Abstract
Changes in the body condition of Antarctic minke whales (Balaenoptera bonaerensis) have been investigated in a number of studies, but remain contested. Here we provide a new analysis of body condition measurements, with particularly careful attention to the statistical model building and to model selection issues. We analyse body condition data for a large number (4704) of minke whales caught between 1987 and 2005. The data consist of five different variables related to body condition (fat weight, blubber thickness and girth) and a number of temporal, spatial and biological covariates. The body condition variables are analysed using linear mixed-effects models, for which we provide sound biological motivation. Further, we conduct model selection with the focused information criterion (FIC), reflecting the fact that we have a clearly specified research question, which leads us to a clear focus parameter of particular interest. We find that there has been a substantial decline in body condition over the study period (the net declines are estimated to 10% for fat weight, 7% for blubber thickness and 3% for the girth). Interestingly, there seems to be some differences in body condition trends between males and females and in different regions of the Antarctic. The decline in body condition could indicate major changes in the Antarctic ecosystem, in particular, increased competition from some larger krill-eating whale species.
Similar content being viewed by others
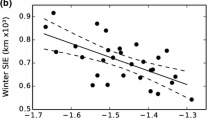
Avoid common mistakes on your manuscript.
Introduction
Most baleen whale species accumulate fat during the summer feeding season at high latitudes and migrate to lower latitudes for reproduction. Whale blubber serves as energy storage and is also important for thermal insulation, structural support, locomotion, streamlining and buoyancy (Parry 1949; Lockyer et al. 1984; Lockyer 1991; Folkow and Blix 1992; Koopman 2007). Measurements of blubber have therefore been used as indicators of body condition, but one may note that energy is also stored in the muscle, bones and as visceral fat. Blubber thickness, total fat weight and whale girth have all been found to increase during the feeding season (Lockyer 1981). Interest lies in the potential temporal changes in body condition of Antarctic minke whales (Balaenoptera bonaerensis, hereafter simply referred to as minke whales), because these could indicate fundamental changes in the Antarctic ecosystem.
Krill, primarily Euphausia superba, are the main prey species for all baleen whales and many seal and penguin species in the Southern Ocean. When the larger baleen whales were hunted down to very low population levels, especially in the period from the mid-1920s to the mid-1960s, the consumption of krill by these whales decreased substantially. Laws (1977) hypothesised that large amount of krill in this period became available for other krill-eating species, among them the Antarctic minke whale, which was never hunted commercially for whale oil. This has been called the krill surplus hypothesis. From the late 1960s, the populations of most of the large baleen whales have been increasing. This increase is best documented for the humpback whales (Megaptera novaeangliae), which in recent years have been increasing by about 8% per year (IWC 2019). According to the krill surplus hypothesis, a response by the minke whales should be expected, first at the level of individual minke whales, and later as a population response. In this paper, we provide quantitative evidence of a potential reversal of the original krill surplus hypothesis effect.
We will analyse five variables with an assumed relation to body condition: total weight of fat dissected from the whale body, blubber thickness at two points and girth at two points. The variables were measured over an 18-year period, from 1987 to 2005. Parts of the same dataset were first analysed in Konishi et al. (2008) using multiple stepwise linear regression. Those analyses indicated that blubber thickness, girth at umbilicus and fat weight had been decreasing over the study period. At the 2011 meeting of the Scientific Committee of the International Whaling Commission (hereafter IWC-SC), a paper was presented suggesting that the linear regression model used by Konishi et al. (2008) might have been inappropriate since it did not account for various forms of possible heterogeneity and interactions (de la Mare 2011). Since then, many different mixed-effects models have been proposed and discussed in the IWC-SC. Model selection has been carried out using model selection criteria such as the Akaike information criterion (AIC) or the Bayesian information criterion (BIC), as recommended by Zuur et al. (2009), see Konishi and Walløe (2015). Both the choice of criterion and various aspects of the modelling have been subject to heated discussions and criticism in several subsequent meetings, see, e.g. de la Mare et al. (2017), McKinlay et al. (2017).
Here, we address this question of body condition decline once again in an attempt to finally resolve the matter. We see our contribution in this article as two-fold. First, we propose, motivate and analyse a larger and more biologically plausible model than the ones used in previous studies. We call this model the wide model. Secondly, and crucially, we perform model selection with the focused information criterion (FIC). The FIC framework is perfectly suited for the problem at hand, because we have a clear question of primary interest, which can be translated into a well-defined focus parameter. In the IWC-SC discussions, the question of primary interest was whether there has been a decline in body condition during the 18 study years or not. The FIC is in widespread use in several application areas, though so far with limited applications in biology. It has, however, earlier been used in Hermansen et al. (2016) for determining the autoregressive order of the Hjort index for the Atlantic cod (Gadus morhua), and it is hoped that the present article contributes to its further use in applications with mixed-effects models in biological studies.
Methods
Data and model building
The data we analyse come from the Japanese whale research programme (JARPA, later called JARPA I), which took place for 18 years, from 1987 to 2005. Research within JARPA was organised around several aims concerning the abundance and population structure of cetaceans in the Southern Ocean, with a particular emphasis on minke whales and their role in the Antarctic ecosystem (IWC 2008). Sampling of minke whales took place in the feeding season of the minke whales, typically between early December and late March. In addition to variables related to genetics, reproduction, endocrinology and pollutant content, five body condition proxies were recorded: fat weight, blubber thickness at two sites (BT11 and BT7) and half girth at two sites (umbilicus and axilla). The fat weight was the mass of fat dissected from the whale body, mainly blubber and visceral fat. BT7 and BT11 were measured at two well-defined lateral points on the body surface, above the umbilicus for BT7 and below the dorsal fin for BT11. Figure 1 shows the position of the blubber thickness and the girth measurements. Only measurements from sexually mature males and pregnant females have been used in our analyses. Nearly all mature female minke whales were pregnant at the time of capture, as is generally the case for this species at this time of the year. The total number of mature whales sampled during these 18 years was 4704. Girth and blubber thickness measurements are available from almost all these whales. The dissection of fat tissue was time consuming and was carried out only on the first whale sampled each day. There are therefore only 683 observations of the fat weight variable.
An Antarctic minke whale (Balaenoptera bonaerensis) with sites of the blubber thickness and half girth measurements. Open triangle: position of the umbilicus. Arrows: half girth measurements; axilla and umbilicus. Closed circles: Lateral points for blubber thickness measurements; BT7 above the umbilicus, and BT11 below the dorsal fin
The five body condition proxies will serve as the response variables in our analyses. Since all the response variables are meant to reflect body condition, we only specify one wide model to be used for all responses. We will present the results for fat weight and BT7 in the result section. The results for the other responses are given in supplementary material 3.
In some species, the evolution of body condition could perhaps have been studied by repeatedly sampling the same animals over time. Assessing the body condition of a living minke whale is impractical,Footnote 1 however, and we therefore have to resort to careful modelling. In order to obtain a correct impression of the evolution in body condition, the model needs to adjust for all factors which potentially influence, or explain, the body condition of the captured whales. For each whale, we have measurements of several variables that are potentially relevant for explaining differences in body condition: the year of capture, the date within each year, the sex, the body length, the body mass, the length of foetuses for the females, the age, different spatial covariates like latitude and longitude and, finally, the binary indicator \(\mathtt{diatom}\) which denotes whether the whales had little or substantial diatom coverage.
