Abstract
Disagreement among policymakers often involves policy issues and differences between the decision makers’ implicit utility functions. Significant disagreement can also exist concerning conceptual models of the physical system. Disagreement on the validity of a single simulation model delays discussion on policy issues and prevents the adoption of consensus management strategies. For such a contentious situation, the proposed multi-conceptual model optimization (MCMO) can help stakeholders reach a compromise strategy. MCMO computes mathematically optimal strategies that simultaneously satisfy analogous constraints and bounds in multiple numerical models that differ in boundary conditions, hydrogeologic stratigraphy, and discretization. Shadow prices and trade-offs guide the process of refining the first MCMO-developed `multi-model strategy into a realistic compromise management strategy. By employing automated cycling, MCMO is practical for linear and nonlinear aquifer systems. In this reconnaissance study, MCMO application to the multilayer Cache Valley (Utah and Idaho, USA) river–aquifer system employs two simulation models with analogous background conditions but different vertical discretization and boundary conditions. The objective is to maximize additional safe pumping (beyond current pumping), subject to constraints on groundwater head and seepage from the aquifer to surface waters. MCMO application reveals that in order to protect the local ecosystem, increased groundwater pumping can satisfy only 40 % of projected water demand increase. To explore the possibility of increasing that pumping while protecting the ecosystem, MCMO clearly identifies localities requiring additional field data. MCMO is applicable to other areas and optimization problems than used here. Steps to prepare comparable sub-models for MCMO use are area-dependent.
Resumé
Le désaccord parmi les décideurs politiques implique souvent des questions de police de l’eau et des différences entre les fonctions d’utilité implicites des décideurs. Un désaccord significatif peut aussi porter sur les modèles conceptuels des systèmes physiques. Le désaccord sur la validité d’un modèle unique de simulation retarde la discussion sur les problèmes de politique de l’eau et empêche l’adoption de stratégies consensuelles de gestion. Pour une telle situation litigieuse, l’optimisation proposée de modèles multi-conceptuels (OMMC) peut aider les décideurs à dégager une stratégie consensuelle. OMMC calcule les stratégies optimales qui satisfont simultanément les contraintes analogues et relie la stratigraphie hydrogéologique et la discrétisation dans des modèles numériques multiples qui différent par les conditions aux limites. Des coûts cachés et des compromis guident le processus d’affinage de la première stratégie multi-modèle développée vers une stratégie réaliste de gestion des compromis. En employant un cycle automatisé, OMMC est adapté pour les systèmes aquifères linéaires et non linéaires. Dans cette étude test, l’application d’OMMC au système aquifère multi-couches-rivière de Cache Valley (Utah et Idaho, Etats Unis d’Amérique) emploie deux modèles de simulation avec des conditions analogues mais différant par la discrétisation verticale et les conditions aux limites. L’objectif est de maximiser des pompages d’appoint (en deçà des pompages actuels), susceptibles d’impacter la charge hydraulique de l’aquifère et les écoulements de l’aquifère vers les eaux de surface. L’application d’OMMC révèle que l’accroissement du pompage d’eau souterraine destiné à protéger l’écosystème local peut satisfaire seulement 40 % de l’augmentation projetée de la demande en eau. Pour explore la possibilité d’accroître ce pompage en protégeant l’écosystème, OMMC identifie clairement les emplacements nécessitant des données additionnelles de terrain. OMMC est applicable à d’autres secteurs et problèmes d’optimisation que ceux utilisés dans ce cas d’étude. Les étapes de préparation de sous-modèles comparables pour l’utilisation d’OMMC dépendent du terrain.
