/10.1017/s0958344010000261
Bazeley KJP (2019) Qualitative data analysis with NVivo. CiNii Books
Belmonte JL, Moreno-Guerrero A-J, Núñez JAL, Lucena FJH (2020) Augmented reality in education. A scientific mapping in web of science. Interact Learn Environ 31(4):1860–1874. https://doi.org/10.1080/10494820.2020.1859546
Botero-Gomez V, Ruiz-Herrera LG, Arias AV, Díaz AR, Garnique JCV (2023) Use of virtual tools in teaching-learning processes: advancements and future direction. Soc Sci 12(2):70. https://doi.org/10.3390/socsci12020070
Briggs N (2018) Neural machine translation tools in the language learning classroom: students’ use, perceptions, and analyses. JALT CALL J 14(1):3–24. https://doi.org/10.29140/jaltcall.v14n1.221
Chen L, Chen P, Lin Z (2020) Artificial intelligence in education: a review. IEEE Access 8:75264–75278. https://doi.org/10.1109/access.2020.2988510
Chen X, Zou D, Cheng G, Xie H (2021) Artificial intelligence-assisted personalized language learning: systematic review and co-citation analysis. In: 2021 international conference on advanced learning technologies (ICALT), pp. 241–245 . IEEE
Chen X, Bear E, Hui B, Santhi-Ponnusamy H, Meurers D (2022) Education theories and AI affordances: Design and implementation of an intelligent computer assisted language learning system. In: Artificial intelligence in education. posters and late breaking results, workshops and tutorials, industry and innovation tracks, practitioners’ and doctoral consortium, pp 582–585. Springer
Chu H-C, Hwang G-H, Tu Y-F, Yang K-H (2022) Roles and research trends of artificial intelligence in higher education: a systematic review of the top 50 most-cited articles. Australas J Educ Technol 38(3):22–42
Cobo MJ, López-Herrera AG, Liu X, Herrera F (2011) An approach for detecting, quantifying, and visualizing the evolution of a research field: a practical application to the fuzzy sets theory field. J Inf 5(1):146–166. https://doi.org/10.1016/j.joi.2010.10.002
Cumming G, Sussex R, Cropp S (1993) Learning english as a second language: Towards the “mayday’’ intelligent educational system. Comput Educ. https://doi.org/10.1016/0360-1315(93)90078-w
Daǧdeler KO (2023) A systematic review of mobile-assisted vocabulary learning research. Smart Learn Environ. https://doi.org/10.1186/s40561-023-00235-z
Dizon G (2017) Using intelligent personal assistants for second language learning: a case study of Alexa. TESOL J 8(4):811–830. https://doi.org/10.1002/tesj.353
Dizon G (2021) Affordances and constraints of intelligent personal assistants for second-language learning. RELC J. https://doi.org/10.1177/00336882211020548
Dizon G, Tang D, Yamamoto Y (2022) A case study of using Alexa for out-of-class, self-directed Japanese language learning. Comput Educ Artif Intell 3:100088. https://doi.org/10.1016/j.caeai.2022.100088
Dogan ME, Dogan TG, Bozkurt A (2023) The use of artificial intelligence (AI) in online learning and distance education processes: a systematic review of empirical studies. Appl Sci 13(5):3056. https://doi.org/10.3390/app13053056
Dokukina I, Gumanova J (2020) The rise of chatbots-new personal assistants in foreign language learning. Proc Comput Sci 169:542–546. https://doi.org/10.1016/j.procs.2020.02.212
Donthu N, Kumar S, Mukherjee D, Pandey N, Lim WM (2021) How to conduct a bibliometric analysis: an overview and guidelines. J Bus Res 133:285–296. https://doi.org/10.1016/j.jbusres.2021.04.070
Esit O (2011) Your verbal zone: an intelligent computer-assisted language learning program in support of Turkish learners’ vocabulary learning. Comput Assist Lang Learn 24(3):211–232. https://doi.org/10.1080/09588221.2010.538702
Fang X, Ng DTK, Leung JKL, Chu S (2023) A systematic review of artificial intelligence technologies used for story writing. Educ Inf Technol. https://doi.org/10.1007/s10639-023-11741-5
