Abstract
The prediction of fluid dynamics in multiphase bubble column reactors is a subject of major concern to appropriately design and optimize them. This paper employs the combination of computational fluid dynamics (CFD) (i.e., Euler–Euler approach) and adaptive neuro-fuzzy inference system (ANFIS) to propose new a viewpoint for multiphase modeling, including the accuracy of soft computing technique in prediction of a 3D bubble column reactor. Existing experimental, numerical and correlations results in the literature have been used to validate the implementation of the Euler–Euler approach. The results of Euler–Euler approach for a 3D bubble column reactor has been used for input training data which are liquid velocity, turbulent kinetic energy and gas hold-up. The ANFIS results have been also compared with Eulerian results, using root-mean-square error (RMSE) and coefficient of determination and Pearson coefficient. The results show that, flow pattern and gas hold-up are mainly affected by bubble column height, meaning towards sparger region, gas hold-up has a higher value near the ring sparger. According to the results, a greater improvement in estimation has been achieved through the ANFIS. Overall, the results show that ANFIS is a robust method to predict bubble column hydrodynamics parameters (e.g., liquid flow pattern and gas hold-up) as input. In addition, the exactness of the proposed ANFIS model may be boosted by considering more meteorological parameters as input values.












Similar content being viewed by others
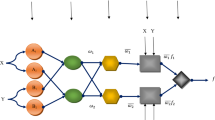
Abbreviations
- \(C_{\text{D}}\) :
-
Drag force coefficient (dimensionless)
- \(C_{\text{TD}}\) :
-
Turbulent dispersion coefficient (dimensionless)
- \(C_{\varepsilon 1}\) :
-
Model parameter in turbulent dissipation energy equation (dimensionless)
- \(C_{\varepsilon 2}\) :
-
Model parameter in turbulent dissipation energy equation (dimensionless)
- \(C_{\mu }\) :
-
Constant in k–ε model (dimensionless)
- \(C_{{\mu ,{\text{BI}}}}\) :
-
Constant in bubble-induced turbulence model (dimensionless)
- \(d_{\text{B}}\) :
-
Bubble diameter (m)
- \(d_{0}\) :
-
Sparger hole diameter (m)
- \(D\) :
-
Diameter of the column (m)
- S d :
-
Sparger diameter
- \(g\) :
-
Gravitational constant (m/s2)
- \(G\) :
-
Generation term (kg/m s2)
- \(H\) :
-
Height (m)
- \(k\) :
-
Turbulent kinetic energy per unit mass (m2/s2)
- \(M_{\text{I}}\) :
-
Total interfacial force acting between two phases (N/m3)
- \(M_{\text{e}}\) :
-
Drag force (N/m3)
- \(P\) :
-
Pressure (N/m2)
- \(r\) :
-
Radial distance (m)
- \(R\) :
-
Column radius (m)
- \({\text{Re}}_{\text{B}}\) :
-
Reynolds number (\(= d_{\text{B}} V_{\text{S}} /v\)) (dimensionless)
- \(V_{\text{G}}\) :
-
Superficial gas velocity (m/s)
- \(V_{\text{T}}\) :
-
Terminal velocity (m2/s)
- \(\varepsilon\) :
-
Turbulent energy dissipation rate per unit mass (m2/s3)
- \(\in\) :
-
Fractional phase hold-up (dimensionless)
- \(\bar{ \in }\) :
-
Average fractional phase hold-up (dimensionless)
- \(\mu\) :
-
Molecular viscosity (Pa s)
- \(\mu_{\text{BI}}\) :
-
Bubble-induced viscosity (Pa s)
- \(\mu_{\text{eff}}\) :
-
Effective viscosity (Pa s)
- \(\rho\) :
-
Density (kg/m3)
- \(\mu_{\text{T}}\) :
-
Turbulent viscosity (Pa s)
- \(\sigma\) :
-
Surface tension (N/m)
- \(\sigma_{\varepsilon }\) :
-
Prandtl number for turbulent energy dissipation rate (dimensionless)
- \(\sigma_{k}\) :
-
Prandtl number for turbulent kinetic energy (dimensionless)
- \(\tau_{k}\) :
-
Shear stress of phase k (Pa)
- \({\text{G}}\) :
-
Gas phase
- \({\text{L}}\) :
-
Liquid phase
References
