Abstract
Coal mine fires due to spontaneous heating have been a great concern both for the industry and researchers worldwide. They start in a very small scale and gradually expand in size resulting in environmental, safety and economic concerns. Most of these fires could be averted if suitable preventive measures are taken. Since the spontaneous heating potential of all types of coals are not the same, its accurate forecasting is essential to plan efficient preventive measures, production schedule and storage capabilities of a mine. This study presents a neuro-fuzzy approach for forecasting the auto-oxidation of coals based on laboratory test results on fifty cone coal samples collected from different coalfields of India. Using the constituents of proximate analysis as input parameters, crossing point temperature (CPT) is predicted using adaptive neuro-fuzzy inference system (ANFIS) model. Gaussian membership function has been adopted for describing the input variables. The results indicate that ANFIS model can predict CPT values with reasonable accuracy.










Similar content being viewed by others
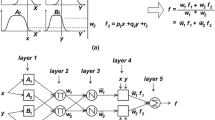
References
Abbasi E, Abouec A (2008) A stock price forecast by using neuro-fuzzy inference system. Proc World Acad Sci Eng Tech 36:320–323
Adamus A (2011) Selected measures to suppress spontaneous combustion in Ostrava-Karvina mines. Górnictwo I Geologia, pp 13–21
Akbarzadeh A, Mehrjardi RT et al (2009) Estimating of soil erosion covered with rolled erosion control systems using rainfall simulator (neuro-fuzzy and artificial neural network approaches). J Appl Sci Res 5(5):505–514
Arisoy A, Beamish BB, Cetegen E (2006) Modelling spontaneous combustion of coal. Turkish J Eng Environ Sci 30:193–201
Banerjee SC (2000) Prevention and combating mine fires. Special Indian edn, Oxford and IBH Publishing Co. Pvt. Ltd., New Delhi
Bhadeshia MKDH (1999) Neural networks in materials science. ISIJ Int 39(10):966–979
Boyacioglu MA, Avci D (2010) An adaptive network-based fuzzy inference system (ANFIS) for the prediction of stock market return: the case of the Istanbul stock exchange. Expert Syst Appl 37:7908–7912
Brooks K, Glasser D (1986) A simplified model of spontaneous combustion in coal stockpiles. Fuel 65(1):1035–1041
Brooks K, Bradshaw S, Glasser D (1988) Spontaneous combustion of coal stockpiles: an unusual chemical reaction engineering problem. Chem Engg Sci 43(8):2139–2145
Cai K, Xia JT, Li LT, Gui ZL (2005) Analysis of the electrical properties of PZT by a BP artificial neural network. Comput Mater Sci 34(2):166–172
Carres JN, Saghafi A (1998) Predicting spontaneous combustion in spoil piles from open cut coal mines. In: Aziz N (eds) Coal 1998: coal operators’ conference, University of Wollongong & the Australasian Institute of Mining and Metallurgy, pp 617–625
Cliff D, Beamish B, Cuddihy P, Rowlands D (2009) Explosions, fires and spontaneous combustion. In: Kinimonth RJ, Baafi EY (eds) Australasian coal mining practice. The Australasian Institute of Mining and Metallurgy, Carlton, Victoria, Australia, pp 800–814
Cygankiewicz J (2003) Forecasting of self heating of coal n underground workings. 30th International conference of safety in mines research institutes. J S Afr Inst Min Metall, pp 493–501
Dezfoli KA (2003) Principles of fuzzy theory and its application on water engineering problems. Jihad Press, Tehran
Didari V (1988) Developing a spontaneous combustion risk index for Turkish coal mines: preliminary studies. J Mines Metals Fuels: 211–215