In order to decide which covariates to include, one has to consider available biological knowledge and also the basic statistical fact that one should only include variables which are (potentially) influencing the body condition and not those that are influenced by body condition (i.e. we do not want to condition on a variable that is an effect of body condition). As an example, consider the variable foetus length. The length of a foetus is a function of time since reproduction, but it is also likely to be influenced by body condition, see Christiansen et al. (2014). Since this variable thus has a somewhat unclear (causal) relationship with body condition, we do not include it in our models. Similar arguments lead us to omit total body mass as a covariate in our models, since body mass will increase as a function of body condition. The model needs to contain a variable accounting for the structural size of the animal: larger whales will have thicker blubber and more fat than smaller whales, even though they may actually have the same body condition. For this role, we will prefer to use body length, rather than body mass, since we can safely assume that the body length at the time of catch will not be influenced by body condition.
According to biological knowledge, the date of capture will be an important covariate when modelling the body condition. The whales are on their feeding grounds and are thus expected to undergo a large improvement in body condition over this summer season. The date covariate, henceforth referred to by \(\mathtt{date}\), is defined so that the December 1 in each year is equal to 1. The coefficients related to the date within each year will therefore be describing the daily increase in body condition for minke whales on their feeding grounds. Diatom infestation disappears in warmer waters and is assumed to increase with time spent in cold waters (Lockyer 1981; Pitman et al. 2020). Diatom coverage can therefore be considered as an indication of time spent in the Antarctic feeding grounds and is likely to be positively correlated with the date of capture. Still, two different whales captured on the same date may have arrived to the feeding grounds at different times and this would influence their body conditions, so we find it natural to include both \(\mathtt{date}\) and the diatom variable. All the whales in our dataset are mature and most have an age between 10 and 35 (the age is determined based on growth curves in ear plugs). Age and body length have a quite strong positive correlation (around 0.4), but we have chosen to include both. The positive relationship between age and body length is only apparent for younger whales (below 15) and flattens out for higher ages. Some of the proxies for body condition might change with age. The sex of the whales is likely an important covariate; previous studies have reported sex-specific differences in blubber thickness, for example (Lockyer 1981).
Antarctic minke whales occurrence is highly influenced by the shape of ice edge which rapidly diminishes in summer (Ainley et al. 2012; Lee et al. 2017; Konishi et al. 2020); there is therefore little reason to believe that the latitude at the time of catch will be strongly related to body condition. We have therefore not included latitude in our current model. We include, however, a factor \(\mathtt{region}\) which denotes one of the three different areas where the whales were caught (West, East and Ross Sea), see Fig. 2. This is because prior studies indicate some degree of genetic segregation between minke whale populations in different parts of the Antarctic (Pastene and Goto 2016); these regions could be subject to different environmental conditions leading to different food availability and subsequently to different body condition.
Map of the Antarctic continent. The three dashed circular lines indicate latitudes of 75 \(^{\circ }\)S, 70 \(^{\circ }\)S and 60 \(^{\circ }\)S. The two dashed straight lines indicate longitudes of 40 \(^{\circ }\)E and 140 \(^{\circ }\)W. The points give the locations where minke whales were caught, with different shapes and colours identifying the three regions: West, East and Ross sea. The map was generated using the maptool package (Bivand and Lewin-Koh 2019)
Preliminary explorative analyses reveal that most of the continuous covariates described above have a fairly linear relationship to body condition, except for \(\mathtt{date}\) and \(\mathtt{year}\), for which we allow quadratic relationships to the response. As explained in the introduction, the focus of our investigations is to estimate, and test, the yearly decline in body condition. A natural focus parameter should therefore summarise this yearly decline, and be a function of the parameters \(\beta _{\mathrm{year}}\) and \(\beta _{\mathrm{yearsq}}\), which are the coefficients describing the fixed ‘year effect’, i.e. \(\mathtt{year}\) and \(\mathtt{year}^2\) , respectively in Eq. (2) below. Since we have a quadratic year term in our wide model, with that part taking the form \(\beta _{\mathrm{year}} x + \beta _{\mathrm{yearsq}}x^2\) for year x, a natural definition of the overall yearly decline is
with \(x_0\) the mean year in the dataset. The focus parameter corresponds to the derivative of the mean response, with respect to \(\mathtt{year}\), evaluated in this mean year time point. This focus parameter can also be interpreted as the overall slope, the mean curve evaluated at the end point subtracting its value at the start point, divided by the length of time. If the focus parameter is negative and significant, we will be able to claim that there has been a significant decline in body condition. This focus parameter will be central both in the analysis of the wide model and in the model selection part.
Further, we include several interaction terms. Many of these terms are likely to be small, but we want our wide model to be flexible and to include all potentially relevant effects. The reasoning behind the interaction terms is often quite self-explanatory; it is, for example, natural that the relationship between body condition and body length might be different for males and females, and therefore, we include an interaction between body length and sex. Certain three-way interactions are also included, e.g. between body length, sex and \(\mathtt{date}\), since the rate at which energy accumulates during the season might be different for whales of different length and sex. We also let the relationship between body condition and time (both \(\mathtt{year}\) and \(\mathtt{date}\)) be different in the three different regions.
Finally, we include random effects, and our regression model falls therefore into the class of mixed-effect models, see Demidenko (2013), Pinheiro and Bates (2000) and Cunen et al. (2020). Mixed-effect models are often used when the observations form natural groups, which typically correspond to observations collected at close to the same location or time. For our JARPA dataset, we let the groups be defined by the year of capture, and this variable thus defines 18 groups (one for each year). We let the random effect influence both the intercept and the terms related to \(\mathtt{date}\). The random effect influencing the intercept should be understood as letting all the observations from the same year having a (potential) year-specific deviation from the fixed year effect, i.e. the mean line \(\beta _{\mathrm{year}} x + \beta _{\mathrm{yearsq}}x^2\). The random effect influencing \(\mathtt{date}\) means that each year will have potentially different coefficients for \(\mathtt{date}\) and \(\mathtt{date}^2\). We find it natural to assume that body condition is influenced by many random processes with yearly variations. In particular, the relationship between body condition and \(\mathtt{date}\) could be different from year to year due to random fluctuations in krill production.
The wide model that results can be presented with the following R-type notation:
This model has \(p=40\) fixed-effect coefficients. The notation \((1 + \mathtt{date}+ \mathtt{date}^\mathtt{2}\,|\,\mathtt{yearCat})\) specifies the random-effect structure. The notation \(\mathtt{yearCat}\) is meant to highlight the fact that in this context year serves as a categorical variable which defines a particular grouping of the data, as opposed to its role in the fixed-effect part of the model where \(\mathtt{year}\) is a continuous covariate. The random-effect structure defines a symmetric \(3\times 3\) covariance matrix, giving 6 additional parameters. Including the residual variance, we have a total of 47 parameters to estimate.