Buwa VV, Gerlach D, Durst F, Schlücker E (2007) Numerical simulations of bubble formation on submerged orifices: period-1 and period-2 bubbling regimes. Chem Eng Sci 62:7119–7132
Chen P (2004) Modeling the fluid dynamics of bubble column flows, Washington University, 2004. Department of Chemical Engineering
Dhotre MT, Ekambara K, Joshi JB (2004) CFD simulation of sparger design and height to diameter ratio on gas hold-up profiles in bubble column reactors. Exp Thermal Fluid Sci 28(5):407–421
Bouaifi M, Hebrard G, Bastoul D, Roustan M (2001) A comparative study of gas hold-up, bubble size, interfacial area and mass transfer coefficients in stirred gas–liquid reactors and bubble columns. Chem Eng Process 40(2):97–111
Kantarci N, Borak F, Ulgen KO (2005) Bubble column reactors. Process Biochem 40:2263–2283
Krishna R, Urseanu M, Van Baten J, Ellenberger J (2000) Liquid phase dispersion in bubble columns operating in the churn-turbulent flow regime. Chem Eng J 78:43–51
Krishna R, Van Baten J (1999) Rise characteristics of gas bubbles in a 2D rectangular column: VOF simulations vs experiments. Int Commun Heat Mass Transfer 26:965–974
Krishna R, Van Baten J (2001) Eulerian simulations of bubble columns operating at elevated pressures in the churn turbulent flow regime. Chem Eng Sci 56:6249–6258
Olmos E (2002) Étude expérimentale et numérique des écoulements gaz-liquide en colonne à bulles. INPL, Vandoeuvre-les-Nancy
Olmos E, Gentric C, Midoux N (2003) Numerical description of flow regime transitions in bubble column reactors by a multiple gas phase model. Chem Eng Sci 58:2113–2121
Zhang D, Deen N, Kuipers J (2006) Numerical simulation of the dynamic flow behavior in a bubble column: a study of closures for turbulence and interface forces. Chem Eng Sci 61:7593–7608
Pfleger D, Becker S (2001) Modelling and simulation of the dynamic flow behaviour in a bubble column. Chem Eng Sci 56:1737–1747
Pfleger D, Gomes S, Gilbert N, Wagner H-G (1999) Hydrodynamic simulations of laboratory scale bubble columns fundamental studies of the Eulerian-Eulerian modelling approach. Chem Eng Sci 54:5091–5099
Lin HY, Chen W, Tsutsumi A (2003) Long-term prediction of nonlinear hydrodynamics in bubble columns by using artificial neural networks. Chem Eng Process 42:611–620
Al-Masry WA (2006) Analysis of hydrodynamics of external loop circulating bubble columns with open channel gas separators using neural networks. Chem Eng Res Des 84:483–486
Massinaei M, Doostmohammadi R (2010) Modeling of bubble surface area flux in an industrial rougher column using artificial neural network and statistical techniques. Miner Eng 23:83–90
Shaikh A, Al-Dahhan M (2003) Development of an artificial neural network correlation for prediction of overall gas holdup in bubble column reactors. Chem Eng Process 42:599–610
Lemoine R, Behkish A, Sehabiague L, Heintz YJ, Oukaci R, Morsi BI (2008) An algorithm for predicting the hydrodynamic and mass transfer parameters in bubble column and slurry bubble column reactors. Fuel Process Technol 89:322–343
Chen W, Hasegawa T, Tsutsumi A, Otawara K, Shigaki Y (2003) Generalized dynamic modeling of local heat transfer in bubble columns. Chem Eng J 96:37–44
Gandhi AB, Joshi JB, Kulkarni AA, Jayaraman VK, Kulkarni BD (2008) SVR-based prediction of point gas hold-up for bubble column reactor through recurrence quantification analysis of LDA time-series. Int J Multiph Flow 34(12):1099–1107
Gandhi AB, Joshi JB, Jayaraman VK, Kulkarni BD (2007) Development of support vector regression (SVR)-based correlation for prediction of overall gas hold-up in bubble column reactors for various gas–liquid systems. Chem Eng Sci 62(24):7078–7089