Resumen
El desacuerdo entre los funcionarios a menudo implica temas de política y diferencias entre las funciones de utilidad implícitas de los tomadores de decisiones. También puede existir un desacuerdo significativo en relación con los modelos conceptuales del sistema físico. El desacuerdo en la validez de un único modelo de simulación retrasa el debate sobre temas políticos y dificulta la adopción de estrategias de manejo consensuadas. Para tal situación conflictiva, la optimización del modelo multi-conceptual propuesto (MCMO) puede ayudar a las partes interesadas para alcanzar una estrategia de compromiso. MCMO calcula matemáticamente las estrategias óptimas que satisfagan simultáneamente las restricciones análogas y limita los modelos numéricos múltiples que difieren en las condiciones límites, estratigrafía hidrogeológica y discretización. Los precios ocultos y las compensaciones guían el proceso de refinamiento de la primera estrategia multi-modelo-MCMO convertida en una estrategia realista de manejo de compromiso. Mediante el empleo de la ciclicidad automática, el MCMO es práctico para los sistemas acuíferos lineales y no lineales. En este estudio de reconocimiento, la aplicación del MCMO al sistema multicapa acuífero–rio del Cache Valley (Utah e Idaho, EEUU) emplea dos modelos de simulación con análogas condiciones de fondo pero diferente discretización vertical y condiciones de contorno. El objetivo es maximizar el bombeo seguro adicional (más allá de bombeo actual), sujeto a las limitaciones en la carga hidráulica de las aguas subterráneas y las filtraciones desde el acuífero hacia las aguas superficiales. La aplicación MCMO revela que con el fin de proteger el ecosistema local, el aumento de bombeo de agua subterránea puede satisfacer sólo el 40 % del aumento proyectado de la demanda de agua. Para explorar la posibilidad de incrementar el bombeo y al mismo tiempo proteger el ecosistema, MCMO identifica claramente localidades que requieren datos de campo adicionales. MCMO es aplicable a otras áreas y a problemas de optimización que se utilizan aquí. Los pasos para preparar sub-modelos comparables para el uso MCMO son dependiente del área.
خلاصة
ينطوي الخلاف بين واضعي السياسة غالباً على أمور السياسات والاختلافات بين وجهات النظر الضمنية تبعاً لعمل كل فرد من صانعي القرار. كما يمكن أن توجد خلافات كبيرة أيضا فيما يتعلق بالنماذج المفاهيمية للنظام الفعلي. تؤَّخر قضايا الخلاف في صحة إحدى النماذج النقاش حول السياسة وتمنع اعتماد استراتيجيات توافقية لإدارة المياه. التحسين المقترح لهذه الحالة المثيرة للجدل هو نموذج مفاهيم متعددة (MCMO) الذي يمكن أن يساعد الأطراف المعنية التوصل إلى استراتيجية توفيقية. يقوم (MCMO) بحساب الحل الأمثل رياضيا بين الاستراتيجيات التي تلبي في الوقت نفسه قيودا مماثلة، وحدودا في العديد من النماذج العددية التي تختلف في شروط الحدود وتحديد دراسة طبقات الأرض، والتفريد (Discretization). توجه أسعار الظل Shadow prices)) والمقايضات (Trade-offs) عملية صقل الاستراتيجية النموذجية المتعددة التي حسبت أصلاً لتسوية الاستراتيجية الإدارية وجعلها واقعية. عن طريق استخدام الدورات الآلية (Automated cycling)، (MCMO) هو عملي لنظم المياه الجوفية الخطية (Linear) وغير الخطية. في هذه الدراسة اﻻستطلاعية، تم تطبيق (MCMO) على وادي (Cache Valley) المكون من مياه سطحية وجوفية متعددة الطبقات (ولايتي يوتا وإيداهو، الولايات المتحدة الأمريكية) وتم استخدام اثنين من النماذج المفاهيمية التي تختلف في التفريد الرأسي وشروط الحدود. الهدف هنا هو الزيادة الآمنة للضخ الأقصى (إضافة للضخ الحالي)، رهنا بالقيود على رأس المياه الجوفية والتقلّص في تسرب المياه الجوفية إلى المياه السطحية. يكشف التطبيق هذا أنه بغية حماية النظام الإيكولوجي المحلي، الزيادة المثلى في ضخ المياه الجوفية يمكن أن يرضي فقط 40 % من الزيادة المتوقعة في الطلب على المياه. لاستكشاف إمكانية زيادة هذا الضخ بالتزامن مع حماية النظام الإيكولوجي، يحدد (MCMO) بوضوح الأماكن التي تتطلب بيانات ميدانية إضافية. ينطبق (MCMO) أيضا على مناطق أخرى ومشاكل تحسين غير التي استخدمت في هذه الدراسة. إن الخطوات ﻹعداد النماذج الفرعية القابلة للمقارنة لاستخدام (MCMO) تعتمد على المنطقة.