Dong G, Longtu L, Cewen N, Juntao X, Zhilun G (2003) Modeling and analysis of the electrical properties of PZT through neural networks. J Eur Ceram Soc 23(12):2177–2181
Eroglu HN (2001) Spontaneous combustion testing techniques in South Africa, proceedings of 7th international mine ventilation congress. Krakow, Poland, pp 473–478
Feng KK, Chakravarty RN, Cochrane TS (1973) Spontaneous combustion: a coal mining hazard. CIM Bull: 75–84
Garcia P, Peter JH, Fanor M (1999) The use of differential scanning calorimetry to identify coals susceptible to spontaneous combustion. Thermochim Acta 336:41–46
Gouws MJ, Knotze TP (1995) Coal self-heating and explosibility. J S Afr Inst Min Metall: 37–43
Gouws MJ, Wade L (1989) The self-heating liability of coal: prediction based on simple indices. Min Sci Tech 9:75–80
Indian Standard:(1975) Methods of test for coal and coke—ultimate analysis, determination of nitrogen, pp 3–10
Indian Standard: Part-I (1979) Methods for petrographic analysis of coal, pp 5–7
Indian Standard: Part-II (1979) Methods for petrographic analysis of coal-preparation of coal samples for petrographic analysis, pp 4–8
Indian Standard:436 (1964) Methods of sampling of coal and coke, p 13
Indian Standard:Part–I (1969) Methods of test for coal and coke: proximate analysis, pp 5–18
Indian Standard:Part-III (1969) Methods of test for coal and coke—determination of sulphur, pp 5–8
Indian Standard:Part–IV/Sec 1 (1974) Methods of test for coal and coke—ultimate analysis, determination of carbon and hydrogen, pp 5–12
Iphar M (2012) ANN and ANFIS performance prediction models for hydraulic impact hammers. Tunnel Underg Space Technol 27:23–29
Jang RJ (1991a) Fuzzy modeling using generalized neural networks and Kalmman filter algorithm. In: Proceedings of ninth national conference on artificial intelligence, Anaheim, California, pp 762–767
Jang RJ (1991b) Rule extraction using generalized neural networks’. Proceedings of 4th IFSA world congress, Brussels, pp 82–86
Jang RJ (1993) ANFIS:adaptive-network-based fuzzy inference system. IEEE Trans Syst Man Cyber 23(3):665–685
Jang RJ, Gulley N (1996) Fuzzy logic toolbox: reference manual. The Math Works Inc, Massachusetts
Jang JSR, Sun CT, Mizutani E (1997) Neuro-fuzzy and soft computing, a computational approach to learning and machine intelligence, 1st edn. Prentice Hall, New Jersey
Jones RE, Townend DTA (1949) Oxidation of coal. J Soc Chem Ind 68:197
Jose JP, Puente G, De la Fuente E, Moran A, Rubiera F (1996) A study of self-heating of fresh and oxidised coal by differential thermal analysis. Thermochim Acta 279:93–101
Klassen MS, Pao YH (1988) Characteristics of the functional link net: a higher order delta rule net, In IEEE proceedings of the international conference on neural networks, San Diego
Krajčiová M, Jelemenský L, Kiša M, Markoš J (2004) Model predictions on self-heating and prevention of stockpiled coals. J Loss Prev Process Ind 17:205–216
Krishnaswamy S, Agarwal PK, Gunn RD (1996) Low-temperature oxidation of coal: modelling spontaneous combustion in coal stockpiles. Fuel 75(3):353–361
Mahadevan V, Ramlu MA (1985) Fire risk rating of coal mines due to spontaneous heating. J Mines Metals Fuels: 357–362
Mahajan OP, Tomita A, Walker PL Jr (1976) Differential scanning calorimetry studies on coal. 1. Pyrolysis in an inert atmosphere. Fuel 55:63–69
Mehrabi M, Pesteei SM, Pashaee GT (2011) Modeling of heat transfer and fluid flow characteristics of helicoidal double-pipe heat exchangers using adaptive neuro-fuzzy inference system (ANFIS). Int Comm Heat Mass Transf 38:525–532
