Abstract
Background
Osteoarthritis is the most common degenerative joint disease. It is associated with significant socioeconomic burden and poor quality of life, mainly due to knee osteoarthritis (KOA), and related total knee arthroplasty (TKA). Since early detection method and disease-modifying drug is lacking, the key of KOA treatment is shifting to disease prevention and progression slowing. The prognostic prediction models are called for to guide clinical decision-making. The aim of our review is to identify and characterize reported multivariable prognostic models for KOA about three clinical concerns: (1) the risk of developing KOA in the general population, (2) the risk of receiving TKA in KOA patients, and (3) the outcome of TKA in KOA patients who plan to receive TKA.
Methods
The electronic datasets (PubMed, Embase, the Cochrane Library, Web of Science, Scopus, SportDiscus, and CINAHL) and gray literature sources (OpenGrey, British Library Inside, ProQuest Dissertations & Theses Global, and BIOSIS preview) will be searched from their inception onwards. Title and abstract screening and full-text review will be accomplished by two independent reviewers. The multivariable prognostic models that concern on (1) the risk of developing KOA in the general population, (2) the risk of receiving TKA in KOA patients, and (3) the outcome of TKA in KOA patients who plan to receive TKA will be included. Data extraction instrument and critical appraisal instrument will be developed before formal assessment and will be modified during a training phase in advance. Study reporting transparency, methodological quality, and risk of bias will be assessed according to the TRIPOD statement, CHARMS checklist, and PROBAST tool, respectively. Prognostic prediction models will be summarized qualitatively. Quantitative metrics on the predictive performance of these models will be synthesized with meta-analyses if appropriate.
Discussion
Our systematic review will collate evidence from prognostic prediction models that can be used through the whole process of KOA. The review may identify models which are capable of allowing personalized preventative and therapeutic interventions to be precisely targeted at those individuals who are at the highest risk. To accomplish the prediction models to cross the translational gaps between an exploratory research method and a valued addition to precision medicine workflows, research recommendations relating to model development, validation, or impact assessment will be made.
Systematic review registration
PROSPERO CRD42020203543
Similar content being viewed by others
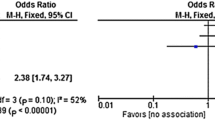
Background
Osteoarthritis, a major source of pain, disability, and socioeconomic cost worldwide, is the most common degenerative joint disease leading to substantial and growing burden, and a large proportion of patients suffering from osteoarthritis is due to knee osteoarthritis (KOA) [1,2,3]. It has been estimated that healthcare costs of osteoarthritis account for about 1 to 2.5% of national gross domestic product, mainly driven by knee joint replacement, in particular, total knee arthroplasty (TKA) [2]. As the difficulty of being detected early and deficiency of disease-modifying drug [2, 3], the focus of KOA is shifting to disease prevention and the treatment to delay its rapid progression. Here, the prognostic prediction models are called for to distinguish individuals who are at higher risk of development or progression of KOA and who are more likely to acquire a better quality of life after TKA, which in turn could be used to guide clinical decision-making.
Firstly, prevention is the best cure. Although the etiology of KOA has not been fully elucidated, a combination of risk factors is deemed to be related to this disease [4, 5], which allows the establishment of KOA risk prediction models. The evolving understanding of the pathophysiological aspect of KOA [2, 3] is paralleled by improvements in prediction models, from only considering limited factors to a model combined clinical, genetic, biochemical, and imaging information [6,7,8,9,10]. Losina et al. [6] developed an interactive KOA risk calculator only based on a set of demographic and clinical factors and select risk factors; further, Kerkhof et al. [7] include genetic and imaging information and found that doubtful minor radiographic degenerative features in the knee are a very strong predictor of future KOA. Zhang et al. [8] developed models based on radiographic assessments and risk factors to separately predict radiographic KOA and incidence of symptomatic KOA, while Joseph et al. [10] combined radiograph and magnetic resonance imaging for KOA prediction. An artificial neural network method was also introduced into future KOA prediction by Yoo et al. [9]. Prognostic models that showed moderate performance in evaluating KOA risk in the general population may serve as a potential applicable tool for clinicians to stratify individuals by their risk level to provide a suitable prevention strategy.
Secondly, current widely available diagnostic modalities do not fulfill the needs of clinicians to reduce the prognosis of KOA patients [11]. Therefore, the development and validation of prediction models that are capable of identifying KOA patients at high risk of rapid progression is now recognized as a priority [12, 13]. TKA is the only available treatment option for KOA patients at the end stage, and most of healthcare costs attributed to KOA are brought by this approach [2, 4]. Thus, it is preferable for KOA patients to delay TKA and to prolong the good health of their knees. Several models concerning TKA risk in KOA patients that conducted based on clinical information are reported, whose performance could be improved with the introduction of imaging data [14,15,16,17,18]. Chan et al. [14] developed a formula reflecting the decision for TKA in patients with a painful KOA based on clinical and radiographic information, while Yu et al. [15] automatically extracted patient data from electronic records to allow individuals TKA risk estimation. Machine learning and deep learning methods were also used in building prediction models for identifying KOA patients at high risk of TKA [16,17,18]. Such models are necessitated for clinicians to pursue appropriate treatment options.
Thirdly, although TKA is a cost-effective surgical procedure that can advance the quality of life [19], up to one third of KOA patients did not satisfy with their clinical outcomes [20]. A series of systematic reviews are conducted to report pooled survival of knee replacement [21], but a study that concentrates on prediction models for TKA outcomes has not been performed so far. The measures of prognostic models regarding TKA outcome vary [22,23,24,25]. Models include sociodemographic, psychosocial, clinical, functional, and quality-of-life measures for predicting pain, stiffness, and functional status [22, 25]; persistent mobility limitations [23]; and post-operation satisfaction [24] for KOA patients after TKA. As the lack of agreement respecting indications for TKA currently [26], it is of significance to develop prediction models for aiding clinicians to KOA patient selection and therapeutic decision-making in TKA.