摘要
决策人中的意见不一致经常包括政策问题和决策人固有公用功能之间的差别。实体系统的概念模型也存在着重大的不一致。单一模拟模型有效性的不一致延误政策问题的讨论,妨碍一致同意管理策略的采纳。针对这样有争议的情景,所提出的多概念模型最优化可以帮助利益相关者达到一个妥协的策略。多概念模型最优化能够精确地计算最优化策略,同时满足边界条件、水文地质地层和识别值有所不同的多重数值模型中的类似约束条件及界限。影子价格及取舍指导着把多模型策略提高成实事求是的妥协管理策略。通过采用自动化循环,多概念模型最优化对于线性和非线性含水层系统非常实用。在这项调查研究中,多概念模型最优化在(美国犹他州和爱达荷州)Cache 河谷多层河流-含水层系统中的应用采用了两个模拟模型,两个模拟模型背景条件类似,但垂直识别值和边界条件不同。目的就是在受限于地下水水头和含水层渗漏到地表水体的约束条件下,使额外的安全抽水量(超出目前的抽水量)增加到最大量。多概念模型最优化的应用显示,为了保护当地的生态系统,增加的地下水抽水量只能满足预测的水需求增加量的40%。为了探索在保护生态系统的前提下增加抽水量的可能性,多概念模型最优化清晰地识别出需要额外野外数据的地区。多概念模型最优化适用于其他地区,也适用于除了这里提到的最优化问题之外的其它最优化问题。在使用多概念模型最优化中,准备可比较的分模型的步骤取决于各自地区的具体情况。
Abstrak
Ketidaksepakatan di antara pembuat kebijakan sering melibatkan isu dalam pengambilan keputusan dan perbedaan preferensi dalam hal strategi manajemen air. Ketidaksepakatan yang substansial juga bisa terjadi mengenai keakuratan model konseptual terhadap sitem aktual. Keraguan atas validitas model simulasi tunggal menyebabkan tertundanya pembahasan isu-isu kebijakan dan mencegah tercapainya konsensus dalam strategi manajemen. Pada situasi dimana kesepakatan sulit dicapai, teknik Multi-Conceptual Model Optimization (MCMO), yang artinya optimasi secara multi model konseptual, dapat membantu para pemangku kepentingan mencapai strategi yang dapat disepakati bersama. Secara matematis, MCMO menghitung strategi optimal dari beberapa model numerik yang memiliki constraint and boundary conditions yang sama secara simultan. Model-model numerik ini memiliki boundary condition, stratigrafi hidrogeologi, dan metode diskretisasi yang berbeda. Shadow price dan trade-off memandu proses penyempurnaan strategi multi-model menjadi strategi manajemen yang realistis dan dapat dikompromikan. Dengan menggunakan automatic cycling, MCMO dapat digunakan untuk akuifer linear maupun nonlinear. Dalam study kasus untuk sistem sungai–akuifer berlapis di Lembah Cache (Utah dan Idaho, Amerika Serikat), MCMO digunakan untuk dua model simulasi dengan kondisi latar belakang yang sama, tetapi berbeda dalam diskretisasi vertikal dan boundary condition. Tujuannya adalah untuk memaksimalkan safe pumping yield (diluar pumping yield saat ini), berdasarkan constraint untuk tinggi muka air tanah dan rembesan dari akuifer ke air permukaan. Pengaplikasian MCMO memperlihatkan bahwa guna melindungi ekosistem lokal, peningkatan pemompaan air tanah hanya dapat memenuhi 40 % dari proyeksi kenaikan kebutuhan air. Untuk menjajaki kemungkinan peningkatan pumping yield tersebut dan sekaligus melindungi ekosistem, MCMO mengidentifikasi secara spesifik daerah yang membutuhkan data lapangan tambahan. MCMO dapat digunakan pada daerah dengan masalah optimasi yang berbeda dari yang digunakan di sini. Proses dalam mempersiapkan sub-model MCMO yang sesuai untuk digunakan sangat tergantung pada kondisi daerah tersebut.