Some initial investigations reveal that the residuals are close to normally distributed (see supplementary material 3), and we can therefore remain within the class of linear mixed-effects models. We fit our models using the lme4 package, see also Bates et al. (2014). To help convergence of the model, the covariate \(\mathtt{date}\) was scaled (i.e. by subtracting the mean \(\mathtt{date}\) value and dividing by its standard deviation). For ease of interpretation, the covariate \(\mathtt{bodylength}\) was centred. We defined the interactions between \(\mathtt{year}\) and factor variables, i.e. \(\mathtt{region}\) and \(\mathtt{sex}\), as sum-to-zero contrasts. This ensures that \(\beta _{\mathrm{year}}\) and \(\beta _{\mathrm{yearsq}}\) can be interpreted as the parameters governing the overall yearly decline, and not the yearly decline for say males in some particular region.
There were some incomplete observations for each response, so that we ended up with the following numbers of observations for each variable: 683 for fat weight (in kg), 4318 for BT7 (in mm), 4306 for BT11 (in mm), 4298 for umbilicus girth (in cm) and 3518 for axillary girth (in cm).
Confidence curves
Confidence curves are a useful tool for presenting all aspects of frequentist inference for the parameter of interest. They belong to the wider topic of confidence distributions, which is given a thorough treatment in Schweder and Hjort (2016). Within the scope of this article, it is sufficient to understand how these curves need to be interpreted, for example in the upper right panel of Fig. 3. Along the horizontal axis, we have potential values of the parameter of interest, while the vertical axis gives different degrees of confidence. The lowest value of the confidence curve corresponds to the point estimate of the parameter, here \(-\,8.9\) kg. In addition, confidence intervals at all levels can be read off the curve. For instance, if we are interested in the 95% interval, we find it by reading off where the curve crosses the 0.95 line (marked in red in the figure).
Focused model selection
The main distinction between FIC and various other information criteria is the presence of a focus. The focus parameter, here denoted by \(\mu\), is a quantity of interest that depends on the model parameters and is estimable from the data. We defined our focus parameter in (1). The goal of model selection with FIC is to find the model, among a set of candidate models, which provides the most precise estimates of this focus parameter. In our model selection problem, we will specify nine candidate models in addition to the wide model given in (2) and hence have ten estimators for \(\mu\). Each such estimator, say \(\widehat{\mu }_M\) for a candidate model M, comes with its own bias and variance, say \(b_M\) and \(\tau _M^2\). Thus, for each candidate model, there is a corresponding mean squared error (mse), which constitutes a natural measure of the precision of the estimator from model M,
The basic idea of the FIC is to estimate these \(\mathrm{mse}\) values from the data, for the wide as well as for each candidate model, i.e. to construct
with the second term indicating estimation of the squared bias \(\mathrm{bsq}_M=b_M^2\). In the end, one selects the model with the smallest estimated mse.
There are two main strategies for the actual computation of the FIC scores, see, e.g. Claeskens et al. (2019) for an accessible review. The different strategies all have the same goal, but use different mathematical approximation tools, and can therefore have somewhat different forms. In our case, we use the formulae from Cunen et al. (2020), which are derived specifically for the class of linear mixed-effects models. All FIC strategies require the biases and variances in (3) to be defined with respect to a wide model. The wide model is thus assumed to be the true data-generating mechanism; we return to this assumption in the discussion.
The nine candidate models we have examined are described briefly in Table 1 and given in full in supplementary material 1. Note that for candidate models with a linear relationship between body condition and \(\mathtt{year}\) the focus parameter in (1) simplifies to \(\beta _{\mathrm{year}}\) only. The candidate models correspond to nine different simplifications of the wide model, and most of them reflect variations of the wide model which we consider biologically plausible. The exception is model \(M_9\) which is unrealistically simple. Our model analysis and model selection machinery can handle many more models with relative ease, also those that would be automatically generated by taking all further submodels of a given type, etc. Keeping the list of candidate models relatively small is, however, beneficial, not merely because of the numerical burden, but because the final analyses risk becoming less clear when too many candidate models are included. Model \(M_1\) is of particular importance. This model is the same as the wide, but without any continuous year terms (so that \(\beta _{\mathrm{year}}=\beta _{\mathrm{yearsq}}=0\)). The focus parameter will therefore simply be equal to zero in this model and will also have zero variance, but with potentially large bias. The performance, in terms of FIC, of this model compared to the ones containing the focus parameter constitutes an implicit test of the ‘significance’ of the yearly decline: if \(M_1\) is favoured by FIC, this would indicate that the yearly decline is very small compared to the variance of the estimate.
When a model has been chosen via a model selection procedure, the ensuing inference needs to take the first-step model selection uncertainty into account, to avoid p values that are too small, etc., see Claeskens and Hjort (2008, Chap. 7). In order to avoid this problem, we have chosen a simple but conservative approach. We randomly split the dataset into two halves and use the first half for the model selection procedure and the second half for inference (estimating coefficients, constructing confidence curves). Consequently, the estimates (and corresponding test statistics) and the FIC scores are computed using two different halves of the dataset. This means that we lose considerable estimation power, so that our confidence curves after model selection should be considered conservative.
Results from model selection with FIC are presented in the form of so-called FIC plots, see, for example, the left panel of Fig. 6. Along the horizontal axis, we have the square roots of the FIC scores, i.e. our estimates of the root mean squared error of the focus parameter. A lower FIC score means that the model gives a more precise estimate of the focus parameter. On the vertical axis, we give the estimates of the focus parameter, in our case, the overall yearly decline.
Results
Here we present the results for fat weight and BT7; the analyses and results for the other responses are found in supplementary material 3. First, we present the fitted wide model by means of various figures. Then we carry out model selection for each of these response variables using FIC and present the inference from the winning model, i.e. the model favoured by FIC. After fitting, it is crucial to evaluate whether the wide model fits the data adequately. This is also important for the use of FIC, since the performance of the framework relies on the wide model being close to the true data-generating mechanism. We comment on the use of diagnostic plots in Sect. 1 in supplementary material 3, and there we also display a number of diagnostic plots.
Analysing the wide model
The full fitted wide model for fat weight, and all the other responses, is given in the first section of supplementary material 2, but some results of particular interest are displayed in Figs. 3 and 4. Regression estimates are not straightforward to interpret in a model containing a large number of interaction terms, and we therefore illustrate the effect of some explanatory variables by predictor effect displays, see, for example, Fox and Weisberg (2018), demonstrating the predictor’s estimated contribution with respect to the response variable. Note that the uncertainty bands in the predictor effect displays also take into account the standard errors of the intercept estimate. The width of these bands should therefore not be directly interpreted as tests of significance of the predictor on the horizontal axis (unlike the confidence curves which serve that purpose). The explanatory variables not involved in each effect plot are set to their mean value.