In spite of the huge amount of prediction models for KOA established, none of them is widely accepted as an addition to precision medicine workflows. A systematic review of various models is helpful for improving their methodological quality and is necessary before their translation into clinical practice [27, 28]. It is timely to conduct a critical appraisal thorough specialized tools for prediction models for KOA [29,30,31,32]. Further, to provide a whole view of current prognostic models for KOA, we will include three sorts of models which run thorough clinical practice procedures of KOA [2, 3, 11, 27, 29].
This study will systematically review the prognostic models for the development and prognosis of KOA. The framing of the review question, study identification, data collection, critical appraisal, data synthesis, and result interpretation and reporting will be conducted according to previous guidelines and several developments in prediction model research methodology [29,30,31,32,33,34,35,36,37,38]. We plan to systematically review prognostic models aiming (1) to predict KOA risk in the general population, (2) to predict TKA risk in KOA patient, and (3) to predict TKA-related outcomes or complications in KOA patients who intend to receive TKA, respectively, while studies reporting prognostic models with other objectives will not be considered. We aim to map their characteristics; to critically appraise their reporting transparency, methodological quality, and risk of bias; and to meta-analyze their performance measures if possible.
Methods/design
Study design
This protocol is reported according to the Preferred Reporting Items for Systematic Reviews and Meta-Analyses Protocols (PRISMA-P) statement [39], and the corresponding checklist can be found in Additional file 1. This protocol was registered on the International Prospective Register of Systematic Reviews (PROSPERO) (CRD42020203543; Additional file 2) [40].
Key items of this review are clarified with assistance of the CHARMS checklist [31] (Additional file 3 Supplementary Table 1). A prognostic model will be defined as a combination of two or more predictors within statistical methods, machine learning methods, or deep learning methods [33], which is used to predict the risk of the future outcome, and may help the health professionals and patients approach appropriate therapeutic decision. Studies investigated the association between a single risk factor and the outcome will be excluded, as they are limited in their utility for individual risk prediction. Specially, machine learning models in medical imaging, although they are usually based only on one modality, will be considered as multivariable, if multiple features have been extracted or deep learning methods have been employed. Studies reporting the following types of prognostic models will be eligible for inclusion for our review: prediction model development with validation or external model validation. Studies that have developed prognostic models without validation will not be included into the analysis, but records of these studies will be kept.
Study inclusion
Eligibility criteria
Prognostic prediction models concern the prediction of the probability or risk of the future occurrence of a particular outcome or event in individuals at risk of such an event [29]. PICOTS (Population, Intervention, Comparison, Outcome, Timing, Setting) approach will be used to frame the eligibility criteria and to guide the selection of prognostic prediction models with three different aims, separately [29, 30] (Additional file 3 Supplementary Table 2). PICOTS approach is modified from PICO (Population, Intervention, Comparison, Outcome) approach, which additionally considers timing, i.e., specifically for prognostic models, when and over what time period the outcome is predicted, and setting, i.e., the intended role or setting of the prediction model.
We further established eligibility criteria as follows. (1) Study design: we will include randomized controlled trials and observational studies, such as prospective or retrospective cohort studies, case-control studies, and cross-sectional studies. (2) Countries and regions: we will consider studies from all countries and regions. (3) Journal: we will consider studies from peer-reviewed journals of all research fields, which are representative of the high-quality studies on prognostic models for KOA. (4) Publish period: we will include only studies published after 2000, to display the current status of prediction modeling studies for KOA. Furthermore, the prediction model building approaches have significantly improved in the last two decades, particularly the machine learning methods and leading-edge deep learning methods. (5) Language: we will include studies published in English, Chinese, Japanese, German, or French. One reviewer has expertise in those five languages. (6) Publication type: we will include only peer-reviewed full-text studies with original results, as they are expected to exhibit high-quality models and detailed methodology. Therefore, we will not consider abstracts only, conference abstracts, short communications, correspondences, letters, or comments and do not intend to search the gray literature. Any identified and relevant review articles will be used to identify eligible primary studies.
Information sources and search strategy
We will search the following seven electronic databases from their inception onwards, including PubMed, Embase, the Cochrane Library, Web of Science, Scopus, SportDiscus, and Cumulative Index of Nursing and Allied Health Literature (CINAHL) [41,42,43,44,45,46,47]. SportDiscus is the leading bibliographic database for sports and sports medicine research, and CINAHL is the largest collection of full text for nursing and allied health journals in the world. They will be included into the electronic database search because nursing and sports medicine professionals are also interested in the management of KOA patients, and these two databases were searched as routine in previous studies [48]. Four gray literature sources will also be included as information sources, namely OpenGrey, British Library Inside, ProQuest Dissertations & Theses Global, and BIOSIS preview [49,50,51,52]. The authors of potential available studies will be contacted to request information about undergoing researches.
Search keywords will be selected from the MeSH terms and appropriate synonyms, based on the review question clarified by the PICOTS approach, including three concept terms: “knee,” “osteoarthritis,” and “prediction model.” Each concept will be searched by MeSH term and free words combined with the OR Boolean operator, and then the three concepts will be combined with the AND Boolean operator. For each database, keywords will be translated into controlled vocabulary (MeSH, Emtree, and others) and will be chosen from free text. We will take search strategies in former studies as reference [48] and will co-design the search strategy. The search strategies will be tested for eligibility by two reviewers before formal search. A draft search strategy is presented in Additional file 4.
The formal search will be performed by two same reviewers according to the PRESS guideline [34]. In case of uncertainties, a third reviewer was consulted to reach a final consensus. The reference list of included studies and relevant reviews will be hand-searched for additional potentially relevant citations. However, we do not intend to search gray literature due to concerns on their methodological quality.