Mellit A, Kalogirou SA (2011) ANFIS-based modelling for photovoltaic power supply system: a case study. Renewable Energy 36:250–258
Mohalik N, Singh R, Singh V, Tripathi D (2009) Critical appraisal to assess the extent of fire in old abandoned coal mine areas—Indian context. In: Proceedings of 9th coal operators’ conference, University of Wollongong and the Australasian Institute of Mining and Metallurgy, 2009, pp 271–280
Munzer A (1975) Stach’s textbook of coal petrology. In: Stach E et al. (eds) 2nd edn. Gebruder Borntraeger, Berlin, pp 387–388
Naderloo L, Alimardani R et al (2012) Application of ANFIS to predict crop yield based on different energy inputs. Measurement 45:1406–1413
Nazari A, Khalaj G (2012) Prediction compressive strength of lightweight geopolymers by ANFIS. Ceram Int 38:4501–4510
Nordon P (1979) A model for the self-heating reaction of coal and char. Fuel 58:456–464
Panigrahi DC, Sahu HB (2004) Classification of coal seams with respect to their spontaneous heating susceptibility—a neural network approach. Geotech Geol Eng 22:457–476
Panigrahi DC, Sahu HB (2005) Development of a new method for the assessment of spontaneous heating susceptibility of coal. IE(I) J MN 85:42–45
Panigrahi DC, Ojha A, Saxena NC, Kejriwal BK (1997) A study of coal oxygen interaction by using Russian U-index and its correlation with basic constituents of coal with particular reference to Jharia coalfield, proceedings of 27th international conference of safety in mines, New Delhi, India, vol I, pp 493–499
Panigrahi DC, Saxena VK, Udaybhanu G A (2000) study of susceptibility of Indian coals to spontaneous combustion and its correlation with their intrinsic properties, Proceeding 1st international conference on mine environment and ventilation, Dhanbad, India, pp 347–353
Panigrahi DC, Sahu HB, Udayabhanu G, Saxena VK (2004) Wet oxidation method for predicting the spontaneous heating susceptibility of Indian coals. IICM June–Aug, pp 13–21
Panigrahi DC, Sahu HB, Mishra NM (2008) Development of a spontaneous heating liability index of coal from kinetic parameters. CIM Bull 3(4):71–77
Pao YH (1989) Adaptive pattern recognition and neural network, 1st edn. Addison Wesley Publishing Company, Massachusetts
Peters WC (1978) Exploration and mining geology. Wiley, New York
Pradhan B, Sezer EA, Gokceoglu C, Buchroithner MF (2010) Landslide susceptibility mapping by neuro-fuzzy approach in a landslide-prone area (Cameron Highlands, Malaysia). IEEE Trans Geosci Remote Sens 48(12):4164–4177
Ren TX, Richards MJ (1994) A computerised system for the study of the spontaneous combustion of coal. Min Eng: 121–127
Rigby LS (1983) Self heating can be understood and controlled. J. Coal Qual 2:16–20
Rumelhart DE, Hinton GE, William DE (1986) Learning internal representations by error propagation, In: DE Rumelhart, JL McClelland (eds) Parallel distributed processing: explorations in the microstructure of cognition, The MIT Press, Cambridge, 1–8, pp 318–362
Saghafi A, Carras JN (1997) Modelling of spontaneous combustion in underground coal mines: application to a gassy longwall panel. Proceedings of 27th international conference of safety in mines, New Delhi, vol II, pp 1207–1214
Sahu HB, Panigrahi DC, Mishra NM (2004) Assessment of spontaneous heating susceptibility of coal seams by differential scanning calorimetry. J Mines Metals Fuels 52(7&8):117–121
Sahu M, Mahapatra SS, Sahu HB, Patel RK (2011) Prediction of water quality index using neuro fuzzy inference system. Water Qual Expo Health 3:175–191
Schmal D, Duyzer H, Van Heuven JW (1985) A model for the spontaneous heating of coal. Fuel 64:963–972