Selected results from the wide model analysis of fat weight. a Predictor effect display for \(\mathtt{year}\); the overall year effect (thin solid black line), with pointwise error bands (shaded grey area); female whales are shown by thick solid red lines, and male whales by thick dashed blue lines; dark colours represent the West region, medium light colour represents the East region, and lightest colour represents the Ross Sea. b Confidence curve for the overall yearly decline, \(\mu\), in the wide model. The point estimate is \(-\,8.9\) kg. From the confidence curve, we can read off confidence intervals of all levels, for instance, the 95% interval which is equal to \([-15.1, -2.7]\) kg. c Predictor effect display for \(\mathtt{date}\); the overall effect (thin black line) with pointwise error bands (shaded grey area), for the two sexes (thick lines, solid red for females, blue dashed for males) and for low (dark) and high (light) diatom load. d Predictor effect display for \(\mathtt{date}\); the coloured lines represent the seasonal evolutions for the 18 different years
As explained above, the main focus of our analysis lies in quantifying the yearly decline in body condition. The estimated overall relationship between fat weight and \(\mathtt{year}\) indicates a gradual almost linear decline over the study years (Fig. 3, top left panel). The overall yearly decline is estimated to be \(-\,8.9\) kg and is significantly different from zero at all reasonable levels, as we can see from the confidence curve in the top right panel. Females have generally somewhat more fat than males (Fig. 3, top left panel), but this difference is not significant (supplementary material 2). As expected, the fat weight increases considerably with \(\mathtt{date}\) (Fig. 3, bottom panels). The general estimated seasonal evolution is close to linear, but there are differences between males and females, and between whales with different diatom loads (Fig. 3, bottom left panel). The 18 years of study have somewhat different seasonal evolutions (Fig. 3, bottom right panel), which indicates that the random-effect terms pick up quite a lot of variation.
The estimated overall relationship between BT7 and \(\mathtt{year}\) indicates a gradual linear decline over the study years (Fig. 4, top left panel). The overall yearly decline is estimated to be \(-\,0.15\) mm and is significantly different from zero at the 5 % level, as we can see from the confidence curve in the top right panel. The relationship between BT7 and \(\mathtt{year}\) varies more across sex and especially regions than for fat weight. The yearly decline is most pronounced in the West region, for both male and female whales. There, the whales experienced a relatively steep decline in blubber thickness in the first half of the study period, before the decline flattens in the second half. For the Eastern region and the Ross sea, the decline in blubber thickness is much less pronounced. The overall relationship between \(\mathtt{date}\) and BT7 (Fig. 4, bottom left panel) is relatively similar to the seasonal evolution in fat weight. Male whales have a lower blubber thickness than females at the beginning of the season, but experience a faster increase with \(\mathtt{date}\). For whales with a low diatom load, the increase is the fastest at the end of the season. There is a substantial variation in seasonal evolution between the years, giving support to the inclusion of random effects of year influencing the effect of \(\mathtt{date}\) (Fig. 4, bottom right panel).
Selected results from the wide model analysis of BT7. a Predictor effect display for \(\mathtt{year}\); the overall year effect (thin solid black line), with pointwise error bands (shaded grey area); female whales are shown by thick solid red lines, and male whales by thick dashed blue lines; dark colours represent the West region, medium light colour represents the East region, and lightest colour represents the Ross Sea. b Confidence curve for the overall yearly decline, \(\mu\), in the wide model. The point estimate for the decline in the mid-point year is around \(-\,0.15\) mm; the 95% confidence interval is \([-0.27, -0.02]\) mm. c Predictor effect display for \(\mathtt{date}\); the overall effect (thin black line) with pointwise error bands (shaded grey area), for the two sexes (thick lines, solid red for females, blue dashed for males) and for low (dark) and high (light) diatom load. d Predictor effect display for \(\mathtt{date}\); the coloured lines represent the seasonal evolutions for the 18 different years
For the three remaining responses, we provide figures similar to Figs. 3 and 4 in supplementary material 3. These figures demonstrate that most of the principal patterns in the data are consistent across the five responses. Importantly, the relationship between the response and \(\mathtt{year}\) is negative and significant. For BT11, the estimated overall yearly decline was \(-\,0.160\) mm, with a 95 % confidence interval of \([-0.318;-0.002]\), for axillary girth we have \(-\,1.11\) cm and \([-1.50;-0.73]\), and for umbilicus girth we have \(-\,0.44\) cm and \([-0.73;-0.15]\). The fitted interaction terms indicate some differences between males and females, and across the three regions. The relationship between the response and \(\mathtt{date}\) is positive, but the seasonal evolution is somewhat dependent on diatom coverage and sex and is also different for each of the 18 years of study.
The scaled fitted random effects for each year for each of the five responses (in different colours and line type, see legend). The three panels correspond to the random effects influencing the intercept (a), \(\mathtt{date}\) (b) and \(\mathtt{date}^2\) terms (c). In order to show them on the same figure, the fitted random effects have been scaled with their respective mean response values (the vertical axes are thus not directly interpretable)
From the fitted wide model, one can obtain the \(18 \times 3\) fitted random effects (called conditional modes of the random effects in parts of the literature). In Fig. 5, we look at scaled versions of these quantities, where we have divided the fitted random effects in each of the five analyses with its respective mean response value. The goal is simply to be able to display the five sets of fitted random effects in the same figure. We note that the fitted random effects are quite consistent in the five responses. For example, year five has a larger than usual intercept value for fat weight and also for BT7, BT11 and umbilicus girth. Remember that in our model the random effects on the intercept should be understood as year-specific deviations from the fixed year effect, i.e. the mean line \(\beta _{\mathrm{year}} x + \beta _{\mathrm{yearsq}}x^2\). This figure thus points to years which can be considered as particularly ‘good’ or ‘bad’ for body condition according to the random year effect. Good years are characterised by unusually large intercepts, i.e. bigger average body condition, or an unusually large \(\mathtt{date}\) coefficient, i.e. faster increase in body condition over the season, or both. The random effect on the \(\mathtt{date}^2\) coefficient is less straightforward to interpret. The random effects of intercept and \(\mathtt{date}\) are quite strongly correlated. Both indicate particularly good years in year 5, 12 and 16 (and to a lesser extent in year 9), and particularly bad years in year 11, 15 and 18 (and also 8).
Results from model selection
For fat weight, we see that the models \(M_2\), \(M_3\) and \(M_5\) were considered best according to FIC. In fact, all these three models obtained a FIC score equal to zero. The wide model \(M_0\) obtained a root-FIC score of around 4, while the model without fixed year effect, \(M_1\), obtained a considerably larger score of around 10. Since \(M_5\) is the smallest (in terms of number of parameters) among the three models with minimal FIC scores, we chose to proceed with this model. The confidence curves in the middle panel of Fig. 6 clearly show that the winning model has lower variance than the wide model, with a minimal bias. In the right panel, we plot the estimated relationship between fat weight and \(\mathtt{year}\) from both models. Here, we see that the yearly decline in the winning model, \(M_5\), clearly approximates the yearly decline in the wide model (especially in the mid-point year, as expected according to our choice of focus parameter).
