Abstract
Purpose
Model-based iterative reconstruction (MBIR) was recently shown to enable dose reduction in computed tomography (CT). The detectability of low-contrast lesions was assessed on CT images reconstructed with MBIR compared with the conventional filtered back-projection (FBP) method.
Materials and methods
A phantom simulating brain gray matter containing small lesions mimicking early cerebral infarctions was scanned at tube currents of 50, 100, 200, and 400 mA. Images were reconstructed by use of both methods. Round regions were cropped from the reconstructed images, half with a lesion, the other half without. Eight radiologists reviewed the images and scored the certainty of lesion detection on a 5-point scale. Overall performance was analyzed by use of a receiver operating characteristic curve.
Results
For the tube currents investigated, the analysis showed that the mean areas under the curves for the reviewers were 0.65, 0.70, 0.82, and 0.83 for FBP and 0.70, 0.76, 0.78, and 0.90 for MBIR. For each current, there was no significant difference between the areas under the curves for the different reconstruction methods (p = 0.32, 0.24, 0.49, and 0.17).
Conclusion
For the small, low-contrast lesions in the phantom model used in this study, no significant difference between detectability was observed for MBIR and FBP.







Similar content being viewed by others
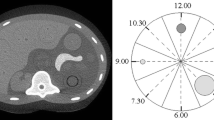
References
Brenner DJ, Hall EJ. Computed tomography—an increasing source of radiation exposure. N Engl J Med. 2007;357(22):2277–84.
Pan X, Sidkey EY, Vannier M. Why do commercial CT scanners still employ traditional, filtered back-projection for image reconstruction? Inverse Prob. 2009;25:1–37.
Cohnen M, Fischer H, Hamacher J, Lins E, Kötter R, Mödder U. CT of the head by use of reduced current and kilovoltage: relationship between image quality and dose reduction. Am J Neuroradiol. 2000;21:1654–60.
Mullins ME, Lev MH, Bove P, O’Reilly CE, Saini S, Rhea JT, et al. Comparison of image quality between conventional and low-dose nonenhanced head CT. Am J Neuroradiol. 2004;25:533–8.
Willemink MJ, de Jong PA, Leiner T, de Heer LM, Nievelstein RA, Budde RP, et al. Iterative reconstruction techniques for computed tomography Part 1: technical principles. Eur Radiol. 2013;23:1623–31.
Willemink MJ, Leiner T, de Jong PA, de Heer LM, Nievelstein RA, Schilham AM, et al. Iterative reconstruction techniques for computed tomography part 2: initial results in dose reduction and image quality. Eur Radiol. 2013;23:1632–42.
Thibault JB, Sauer KD, Bouman CA, Hsieh J. A three-dimensional statistical approach to improved image quality for multislice helical CT. Med Phys. 2007;34:4526–44.
Yu Z, Thibault J-B, Bouman CA, Sauer KD, Hsieh J. Fast model-based X-ray CT reconstruction using spatially nonhomogeneous ICD optimization. IEEE Trans Image Process. 2010;20:161–75.
Scheffel H, Stolzmann P, Schlett CL, Engel LC, Major GP, Karolyi M, et al. Coronary artery plaques: cardiac CT with model-based and adaptive-statistical iterative reconstruction technique. Eur J Radiol. 2012;81:e363–9.
Katsura M, Matsuda I, Akahane M, Sato J, Akai H, Yasaka K, et al. Model-based iterative reconstruction technique for radiation dose reduction in chest CT: comparison with the adaptive statistical iterative reconstruction technique. Eur Radiol. 2012;22:1613–23.
Pickhardt PJ, Lubner MG, Kim DH, Tang J, Ruma JA, del Rio AM, et al. Abdominal CT with model-based iterative reconstruction (MBIR): initial results of a prospective trial comparing ultralow-dose with standard-dose imaging. Am J Roentgenol. 2012;199:1266–74.
Deak Z, Grimm JM, Treitl M, Geyer LL, Linsenmaier U, Korner M, et al. Filtered back projection, adaptive statistical iterative reconstruction, and a model-based iterative reconstruction in abdominal CT: an experimental clinical study. Radiology. 2013;266:197–206.
Machida H, Takeuchi H, Tanaka I, Fukui R, Shen Y, Ueno E, et al. Improved delineation of arteries in the posterior fossa of the brain by model-based iterative reconstruction in volume-rendered 3D CT angiography. Am J Neuroradiol. 2013;34:971–5.
Machida H, Tanaka I, Fukui R, Kita K, Shen Y, Ueno E, et al. Improved delineation of the anterior spinal artery with model-based iterative reconstruction in CT angiography: a clinical pilot study. Am J Roentgenol. 2013;200:442–6.
Vardhanabhuti V, Loader RJ, Mitchell GR, Riordan RD, Roobottom CA. Image quality assessment of standard- and low-dose chest CT using filtered back projection, adaptive statistical iterative reconstruction, and novel model-based iterative reconstruction algorithms. Am J Roentgenol. 2013;200:545–52.
Suzuki S, Machida H, Tanaka I, Ueno E. Vascular diameter measurement in CT angiography: comparison of model-based iterative reconstruction and standard filtered back projection algorithms in vitro. Am J Roentgenol. 2013;200:652–7.
Shuman WP, Green DE, Busey JM, Kolokythas O, Mitsumori LM, Koprowicz KM, et al. Model-based iterative reconstruction versus adaptive statistical iterative reconstruction and filtered back projection in liver 64-MDCT: focal lesion detection, lesion conspicuity, and image noise. Am J Roentgenol. 2013;200:1071–6.