Riassunto
Disaccordo fra politici spesso implica questioni politiche e differenze tra le funzioni di utilità implicite dei decisori. Un Disaccordo significativo può esistere anche in merito ai modelli concettuali del sistema fisico. Disaccordo sulla validità di un unico modello di simulazione ritarda la discussione sulle questioni politiche e impedisce l’adozione di strategie per la gestione consensuale. A causa di una tale situazione contenziosa, il modello di ottimizzazione multi-concettuale (MCMO) proposto può aiutare gli azionisti a raggiungere un compromesso strategico. Il MCMO calcola matematicamente strategie ottimali che simultaneamente soddisfano confini analoghi legati a svariati modelli numerici che differiscono nelle condizioni limite, stratigrafia idrogeologica, e discretizzazione. Prezzi ombra e compromessi guidano il processo di perfezionamento della prima strategia multi modello del MCMO in un compromesso di strategia realistica di gestione. La ciclicità automatizzata, è un metodo pratico per l’utilizzo dei sistemi di falde acquifere lineari e non lineari. In questo studio esplorativo, l’applicazione MCMO, nel sistema fiume-acquifero multistrato di Cache Valley (Utah e Idaho, USA), utilizza due modelli con condizioni di fondo analoghe ma con diverse discretizzazioni verticali e condizioni di limite. L’obiettivo è di sfruttare al massimo, con tutta sicurezza, ulteriori pompaggi (in aggiunta a quelli attuali), soggetti ai limiti del carico piezometrico dell’acqua di falda ed infiltrazione della stessa sulle acque di superficie. L’impiego del MCMO rivela che per proteggere l’ecosistema locale, l’incremento del pompaggio delle acque di falda può soddisfare soltanto il 40% della domanda d’aumento prevista. Nell’esplorare la possibilità di aumentare il pompaggio, proteggendo allo stesso tempo l’ecosistema, MCMO indentifica chiaramente le località che necessitano ricerche addizionali. MCMO è applicabile ad altri soggetti e problemi di ottimizzazione, a parte quelli sopra discussi. I passi per preparare sub-modelli adatti all’uso del MCMO, sono specificamente adattati per l’uso della località di provenienza.
چکیده
عدم توافق بین سیاست گذران اغلب ناشی از مسائل مربوط به سیاستگذاریها و یا تفاوتهای ضمنی بین وظایف تصمیم گیرندگان میباشد. همچنین ممکن است در رابطه با چندین مدل مفهومی مفروض برای یک سیستم فیزیکی خاص، عدم توافق قابل ملاحظهای وجود داشته باشد. عدم توافق بر روی اعتبار یک مدل شبیه سازی بخصوص ، به عنوان مدل مرجع، باعث به تاخیرافتادن تبادل افکار بر روی مسائل مربوط به سیاستگذاریها شده و از حصول یک راه حل مورد تفاهم جلوگیری میکند. در این شرایط چالش برانگیز، روش پیشنهادی در این مطالعه با عنوان بهینه سازی سیستمهای با چندین مدل مفهومی (MCMO) به ذینفعان کمک میکند تا به یک راه حل مورد پذیرش طرفین برسند. MCMO راه حلهای بهینه ریاضیاتی را محاسبه میکند، راه حلهایی که به طور همزمان محدودیتها و قیود متناظر در چندین مدل عددی (که ممکن هست در شرایط مرزی، چینه بندی هیدروژئولوژیکی و یا نحوه شبکه بندی (ِDiscretization) با هم متفاوت باشند) را ارضا میکند. قیمتهای سایه و بده بستانها (Trade-Offs) وظیفه بهبود اولیل راه حل حاصل از MCMO به یک راه حل نهایی مورد پذیرش واقعبینانه را به عهده دارند. با به کارگیری بهینه سازی پی در پی (Cycling)، MCMO قابلیت استفاده در سیستمهای آبخوان خطی و غیر خطی را داراست. در این مطالعه اکتشافی، برای استفاده از MCMO بر روی سیستم چند لایه ای آبخوان- رودخانه در Cache Valley در ایالات یوتا و آیداهو درآمریکا، دو مدل شبیه سازی با شرایط پسزمینه مشابه ولی با شبکه بندی عمودی و شرایط مرزی متفاوت بکار گرفته میشوند. هدف مطالعه بیشینه کردن اضافه برداشت از آب زیرزمینی (بیش از بهره برداری فعلی) با در نظر گرفتن قیودی از قبیل کنترل سطح آب زیرزمینی و کنترل مقدار نشت از آبخوان به آبهای سطحی میباشد. استفاده از MCMO نشان میدهد که برای محافظت از اکوسیستم منطقه، اضافه برداشت از آب زیر زمینی میتواند فقط ۴۰% از اضافه آب مورد نیاز برآورد شده را تامین کند. برای بررسی امکان افزایش بهرهبرداری از آبهای زیرزمینی همزمان با محافظت از اکو سیستم، MCMO مناطقی که احتیاج به دادههای میدانی بیشتری دارند را به طور واضح تعیین میکند. غیر از روش مورد استفاده در این تحقیق، MCMO قابلیت استفاده در مناطق دیگر و همچنین دیگر مسائل بهینه سازی را داراست. گامهای لازم برای آماده کردن مدلهای مورد استفاده در MCMO بستگی به منطقه مورد مطالعه دارند.