Petković D, Pavlović ND, Ćojbašić Ž, Pavlović NT (2013) Adaptive neuro fuzzy estimation of underactuated robotic gripper contact forces. Expert Syst Appl 40:281–286
Petković D, Issa M, Pavlović ND, Pavlović NT, Zentner L (2012) Adaptive neuro-fuzzy estimation of conductive silicone rubber mechanical properties. Expert Syst Appl 39:9477–9482
Petković D, Ćojbašić Ž, Lukić S (2013) Adaptive neuro fuzzy selection of heart rate variability parameters affected by autonomic nervous system. Expert Syst Appl 40:4490–4495
Petković D, Ćojbašić Ž (2012) Adaptive neuro-fuzzy estimation of autonomic nervous system parameters effect on heart rate variability. Neural Comput Appl 21:2065–2070
Dastorani MT (2010) Application of ANN and ANFIS models on dryland precipitation prediction (case study: Yazd in central Iran). J Appl Sci 10:2387–2394
El-Shafie A, Jaafer O, Seyed A (2011) Adaptive neuro-fuzzy inference system based model for rainfall forecasting in Klang River, Malaysia. Int J Phys Sci 6:2875–2888
Sato Y, Sekoguchi K (1975) Liquid velocity distribution in two-phase bubble flow. Int J Multiph Flow 2:79–95
Lopez de Bertodano M, Lahey RT Jr, Jones OC (1994) Turbulent bubbly two-phase flow data in a triangular duct. Nucl Eng Des 146:43–52
Sokolichin A, Eigenberger G (1999) Applicability of the standard k-e turbulence model to the dynamic simulation of bubble columns-Part I: detailed numerical simulations. Chem Eng Sci 54:2273–2284
Deen NG, Solberg T, Hjertager BH (2000) Numerical simulation of the gas-liquid flow in a square cross-sectioned bubble column. In: 14th int. congress of chemical and process engineering, Praha-Czech Republic
Safa M, Shariati M, Ibrahim Z, Toghroli A, Baharom SB, Nor NM et al (2016) Potential of adaptive neuro fuzzy inference system for evaluating the factors affecting steel-concrete composite beam’s shear strength. Steel Compos Struct 21(3):679–688
Toghroli A, Mohammadhassani M, Shariati M, Suhatril M, Ibrahim Z, Ramli Sulong NH (2014) Prediction of shear capacity of channel shear connectors using the ANFIS model. Steel Compos Struct 17(5):623–639
Mansouri I, Shariati M, Safa M, Ibrahim Z, Tahir MM, Petković D (2019) Analysis of influential factors for predicting the shear strength of a V-shaped angle shear connector in composite beams using an adaptive neuro-fuzzy technique. J Intell Manuf 30(3):1247–1257
Mohammadhassani M, Saleh A, Suhatril M, Safa M (2015) Fuzzy modelling approach for shear strength prediction of RC deep beams. Smart Struct Syst 16(3):497–519
Toghroli A, Darvishmoghaddam E, Zandi Y, Parvan M, Safa M, Abdullahi M et al (2018) Evaluation of the parameters affecting the Schmidt rebound hammer reading using ANFIS method. Comput Concrete 21(5):525–530
Sedghi Y, Zandi Y, Shariati M, Ahmadi E, Moghimi Azar V, Toghroli A et al (2018) Application of ANFIS technique on performance of C and L shaped angle shear connectors. Smart Struct Syst 22(3):335–340
Toghroli A (2015) Applications of the ANFIS and LR models in the prediction of shear connection in composite beams. Jabatan Kejuruteraan Awam, Fakulti Kejuruteraan, Universiti Malaya
Author information
Authors and Affiliations
Corresponding author
Additional information
Publisher's Note
Springer Nature remains neutral with regard to jurisdictional claims in published maps and institutional affiliations.
Rights and permissions
About this article
Cite this article
Jović, S. Adaptive neuro-fuzzy prediction of flow pattern and gas hold-up in bubble column reactors. Engineering with Computers 37, 1723–1734 (2021). https://doi.org/10.1007/s00366-019-00905-y
Received:
Accepted:
Published:
Issue Date:
DOI: https://doi.org/10.1007/s00366-019-00905-y