Volders D, Bols A, Haspeslagh M, Coenegrachts K. Model-based iterative reconstruction and adaptive statistical iterative reconstruction techniques in abdominal ct: comparison of image quality in the detection of colorectal liver metastases. Radiology. 2013;269:469–74.
Nishida J, Kitagawa K, Nagata M, Yamazaki A, Nagasawa N, Sakuma H. Model-based iterative reconstruction for multi-detector row CT assessment of the Adamkiewicz artery. Radiology. 2014;270:282–91.
Smith EA, Dillman JR, Goodsitt MM, Christodoulou EG, Keshavarzi N, Strouse PJ. Model-based iterative reconstruction: effect on patient radiation dose and image quality in pediatric body CT. Radiology. 2014;270:526–34.
Korn A, Fenchel M, Bender B, Danz S, Hauser TK, Ketelsen D, et al. Iterative reconstruction in head CT: image quality of routine and low-dose protocols in comparison with standard filtered back-projection. Am J Neuroradiol. 2012;33:218–24.
Wu TH, Hung SC, Sun JY, Lin CJ, Lin CH, Chiu CF, et al. How far can the radiation dose be lowered in head CT with iterative reconstruction? Analysis of imaging quality and diagnostic accuracy. Eur Radiol. 2013;23:2612–21.
Vorona GA, Zuccoli G, Sutcavage T, Clayton BL, Ceschin RC, Panigrahy A. The use of adaptive statistical iterative reconstruction in pediatric head CT: a feasibility study. Am J Neuroradiol. 2013;34:205–11.
Rapalino O, Kamalian S, Kamalian S, Payabvash S, Souza LC, Zhang D, et al. Cranial CT with adaptive statistical iterative reconstruction: improved image quality with concomitant radiation dose reduction. Am J Neuroradiol. 2012;33:609–15.
Kilic K, Erbas G, Guryildirim M, Arac M, Ilgit E, Coskun B. Lowering the dose in head CT using adaptive statistical iterative reconstruction. AJNR Am J Neuroradiol. 2011;32:1578–82.
Ren Q, Dewan SK, Li M, Li J, Mao D, Wang Z, et al. Comparison of adaptive statistical iterative and filtered back projection reconstruction techniques in brain CT. Eur J Radiol. 2012;81:2597–601.
Tanaka C, Ueguchi T, Shimosegawa E, Sasaki N, Johkoh T, Nakamura H, et al. Effect of CT acquisition parameters in the detection of subtle hypoattenuation in acute cerebral infarction: a phantom study. Am J Neuroradiol. 2006;27:40–5.
Dorfman DD, Berbaum KS, Metz CE. Receiver operating characteristic rating analysis. Generalization to the population of readers and patients with the jackknife method. Invest Radiol. 1992;27:723–31.
Medical Image Perception Laboratory > Software > Receiver Operating Characteristic (ROC) > DBM MRMC. 2013. http://perception.radiology.uiowa.edu/Software/ReceiverOperatingCharacteristicROC/DBMMRMC/tabid/116/Default.aspx. Accessed 07 Oct 2013.
Siewerdsen JH, Antonuk LE, el-Mohri Y, Yorkston J, Huang W, Cunningham IA. Signal, noise power spectrum, and detective quantum efficiency of indirect-detection flat-panel imagers for diagnostic radiology. Med Phys. 1998;25:614–28.
Giger ML, Doi K, Metz CE. Investigation of basic imaging properties in digital radiography. 2. Noise wiener spectrum. Med Phys. 1984;11:797–805.
Baker ME, Dong F, Primak A, Obuchowski NA, Einstein D, Gandhi N, et al. Contrast-to-noise ratio and low-contrast object resolution on full- and low-dose MDCT: sAFIRE versus filtered back projection in a low-contrast object phantom and in the liver. Am J Roentgenol. 2012;199:8–18.
Schindera ST, Odedra D, Raza SA, Kim TK, Jang H-J, Szucs-Farkas Z, et al. Iterative reconstruction algorithm for CT: can radiation dose be decreased while low-contrast detectability is preserved? Radiology. 2013;269:511–8.
Mieville FA, Gudinchet F, Brunelle F, Bochud FO, Verdun FR. Iterative reconstruction methods in two different MDCT scanners: physical metrics and 4-alternative forced-choice detectability experiments–a phantom approach. Phys Med. 2013;29:99–110.
Wagner RF. Decision theory and the detail signal-to-noise ratio of otto schade. Photogr Sci Eng. 1978;22:1–6.
Hanson KM. Detectability in computed tomographic images. Med Phys. 1979;6:441–51.
Loo LN, Doi K, Metz CE. A comparison of physical image quality indices and observer performance in the radiographic detection of nylon beads. Phys Med Biol. 1984;29:837–56.
Barrett HH, Myers KJ. Foundations of image science. Wiley Intersci. 2004:801–1000.
Conflict of interest
The authors declare that they have no conflict of interest.
Author information
Authors and Affiliations
Corresponding author
About this article
Cite this article
Nishizawa, M., Tanaka, H., Watanabe, Y. et al. Model-based iterative reconstruction for detection of subtle hypoattenuation in early cerebral infarction: a phantom study. Jpn J Radiol 33, 26–32 (2015). https://doi.org/10.1007/s11604-014-0376-z
Received:
Accepted:
Published:
Issue Date:
DOI: https://doi.org/10.1007/s11604-014-0376-z