Resumo
Divergências entre legisladores geralmente envolvem questões políticas e diferenças entre as funções de utilidade implícitas aos tomadores de decisão. Divergências significativas também podem existir quanto a modelos conceituais do sistema físico. Desacordo sobre a validade de um simples modelo simulado adia a discussão sobre questões de política e impede a adoção de estratégias de gestão consensuais. Para uma situação tão controversa, a otimização de modelos multiconceitual (OMMC) proposta pode ajudar os interessados a chegar a uma estratégia de consenso. OMMC calcula matematicamente estratégias ótimas que satisfazem simultaneamente restrições análogas e limites em modelos numéricos múltiplos que diferem em condições de contorno, estratigrafia hidrogeológica, e discretização. Preços sombra (shadow prices) e permutações (trade-offs) orientam o processo de refinamento da primeira estratégia multimodelo OMMC desenvolvida na direção de uma estratégia de gestão consensual realista. Com o emprego de ciclos automatizados, OMMC é útil para sistemas aquíferos lineares e não lineares. Nesse estudo exploratório, a aplicação da OMMC ao sistema rio–aquífero multicamadas do Vale Cache (Utah e Idaho, EUA) emprega dois modelos de simulação com condições de conhecimento análogas mas diferentes discretizações verticais e condições de contorno. O objetivo é maximizar bombeamentos seguros adicionais (além dos bombeamentos em curso), sujeitos a limitações de potencial hidráulico e descarga do aquífero às águas superficiais. A aplicação da OMMC revela que, a fim de proteger o ecossistema local, um aumento no bombeamento das águas subterrâneas pode satisfazer apenas 40 % do aumento da demanda de água projetada. Para explorar a possibilidade de aumentar esse bombeamento e ao mesmo tempo proteger o ecossistema, OMMC identifica claramente localidades que requerem dados de campo adicionais. OMMC é aplicável a outras áreas e problemas de otimização além do utilizado aqui. Passos para preparar submodelos comparáveis para uso OMMC são dependentes da área.










Similar content being viewed by others
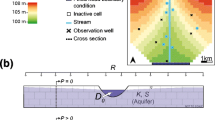
References
Ahlfeld DP, Mulligan AE (2000) Optimal management of flow in groundwater systems. Academic, San Diego, CA
Ahlfeld DP, Baker KM, Barlow PM (2009) GWM-2005: a groundwater-management process for MODFLOW-2005 with local grid refinement (LGR) capability. US Geol Surv Techniques Methods 6-A33, 65 pp
Ajami NK, Duan Q, Gao X, Sorooshian S (2006) Multimodel combination techniques for analysis of hydrological simulations: application to distributed model intercomparison project results. J Hydrometeorol 7:755–768
Aly AH, Peralta RC (1999) Comparison of a genetic algorithm and mathematical programming to the design of groundwater cleanup system. Water Resour Res 35(8):2415–2426
American Society of Civil Engineers (ASCE) (1987) Ground water management, 3rd edn. ASCE manuals and reports on engineering practice no. 40. ASCE, New York
ASTM Standard D 5981-96 (2007) Standard guide for calibrating a ground-water flow model application. ASTM, West Conshohocken, PA
Berard S, Trinkle J, Nguyen B, Roghani B, Fink J, Kumar V (2007) daVinci Code: a multi-model simulation and analysis tool for multi-body systems. Proceedings 2007 I.E. International Conference on Robotics and Automation, Rome, April 2007, pp 2588–2593. doi:10.1109/ROBOT.2007.363855
Das R (2002) Planning sustainable optimal groundwater yield for the Utah part of Cache Valley. MSc Thesis, Utah State University, Logan, UT, USA, 123 pp
Das Gupta A, Nobi N, Paudyal GN (1996) Ground-water management model for an extensive multiaquifer system and an application. Ground Water 34(2):349–357
De Paly M, Bürger CM, Bayer P (2013) Optimization under worst case constraints: a new global multimodel search procedure. Struct Multidiscip Optim 48:1153–1172. doi:10.1007/s00158-013-0950-5