Data management
We will use Endnote reference manager software version X9.2 (Clarivate Analytics, Philadelphia, PA, USA) [53] to merge the retrieved studies. Duplicates will be removed using a systematic, rigorous, and reproducible method utilizing a sequential combination of fields including author, year, title, journal, and pages [35]. We will use a free online Tencent Document software (Tencent, Shenzhen, China) [54] to manage records throughout the review, to make sure all reviewers follow the latest status of the review process timely, and to ensure two senior reviewers can supervise the process remotely during the difficult period of the coronavirus disease 2019 pandemic.
Study selection
Two independent reviewers will screen the titles and abstracts of all the potential records to identify all relevant studies using the pre-defined inclusion and exclusion criteria. In case of an unavailable abstract, full-text articles will be obtained unless the title is clearly irrelevant. Two same reviewers will obtain the full text and supplementary materials of all selected records and will thoroughly read them independently, to further determine their eligibility before extracting data. The corresponding authors of potential records may be contacted to request the full text if it is not available otherwise. Disagreements will be resolved by consensus to reach the final decision, with assistance from our review group consisting of a computer engineer with experience in prediction model building, an orthopedist with experience in OA management, and musculoskeletal radiologists.
Data collection
Data extraction
We will develop a data extraction instrument for study data based on several previous systematic reviews of the prediction model [55,56,57]. A draft data extraction instrument is presented in Additional file 3 Supplementary Table 3. As the reviewers have different levels of experience and knowledge, the items listed will be reviewed and discussed to ensure that all reviewers had clear knowledge of the procedures. A training phase will be introduced before the formal extraction.
During the training phase, two randomly chosen articles from all articles that fulfilled the inclusion criteria for discussion will be used to train two independent reviewers. They will thoroughly read the two randomly chosen articles including the supplementary materials and will measure each study independently. A structured data collection instrument will be modified and used to help them reach agreement. Disagreements will be discussed in order to achieve a shared understanding of each parameter. This pre-defined and piloted data extraction instrument will be used in the formal data extraction phase.
During the formal extraction phase, two independent reviewers will thoroughly read all articles including the supplementary materials, to extract the data from the studies to describe their characteristics. Any disagreement will be resolved by discussion to reach a consensus and consultation with other members of our review group if required. Missing data will be obtained from the authors wherever possible; studies with insufficient information will be noted.
Critical appraisal
We will develop a critical appraisal instrument according to the TRIPOD statement, CHARMS checklist, and PROBAST tool [30,31,32]. The TRIPOD is a set of recommendations, deemed essential for transparent reporting of a prediction model study, and allows the quality evaluation and potential usefulness analysis. The CHARMS checklist identifies eleven domains to facilitate a structured critical appraisal of primary studies on prediction models, mainly focus on the methodological quality of included models. The PROBAST tool is designed for assessing the risk of bias and applicability concerning four domains, i.e., participants, predictors, outcome, and analysis, with a total of 20 signaling questions. These three instruments, although focus on different aspects of prediction model studies, overlap each other in several domain and items. Therefore, we will merge them into a critical appraisal instrument to reduce the workload during the systemic critical evaluation.
During the development period of this instrument, we also considered machine learning and deep learning relevant checklists, e.g., radiomics quality score [58], Checklist for Artificial Intelligence in Medical Imaging [59], and Guidelines for Developing and Reporting Machine Learning Predictive Models in Biomedical Research [60], all of which are specialized assessment tools for cutting-edge artificial intelligence models. However, they include many items that may not be available for prediction models built with traditional statistical methods based on clinical characteristics, laboratory examinations, or genetic factors. On the other hand, TRIPOD, CHARMS, and PROBAST have been already proved suitable for assessing prediction models using artificial intelligence methods [57]. Thus, we will choose three more widely adapted and more extensively accepted tools, to develop our critical appraisal instrument.
A similar training phase is introduced before the formal critical appraisal, to ensure its eligibility and to achieve a shared understanding of each parameter. During the formal evaluation phase, two independent reviewers will assess all the articles and corresponding supplementary materials, to measure and rate all studies according to established criteria. Any disagreement will be solved as described before.
Data pre-processing
The necessary results or performance measures and their precision are needed to allow quantitative synthesis of the predictive performance of the prediction model under study [29]. However, model performance measurements vary among reported prediction model studies and sometimes are unreported or inconsistent for further analysis. In cases where pertinent information is not reported, efforts will be made to contact study authors to request this information. If there is any non-response, missing performance measures and their measures of precision will be calculated if possible, according to the methods previously described [29]. If this is impossible due to limited data, the exclusion of the study will be determined by discussion among the reviewers.
Data synthesis
The data synthesis process will be guided by serval methodological reference books and guidelines [29, 61,62,63,64]. Two reviewers of this study have significant expertise in statics and meta-analysis methods that would be used in this review. In case of doubt, the reviewers will discuss to approach consensus or consult a statistician for advice.
Narrative synthesis
All extracted data on prediction models will be narratively summarized, and the key findings will be tabulated to facilitate comparison according to the PICOTS approach [30], and in particular, what prediction factors were included in different models, when and how the included variables were coded, what the outcomes of models were, the reported predictive accuracy of the model, and whether the model was validated internally and/or externally, and if so, how. Heterogeneity among models will be explored by summary tables including model characteristics, their risk of bias, and whether the models were validated in an external population. Models relating to different aims will be considered separately.
Quantitative synthesis
The two most common statistical measures of predictive performance, discrimination (such as area under the receiver operating characteristic curve, concordance statistic, sensitivity, specificity, positive predictive value, and negative predictive value) and calibration (such as observed and expected events, observed and expected ratio, calibration slope), will be reported when published or approximated using published methods [30]. Individual results of CHARMS, TRIPOD, and PROBAST and the overall reporting transparency, methodological quality, and risk of bias will be reported [30,31,32].
