Abstract
Advances in air monitoring methods have made it possible to analyze large-scale air pollution phenomena. Mining potential air pollution information from large-scale air pollution data is an important issue in the current environmental field. Although direct data visualization provides an intuitive presentation, the method is less applicable in long-time domains with high temporal resolution. To better meet the analysis needs of domain experts, we design a visual analysis framework based on friendly multi-view interactions and novel visual view designs. This framework can explore the spatiotemporal dynamics of multiple pollution data. In this paper, a two-stage cluster analysis method is proposed to extract possible transport patterns from large-scale pollutant transport trajectories. This method will be substantially helpful for domain experts to make relevant decisions. At the same time, the index is constructed from long-time series data at the grid point in the specific transport trajectories. This structure can help experts complete the sketch match with custom time resolution. It can assist domain experts in extracting key possible time-varying features. Finally, we verified the validity through spatial and temporal case analysis for pollutant data.
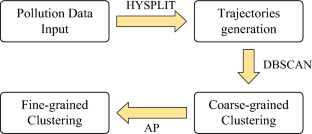













Similar content being viewed by others
References
Xu, X., Yang, H., Li, C.: Theoretical model and actual characteristics of air pollution affecting health cost: a review. Int. J. Environ. Res. Public Health (2022). https://doi.org/10.3390/ijerph19063532
Pandya, S., Gadekallu, T.R., Maddikunta, P.K.R., Sharma, R.: A study of the impacts of air pollution on the agricultural community and yield crops (indian context). Sustainability (2022). https://doi.org/10.3390/su142013098
Bachechi, C., Po, L., Rollo, F.: Big data analytics and visualization in traffic monitoring. Big Data Res. 27, 100292 (2022). https://doi.org/10.1016/j.bdr.2021.100292
Blázquez-García, A., Conde, A., Mori, U., Lozano, J.A.: A review on outlier/anomaly detection in time series data. ACM Comput. Surv. (2021). https://doi.org/10.1145/3444690
Guo, Y., Guo, S., Jin, Z., Kaul, S., Gotz, D., Cao, N.: Survey on visual analysis of event sequence data. IEEE Trans. Vis. Comput. Graph. 28(12), 5091–5112 (2022). https://doi.org/10.1109/TVCG.2021.3100413
Taylor, G.I.: I. eddy motion in the atmosphere. Philos. Trans. R. Soc. Lond. Ser. A Contain. Pap. Math. Phys. Character 215(523-537), 1–26 (1915). https://doi.org/10.1098/rsta.1915.0001
Taylor, G.I.: Diffusion by continuous movements. Proc. Lond. Math. Soc. 2(1), 196–212 (1922). https://doi.org/10.1112/plms/s2-20.1.196
Liu, Y., Wang, T.: Worsening urban ozone pollution in china from 2013 to 2017—part 2: the effects of emission changes and implications for multi-pollutant control. Atmos. Chem. Phys. 20(11), 6323–6337 (2020). https://doi.org/10.5194/acp-20-6323-2020
Dong, Z., Wang, S., Xing, J., Chang, X., Ding, D., Zheng, H.: Regional transport in beijing-tianjin-hebei region and its changes during 2014–2017: the impacts of meteorology and emission reduction. Sci. Total Environ. 737, 139792 (2020). https://doi.org/10.1016/j.scitotenv.2020.139792
Ballesteros-González, K., Sullivan, A.P., Morales-Betancourt, R.: Estimating the air quality and health impacts of biomass burning in northern south America using a chemical transport model. Sci. Total Environ. 739, 139755 (2020). https://doi.org/10.1016/j.scitotenv.2020.139755
Vander Hoorn, S., Johnson, J.S., Murray, K., Smit, R., Heyworth, J., Lam, S., Cope, M.: Emulation of a chemical transport model to assess air quality under future emission scenarios for the southwest of western australia. Atmosphere (2022). https://doi.org/10.3390/atmos13122009
Tao, H., Xing, J., Pan, G., Pleim, J., Ran, L., Wang, S., Chang, X., Li, G., Chen, F., Li, J.: Impact of anthropogenic heat emissions on meteorological parameters and air quality in Beijing using a high-resolution model simulation. Front. Environ. Sci. Eng. 16(4), 1–11 (2022). https://doi.org/10.1007/s11783-021-1478-3