Enzenhoefer R, Nowak W, Helmig R (2012) Probabilistic exposure risk assessment with advective–dispersive well vulnerability criteria. Adv Water Resour 36:121–132. doi:10.1016/j.advwatres.2011.04.018
Eyring V, Stevenson DS, Lauer A, Dentener FJ, Butler T, Collins WJ et al (2007) Multi-model simulations of the impact of international shipping on atmospheric chemistry and climate in 2000 and 2030. Atmos Chem Phys 7(3):757–780. doi:10.5194/acp-7-757-2007
Faure F, Duriez C, Delingette H, Allard J, Gilles B, Marchesseau S, Talbot H et al (2012) SOFA: a multi-model framework for interactive physical simulation. Stud Mechanobiol Tissue Eng Biomater 11:283–321. doi:10.1007/8415_2012_125
Fishwick PA, Narayanan NH, Sticklen J, Bonarini A (1994) A multimodel approach to reasoning and simulation. IEEE Trans Syst Man Cybern 24(10):1433–1449
Godding GW (2008) A multi-modeling approach using simulation and optimization for supply-chain network systems. PhD Thesis, Arizona State University, Tempe, AZ, USA, 202 pp
Gorelick SM (1982) A review of distributed parameter groundwater management modeling methods. Water Resour Res 19(2):305–319
Gorelick SM (1990) Large scale nonlinear deterministic and stochastic optimization: formulations involving simulation of subsurface contamination. Math Program 48(1–3):19–39
Greenwald R (1998) Documentation and user’s guide: MODMAN, an optimization module for MODFLOW, ver 4.0. HSI GeoTrans, Freehold, NJ, 112 pp
Guber AK, Pachepsky YA, van Genuchten TM, Rowland RA, Nicholson TJ, Cady RE (2009) Multimodel simulation of water flow: uncertainty analysis. Geophys Res Abstr 11, EGU2009-6539
Haimes YY (2012) Modeling complex systems of systems with phantom system models. Syst Eng 15(3):333–346. doi:10.1002/sys.21205
Halford KJ (2006) MODOPTIM: a general optimization program for ground-water flow model calibration and ground-water management with MODFLOW. US Geol Surv Sci Invest Rep 2006-5009, 71 pp
Harbaugh AW (2005) MODFLOW–2005, the U.S. Geological Survey modular ground-water model: the ground-water flow process. US Geol Surv Techniques Methods 6-A16
Harbaugh AW, Banta ER, Hill MC, McDonald MG (2000) MODFLOW–2000, the U.S. Geological Survey modular ground-water model: user guide to modularization concepts and the ground-water flow process. US Geol Surv Open-File Rep 00-92
Heidari M (1982) Application of linear system’s theory and linear programming to ground water management in Kansas. Water Resour Bull 18(6):1003–1012
Izaguirre JA, Chaturvedi R, Huang C, Cickovski T, Coffland J, Thomas G, Forgacs G, Alber M, Hentschel G, Newman SA, Glazier JA (2004) CompuCell, a multi-model framework for simulation of morphogenesis. Bioinformatics 20(7):1129–1137. doi:10.1093/bioinformatics/bth050
Kalwij I, Peralta R (2006) Simulation/optimization modeling for robust pumping strategy design. Groundwater 44(4):574–582
Kariya KA, Roark MD, Hanson KM (1994) Hydrology of Cache Valley, Cache County, Utah and adjacent part of Idaho, with emphasis on simulation of ground-water flow. Technical Publ. 108, Utah Department of Natural Resources, Salt Lake City, UT, 120 pp
Lachmar TE, Myers B, Robinson M (2004) Updated conceptual MODFLOW groundwater models of Cache Valley, Utah and Idaho. In: Ground water in Utah: resource, protection, and remediation. UGA Publ. 31, Utah Geological Association, Salt Lake City, UT, pp 43–58
Maddock T (1974) Nonlinear technological functions for aquifers whose transmissivities vary with drawdown. Water Resour Res 10(3):877–881
McDonald MG, Harbaugh AW (1988) A modular three-dimensional finite-difference ground water flow model. US Geol Surv Tech Water-Resources Invest, Book 6, Chapter A1, USGS, Reston, VA
Miao C, Duan Q, Sun Q, Li J (2013) Evaluation and application of Bayesian multi-model estimation in temperature simulations. Prog Phys Geogr 37(6):727–744. doi:10.1177/0309133313494961