The statistics analysis will be performed via SPSS software version 26.0 (SPSS Inc., Chicago, IL, USA) [65]. p-value < 0.05 will be recognized as statistical significance, unless otherwise specified. The elements of TRIPOD will be treated as binary categorical variables, with their inter-rater agreement assessed by Cohen’s kappa statistic [66]. The elements of CHARMS and PROBAST include ordinal categories with more than two possible ratings; therefore, Fleiss’ kappa statistic will be used to assess their inter-rater agreement [67]. The summed TRIPOD rating will be treated as a continuous variable, and their inter-rater agreement will be assessed using the interclass correlation coefficient (ICC) [68]. Further, we will provide correlation information among these three instruments to present whether they are complimentary critiques [69], where possible.
Meta-analysis
Studies would be included in a meta-analysis of a large enough subset of the included studies if a similar clinical question was assessed repeatedly (≥ 5 studies). The meta-analysis will be conducted via Stata/SE software version 15.1 (Stata Corp., College Station, TX, USA) with the metan, midas, and metandi packages [70,71,72,73] and any other packages depending on the data we extract. The plan of the meta-analysis will be dependent on the studies identified in the systematic review. If a similar clinical question was assessed repeatedly in a large enough subset of the included studies, meta-analysis will be considered to jointly summarize calibration and discrimination statics with their 95% confidence intervals to obtain average model performance. Relevant forest plots and a hierarchical summary receiver operating characteristic (HSROC) curve will be obtained to visually show the model performance [74].
For assessment of heterogeneity between the meta-analyzed studies, Cochran’s Q and the I2 statistic will be calculated [75]. Difference between the 95% confidence region and prediction region in the HSROC curve was used to visually assess the heterogeneity, and a large difference indicates the presence of heterogeneity [74]. Potential sources of heterogeneity will be investigated by means of meta-regression if there are > 10 studies included in the meta-analysis [76].
Metabiases
Publication biases arise when the dissemination of research findings is influenced by the nature and direction of results. A Deeks funnel plot will be generated to visually assessed publication bias if there are > 10 studies included in the meta-analysis [77, 78]. An Egger’s test was performed to assess the publication bias, and a p-value > 0.10 indicated a low publication bias [79]. A Deeks funnel plot asymmetry test was also constructed to explore the risk of publication bias, and a p-value > 0.10 indicated a low publication bias [80]. The trim and fill method will be conducted to estimate the number of missing studies [81].
Subgroup analysis
A common aim of prognostic studies concerns the development of prognostic prediction models or indices by combining information from multiple prognostic factors via multiple methods [30,31,32]. Whether the model performed a validation, the input predictor, and the model building method may introduce heterogeneity. Therefore, we plan to carry out the following subgroup analyses for exploring potential sources of heterogeneity [78]: (1) the type of model validation: internal validation or external validation; (2) the predictor of model: clinical characteristics, laboratory examinations, genetic factors, objective or quantitative-extracted imaging feature, or their combinations; and (3) the method of prognostic model building: statistic method, machine learning method, or deep learning method, etc. Further subgroup analysis will depend on the data extracted.
Sensitivity analysis
Sensitivity analyses will be performed by excluding studies with a high risk of bias assessed by the PROBAST tool (at least 4/7 domain to be high), studies with high methodological quality assessed by the CHARMS checklist (at least 6/11 domain to be high), and studies with low reporting transparency assessed by the TRIPOD statement (at least half of available items not to be mentioned), to explore their influence on effect size. This analysis will be a narrative summary that covers the same elements as the primary analysis if appropriate.
Reporting and dissemination
The results of the review will be reported guided by the Preferred Reporting Items for Systematic Reviews and Meta-Analyses (PRISMA) statement [36]. The confidence in estimates will be determined according to the GRADE approach (Grades of Recommendation, Assessment, Development, and Evaluation) [37, 38]. The approval of ethics and consent to participate are not required for our study due to its nature of systematic review and meta-analysis. Our findings will be disseminated through peer-reviewed publications, and presentation at conferences if possible. Any amendments made to this protocol when conducting the study will be outlined in PROSPERO and in the final manuscript.
Discussion
This systematic review will identify all published prognostic prediction models for three important KOA-related clinical questions. These prognostic prediction models will be comprehensively summarized and critically appraised. Their performance will be meta-analyzed if appropriate and further compared across pre-defined subgroups.
The major strength of our study is that it will provide a bird view of the prognosis prediction model for this disease and may point out future research directions for this field. Next, our review team is composed of musculoskeletal radiologists, an orthopedist, and a computer engineer, which allows us to share our knowledge and expertise. We will introduce a training phase to reach a better understanding of included studies and used assessment tools. Then, the data extraction and critical appraisal instruction may become a reference for future reviews. Finally, we will calculate the inter-rater agreements which are seldom reported by previous reviews. This may improve the transparency and quality of our review.
Our study has several limitations. Firstly, we will exclude several existing models for KOA patients regarding valuable aims apart from our set three clinical questions, such as knee pain, KOA progression, and response to other treatments. Secondly, we will only consider models predicting clinical outcomes, but neither socioeconomic burdens nor cost-effective aspects which are important in model practical translation. Thirdly, the predictive models may report their performance in various ways, and the reconstruction process of the data may introduce additional bias. Fourthly, the predictive model building is a complicated process which needs medical, statistical, and programming knowledge; therefore, physicians or surgeons alone have not enough expertise to assess the models. Our team includes physicians, surgeons, statisticians, and programming experts to allow the review. Fifthly, there may be limited studies that meet our eligibility criteria, which allow us to perform a meta-analysis. Finally, the instruments that we will use have limitations. While the sum score of TRIPOD is a quantitative metric, the CHARMS and PROBAST are qualitative scores and therefore less easily interpretable.