Kim, M.: Visualization of dynamic network evolution with quantification of node attributes. IEEE Trans. Netw. Sci. Eng. 8(3), 2316–2325 (2021). https://doi.org/10.1109/TNSE.2021.3087334
Feng, Z., Li, H., Zeng, W., Yang, S.-H., Qu, H.: Topology density map for urban data visualization and analysis. IEEE Trans. Vis. Comput. Graph. 27(2), 828–838 (2021). https://doi.org/10.1109/TVCG.2020.3030469
Linhares, C.D., Ponciano, J.R., Paiva, J.G.S., Travençolo, B.A., Rocha, L.E.: A comparative analysis for visualizing the temporal evolution of contact networks: a user study. J. Vis. 24(5), 1011–1031 (2021). https://doi.org/10.1007/s12650-021-00759-x
Meidiana, A., Hong, S.-H., Eades, P.: New quality metrics for dynamic graph drawing. In: International Symposium on Graph Drawing and Network Visualization, pp. 450–465 (2020). https://doi.org/10.1007/978-3-030-68766-3_35
Ponciano, J.R., Linhares, C.D.G., Rocha, L.E.C., Faria, E.R., Travençolo, B.A.N.: Combining clutter reduction methods for temporal network visualization. In: Proceedings of the 37th ACM/SIGAPP Symposium on Applied Computing. SAC ’22, pp. 1748–1755. Association for Computing Machinery, New York, NY, USA (2022). https://doi.org/10.1145/3477314.3507018
Liu, Z., Huang, C., Yu, Y., Dong, J.: Motif-preserving dynamic attributed network embedding. In: Proceedings of the Web Conference 2021. WWW ’21, pp. 1629–1638. Association for Computing Machinery, New York, NY, USA (2021). https://doi.org/10.1145/3442381.3449821
Sabarish, B.A., Karthi, R., Kumar, T.G.: Graph similarity-based hierarchical clustering of trajectory data. Procedia Comput. Sci. 171, 32–41 (2020). https://doi.org/10.1016/j.procs.2020.04.004
Feng, K., Wang, P., Wu, J., Wang, W.: L-match: a lightweight and effective subsequence matching approach. IEEE Access 8, 71572–71583 (2020). https://doi.org/10.1109/ACCESS.2020.2987761
Fernandez, I., Manglik, A., Giannoula, C., Quislant, R., Mansouri Ghiasi, N., Gómez-Luna, J., Gutierrez, E., Plata, O., Mutlu, O.: Accelerating Time Series Analysis via Processing using Non-Volatile Memories. arXiv e-prints (2022) https://doi.org/10.48550/arXiv.2211.04369
Li, Z., Guo, J., Li, H., Wu, T., Mao, S., Nie, F.: Speed Up Similarity Search of Time Series Under Dynamic Time Warping, vol. 7, pp. 163644–163653 (2019). https://doi.org/10.1109/ACCESS.2019.2949838
Rakthanmanon, T., Campana, B., Mueen, A., Batista, G., Westover, B., Zhu, Q., Zakaria, J., Keogh, E.: Searching and mining trillions of time series subsequences under dynamic time warping. KDD ’12, pp. 262–270. Association for Computing Machinery, New York, NY, USA (2012). https://doi.org/10.1145/2339530.2339576
Linardi, M., Palpanas, T.: Scalable data series subsequence matching with ulisse. VLDB J. 29(6), 1449–1474 (2020). https://doi.org/10.1007/s00778-020-00619-4
Barandas, M., Folgado, D., Fernandes, L., Santos, S., Abreu, M., Bota, P., Liu, H., Schultz, T., Gamboa, H.: Tsfel: time series feature extraction library. SoftwareX 11, 100456 (2020). https://doi.org/10.1016/j.softx.2020.100456
Gao, J., Song, X., Wen, Q., Wang, P., Sun, L., Xu, H.: RobustTAD: Robust Time Series Anomaly Detection via Decomposition and Convolutional Neural Networks. arXiv e-prints (2020) https://doi.org/10.48550/arXiv.2002.09545arXiv:2002.09545
Ceci, M., Corizzo, R., Japkowicz, N., Mignone, P., Pio, G.: Echad: embedding-based change detection from multivariate time series in smart grids. IEEE Access 8, 156053–156066 (2020). https://doi.org/10.1109/ACCESS.2020.3019095
Boniol, P., Palpanas, T.: Series2Graph: Graph-based Subsequence Anomaly Detection for Time Series. arXiv e-prints, pp. 2207–12208 (2022) https://doi.org/10.48550/arXiv.2207.12208
Liu, D., Veeramachaneni, K., Geiger, A., Li, V.O.K., Qu, H.: AQEyes: Visual Analytics for Anomaly Detection and Examination of Air Quality Data. arXiv e-prints (2021) https://doi.org/10.48550/arXiv.2103.12910
Deng, Z., Weng, D., Chen, J., Liu, R., Wang, Z., Bao, J., Zheng, Y., Wu, Y.: Airvis: visual analytics of air pollution propagation. IEEE Trans. Vis. Comput. Graph. 26(1), 800–810 (2020). https://doi.org/10.1109/TVCG.2019.2934670
Lu, W., Ai, T., Zhang, X., He, Y.: An interactive web mapping visualization of urban air quality monitoring data of china. Atmosphere (2017). https://doi.org/10.3390/atmos8080148