Mishra S, Deeds N, Ruskauff G (2009) Global sensitivity analysis techniques for probabilistic ground water modeling. Ground Water 47(5):730–747. doi:10.1111/j.1745-6584.2009.00604.x
Morel-Seytoux HJ (1975) A simple case of conjunctive surface groundwater management. Ground Water 13(6):506–515
Myers BR (2003) Simulation of groundwater flow in Cache Valley, Utah and Idaho. MSc Thesis, Utah State University, Logan, UT, USA, 89 pp
Peralta RC, Kalwij I (2012) Groundwater optimization handbook: flow, contaminant transport, and conjunctive management. International Water Association, London
Peralta RC, Timani B, Das R (2011) Optimizing safe yield policy implementation. J Water Resour Manag 25:483–508
Peterson W (1946) Ground water supply in Cache Valley, Utah, available for domestic use and irrigation. Utah State Agricultural College Extension Service no. 133, USES, Logan, UT, 59 pp
Poeter EP, Hill MC (2007) MMA: a computer code for multi-model analysis. US Geol Surv Techniques Methods 6-E3, 125 pp
Rajabi MM, Ataie-Ashtiani B (2014) Sampling efficiency in Monte Carlo based uncertainty propagation strategies: application in seawater intrusion simulations. Adv Water Resour 67:46–64. doi:10.1016/j.advwatres.2014.02.004
Ranjha AY, Peralta RC, Yazdanian A (1990) Conjunctive water use/sustained groundwater yield planning: case history. ICID Bull 39(1):1–12
Renard B, Kavetski D, Leblois E, Thyer M, Kuczera G, Franks SW (2011) Toward a reliable decomposition of predictive uncertainty in hydrological modeling: characterizing rainfall errors using conditional simulation. Water Resour Res 47(11). doi:10.1029/2011WR010643
Robinson JM (1999) Chemical and hydrostratigraphic characterization of groundwater and surface water interaction in Cache Valley. MSc Thesis, Utah State University, Logan, UT, 184 pp
Scherer RJ, Schapke S-E (2011) A distributed multi-model-based management information system for simulation and decision-making on construction projects. Adv Eng Inform 25(4):582–599. doi:10.1016/j.aei.2011.08.007
Sepúlveda N, Doherty J (2014) Uncertainty analysis of a groundwater flow model in east-central Florida. Ground Water. doi:10.1111/gwat.12232
Slater AG, Bohn TJ, McCreight JL, Serreze MC, Lettenmaier DP (2007) A multimodel simulation of pan-Arctic hydrology. J Geophys Res 112(G4), G04S45. doi:10.1029/2006JG000303
Systems Simulation/Optimization Laboratory (SSOL) (2009) SOMOS users manual. SSOL, Utah State University, Logan, UT, 470 pp
Takahashi S, Peralta RC (1995) Optimal perennial yield planning for complex non-linear aquifers: methods and examples. Adv Water Resour 18:49–62
Timani B, Peralta RC (2009) Hydraulic conductivity maps of newly calibrated Cache Valley simulation model. Draft SSOL report, SSOL, Utah State University, Logan, UT
Wagner BJ, Gorelick SM (1989) Reliable aquifer remediation in the presence of spatially variable hydraulic conductivity: from data to design. Water Resour Res 25(10):2211–2225. doi:10.1029/WR025i010p02211
Waterloo Hydrogeologic Software (2008) Visual MODFLOW v. 4.3.0.154 user’s manual. Waterloo Hydrogeologic, Waterloo, ON
Yakirevich A, Pachepsky YA, Gish TJ, Guber AK, Kuznetsov MY, Cady RE, Nicholson TJ (2013) Augmentation of groundwater monitoring networks using information theory and ensemble modeling with pedotransfer functions. J Hydrol 501:13–24. doi:10.1016/j.jhydrol.2013.07.032
Zhu R, Zheng H, Wang E, Zhao W (2013) Multi-model ensemble simulation of flood events using Bayesian model averaging. In: Piantadosi J, Anderssen RS, Boland J (eds) 20th International Congress on Modelling and Simulation. Modelling and Simulation Society of Australia and New Zealand, Adelaide, Australia, pp 455–461
Acknowledgements
We gratefully acknowledge the support of the Utah Agricultural Experiment Station. This research was supported by the Utah Agricultural Experiment Station, Utah State University, and approved as journal paper number UAES 8717. We are grateful for the continuous support of Dr. Ineke Kalwij.
