Abstract
Mesh membranes are widely used in nebulizers for inhalation therapy in the medical industry and in piezoelectric actuators for humidifiers, so the most important dimension of a mesh membrane is the diameter of the circular holes. Traditionally, a number of holes in a mesh membrane and non-coplanar mesh membrane array are manually inspected, which is expensive and time consuming. To address this problem, this study develops a novel auto-focus measurement system, which includes the hardware and software systems. The hardware system is composed of the modules for the motion stage and the auto-focus optical mechanism. The software system consists of two phases: (1) training the general regression neural network (GRNN) for auto-focus technology, in the offline phase, and (2) automatically capturing sharp mesh images using a GRNN and reliably measuring holes using the three measurement algorithms, in the online phase. The results of the experiments confirm that the proposed auto-focus technology, which uses a GRNN, obtains the correct focus position. The accuracy of the measurement of the diameter of a hole can reach 0.89 μm, the successful measure rate is almost 98 % and the average measurement time is 4.8 s per mesh. These results show that the proposed system is not only robust for auto-focus technology, but also efficient and effective for the measurement of mesh membrane arrays.














Similar content being viewed by others
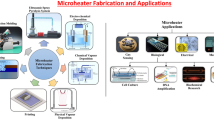
References
Arlot S, Celisse A (2010) A survey of cross-validation procedures for model selection. Stat Surv 4:40–79. doi:10.1214/09-SS054
Chen CS, Yeh CW, Yin PY (2009) A novel Fourier descriptor based image alignment algorithm for automatic optical inspection. J Vis Commun Image Represent 20: 178–189. doi:10.1016/j.jvcir.2008.11.003
Chtioui Y, Panigrahi S, Francl L (1999) A generalized regression neural network and its application for leaf wetness prediction to forecast plant disease. Chemom Intell Lab Syst 48:47–58. doi:10.1016/S0169-7439(99)00006-4
Di SJ, Li XM, Wei Q (2011) Application of generalized regression neural network in prediction and analysis of slope stability. Adv Sci Technol Water Resour 31:80–83. doi:10.3880/j.issn.1006-7647.2011.03.022
Dobruschin PL (1968) The description of a random field by means of conditional probabilities and conditions of its regularity. Theory Probab Appl 13(2):197–224. doi:10.1137/1113026
Kohavi R (1995) A study of cross-validation and bootstrap for accuracy estimation and model selection. Paper presented at the Proceedings of the 14th international joint conference on Artificial intelligence, Montreal, Quebec, Canada, vol 2, pp 1137–1143. ISBN:1-55860-363-8
Liu HW, Chen CS (2014) Visual measurement system for circular workpiece. Paper presented at the computing, measurement, control and sensor network (CMCSN), 2014 International Conference on, Taichung, Taiwan
Myers RH, Montgomery DC, Cook CMA (2008) Response surface methodology: process and product optimization using designed experiments. Wiley, New York
Parzen E (1962) On estimation of a probability density function and mode. Ann Math Stat 33(3):1065–1076. doi:10.1214/aoms/1177704472
Schløler H, Hartmann U (1992) Mapping neural network derived from the parzen window estimator. Neural Netw 5:903–909. doi:10.1016/S0893-6080(05)80086-3
Specht DF (1991) A general regression neural network. IEEE Trans Neural Netw 2:568–576. doi:10.1109/72.97934
Huang TW (2008) A study of the algorithms for the evaluation of circularity based on geometrical graphics. Master’s thesis, National Taiwan University, Taipei
Yu S, Mantian L (2006) Research on SUSAN based auto-focusing algorithm for optical microscope application. In: Mechatronics and automation, proceedings of the 2006 IEEE international conference, vol 3, pp 1237–1241. doi:10.1109/ICMA.2006.257803
Acknowledgments
This research was performed with the support of LIGA Precision Technology Co. Ltd., Taiwan, ROC. The authors particularly thank the LIGA Precision Technology Co. Ltd. for providing the test samples and for sharing valuable experience of mesh membrane arrays.
Author information
Authors and Affiliations
Corresponding author
Rights and permissions
About this article
Cite this article
Chen, CS., Weng, CM., Lin, CJ. et al. The use of a novel auto-focus technology based on a GRNN for the measurement system for mesh membranes. Microsyst Technol 23, 343–353 (2017). https://doi.org/10.1007/s00542-015-2473-z
Received:
Accepted:
Published:
Issue Date:
DOI: https://doi.org/10.1007/s00542-015-2473-z