Serge G (2001) Designing fuzzy inference systems from data: interpretability oriented review. IEEE Trans Fuzzy Syst 9(3):426–442
Singh RN, Demirbilek S (1987) Statistical appraisal of intrinsic factors affecting spontaneous combustion of coal. Min Sci Tech 4(2):155–165
Singh J, Singh SG (2010) Modeling for tensile strength of friction welded aluminium pipes by ANFIS. Intell Eng Inform 1(1):3–20
Smith AC, Moron Y, Lazzara CP (1991) Large scale studies of spontaneous combustion of coal. US Bureau of Mines Report of Investigation 9346, pp 1–30
Sobhani J, Najimi M, Pourkhorshidi AR, Parhizkar T (2010) Prediction of the compressive strength of no-slump concrete: a comparative study of regression, neural network and ANFIS models. Construct Build Mater 24:709–718
Stach E, Machowsky MH et al (1982) Stach’s textbook of coal petrology, 3rd edn. Gebruder Borntraeger, Berlin
Stracher GB, Taylor TP (2004) Coal fires burning out of control around the world: thermodynamic recipe for environmental catastrophe. Int J Coal Geol 59:7–17
Sugeno M, Kang GT (1988) Structure identification of fuzzy model. Fuzzy Sets Syst 28:15–33
Takagi T, Sugeno M (1985) Fuzzy identification of systems and its applications to modeling and control. IEEE Trans Syst Man Cyber 15:116–132
Tarafdar MN, Guha D (1989) Application of wet oxidation processes for the assessment of the spontaneous heating of coal. Fuel 68:315–317
Wang H, Dlugogorski BZ, Kennedy EM (2003) Coal oxidation at low temperatures: oxygen consumption, oxidation products, reaction mechanism and kinetic modeling. Progress Energy Combust Sci 29:487–513
Werbos P (1974) Beyond regression: new tools for prediction and analysis in the behavioral sciences, Ph.D. Dissertation, Harvard University, United States
Xie J, Xue S, Cheng W, Wang G (2011) Early detection of spontaneous combustion of coal in underground coal mines with development of an ethylene enriching system. Int J Coal Geol 85:123–127
Yilmaz I, Kaynar O (2011) Multiple regression, ANN (RBF MLP) and ANFIS models for prediction of swell potential of clayey soils. Expert Syst Appl 38:5958–5966
Yohe GR, Harman CA (1941) Oxidising power of Illinois coal. J Am Chem Soc 63:555
Yuan L, Smith A (2008) Numerical study on effects of coal properties on spontaneous heating in longwall gob areas. Fuel 87:3409–3419
Zahariev Z, Christov Ch, Krougliy V, Batchvarov D (2001) Installation for evaluation of spontaneous combustion tendency of solid fuels and materials in the adiabatic conditions. In: Proceedings 7th international mine ventilation congress, Krakow, Poland, pp 505–507
Zhou F, Wang D (2004) Directory of recent testing methods for the propensity of coal to spontaneous heating. J Fire Sci 22:91–96
Zubíček V (2008) Assessment of susceptibility of coal to spontaneous combustion in OKR. Geo Sci Engg LIV(4):1–9
Acknowledgments
The authors are thankful to the Director of National Institute of Technology, Rourkela for his permission to publish this paper. The views expressed are of the authors and not necessarily of the organisations to which they belong. The authors are also thankful to the anonymous reviewers for their suggestions, which have helped in improving the technical content of the paper.
Author information
Authors and Affiliations
Corresponding author
Rights and permissions
About this article
Cite this article
Sahu, H.B., Mahapatra, S.S. Forecasting Spontaneous Heating Susceptibility of Indian Coals Using Neuro Fuzzy System. Geotech Geol Eng 31, 683–697 (2013). https://doi.org/10.1007/s10706-013-9618-6
Received:
Accepted:
Published:
Issue Date:
DOI: https://doi.org/10.1007/s10706-013-9618-6