Author information
Authors and Affiliations
Corresponding author
Additional information
Published in the theme issue “Optimization for Groundwater Characterization and Management
Appendix: Acronymns and defined terms
Appendix: Acronymns and defined terms
- ASTM:
-
American Standards of Testing and Materials
- BG:
-
Background
- CEC:
-
Convolution-equation computed (state variable)
- CG:
-
Coefficient generation
- convsm :
-
DV units conversion coefficient applied to sub-model sm flows
- CPB:
-
Candidate pumping block
- CU:
-
Coefficient utilization
- DASG:
-
Drain-aquifer seepage group
- DV:
-
Decision variable
- F:
-
Formulation
- GASG:
-
GHB-aquifer seepage group
- GASG1:
-
GHB cells representing surface waters inside the study area
- GASG2:
-
GHB cells at the periphery of the study area
- GHB:
-
General head boundary (conditions)
- h ô,sm :
-
Aquifer potentiometric surface elevation (head) at observation location ô belonging to sub-model sm at equilibrium
- HCL:
-
Head control location
- IC:
-
Influence coefficient
- (L,R,C):
-
A particular location (layer, row, column) combination used to identify a cell within the finite difference grid of a simulation model
- M hsm :
-
Total number of head control locations defined in sub-model sm
- M GDASsm :
-
Number of drain seepage groups
- M GRASsm :
-
Number of river seepage groups
- M GGASsm :
-
Number of GHB seepage groups
- M p :
-
Number of candidate managed groundwater extraction locations or pumping locations
- M Psm :
-
Number of managed groundwater pumping locations in sub-model sm
- MCMO:
-
Multi-conceptual model optimization
- p ê :
-
Managed DV pumping rate at location ê
- p ê,NDM :
-
Town ê pumping rate in sub-model NDM
- p ê,sm :
-
Extraction rate from well ê belonging to sub-model sm
- p ê,USGS :
-
Town ê pumping rate in sub-model USGS
- qDAS GL û,sm :
-
Net seepage rate between aquifer and groups of drain cells
- qRAS GL û,sm :
-
Net seepage rate between aquifer and groups of river cells
- qGAS GL û,sm :
-
Net seepage rate between aquifer and groups of GHB cells
- RASG:
-
River–aquifer seepage group
- RMM:
-
Response matrix method
- SC:
-
Simulator-computed state variable value
- sm:
-
Sub-model (component of an MCMO model)
- SM:
-
Total number of sub-models used within MCMO
- S-O:
-
Simulation-optimization
- Superscripts L and U:
-
Lower and upper bounds on a variable value, respectively
- SV:
-
State variable
- USGS:
-
United States Geological Survey
- Z :
-
Objective function value
- (ê, NDM):
-
DV index numbers in the NDM sub-model
- (ê, USGS):
-
DV index numbers in the USGS sub-model
- Δ demand 2020 ê :
-
Projected increase in water demand by year 2020
- δ ψp ô,ê :
-
SV (Ψ) influence coefficient describing Ψ response at location ô at equilibrium to a unit pumping (p ut ê ) at well ê
- Ψ ô :
-
SV value at observation location ô at equilibrium
- Ψ non ô :
-
Background (non-optimal) SV value at observation location ô at equilibrium
Rights and permissions
About this article
Cite this article
Timani, B., Peralta, R. Multi-model groundwater-management optimization: reconciling disparate conceptual models. Hydrogeol J 23, 1067–1087 (2015). https://doi.org/10.1007/s10040-015-1259-9
Received:
Accepted:
Published:
Issue Date:
DOI: https://doi.org/10.1007/s10040-015-1259-9