Farrokhnia M, Banihashem SK, Noroozi O, Wals AEJ (2023) A SWOT analysis of ChatGPT: implications for educational practice and research. Innov Educ Teach Int. https://doi.org/10.1080/14703297.2023.2195846
Fryer LK, Ainley M, Thompson A, Gibson A, Sherlock Z (2017) Stimulating and sustaining interest in a language course: an experimental comparison of Chatbot and human task partners. Comput Hum Behav 75:461–468. https://doi.org/10.1016/j.chb.2017.05.045
Fryer LK, Nakao K, Thompson A (2019) Chatbot learning partners: connecting learning experiences, interest and competence. Comput Hum Behav 93:279–289. https://doi.org/10.1016/j.chb.2018.12.023
Gayed JM, Carlon MKJ, Oriola AM, Cross JS (2022) Exploring an AI-based writing assistant’s impact on english language learners. Comput Educ Artif Intell 3:100055. https://doi.org/10.1016/j.caeai.2022.100055
Godwin-Jones R (2022) Partnering with AI: intelligent writing assistance and instructed language learning. Lang Learn Technol 26(2):5–24. https://doi.org/10125/73474
Goodell JW, Kumar S, Lim WM, Pattnaik D (2021) Artificial intelligence and machine learning in finance: identifying foundations, themes, and research clusters from bibliometric analysis. J Behav Exp Financ 32:100577. https://doi.org/10.1016/j.jbef.2021.100577
Gruetzemacher R (2022) The power of natural language processing. https://hbr.org/2022/04/the-power-of-natural-language-processing
Gugerty L (2006) Newell and Simon’s logic theorist: historical background and impact on cognitive modeling. Proc Human Fact Ergon Soc Ann Meet 50(9):880–884. https://doi.org/10.1177/154193120605000904
Górriz JM, Ramírez J, Ortíz A, Martinez-Murcia FJ, Segovia F, Suckling J, Leming M, Zhang Y-D, Álvarez-Sánchez JR, Bologna G et al (2020) Artificial intelligence within the interplay between natural and artificial computation: advances in data science, trends and applications. Neurocomputing 410:237–270
Hariri W (2023) Unlocking the potential of chatgpt: A comprehensive exploration of its applications, advantages, limitations, and future directions in natural language processing. arXiv preprint arXiv:2304.02017
Hou Y, Yu Z (2023) A bibliometric analysis of synchronous computer-mediated communication in language learning using VOSViewer and CITNetExplorer. Educ Sci 13(2):125. https://doi.org/10.3390/educsci13020125
Huang W, Hew KF, Fryer LK (2021) Chatbots for language learning-Are they really useful? A systematic review of chatbot-supported language learning. J Comput Assist Learn 38(1):237–257. https://doi.org/10.1111/jcal.12610
Huang X, Zou D, Cheng G, Chen X, Xie H (2023) Trends, research issues and applications of artificial intelligence in language education. Educ Technol Soc 26(1):112–131
Jeon JH (2021) Chatbot-assisted dynamic assessment (CA-DA) for L2 vocabulary learning and diagnosis. Comput Assist Lang Learn. https://doi.org/10.1080/09588221.2021.1987272
Jeon JH, Lee S, Choi S (2023) A systematic review of research on speech-recognition chatbots for language learning: implications for future directions in the era of large language models. Interact Learn Environ. https://doi.org/10.1080/10494820.2023.2204343
Ji H, Han I, Ko Y (2022) A systematic review of conversational AI in language education: focusing on the collaboration with human teachers. J Res Technol Educ 55(1):48–63. https://doi.org/10.1080/15391523.2022.2142873
Katsarou E, Wild F, Sougari A-M, Chatzipanagiotou P (2023) A systematic review of voice-based intelligent virtual agents in EFL education. Int J Emerg Technol Learn (IJET) 18(10):65–85. https://doi.org/10.3991/ijet.v18i10.37723
Kessler G (2018) Technology and the future of language teaching. Foreign Lang Ann 51(1):205–218. https://doi.org/10.1111/flan.12318
Kienberger M, García-Holgado A, Schramm K, Raveling A, Meurers D, Labinska B, Koropatnitska T, Therón R (2022) Enhancing adaptive teaching of reading skills using digital technologies: the latill project. In: International conference on technological ecosystems for enhancing multiculturality, pp 1092–1098. Springer