To summarize, our systematic review will be an important step towards developing and applying prognostic prediction models that can be used through the whole process of KOA. This will allow personalized preventative and therapeutic interventions to be precisely targeted at individuals at highest risk and to avoid harm and additional expense for those who are not.
Availability of data and materials
Not applicable
Abbreviations
- CHARMS:
-
CHecklist for critical Appraisal and data extraction for systematic Reviews of prediction Modelling Studies
- CINAHL:
-
Cumulative Index of Nursing and Allied Health Literature
- GRADE:
-
Grades of Recommendation, Assessment, Development, and Evaluation
- ICC:
-
Interclass correlation coefficient
- KOA:
-
Knee osteoarthritis
- PICO:
-
Population, Intervention, Comparison, Outcome
- PICOTS:
-
Population, Intervention, Comparison, Outcome, Timing, Setting
- PRESS:
-
Peer Review of Electronic Search Strategies
- PRISMA-P:
-
Preferred Reporting Items for Systematic Reviews and Meta-Analyses Protocols
- PROBAST:
-
Prediction model Risk Of Bias ASsessment Tool
- PROSPERO:
-
International Prospective Register of Systematic Reviews
- TKA:
-
Total knee arthroplasty
- TRIPOD:
-
Transparent Reporting of a multivariable prediction model for Individual Prognosis Or Diagnosis
References
Safiri S, Kolahi AA, Smith E, Hill C, Bettampadi D, Mansournia MA, et al. Global, regional and national burden of osteoarthritis 1990-2017: a systematic analysis of the Global Burden of Disease Study 2017. Ann Rheum Dis. 2020;79(6):819–28. https://doi.org/10.1136/annrheumdis-2019-216515.
Hunter DJ, Bierma-Zeinstra S. Osteoarthritis. Lancet. 2019;393(10182):1745–59. https://doi.org/10.1016/S0140-6736(19)30417-9.
Martel-Pelletier J, Barr AJ, Cicuttini FM, Conaghan PG, Cooper C, Goldring MB, et al. Osteoarthritis. Nat Rev Dis Primers. 2016;2(1):16072. https://doi.org/10.1038/nrdp.2016.72.
Palazzo C, Nguyen C, Lefevre-Colau MM, Rannou F, Poiraudeau S. Risk factors and burden of osteoarthritis. Ann Phys Rehabil Med. 2016;59(3):134–8. https://doi.org/10.1016/j.rehab.2016.01.006.
Cooper C, Snow S, McAlindon TE, et al. Risk factors for the incidence and progression of radiographic knee osteoarthritis. Arthritis Rheum. 2000;43(5):995–1000. https://doi.org/10.1002/1529-0131(200005)43:5<995::AID-ANR6>3.0.CO;2-1.
Losina E, Klara K, Michl GL, Collins JE, Katz JN. Development and feasibility of a personalized, interactive risk calculator for knee osteoarthritis. BMC Musculoskelet Disord. 2015;16(1):312. https://doi.org/10.1186/s12891-015-0771-3.
Kerkhof HJ, Bierma-Zeinstra SM, Arden NK, et al. Prediction model for knee osteoarthritis incidence, including clinical, genetic and biochemical risk factors. Ann Rheum Dis. 2014;73(12):2116–21. https://doi.org/10.1136/annrheumdis-2013-203620.
Zhang W, McWilliams DF, Ingham SL, et al. Nottingham knee osteoarthritis risk prediction models. Ann Rheum Dis. 2011;70(9):1599–604. https://doi.org/10.1136/ard.2011.149807.
Yoo TK, Kim DW, Choi SB, Oh E, Park JS. Simple scoring system and artificial neural network for knee osteoarthritis risk prediction: a cross-sectional study. PLoS One. 2016;11(2):e0148724. https://doi.org/10.1371/journal.pone.0148724.
Joseph GB, McCulloch CE, Nevitt MC, et al. Tool for osteoarthritis risk prediction (TOARP) over 8 years using baseline clinical data, X-ray, and MRI: data from the osteoarthritis initiative. J Magn Reson Imaging. 2018;47(6):1517–26. https://doi.org/10.1002/jmri.25892.
Jamshidi A, Pelletier JP, Martel-Pelletier J. Machine-learning-based patient-specific prediction models for knee osteoarthritis. Nat Rev Rheumatol. 2019;15(1):49–60. https://doi.org/10.1038/s41584-018-0130-5.
Bruyère O, Cooper C, Arden N, Branco J, Brandi ML, Herrero-Beaumont G, et al. Can we identify patients with high risk of osteoarthritis progression who will respond to treatment? A focus on epidemiology and phenotype of osteoarthritis. Drugs Aging. 2015;32(3):179–87. https://doi.org/10.1007/s40266-015-0243-3.
Arden N, Richette P, Cooper C, Bruyère O, Abadie E, Branco J, et al. Can we identify patients with high risk of osteoarthritis progression who will respond to treatment? A focus on biomarkers and frailty. Drugs Aging. 2015;32(7):525–35. https://doi.org/10.1007/s40266-015-0276-7.
Chan WP, Hsu SM, Huang GS, Yao MS, Chang YC, Ho WP. Creation of a reflecting formula to determine a patient’s indication for undergoing total knee arthroplasty. J Orthop Sci. 2010;15(1):44–50. https://doi.org/10.1007/s00776-009-1418-8.
Yu D, Jordan KP, Snell KIE, Riley RD, Bedson J, Edwards JJ, et al. Development and validation of prediction models to estimate risk of primary total hip and knee replacements using data from the UK: two prospective open cohorts using the UK Clinical Practice Research Datalink. Ann Rheum Dis. 2019;78(1):91–9. https://doi.org/10.1136/annrheumdis-2018-213894.