Model selection results for fat weight. a FIC plot. b Confidence curve for the overall yearly decline, computed after model selection, wide model shown by dashed black line, winning model by solid green line. c Predictor effect display for \(\mathtt{year}\), with pointwise uncertainty bands, for the wide (dashed black and grey lines) and winning model \(M_5\) (solid green lines)
With BT7, the winning model was also \(M_5\), see the left panel of Fig. 7. For this response also, the model without any fixed year effect, \(M_1\), was considered to be much worse than all the other competitors. In this case, the expected reduction in variance is not apparent from the confidence plot in the middle panel. This could be due to randomness from the data-splitting; remember that the FIC scores and confidence curves are computed on two different halves of the dataset. Note also that the wide model \(M_0\) and the winning model \(M_5\) are not actually considered to be very different (in terms of precision of the focus parameter) by the FIC procedure. The two curves in the right panel look somewhat different; this illustrates a possible drawback for our choice of focus parameter, which considers only the mid-point year. Nevertheless, the difference in these two curves would not change our conclusion concerning the decline over the full study period.
Model selection results for BT7. a FIC plot. b Confidence curve for the overall yearly decline, computed after model selection, wide model shown by dashed black line, winning model by solid green line. c Predictor effect display for \(\mathtt{year}\), with pointwise uncertainty bands, for the wide (dashed black and grey lines) and winning model \(M_5\) (solid green lines)
For the three remaining responses, the winning models were \(M_3\) for BT11 and axillary girth and \(M_4\) for umbilicus girth; see supplementary material 3. For all three, \(M_1\), the model without fixed year terms, was considered the worst. For the two girth measurements, the FIC procedure chose models which produced focus parameter estimates with very little bias (compared to the wide model) and with a clear reduction in variance. The results were less conclusive for BT11 where the winning model appeared to have a rather large bias in its estimate of the focus parameter. This could again be due to random variation related to the data-splitting.
Discussion
Interpreting the fitted wide model
Despite its large number of parameters and hence relatively large variances, the wide model supports the hypothesis of a gradual decline in body condition throughout the period. This decline is found in all five response variables, though naturally these sources of information cannot be taken as independent since they are all assumed to be proxies of the same quantity. The decline is fairly linear, but for some of the responses, we see a slight levelling towards the end of the period. Over the whole period, the five response variables exhibit net declines of about 10% for fat weight, 7% for the two blubber thickness measures and around 3% for the two girth measures. These numbers are obtained by considering the difference between the fitted response values in the first and last year. Further, there are indications of differences between the three regions. For fat weight, BT7 and BT11, it is only in the West region that we see a clear decline, while for the two girth measures, all three regions show a clear decline. It is not clear how to interpret these results, and one must be careful not to over-interpret non-significant differences between the regions. Also, one must keep in mind that the West region has the most observations. Nevertheless, differences in the evolution of body condition could be rooted in differences in krill species and in differences in krill-eating competition between the regions. In the East and West regions, Euphausia superba is the primary krill species, while in the Ross Sea, it is Euphausia crystallorophias (Murase et al. 2013). Minke whales in the East and West compete for krill with humpback whales, but the latter seldom go into the Ross Sea (Ainley 2010; Bombosch et al. 2014; Andrews-Goff et al. 2018; Riekkola et al. 2018).
The fitted wide model reveals other interesting features besides the evolution of body condition over time. In particular, there are interesting differences in seasonal evolution (‘date effect’) between males and females, between whales with different diatom coverage and between the different years. For a given date, whales with a low diatom load will have lower body condition than whales with a high diatom load, but this difference appears to become less pronounced towards the end of the season (at least for fat weight, males BT7, males BT11 and males axillary girth). This fits well with the interpretation of diatoms as a measure of time spent in the Antarctic feeding grounds (Lockyer 1981; Pitman et al. 2020): low diatom load whales are newer arrivals, and it is therefore not surprising that they should be leaner than the whales that have been longer on the feeding grounds. As the season progresses, the difference tends to even out. In general, the female whales have a higher body condition than males, but their rate of increase in body condition over each feeding season appears to be slower than for the males. This effect is especially apparent for BT7 (Fig. 4) and BT11 (Fig. 7 in supplementary material 3), but only somewhat evident for fat weight (Fig. 3). The unusually high relative fatness of pregnant females compared to adult males has been observed for other baleen whale species too, see (Lockyer 1981, 1986; Miller et al. 2011). In the Arctic, female minke whales arrive in the feeding grounds earlier in the season than male whales (Jonsgård 1951); if this were to hold in the Antarctic too it could provide a partial explanation for the differences in seasonal evolution. It is also possible that the pattern is due to female whales diverting some of their energy to foetus growth (Christiansen et al. 2014).
Results obtained from our wide model are compared with those obtained from two previous models in Table 2 (Konishi et al. 2008; Konishi and Walløe 2015). In Konishi et al. (2008), the decrease per year was estimated at approximately 0.2 mm for BT11 and 17 kg for fat weight, corresponding to a 9% reduction for both measurements over the whole 18-year period. These two sets of models are considerably different from, and in particular much smaller than, the wide model we have analysed here. The simple linear regression model was justifiably criticised for not allowing interactions or heterogeneity (de la Mare 2011). Even so, the results from the three analyses are not very different. Similar results are not uncommon in other scientific fields. Early results obtained by simple linear regression analyses in medical epidemiology are often confirmed by analyses using mixed-effects models. In some studies, this could be due to relatively modest heterogeneity and interaction terms of small magnitude. More generally, random effects and interaction terms will often have a stronger influence on the residual variance estimate than on the point estimates of overall effects. For blubber thickness below the dorsal fin and half girth at umbilicus, we see that our wide model results in much wider confidence intervals than the simple linear model. It is also the case that for most responses, the intervals from our wide model are wider than the ones from the linear mixed model of Konishi and Walløe (2015).
Our model used \(\mathtt{year}\) both as a fixed-effect covariate with a quadratic shape, and as the group indicator for the random effects, ensuring that the intercept, \(\mathtt{date}\) and \(\mathtt{date}^2\) terms are different for each year. In this way, we remove some of the year-specific variation that could have been absorbed by the fixed-effect year term and transfer it to the random terms instead. This can explain why some of our estimates of the yearly decline are smaller than the estimates found in previous studies where random effects were not used in the same way, see Table 2. In this sense, our wide model can be considered conservative.
Naturally, the results presented here can be somewhat sensitive to the choices involved in building what we term the wide model. We have spent considerable effort in motivating our choice and have strived to justify our choices using biological arguments. Note also that several of the particular covariates have been the subject of discussions in the IWC-SC (de la Mare et al. 2017; McKinlay et al. 2017, 2018). We have investigated several different versions of the wide model presented in this article. In particular, we have fitted versions including latitude as a linear covariate, including foetus length for the females, and even including total body mass instead of total body length. In all these versions, the relationship between body condition and \(\mathtt{year}\) has remained essentially unchanged. The results thus seem reasonably robust to moderate changes of the wide model.
