Abstract
Regarding the multi-phase characteristic of batch process, a new phase separation method is developed in this paper. The method realizes a 3-step sub-phase separation of the batch process using the retained principal components number, loading matrixes and principal component matrixes, which can adequately reflect the features variation of the process. In line with the different features and classification step, automatic identification of ‘burrs’ and transition phases has been expounded. The proposed method can directly separate the stable phases and transition phases in the batch process, and deduce high-precision transition phase models. Based on the proposed method, the MPCA modeling and online monitoring is applied in the injection molding process. The experimental results have verified the effectiveness of the proposed method.
Similar content being viewed by others
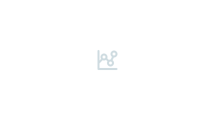
References
J. E. Jackson, A User’s Guide to Principal Components, Wiley, New York, 1991.
T. Kourti and J. F. MacGregor, “Process analysis, monitoring and diagnosis, using multivariate projection methods,” Chemometrics and Intelligent Laboratory Systems, vol. 28, no. 1, pp. 3–21, April 1995.
X. Z. Wang, Data Mining and Knowledge Discovery for Process Monitoring and Control, Springer, London, 1999.
P. Geladi and B. R. Kowalshi, “Partial least squares regression: a tutorial,” Analytica Chimica Acta, vol. 185, no. 1, pp. 1–17, 1986.
A. Hoskuldsson, “PLS regression methods,” Journal of Chemometrics, vol. 2, no. 3, pp. 211–228, 1988.
N. Y. Lu, F. L. Wang, F. R. Gao, and S. Wang, “Statistical modeling and online monitoring for batch process,” Acta Automatica Sinica, vol. 32, no. 3, pp. 400–410, June 2006.
P. Nomikos and J. F. MacGregor, “Monitoring batch processes using multiway principal component analysis,” AIChE Journal vol. 40, no. 8, pp. 1361–1375, 1994.
P. Nomikos and J. F. MacGregor, “Multi-way partial least squares in monitoring batch processes,” Chemometrics and Intelligent Laboratory Systems, no. 1, pp. 30, 97–108, 1995.
P. Nomikos and J. F. MacGregor, “Multivariate SPC charts for monitoring batch processes,” Technometrics, vol. 37, no. 1, pp. 41–59, 1995.
K. A. Kosanovich, M. J. Piovoso, and K. S. Dahl, “Multi-way PCA applied to an industrial batch process,” Proc. of American Control Conference, pp. 1294–1298, 1994.
N. Y. Lu, F. R. Gao, and F. L. Wang, “A sub-PCA modeling and on-line monitoring strategy for batch processes,” AIChE Journal vol. 50, no. 1, pp. 255–259, 2004.
C. H. Zhao, F. L. Wang, N. Y. Lu, and M. X. Jia, “Stage-based soft-transition multiple PCA modeling and on-line monitoring strategy for batch processes,” Journal of Process Control, vol. 17, no. 9, pp. 728–741, 2007.
S. Wold, “Cross-validatory estimation of the number of components in factor and principal components models,” Technometrics, vol. 20, no. 4, pp. 397–405, 1978.
J. A. Westerhuis, T. Kourti, and J. F. MacGregor, “Comparing alternative approaches for multivariate statistical analysis of batch process data,” Journal of Chemometrics, vol. 13, no. 34, pp. 397–413, 1999.
L. Xu, A. Krzyzak, and E. Oja, “Rival penalized competitive learning for clustering analysis, RBF net and curve detection,” IEEE Trans. on Neural Networks, vol. 4, no. 4, pp. 636–649, July 1993.
C. H. Zhao, F. L. Wang, Z. Z. Mao, N. Y. Lu, and M. X. Jia, “Quality prediction based on phasespecific average trajectory for batch processes,” AIChE Journal, vol. 54, no. 3, pp. 693–705, January 2008.
Author information
Authors and Affiliations
Corresponding author
Additional information
Recommended by Editorial Board member Bin Jiang under the direction of Editor Zengqi Sun.
This work was supported by the National Natural Science Foundation of China (61174130,61074074), project 973 under Grant (2009CB320601) and the Fundamental Research Funds for the Central Universities (N110304010, N1100404022).
Shu Wang received her Ph.D. degree from Northeastern University in 2010. Her research interests covers multivariate statistical modeling, process monitoring, and fault diagnosis and their applications in industry process.
Yu-Qing Chang received her Ph.D. degree from Northeastern University in 2002. Her research interests include process modeling, process monitoring and quality prediction and their applications in industry process.
Zhen Zhao received her Ph.D. degree from Northeastern University in 2010. Her research interests covers multivariate statistical modeling, process monitoring, and fault diagnosis and their applications in industry process.
Fu-Li Wang is a Professor at Northeastern University. His research interests covers modeling and optimization of complex system, and failure diagnosis.
Rights and permissions
About this article
Cite this article
Wang, S., Chang, YQ., Zhao, Z. et al. Multi-phase MPCA modeling and application based on an improved phase separation method. Int. J. Control Autom. Syst. 10, 1136–1145 (2012). https://doi.org/10.1007/s12555-012-0608-x
Received:
Revised:
Accepted:
Published:
Issue Date:
DOI: https://doi.org/10.1007/s12555-012-0608-x