Tiulpin A, Klein S, Bierma-Zeinstra SMA, Thevenot J, Rahtu E, Meurs J, et al. Multimodal machine learning-based knee osteoarthritis progression prediction from plain radiographs and clinical data. Sci Rep. 2019;9(1):20038. https://doi.org/10.1038/s41598-019-56527-3.
Tolpadi AA, Lee JJ, Pedoia V, Majumdar S. Deep learning predicts total knee replacement from magnetic resonance images. Sci Rep. 2020;10(1):6371. https://doi.org/10.1038/s41598-020-63395-9.
Leung K, Zhang B, Tan J, et al. Prediction of total knee replacement and diagnosis of osteoarthritis by using deep learning on knee radiographs: data from the osteoarthritis initiative. Radiology. 2020;296(3):584–93. https://doi.org/10.1148/radiol.2020192091.
Murray DW, MacLennan GS, Breeman S, et al. A randomised controlled trial of the clinical effectiveness and cost-effectiveness of different knee prostheses: the Knee Arthroplasty Trial (KAT). Health Technol Assess. 2014;18(19):i–viii.
Bullens PH, van Loon CJ, de Waal Malefijt MC, Laan RF, Veth RP. Patient satisfaction after total knee arthroplasty: a comparison between subjective and objective outcome assessments. J Arthroplasty. 2001;16(6):740–7. https://doi.org/10.1054/arth.2001.23922.
Evans JT, Walker RW, Evans JP, Blom AW, Sayers A, Whitehouse MR. How long does a knee replacement last? A systematic review and meta-analysis of case series and national registry reports with more than 15 years of follow-up. Lancet. 2019;393(10172):655–63. https://doi.org/10.1016/S0140-6736(18)32531-5.
Lungu E, Desmeules F, Dionne CE, Belzile EL, Vendittoli PA. Prediction of poor outcomes six months following total knee arthroplasty in patients awaiting surgery. BMC Musculoskelet Disord. 2014;15(1):299. https://doi.org/10.1186/1471-2474-15-299.
Pua YH, Seah FJ, Clark RA, Poon CL, Tan JW, Chong HC. Development of a prediction model to estimate the risk of walking limitations in patients with total knee arthroplasty. J Rheumatol. 2016;43(2):419–26. https://doi.org/10.3899/jrheum.150724.
Van Onsem S, Van Der Straeten C, Arnout N, Deprez P, Van Damme G, Victor J. A new prediction model for patient satisfaction after total knee arthroplasty. J Arthroplasty. 2016;31(12):2660–7. https://doi.org/10.1016/j.arth.2016.06.004.
Shim J, Mclernon DJ, Hamilton D, Simpson HA, Beasley M, Macfarlane GJ. Development of a clinical risk score for pain and function following total knee arthroplasty: results from the TRIO study. Rheumatol Adv Pract. 2018;2(2):rky021.
Cross WW 3rd, Saleh KJ, Wilt TJ, Kane RL. Agreement about indications for total knee arthroplasty. Clin Orthop Relat Res. 2006;446:34–9. https://doi.org/10.1097/01.blo.0000214436.49527.5e.
Steyerberg EW, Vergouwe Y. Towards better clinical prediction models: seven steps for development and an ABCD for validation. Eur Heart J. 2014;35(29):1925–31. https://doi.org/10.1093/eurheartj/ehu207.
Panken G, Verhagen AP, Terwee CB, Heymans MW. Clinical prediction models for patients with nontraumatic knee pain in primary care: a systematic review and internal validation study. J Orthop Sports Phys Ther. 2017;47(8):518–29. https://doi.org/10.2519/jospt.2017.7142.
Debray TP, Damen JA, Snell KI, Ensor J, Hooft L, Reitsma JB, et al. A guide to systematic review and meta-analysis of prediction model performance. BMJ. 2017;356:i6460.
Collins GS, Reitsma JB, Altman DG, Moons KG. Transparent reporting of a multivariable prediction model for individual prognosis or diagnosis (TRIPOD): the TRIPOD statement. BMJ. 2015;350(jan07 4):g7594. https://doi.org/10.1136/bmj.g7594.
Moons KG, de Groot JA, Bouwmeester W, Vergouwe Y, Mallett S, Altman DG, et al. Critical appraisal and data extraction for systematic reviews of prediction modelling studies: the CHARMS checklist. PLoS Med. 2014;11(10):e1001744. https://doi.org/10.1371/journal.pmed.1001744.
Moons KM, Wolff RF, Riley RD, et al. Probast: a tool to assess risk of bias and applicability of prediction model studies: explanation and elaboration. Ann Intern Med. 2019;170(1):W1–W33. https://doi.org/10.7326/M18-1377.
Steyerberg EW, Moons KG, van der Windt DA, et al. Prognosis Research Strategy (PROGRESS) 3: prognostic model research. PLoS Med. 2013;10(2):e1001381. https://doi.org/10.1371/journal.pmed.1001381.
McGowan J, Sampson M, Salzwedel DM, Cogo E, Foerster V, Lefebvre C. PRESS peer review of electronic search strategies: 2015 guideline statement. J Clin Epidemiol. 2016;75:40–6. https://doi.org/10.1016/j.jclinepi.2016.01.021.
Bramer WM, Giustini D, de Jonge GB, Holland L, Bekhuis T. De-duplication of database search results for systematic reviews in EndNote. J Med Libr Assoc. 2016;104(3):240–3. https://doi.org/10.3163/1536-5050.104.3.014.
Moher D, Liberati A, Tetzlaff J, Altman DG, Group P. Preferred reporting items for systematic reviews and meta-analyses: the PRISMA statement. BMJ. 2009;339(jul21 1):b2535. https://doi.org/10.1136/bmj.b2535.
Iorio A, Spencer FA, Falavigna M, Alba C, Lang E, Burnand B, et al. Use of GRADE for assessment of evidence about prognosis: rating confidence in estimates of event rates in broad categories of patients. BMJ. 2015;350(mar16 7):h870. https://doi.org/10.1136/bmj.h870.