Interpreting the model selection results
The statistical models with which we have worked, including the more complicated ones, are all parametric, which means they are amenable to ranking and selection via the familiar AIC and BIC strategies. Those methods aim at sorting through candidate models from an overall perspective, however, balancing overall-fit with complexity, without taking account of the actual intended use of the fitted models. The FIC, in contrast, actively takes the focused questions into account, via analysis of the estimated precision of the different estimates of the focus parameter. The literature on FIC, see, e.g. Claeskens and Hjort (2008, Chaps. 6–7), shows that model selection via FIC typically does better than overall-modus selectors when it comes to what matters the most: precision of the final estimates for the crucial parameters. The FIC methods of Section 2.3, by construction yielding a ranking of candidate models, can also be supplemented with certain natural model averaging estimators. We do not pursue this theme here, but taking suitable weighted averages of the best estimates, in, e.g. Figs. 6 and 7, often leads to better final estimates; see Claeskens and Hjort (2008, Chap. 7).
Interested readers should note that our FIC procedure can be conducted for different choices of the focus parameter. The only requirement is that the user should be willing to express their focus as a function of the parameters in the wide model. The choice of focus parameter will depend on the main research question. Some researchers could, for example, be interested in studying whales with a particularly high body condition, and could then specify a focus parameter related to the probability of observing a minke whale with a body condition higher than some particular value \(y_0\). Other researchers might perhaps be more interested in the seasonal evolution and choose a focus parameter related to the \(\mathtt{date}\) terms. Different focus parameters will usually lead to different winning models.
Our FIC analysis has provided us with two major insights. First, we obtained a simplification of the wide model with considerably fewer parameters to estimate, and hence smaller variances, but with very little bias in the estimation of the focus parameter. Through the winning model, we learnt that even though some of the interaction terms in the wide model can be important and interesting, they are not strictly necessary in order to estimate the overall yearly decline. We also became aware that the random-effect structure can be somewhat simplified. These insights can be used when constructing models for similar data in the future. Secondly, the FIC analysis constitutes an implicit test of the overall yearly decline. The model without any fixed year terms was not favoured by FIC for any of the five responses, and this provides additional evidence that the fixed year terms are of considerable magnitude compared to the variance associated with their estimation.
The FIC scores for all the candidate models are computed with respect to the wide model, i.e. assuming that the wide model is the correct data-generating mechanism. One might enquire how sensitive the FIC scores are to the choice of the wide model, and indeed, this was one of the major criticisms of the FIC approach levelled by McKinlay et al. (2018) at the IWC-SC. We have conducted some sensitivity checks and found that moderate changes to the wide model had little effect on the ranking of the different candidate models. Also, for the wide models which we have investigated, the estimate of the focus parameter in the selected models was reasonably stable. Further, it is important to be aware of the correct interpretation of the results of model selection with FIC. The wide model needs to have a sound biological motivation, but the winning model should not necessarily be interpreted as being close to the true data-generating mechanism. The winning model is supposed only to serve a particular purpose: provide precise estimates of the focus parameter.
Explaining the decline in body condition
In this brief section, we summarise both historical and modern findings, views and hypotheses related to minke whales and the current decline in body condition. One potential explanation concerns industrial whaling and the krill surplus hypothesis; we will start with some historical remarks along these lines. The modern type of industrial whaling using steam ships and grenade harpoons was developed in the late 19th century, with whale oil as the main commercial product. There were early concerns about possible overexploitation. Johan Hjort (1902) wrote in a report to the Norwegian Parliament (our translation from Norwegian):
In my opinion, too many blue whales and fin whales are being caught at present. It seems clear to me that the whale populations in the seas around the northernmost parts of Norway are being appreciably affected by whaling, particularly where blue whales and fin whales are concerned. What is more, the notion that the oceans contain extraordinarily large numbers of whales is in my view a great exaggeration. I believe that by continuing to take the same number of whales as at present, we will cause their populations to decrease year by year, because they cannot breed fast enough to maintain the number of individuals.
Whaling was prevented by law in Norwegian waters from 1904, but the whaling companies then moved their activity to other parts of the North Atlantic, to the North Pacific, and to the Southern Ocean. The introduction of factory ships with a stern slipway from 1927 led to a dramatic increase in whale catches, and from then on a large number of humpback (Megaptera novaeangliae), blue (Balaenoptera musculus) and fin whales (Balaenoptera physalus) were taken from all regions of the Southern Ocean. Again, Johan Hjort was concerned about possible overexploitation of humpback, blue and fin whales; this is also touched upon in his whaling and sociology parable (Hjort 1937). He managed to arrange an international conference in Geneva under the League of Nations in 1929, but no agreement was obtained. Following another international conference in 1938, the IWC was established in 1946, but without any real reduction in the overexploitation. This hunt for large baleen whales was only closed in the 1960s when the commercial hunt was no longer profitable because of a low number of whales in the Southern Ocean. Richard Laws estimated in 1977 “that the stocks of baleen whales have been reduced by whaling—blue, fin, sei and humpback combined to about 18% of their former numbers. The humpback and blue whales are hardest hit, having been reduced to about 3 and 5% of the estimated initial stocks.”
The minke whale is a baleen whale, but it was never hunted commercially for oil because it has only a thin layer of subcutaneous blubber. The krill surplus hypothesis states that as the large krill-eating whale species were hunted far down, large amounts of krill became available for other krill-eating species (Laws 1977). As a result of greater food availability, the populations of krill-eating species were expected to increase, among them the minke whale population. Increases in food availability were also hypothesised to induce earlier sexual maturity and higher pregnancy rates, due to accelerating body growth rates (Laws 1962). There are various strands of empirical observations which appear to support the predictions of the krill surplus hypothesis. There are no minke whale abundance estimates from the industrial whaling period, but population models based on age data from minke whales caught between 1971 and 2005 indicate that there was an increase in abundance from 1930 until the mid-1970s (IWC 2014). In 1986–1991, the abundance was estimated to about 760 000 from sightings made by Japanese circumpolar research cruises (IWC 2019). A well-documented decline in mean age at sexual maturity of minke whales from 13 years for the 1940 cohort to 7 years for the 1970 cohort has also been interpreted in the context of the krill surplus hypothesis (Thomson et al. 1999). Crab-eater (Lobodon carcinophaga) and fur seals (Arctocephalus gazella), along with some penguin species, increased considerably in numbers between 1930 and 1960 (Sladen 1964; Payne 1977). The mean age at sexual maturity for crab-eater seals decreased between the 1940s and 1960s, but may have subsequently increased (Bengtson and Laws 1985). For fin whales, the mean age at sexual maturity decreased from 10 years in the 1930s to 6 years in the 1970s (Lockyer 1972). In the same period, the pregnancy rates for blue, fin and sei whales increased considerably from around 30% to over 50% (Gambell 1976).
In the last decades, the population of humpback whales has been increasing at a mean rate of 8% per year (IWC 2019). Other large baleen whales are anticipated to undergo a similar recovery. Humpback whales and blue whales are believed to be more efficient krill feeders than minke whales. It has also been observed that minke whales forage at deeper levels when found in areas with humpback whales (Friedlaender et al. 2009), and this is interpreted by Ainley et al. (2012) as energetically unfavourable for the minke whale. The minke whale is therefore likely to suffer in the competition with humpback whales and other larger baleen species. A decline in fat storage during the feeding season would be a first sign of such reversal of the ‘krill surplus hypothesis’. Later, an increase in mean age at sexual maturity is to be expected.