Kohnke L, Moorhouse BL, Zou D (2023) ChatGPT for language teaching and learning. RELC J. https://doi.org/10.1177/00336882231162868
Krippendorff K (2018) Content analysis: an introduction to its methodology. Sage publications, California
Lee S, Jeon JH (2022) Visualizing a disembodied agent: young EFL learners’ perceptions of voice-controlled conversational agents as language partners. Comput Assist Lang Learn. https://doi.org/10.1080/09588221.2022.2067182
Liang J-C, Hwang G-J, Chen M-RA, Darmawansah D (2021) Roles and research foci of artificial intelligence in language education: an integrated bibliographic analysis and systematic review approach. Interact Learn Environ. https://doi.org/10.1080/10494820.2021.1958348
Lin MP-C, Chang D (2020) Enhancing post-secondary writers’ writing skills with a chatbot. J Educ Technol Soc 23(1):78–92
Litman D, Strik H, Lim GS (2018) Speech technologies and the assessment of second language speaking: approaches, challenges, and opportunities. Lang Assess Q 15(3):294–309. https://doi.org/10.1080/15434303.2018.1472265
Liu C, Liu S, Hwang G-J, Tu Y-F, Wang Y, Wang N (2023) Engaging EFL students’ critical thinking tendency and in-depth reflection in technology-based writing contexts: a peer assessment-incorporated automatic evaluation approach. Educ Inf Technol. https://doi.org/10.1007/s10639-023-11697-6
Liu Z, Wang S, Zhang Y, Feng Y, Li J, Zhu H (2023) Artificial intelligence in food safety: a decade review and bibliometric analysis. Foods 12(6):1242. https://doi.org/10.3390/foods12061242
Luo J (2022) A bibliometric review on artificial intelligence for smart buildings. Sustainability 14(16):10230. https://doi.org/10.3390/su141610230
Ma L (2021) An immersive context teaching method for college english based on artificial intelligence and machine learning in virtual reality technology. Mob Inf Syst 2021:1–7. https://doi.org/10.1155/2021/2637439
Meurers D (2012) Natural language processing and language learning. Encyc Appl Ling 4193–4205. https://www.sfs.uni-tuebingen.de/~dm/papers/meurers-13.pdf
Min W, Yu Z (2023) A bibliometric analysis of augmented reality in language learning. Sustainability 15(9):7235. https://doi.org/10.3390/su15097235
Nagata N (2013) An effective application of natural language processing in second language instruction. CALICO J 13(1):47–67. https://doi.org/10.1558/cj.v13i1.47-67
...Page MJ, McKenzie JE, Bossuyt PM, Boutron I, Hoffmann T, Mulrow CD, Shamseer L, Tetzlaff J, Akl EA, Brennan S, Chou R, Glanville J, Grimshaw J, Hróbjartsson A, Lalu MM, Li T, Loder E, Mayo-Wilson E, McDonald S, McGuinness LA, Stewart L, Thomas J, Tricco AC, Welch V, Whiting P, Moher D (2021) The PRISMA 2020 statement: an updated guideline for reporting systematic reviews. BMJ. https://doi.org/10.1136/bmj.n71
Peters ME, Ammar W, Bhagavatula C, Power R (2017) Semi-supervised sequence tagging with bidirectional language models. arXiv preprint arXiv:1705.00108
Pokrivčáková S (2019) Preparing teachers for the application of AI-powered technologies in foreign language education. J Lang Cult Educ 7(3):135–153. https://doi.org/10.2478/jolace-2019-0025
Popenici S, Kerr S (2017) Exploring the impact of artificial intelligence on teaching and learning in higher education. Res Pract Technol Enhanc Learn. https://doi.org/10.1186/s41039-017-0062-8
Rahman A, Tomy P (2023) Intelligent personal assistant–An interlocutor to mollify foreign language speaking anxiety. Interact Learn Environ. https://doi.org/10.1080/10494820.2023.2204324
Rahman A, Karthikeyan J, Tong C (2022) Appropriate Allocation of Specified NLP Tools for the Four Language Skills. Royal Book Publishing-International. https://doi.org/10.1080/10494820.2023.2204324
Ruiz S, Rebuschat P, Meurers D (2019) The effects of working memory and declarative memoryon instructed second language vocabulary learning: insights from intelligent CALL. Lang Teach Res 25(4):510–539. https://doi.org/10.1177/1362168819872859