Schünemann H, Brożek J, Guyatt G, Oxman A, Editors. GRADE handbook for grading quality of evidence and strength of recommendations. The GRADE Working Group; 2013. http://training.cochrane.org/resource/grade-handbook. Accessed 20 Jul 2020.
Moher D, Shamseer L, Clarke M, Ghersi D, Liberati A, Petticrew M, et al. Preferred reporting items for systematic review and meta-analysis protocols (PRISMA-P) 2015 statement. Syst Rev. 2015;4(1):1. https://doi.org/10.1186/2046-4053-4-1.
National Institute for Health Research. International Prospective Register of Systematic Reviews (PROSPERO). Available from: https://www.crd.york.ac.uk/prospero. Accessed 2 Aug 2020.
National Library of Medicine, National Center for Biotechnology Information. PubMed. Available from: https://pubmed.ncbi.nlm.nih.gov. Accessed 2 Aug 2020.
Elsevier. Embase. Available from: https://www.embase.com. Accessed 2 Aug 2020.
Cochrane Library. Cochrane Library. Available from: https://www.cochranelibrary.com. Accessed 2 Aug 2020.
Clarivate Analytics. Web of Science. Available from: http://www.isiknowledge.com. Accessed 2 Aug 2020.
Elsevier. Scopus. Available from: https://www.scopus.com. Accessed 2 Aug 2020.
EBSCO Information Services. SPORTDiscus. Available from: http://search.ebscohost.com. Accessed 2 Aug 2020.
EBSCO Information Services. Cumulative Index of Nursing and Allied Health Literature (CINAHL). Available from: http://search.ebscohost.com. Accessed 2 Aug 2020.
Hislop AC, Collins NJ, Tucker K, Deasy M, Semciw AI. Does adding hip exercises to quadriceps exercises result in superior outcomes in pain, function and quality of life for people with knee osteoarthritis? A systematic review and meta-analysis. Br J Sports Med. 2020;54(5):263–71. https://doi.org/10.1136/bjsports-2018-099683.
OpenGrey. Available from: http://www.opengrey.eu. Accessed 15 Apr 2021.
British Library Inside. Available from: http://explore.bl.uk/primo_library/libweb/action/search.do?vid1/4BLVU1. Accessed 15 Apr 2021.
ProQuest Dissertations & Theses Global. Available from: https://search.proquest.com. Accessed 15 Apr 2021.
BIOSIS previews. Available from: http://www.ovid.com. Accessed 15 Apr 2021.
Clarivate Analytics. EndNote version X9.2. 2018. Available from: https://endnote.com.
Tencent. Tencent Document. 2018. Available from: https://docs.qq.com.
Bellou V, Belbasis L, Konstantinidis AK, Tzoulaki I, Evangelou E. Prognostic models for outcome prediction in patients with chronic obstructive pulmonary disease: systematic review and critical appraisal. BMJ. 2019;367:l5358.
Gerry S, Bonnici T, Birks J, et al. Early warning scores for detecting deterioration in adult hospital patients: systematic review and critical appraisal of methodology. BMJ. 2020;369:m1501.
Nagendran M, Chen Y, Lovejoy CA, et al. Artificial intelligence versus clinicians: systematic review of design, reporting standards, and claims of deep learning studies. BMJ. 2020;368:m689.
Lambin P, Leijenaar RTH, Deist TM, Peerlings J, de Jong EEC, van Timmeren J, et al. Radiomics: the bridge between medical imaging and personalized medicine. Nat Rev Clin Oncol. 2017;14(12):749–62. https://doi.org/10.1038/nrclinonc.2017.141.
Mongan J, Moy L, Kahn CE Jr. Checklist for artificial intelligence in medical imaging (CLAIM): a guide for authors and reviewers. Radiol Artif Intell. 2020;2(2):e200029. https://doi.org/10.1148/ryai.2020200029.
Luo W, Phung D, Tran T, Gupta S, Rana S, Karmakar C, et al. Guidelines for developing and reporting machine learning predictive models in biomedical research: a multidisciplinary view. J Med Internet Res. 2016;18(12):e323. https://doi.org/10.2196/jmir.5870.
Cochrane methods prognosis. Tools. Cochrane methods prognosis; 2020. Available via https://methods.cochrane.org/prognosis/tools. Accessed 20 Jul 2020.
Cochrane methods screening and diagnostic tests. Handbook for DTA reviews. Cochrane methods screening and diagnostic tests; 2020. Available via https://methods.cochrane.org/sdt/handbook-dta-reviews. Accessed 20 Jul 2020.
Zhang TS, Zhong WZ, Li B. Applied methodology for evidence-based medicine. 2nd ed. Changsha: Central South University Press; 2014.
Zhang TS, Dong SJ, Zhou ZR. Advanced meta-analysis in Stata. Shanghai: Fudan University Press; 2016.
International Business Machines Corporation. SPSS Statistics version 26.0. 2019. Available from: https://www.ibm.com/products/spss-statistics.
Cohen JA. A coefficient of agreement for nominal scales. Educ Psychol Meas. 1960;20(1):37–46. https://doi.org/10.1177/001316446002000104.
Marasini D, Quatto P, Ripamonti E. Assessing the inter-rater agreement for ordinal data through weighted indexes. Stat Methods Med Res. 2016;25(6):2611–33. https://doi.org/10.1177/0962280214529560.
Koo TK, Li MY. A guideline of selecting and reporting intraclass correlation coefficients for reliability research. J Chiropr Med. 2016;15(2):155–63. https://doi.org/10.1016/j.jcm.2016.02.012.
Fornacon-Wood I, Faivre-Finn C, O'Connor JPB, Price GJ. Radiomics as a personalized medicine tool in lung cancer: separating the hope from the hype. Lung Cancer. 2020;146:197–208. https://doi.org/10.1016/j.lungcan.2020.05.028.