Increased competition between minke whales and other baleen whales is also expected due to environmental changes in the Antarctic. Minke whales exploit pack ice areas that are unavailable to larger species (Ainley et al. 2012; Konishi et al. 2020), and this habitat may shrink in the coming decades due to climate change (Tynan and Russell 2008). Climate change and subsequent changes in environmental conditions may also influence the krill abundance in the Antarctic (Atkinson et al. 2004; Nicol et al. 2008), and decline in body condition could also be interpreted in this light. Data on krill abundance are still relatively scarce, however. In order to identify the most vital causal mechanisms behind the decline in body condition, one will need to integrate information from several different sources and, in particular, from other krill-dependent species too.
Code availability
The code used for the analyses is available upon request to the corresponding author.
Data availability
The data can be made available upon application to IWC Scientific Committee’s Data Availability Group (DAG).
Notes
Interestingly, a very recent paper introduces a method for estimating the body mass of living whales using aerial photogrammetry (Christiansen et al. 2019).
References
Ainley DG (2010) A history of the exploitation of the Ross Sea, Antarctica. Polar Rec 46:233–243
Ainley DG, Jongsomjit D, Ballard G, Thiele D, Fraser WR, Tynan CT (2012) Modeling the relationship of Antarctic minke whales to major ocean boundaries. Polar Biol 35:281–290
Andrews-Goff V, Bestley S, Gales NJ, Laverick SM, Paton D, Polanowski AM, Schmitt NT, Double MC (2018) Humpback whale migrations to Antarctic summer foraging grounds through the southwest Pacific Ocean. Sci Rep 8:1–14
Atkinson A, Siegel V, Pakhomov E, Rothery P (2004) Long-term decline in krill stock and increase in salps within the Southern Ocean. Nature 432:100–103
Bates D, Maechler M, Bolker B, Walker S (2014) lme4: Linear mixed-effects models using Eigen and S4. R package version 1.1-21. https://github.com/lme4/lme4/
Bengtson JL, Laws RM (1985) Trends in crabeater seal age at maturity: an insight into Antarctic marine interactions. In: Siegfried WR, Condy PR, Laws RM (eds) Antarctic nutrient cycles and food webs. Springer, Berlin, pp 669–675
Bivand R, Lewin-Koh N (2019) maptools: tools for handling spatial objects. R package version 0.9-8. https://CRAN.R-project.org/package=maptools
Bombosch A, Zitterbart DP, Van Opzeeland I, Frickenhaus S, Burkhardt E, Wisz MS, Boebel O (2014) Predictive habitat modelling of humpback (Megaptera novaeangliae) and Antarctic minke (Balaenoptera bonaerensis) whales in the Southern Ocean as a planning tool for seismic surveys. Deep Sea Res Part I 91:101–114
Christiansen F, Víkingsson GA, Rasmussen MH, Lusseau D (2014) Female body condition affects foetal growth in a capital breeding mysticete. Funct Ecol 28:579–588
Christiansen F, Sironi M, Moore MJ, Di Martino M, Ricciardi M, Warick HA, Irschick DJ, Gutierrez R, Uhart MM (2019) Estimating body mass of free-living whales using aerial photogrammetry and 3D volumetrics. Methods Ecol Evol 10:2034–2044
Claeskens G, Hjort NL (2008) Model selection and model averaging. Cambridge University Press, Cambridge
Claeskens G, Cunen C, Hjort NL (2019) Model selection via focused information criteria for complex data in ecology and evolution. Front Ecol Evol 7:1–13. https://doi.org/10.3389/fevo.2019.00415
Cunen C, Walløe L, Hjort NL (2020) Focused model selection for linear mixed models with an application to whale ecology. Ann Appl Stat 14:872–904
de la Mare W (2011) Are reported trends in Antarctic minke whale body condition reliable? Paper SC/62/O16 presented to the IWC Scientific Committee
de la Mare W, McKinlay J, Welsh A (2017) Analyses of the JARPA Antarctic minke whale fat weight data set. Paper SC/67A/EM01 presented to the IWC Scientific Committee
Demidenko E (2013) Mixed models: theory and applications with R. Wiley, Hoboken
Folkow LP, Blix AS (1992) Metabolic rates of minke whales (Balaenoptera acutorostrata) in cold water. Acta Physiol Scand 146:141–150
Fox J, Weisberg S (2018) Visualizing fit and lack of fit in complex regression models with predictor effect plots and partial residuals. J Stat Softw 87:1–27. https://doi.org/10.18637/jss.v087.i09
Friedlaender AS, Lawson GL, Halpin PN (2009) Evidence of resource partitioning between humpback and minke whales around the western antarctic peninsula. Mar Mamm Sci 25:402–415
Gambell R (1976) World whale stocks. Mamm Rev 6:41–53
Hermansen GH, Hjort NL, Kjesbu OS (2016) Modern statistical methods applied on extensive historic data: Hjort liver quality time series 1859–2012 and associated influential factors. Can J Fish Aquat Sci 73:279–295. https://doi.org/10.1139/cjfas-2015-0086
Hjort J (1902) Fiskeri og hvalfangst i det nordlige Norge. J. Grieg, Bergen
Hjort J (1937) The story of whaling: a parable of sociology. Sci Mont 45:19–34
IWC (2008) Report of the intersessional workshop to review data and results from special permit research on minke whales in the Antarctic, Tokyo, 4–8 December 2006. J Cetacean Res Manag 10:411–445
IWC (2014) Report of the scientific committee: annex G—report of the sub-committee on in-depth assessments. J Cetacean Res Manag 15:233–249
IWC (2019) Whale population estimates. https://iwc.int/estimate. Accessed 10 Oct 2019
Jonsgård Å (1951) Studies on the little piked whale or minke whale (Balaenoptera Acutorostrata lacépède): report on Norwegian investigations carried out in the years 1943–1950. Norw Whal Gaz 40:80–96
Konishi K, Walløe L (2015) Substantial decline in energy storage and stomach fullness in Antarctic minke whales during the 1990s. J Cetacean Res Manag 15:77–92
Konishi K, Tamura T, Zenitani R, Bando T, Kato H, Walløe L (2008) Decline in energy storage in the Antarctic minke whale (Balaenoptera bonaerensis) in the Southern Ocean. Polar Biol 31:1509–1520. https://doi.org/10.1007/s00300-008-0491-3
Konishi K, Isoda T, Bando T, Minamikawa S, Kleivane L (2020) Antarctic minke whales find ice gaps along the ice edge in foraging grounds of the Indo-Pacific sector (60\(^{\circ }\) E and 140\(^{\circ }\) E) of the Southern Ocean. Polar Biol 343–357
Koopman HN (2007) Phylogenetic, ecological, and ontogenetic factors influencing the biochemical structure of the blubber of odontocetes. Mar Biol 151:277–291
Laws RM (1962) Some effects of whaling on the southern stocks of baleen whales. In: Le Cren ED, Holgate MW (eds) The exploitation of natural animal populations. Wiley, New York, pp 137–158
Laws RM (1977) Seals and whales of the Southern Ocean. Philos Trans R Soc Lond B 279:81–96. https://doi.org/10.1098/rstb.1977.0073
Lee JF, Friedlaender AS, Oliver MJ, DeLiberty TL (2017) Behavior of satellite-tracked Antarctic minke whales (Balaenoptera bonaerensis) in relation to environmental factors around the western Antarctic Peninsula. Anim Biotelem 5:1–12