Rusmiyanto R, Huriati N, Fitriani N, Tyas NK, Rofi’i A, Sari MN (2023) The role of artificial intelligence (AI) in developing english language learner’s communication skills. J Educ 6(1):750–757. https://doi.org/10.31004/joe.v6i1.2990
Russell S, Norvig P (2010) Artificial intelligence: a modern approach, 3rd edn. Prentice Hall, Hoboken
Segler TM, Pain H, Sorace A (2002) Second language vocabulary acquisition and learning strategies in ICALL environments. Comput Assist Lang Learn 15(4):409–422. https://doi.org/10.1076/call.15.4.409.8272
Seising R (2018) The emergence of fuzzy sets in the decade of the perceptron-Lotfi A. Zadeh’s and Frank Rosenblatt’s research work on pattern classification. Mathematics 6(7):110. https://doi.org/10.3390/math6070110
Shadiev R, Liu J (2022) Review of research on applications of speech recognition technology to assist language learning. ReCALL 35(1):74–88. https://doi.org/10.1017/s095834402200012x
Sharadgah TA, Sa’di RA (2022) A systematic review of research on the use of artificial intelligence in english language teaching and learning (2015–2021): what are the current effects? J Inf Technol Educ 21:337–377. https://doi.org/10.28945/4999
Shazly RE (2021) Effects of artificial intelligence on english speaking anxiety and speaking performance: a case study. Expert Syst. https://doi.org/10.1111/exsy.12667
Simonite T (2020) Baidu’s Artificial-Intelligence supercomputer beats Google at image recognition. MIT Technology Review. https://www.technologyreview.com/2015/05/13/168197/baidus-artificial-intelligence-supercomputer-beats-google-at-image-recognition/
Slavuj V, Kovačić B, Jugo I (2015) Intelligent tutoring systems for language learning. In: 38th international convention on information and communication technology, electronics and microelectronics (MIPRO). https://doi.org/10.1109/mipro.2015.7160383
Tai TY, Chen HH-J (2020) The impact of google assistant on adolescent EFL learners’ willingness to communicate. Interact Learn Environ. https://doi.org/10.1080/10494820.2020.1841801
Tan SC, Lee AVY, Lee M (2022) A systematic review of artificial intelligence techniques for collaborative learning over the past two decades. Comput Educ Artif Intell 3:100097. https://doi.org/10.1016/j.caeai.2022.100097
Thayyib PV, Mamilla R, Khan M, Fatima H, Asim M, Anwar I, Shamsudheen MK, Khan MA (2023) State-of-the-Art of artificial intelligence and big data analytics reviews in five different domains: a bibliometric summary. Sustainability 15(5):4026. https://doi.org/10.3390/su15054026
Triantafillou E, Pomportsis AS, Demetriadis S (2003) The design and the formative evaluation of an adaptive educational system based on cognitive styles. Comput Educ 41(1):87–103. https://doi.org/10.1016/s0360-1315(03)00031-9
Turing A (1950) I.-Computing machinery and intelligence. Mind LIX 236:433–460. https://doi.org/10.1093/mind/lix.236.433
Vaswani A, Shazeer N, Parmar N, Uszkoreit J, Jones L, Gomez AN, Kaiser L, Polosukhin I (2017) Attention is all you need. Adv Neural Inf Process Syst. 30:5998–6008
Verma S, Gustafsson A (2020) Investigating the emerging COVID-19 research trends in the field of business and management: a bibliometric analysis approach. J Bus Res 118:253–261. https://doi.org/10.1016/j.jbusres.2020.06.057
Weitzman T (2023) GPT-4 released: what it means for the future of your business. Forbes. https://www.forbes.com/sites/forbesbusinesscouncil/2023/03/28/gpt-4-released-what-it-means-for-the-future-of-your-business/?sh=1567d4312dc6
Weng X, Chiu TKF (2023) Instructional design and learning outcomes of intelligent computer assisted language learning: systematic review in the field. Comput Educ Artif Intell 4:100117. https://doi.org/10.1016/j.caeai.2022.100117