Stata Corporation. Stata/SE version 15.1. 2019. Available from: https://www.stata.com.
Harris R, Bradburn M, Deeks J, et al. METAN: Stata module for fixed and random effects meta-analysis. Statistical Software Components S456798, Boston College Department of Economics, revised 23 Sep 2010. Avaliable from: https://ideas.repec.org/c/boc/bocode/s456798.html. Accessed 2 Aug 2020.
Dwamena B. MIDAS: Stata module for meta-analytical integration of diagnostic test accuracy studies. Statistical Software Components S456880, Boston College Department of Economics, revised 05 Feb 2009. Avaliable from: https://ideas.repec.org/c/boc/bocode/s456880.html. Accessed 2 Aug 2020.
Harbord RM, Whiting P. Metandi: meta-analysis of diagnostic accuracy using hierarchical logistic regression. Stata J. 2009;9(2):211-29.
Macaskill P. Empirical Bayes estimates generated in a hierarchical summary ROC analysis agreed closely with those of a full Bayesian analysis. J Clin Epidemiol. 2004;57(9):925–32. https://doi.org/10.1016/j.jclinepi.2003.12.019.
Huedo-Medina TB, Sánchez-Meca J, Marín-Martínez F, Botella J. Assessing heterogeneity in meta-analysis: Q statistic or I2 index?[J]. Psychol Methods. 2006;11(2):193–206. https://doi.org/10.1037/1082-989X.11.2.193.
Berlin JA, Santanna J, Schmid CH, Szczech LA, Feldman HI. Anti-lymphocyte antibody induction therapy study group. Individual patient versus group-level data meta-regressions for the investigation of treatment effect modifiers: ecological bias rears its ugly head. Stat Med. 2002;21(3):371–87. https://doi.org/10.1002/sim.1023.
Deeks JJ, Macaskill P, Irwig L. The performance of tests of publication bias and other sample size effects in systematic reviews of diagnostic test accuracy was assessed. J Clin Epidemiol. 2005;58(9):882–93. https://doi.org/10.1016/j.jclinepi.2005.01.016.
Page MJ, Altman DG, McKenzie JE, et al. Flaws in the application and interpretation of statistical analyses in systematic reviews of therapeutic interventions were common: a cross-sectional analysis. J Clin Epidemiol. 2018;95:7–18. https://doi.org/10.1016/j.jclinepi.2017.11.022.
Egger M, Davey Smith G, Schneider M, Minder C. Bias in meta-analysis detected by a simple, graphical test. BMJ. 1997;315(7109):629–34. https://doi.org/10.1136/bmj.315.7109.629.
Song F, Khan KS, Dinnes J, Sutton AJ. Asymmetric funnel plots and publication bias in meta-analyses of diagnostic accuracy. Int J Epidemiol. 2002;31(1):88–95. https://doi.org/10.1093/ije/31.1.88.
Duval S, Tweedie R. Trim and fill: A simple funnel-plot-based method of testing and adjusting for publication bias in meta-analysis. Biometrics. 2000;56(2):455–63. https://doi.org/10.1111/j.0006-341X.2000.00455.x.
Acknowledgements
The authors would like to thank the editors and reviewers for their kindness and enlightening comments on this manuscript.
Funding
This work is supported by the National Natural Science Foundation of China (81771790) and the Medicine and Engineering Combination Project of Shanghai Jiao Tong University (YG2019ZDB09). They played no role in the design of the study and collection, analysis, and interpretation of data and in writing the manuscript.
Author information
Authors and Affiliations
Contributions
JZ, LS, and GZ originally conceptualized the study. All the authors contributed to the development of the protocol. JZ drafted the original manuscript. JH brought expertise in prediction model building. GZ brought expertise in clinical managements of osteoarthritis. JZ and LS did the preliminary searches, piloted the study selection process, and developed the data analysis strategy, with assistance from YX, YH, JH, and GZ. GZ polished the English language of the manuscript. WY and HZ supervised the protocol developing process. WY acquired the funding and is the guarantor of this manuscript. All authors critically reviewed and approved the final version of the manuscript.
Corresponding authors
Ethics declarations
Ethics approval and consent to participate
Not applicable
Consent for publication
Not applicable
Competing interests
The authors declare that they have no competing interests.
Additional information
Publisher’s Note
Springer Nature remains neutral with regard to jurisdictional claims in published maps and institutional affiliations.
Supplementary Information
Additional file 1.
PRISMA-P checklist.
Additional file 2.
PROSPERO registration (CRD42020203543).
Additional file 3.
Supplementary Tables.
Additional file 4.
Sample search strategies.
Rights and permissions
Open Access This article is licensed under a Creative Commons Attribution 4.0 International License, which permits use, sharing, adaptation, distribution and reproduction in any medium or format, as long as you give appropriate credit to the original author(s) and the source, provide a link to the Creative Commons licence, and indicate if changes were made. The images or other third party material in this article are included in the article's Creative Commons licence, unless indicated otherwise in a credit line to the material. If material is not included in the article's Creative Commons licence and your intended use is not permitted by statutory regulation or exceeds the permitted use, you will need to obtain permission directly from the copyright holder. To view a copy of this licence, visit http://creativecommons.org/licenses/by/4.0/. The Creative Commons Public Domain Dedication waiver (http://creativecommons.org/publicdomain/zero/1.0/) applies to the data made available in this article, unless otherwise stated in a credit line to the data.
About this article
Cite this article
Zhong, J., Si, L., Zhang, G. et al. Prognostic models for knee osteoarthritis: a protocol for systematic review, critical appraisal, and meta-analysis. Syst Rev 10, 149 (2021). https://doi.org/10.1186/s13643-021-01683-9
Received:
Accepted:
Published:
DOI: https://doi.org/10.1186/s13643-021-01683-9