Abstract
A key feature of geodetic adjustment theory is the description of stochastic properties of the estimated quantities. A variety of tools and measures have been developed to describe the quality of ordinary least-squares estimates, for example, variance-covariance information, redundancy numbers, etc. Many of these features can easily be extended to a constrained least-squares estimate with equality constraints. However, this is not true for inequality constrained estimates. In many applications in geodesy the introduction of inequality constraints could improve the results (e.g. filter and network design or the regularization of ill-posed problems). This calls for an adequate stochastic modeling accompanying the already highly developed estimation theory in the field of inequality constrained estimation. Therefore, in this contribution, an attempt is made to develop measures for the quality of inequality constrained least-squares estimates combining Monte Carlo methods and the theory of quadratic programming. Special emphasis is placed on the derivation of confidence regions.
Access this chapter
Tax calculation will be finalised at checkout
Purchases are for personal use only
Similar content being viewed by others
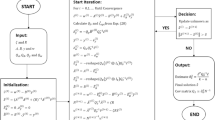
References
Boyd S, Vandenberghe L (2004) Convex optimization. Cambridge University Press, Cambridge
Chen MH, Shao QM (1999) Monte Carlo estimation of Bayesian credible and HPD intervals. J Comput Graph Stat 8(1):69–92
Dantzig G (1998) Linear programming and extensions. Princeton University Press, Princeton
Fritsch D (1985) Some additional informations on the capacity of the linear complementarity algorithm. In: Grafarend E, Sansò F (eds) Optimization and design of geodetic networks. Springer, Berlin/Heidelberg/New York/Tokyo, pp 169–184
Geweke J (1986) Exact Inference in the inequality constrained normal linear regression model. J Appl Econ 1(2):127–41
Gill P, Murray W, Wright M (1981) Practical optimization. Academic Press, London
ISO (2008) Evaluation of measurement data—Supplement 1 to the “Guide to the expression of uncertainty in measurement”—Propagation of distributions using a Monte Carlo method, Joint Committee for Guides in Metrology, Bureau International des Poids et Mesures, Geneva
Koch A (2006) Semantische Integration von zweidimensionalen GIS-Daten und Digitalen Geländemodellen. Ph.D. thesis, Universität Hannover
Koch KR (1981) Hypothesis testing with inequalities. Bollettino di geodesia e scienze affini 2:136–144
Koch KR (2007) Introduction to bayesian statistics, 2nd edn. Springer, Berlin
Liew CK (1976) Inequality constrained least-squares estimation. J Am Stat Assoc 71(355):746–751
Peng J, Zhang H, Shong S, Guo C (2006) An aggregate constraint method for inequality-constrained least squares problems. J Geodesy 79:705–713. Doi:10.1007/s00190-006-0026-z
Roese-Koerner L, Krasbutter I, Schuh WD (2012a) A constrained quadratic programming technique for data-adaptive design of decorrelation filters. In: Sneeuw N, Novak P, Crespi M, Sanso F, Sideris MG (eds) VII Hotine-Marussi Symposium on Mathematical Geodesy, Rome, 2009, Springer, International Association of Geodesy Symposia, vol 137, pp 165–170. DOI 10.1007/978-3-642-22078-4_25
Roese-Koerner L, Devaraju B, Sneeuw N, Schuh WD (2012b) A stochastic framework for inequality constrained estimation. J Geodesy 86(11):1005–1018. Doi:10.1007/s00190-012-0560-9
Zhu J, Santerre R, Chang XW (2005) A Bayesian method for linear, inequality-constrained adjustment and its application to GPS positioning. J Geodesy 78:528–534. Doi:10.1007/s00190-004-0425-y
Author information
Authors and Affiliations
Corresponding author
Editor information
Editors and Affiliations
Rights and permissions
Copyright information
© 2015 Springer International Publishing Switzerland
About this paper
Cite this paper
Roese-Koerner, L., Devaraju, B., Schuh, WD., Sneeuw, N. (2015). Describing the Quality of Inequality Constrained Estimates. In: Kutterer, H., Seitz, F., Alkhatib, H., Schmidt, M. (eds) The 1st International Workshop on the Quality of Geodetic Observation and Monitoring Systems (QuGOMS'11). International Association of Geodesy Symposia, vol 140. Springer, Cham. https://doi.org/10.1007/978-3-319-10828-5_3
Download citation
DOI: https://doi.org/10.1007/978-3-319-10828-5_3
Published:
Publisher Name: Springer, Cham
Print ISBN: 978-3-319-10827-8
Online ISBN: 978-3-319-10828-5
eBook Packages: Earth and Environmental ScienceEarth and Environmental Science (R0)