Wijekumar K, Meyer BJF, Lei PW (2013) High-fidelity implementation of web-based intelligent tutoring system improves fourth and fifth graders content area reading comprehension. Comput Educ 68:366–379. https://doi.org/10.1016/j.compedu.2013.05.021
Xu Z, Wijekumar K, Ramirez G, Hu X, Irey R (2019) The effectiveness of intelligent tutoring systems on K-12 students’ reading comprehension: a meta-analysis. Br J Edu Technol 50(6):3119–3137. https://doi.org/10.1111/bjet.12758
Yang CT-Y, Lai SL, Chen HH-J (2022) The impact of intelligent personal assistants on learners’ autonomous learning of second language listening and speaking. Interact Learn Environ 1:21. https://doi.org/10.1080/10494820.2022.2141266
Yang H, Kyun S (2022) The current research trend of artificial intelligence in language learning: a systematic empirical literature review from an activity theory perspective. Australasian J Edu Technol 180–210. https://doi.org/10.14742/ajet.7492
Zhang R, Zou D, Cheng G (2023) A review of chatbot-assisted learning: pedagogical approaches, implementations, factors leading to effectiveness, theories, and future directions. Interact Learn Environ. https://doi.org/10.1080/10494820.2023.2202704
Ziegler N, Meurers D, Rebuschat P, Ruiz S, Moreno-Vega JL, Chinkina M, Li W, Grey S (2017) Interdisciplinary research at the intersection of CALL, NLP, and SLA: methodological implications from an input enhancement project. Lang Learn 67(S1):209–231. https://doi.org/10.1111/lang.12227
Zilio L, Wilkens R, Fairon C (2017) Using nlp for enhancing second language acquisition. In: RANLP, pp 839–846
Funding
The study received no external funding.
Author information
Authors and Affiliations
Contributions
AR wrote the main manuscript made substantial contributions to the conception of the work. AR made substantial contributions to the conception, data analysis, and formatting of the work. PT drafted revised it critically for important intellectual content. MSH prepared drafted revised it critically for important intellectual content. All the authors reviewed the manuscript.
Corresponding author
Ethics declarations
Conflict of interest
The authors of this paper declare no conflicts of interest related to the collection, analysis, interpretation, or presentation of the findings.
Ethical approval
We have adhered to rigorous ethical standards throughout the research process. We obtained all data from the Scopus database, and we acknowledge Scopus for providing access to this resource. We have complied with the terms and conditions of data usage set forth by Scopus.
Additional information
Publisher's Note
Springer Nature remains neutral with regard to jurisdictional claims in published maps and institutional affiliations.
Rights and permissions
Open Access This article is licensed under a Creative Commons Attribution 4.0 International License, which permits use, sharing, adaptation, distribution and reproduction in any medium or format, as long as you give appropriate credit to the original author(s) and the source, provide a link to the Creative Commons licence, and indicate if changes were made. The images or other third party material in this article are included in the article's Creative Commons licence, unless indicated otherwise in a credit line to the material. If material is not included in the article's Creative Commons licence and your intended use is not permitted by statutory regulation or exceeds the permitted use, you will need to obtain permission directly from the copyright holder. To view a copy of this licence, visit http://creativecommons.org/licenses/by/4.0/.
About this article
Cite this article
Rahman, A., Raj, A., Tomy, P. et al. A comprehensive bibliometric and content analysis of artificial intelligence in language learning: tracing between the years 2017 and 2023. Artif Intell Rev 57, 107 (2024). https://doi.org/10.1007/s10462-023-10643-9
Accepted:
Published:
DOI: https://doi.org/10.1007/s10462-023-10643-9