Kalo, M., Zhou, X., Li, L., Tong, W., Piltner, R.: Chapter 8—sensing air quality: spatiotemporal interpolation and visualization of real-time air pollution data for the contiguous united states, pp. 169–196 (2020). https://doi.org/10.1016/B978-0-12-815822-7.00008-X
Qu, D., Lin, X., Ren, K., Liu, Q., Zhang, H.: Airexplorer: visual exploration of air quality data based on time-series querying. J. Vis. 23(6), 1129–1145 (2020). https://doi.org/10.1007/s12650-020-00683-6
Chen, P.: Visualization of real-time monitoring datagraphic of urban environmental quality. Eurasip J. Image Video Process. 2019(1), 1–9 (2019). https://doi.org/10.1186/s13640-019-0443-6
Yang, X., Peng, H., Zhang, Q.: Visual analysis of heterogenous air pollution data. In: Proceedings of the 4th International Conference on Computer Science and Software Engineering. CSSE ’21, pp. 300–306. Association for Computing Machinery, New York, NY, USA (2021). https://doi.org/10.1145/3494885.3494940
Kong, L., Tang, X., Zhu, J., Wang, Z., Li, J., Wu, H., Wu, Q., Chen, H., Zhu, L., Wang, W., Liu, B., Wang, Q., Chen, D., Pan, Y., Song, T., Li, F., Zheng, H., Jia, G., Lu, M., Wu, L., Carmichael, G.R.: A 6-year-long (2013–2018) high-resolution air quality reanalysis dataset in china based on the assimilation of surface observations from cnemc. Earth Syst. Sci. Data 13(2), 529–570 (2021). https://doi.org/10.5194/essd-13-529-2021
Jooybari, S.A., Peyrowan, H., Rezaee, P., Gholami, H.: Evaluation of pollution indices, health hazards and source identification of heavy metal in dust particles and storm trajectory simulation using hysplit model (case study: Hendijan center dust, southwest of iran). Environ. Monit. Assess. 194(2), 107 (2022). https://doi.org/10.1007/s10661-022-09760-9
Zaib, S., Lu, J., Bilal, M.: Spatio-temporal characteristics of air quality index (aqi) over northwest china. Atmosphere (2022). https://doi.org/10.3390/atmos13030375
Ouyang, T., Shen, X.: Online structural clustering based on dbscan extension with granular descriptors. Inf. Sci. 607, 688–704 (2022). https://doi.org/10.1016/j.ins.2022.06.027
Chen, J., Chen, B.: Development of driving cycle for light vehicle based on the ap clustering method. In: Computational and Experimental Simulations in Engineering: Proceedings of ICCES 2020, vol. 2, pp. 495–506 (2021). https://doi.org/10.1007/978-3-030-67090-0_40
Chatzigeorgakidis, G., Skoutas, D., Patroumpas, K., Athanasiou, S., Skiadopoulos, S.: Indexing geolocated time series data. In: Proceedings of the 25th ACM SIGSPATIAL International Conference on Advances in Geographic Information Systems. SIGSPATIAL ’17. Association for Computing Machinery, New York, NY, USA (2017). https://doi.org/10.1145/3139958.3140003
Wen, M., Ma, Y., Zhang, W., Tian, Y., Wang, Y.: High-resolution load profile clustering approach based on dynamic largest triangle three buckets and multiscale dynamic warping path under limited warping path length. J. Mod. Power Syst. Clean Energy (2022). https://doi.org/10.35833/MPCE.2022.000386
Acknowledgements
This work was partially supported by the National Key R &D Program of China under Grand No. 2021YFE0108400 and partly supported by the National Natural Science Foundation of China under Grant No. 62172294.
Author information
Authors and Affiliations
Corresponding author
Additional information
Publisher's Note
Springer Nature remains neutral with regard to jurisdictional claims in published maps and institutional affiliations.
Rights and permissions
Springer Nature or its licensor (e.g. a society or other partner) holds exclusive rights to this article under a publishing agreement with the author(s) or other rightsholder(s); author self-archiving of the accepted manuscript version of this article is solely governed by the terms of such publishing agreement and applicable law.
About this article
Cite this article
Li, J., Bi, C. Visual analysis of air pollution spatio-temporal patterns. Vis Comput 39, 3715–3726 (2023). https://doi.org/10.1007/s00371-023-02961-4
Accepted:
Published:
Issue Date:
DOI: https://doi.org/10.1007/s00371-023-02961-4