Lockyer C (1972) The age at sexual maturity of the southern fin whale (Balaenoptera physalus) using annual layer counts in the ear plug. ICES J Mar Sci 34:276–294
Lockyer C (1981) Growth and energy budgets of large baleen whales from the southern hemisphere. Mammals in the Seas, vol 3. Food and Agriculture Organization of the United Nations, Rome, pp 379–487
Lockyer C (1986) Body fat condition in Northeast Atlantic fin whales, Balaenoptera physalus, and its relationship with reproduction and food resource. Can J Fish Aquat 43:142–147
Lockyer C (1991) Body composition of the sperm whale, Physeter catodon, with special reference to the possible functions of fat depots. Rit Fisk 12:1–24
Lockyer C, McConnell LC, Waters TD (1984) The biochemical composition of fin whale blubber. Can J Zool 62:2553–2562. https://doi.org/10.1139/z84-373
McKinlay J, de la Mare W, Welsh A (2017) A re-examination of minke whale body condition as reflected in the data. Paper SC/67A/EM02 presented to the IWC Scientific Committee
McKinlay J, de la Mare W, Welsh A (2018) Issues Cunen, Walløe and Hjort should consider in relation to their analyses of Antarctic minke whale body condition. Paper SC/67B/EM01 presented to the IWC Scientific Committee
Miller CA, Reeb D, Best PB, Knowlton AR, Brown MW, Moore MJ (2011) Blubber thickness in right whales Eubalaena glacialis and Eubalaena australis related with reproduction, life history status and prey abundance. Mar Ecol Prog Ser 438:267–283
Murase H, Kitakado T, Hakamada T, Matsuoka K, Nishiwaki S, Naganobu M (2013) Spatial distribution of Antarctic minke whales (Balaenoptera bonaerensis) in relation to spatial distributions of krill in the Ross sea, Antarctica. Fish Oceanogr 22:154–173. https://doi.org/10.1111/fog.12011
Nicol S, Worby A, Leaper R (2008) Changes in the Antarctic sea ice ecosystem: potential effects on krill and baleen whales. Mar Freshw Res 59:361–382
Parry DA (1949) The structure of whale blubber, and a discussion of its thermal properties. J Cell Sci 3:13–25
Pastene LA, Goto M (2016) Genetic characterization and population genetic structure of the Antarctic minke whale (Balaenoptera bonaerensis) in the Indo-Pacific region of the Southern Ocean. Fish Sci 82:873–886. https://doi.org/10.1007/s12562-016-1025-5
Payne MR (1977) Growth of a fur seal population. Philos Trans R Soc Lond B 279:67–79
Pinheiro J, Bates D (2000) Mixed-effects models in S and S-PLUS. Springer, New York
Pitman RL, Durban JW, Joyce T, Fearnbach H, Panigada S, Lauriano G (2020) Skin in the game: epidermal molt as a driver of long-distance migration in whales. Mar Mamm Sci 36:565–594
Riekkola L, Zerbini AN, Andrews O, Andrews-Goff V, Baker CS, Chandler D, Childerhouse S, Clapham P, Dodémont R, Donnelly D et al (2018) Application of a multi-disciplinary approach to reveal population structure and Southern Ocean feeding grounds of humpback whales. Ecol Indic 89:455–465
Schweder T, Hjort NL (2016) Confidence, likelihood, probability: statistical inference with confidence distributions. Cambridge University Press, Cambridge
Sladen WJL (1964) The distribution of the adelie and chinstrap penguins. In: Carrick R, Holdgate MW, Prevost J (eds) Biologie antarctique. Hermann, Paris, pp 359–365
Thomson RB, Butterworth DS, Kato H (1999) Has the age at transition of Southern Hemisphere minke whales declined over recent decades? Mar Mamm Sci 15:661–682. https://doi.org/10.1111/j.1748-7692.1999.tb00835.x
Tynan CT, Russell JL (2008) Assessing the impacts of future 2 \(^{\circ }\text{C}\) global warming on southern ocean cetaceans. Paper SC/60/E3 presented to the IWC Scientific Committee
Zuur A, Ieno EN, Walker N, Saveliev AA, Smith GM (2009) Mixed effects models and extensions in ecology with R. Springer, New York
Acknowledgements
The authors thank the Institute of Cetacean Research for obtaining the body condition data of Antarctic minke whale and the IWC Scientific Committee’s Data Availability Group (DAG) for facilitating the access to the data. We are also deeply grateful for detailed comments from two reviewers.
Funding
Parts of this research have been funded by the Norwegian Research Council, via the five-year programme FocuStat (Grant No. 235116), Focus Driven Statistical Inference with Complex Data, led by Hjort.
Author information
Authors and Affiliations
Contributions
All authors contributed to the study conception and design. KK was central in the data collection. Data analyses have been conducted by CC, using methodology developed by CC, LW and NLH. The first draft of the manuscript was written by CC, LW and NLH. All authors commented on previous versions of the manuscript. All authors read and approved the final manuscript.
Corresponding author
Ethics declarations
Conflict of interest
The authors declare that they have no conflicts of interest.
Additional information
Publisher's Note
Springer Nature remains neutral with regard to jurisdictional claims in published maps and institutional affiliations.
The research reported here involved lethal sampling of Antarctic minke whales, which was based on a permit issued by the Japanese Government in terms of Article VIII of the International Convention for the Regulation of Whaling. Reasons for the scientific need for this sampling have been stated by the Japanese Government. The species under study is not classified by the IUCN as ‘endangered’ or ‘vulnerable’.
Supplementary information
Below is the link to the electronic supplementary material.
Rights and permissions
Open Access This article is licensed under a Creative Commons Attribution 4.0 International License, which permits use, sharing, adaptation, distribution and reproduction in any medium or format, as long as you give appropriate credit to the original author(s) and the source, provide a link to the Creative Commons licence, and indicate if changes were made. The images or other third party material in this article are included in the article's Creative Commons licence, unless indicated otherwise in a credit line to the material. If material is not included in the article's Creative Commons licence and your intended use is not permitted by statutory regulation or exceeds the permitted use, you will need to obtain permission directly from the copyright holder. To view a copy of this licence, visit http://creativecommons.org/licenses/by/4.0/.
About this article
Cite this article
Cunen, C., Walløe, L., Konishi, K. et al. Decline in body condition in the Antarctic minke whale (Balaenoptera bonaerensis) in the Southern Ocean during the 1990s. Polar Biol 44, 259–273 (2021). https://doi.org/10.1007/s00300-020-02783-3
Received:
Revised:
Accepted:
Published:
Issue Date:
DOI: https://doi.org/10.1007/s00300-020-02783-3