Abstract
Exposure–response modeling is important to optimize dose and dosing regimens in clinical drug development. While primary clinical trial endpoints often have few categories and thus provide only limited information, sometimes there may be additional, more informative endpoints. Benefits of fully incorporating relevant information in longitudinal exposure–response modeling through joint modeling have recently been shown. This manuscript aims to further investigate the benefit of joint modeling of an ordered categorical primary endpoint with a related near-continuous endpoint, through the sharing of model parameters in the latent variable indirect response (IDR) modeling framework. This is illustrated by analyzing the data collected through up to 116 weeks from a phase 3b response-adaptive trial of ustekinumab in patients with psoriasis. The primary endpoint was based on the 6-point physician’s global assessment (PGA) score. The Psoriasis area and severity Index (PASI) data, ranging from 0 to 72 with 0.1 increments, were also available. Separate and joint latent variable Type I IDR models of PGA and PASI scores were developed and compared. The results showed that the separate PGA model had a substantial structural bias, which was corrected by the joint modeling of PGA and PASI scores.



Similar content being viewed by others
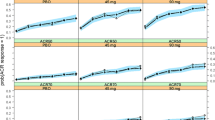
References
Overgaard RV, Ingwersen SH, Tornoe CW (2015) Establishing good practices for exposure-response analysis of clinical endpoints in drug development. CPT 4(10):565–575. https://doi.org/10.1002/psp4.12015
Hu C, Zhou H, Sharma A (2017) Landmark and longitudinal exposure-response analyses in drug development. J Pharmacokinet Pharmacodyn 44(5):503–507. https://doi.org/10.1007/s10928-017-9534-0
Sharma A, Jusko WJ (1996) Characterization of four basic models of indirect pharmacodynamic responses. J Pharmacokinet Biopharm 24(6):611–635
Blauvelt A, Ferris LK, Yamauchi PS, Qureshi A, Leonardi CL, Farahi K, Fakharzadeh S, Hsu MC, Li S, Chevrier M, Smith K, Goyal K, Chen Y, Munoz-Elias EJ, Callis Duffin K (2017) Extension of ustekinumab maintenance dosing interval in moderate-to-severe psoriasis: results of a phase IIIb, randomized, double-blinded, active-controlled, multicentre study (PSTELLAR). Br J Dermatol 177(6):1552–1561. https://doi.org/10.1111/bjd.15722
Hutmacher MM, Krishnaswami S, Kowalski KG (2008) Exposure-response modeling using latent variables for the efficacy of a JAK3 inhibitor administered to rheumatoid arthritis patients. J Pharmacokinet Pharmacodyn 35:139–157
Hu C, Xu Z, Rahman MU, Davis HM, Zhou H (2010) A latent variable approach for modeling categorical endpoints among patients with rheumatoid arthritis treated with golimumab plus methotrexate. J Pharmacokinet Pharmacodyn 37(4):309–321
Hu C, Xu Z, Mendelsohn A, Zhou H (2013) Latent variable indirect response modeling of categorical endpoints representing change from baseline. J Pharmacokinet Pharmacodyn 40(1):81–91
Hu C (2014) Exposure-response modeling of clinical end points using latent variable indirect response models. CPT 3:e117. https://doi.org/10.1038/psp.2014.15
Laffont CM, Vandemeulebroecke M, Concordet D (2014) Multivariate analysis of longitudinal ordinal data with mixed effects models, with application to clinical outcomes in osteoarthritis. J Am Stat Assoc 109(507):955–966
Hu C, Randazzo B, Sharma A, Zhou H (2017) Improvement in latent variable indirect response modeling of multiple categorical clinical endpoints: application to modeling of guselkumab treatment effects in psoriatic patients. J Pharmacokinet Pharmacodyn 44(5):437–448. https://doi.org/10.1007/s10928-017-9531-3
Hu C, Yao Z, Chen Y, Randazzo B, Zhang L, Xu Z, Sharma A, Zhou H (2018) A comprehensive evaluation of exposure-response relationships in clinical trials: application to support guselkumab dose selection for patients with psoriasis. J Pharmacokinet Pharmacodyn 45(4):523–535. https://doi.org/10.1007/s10928-018-9581-1
Hu C, Szapary PO, Mendelsohn AM, Zhou H (2014) Latent variable indirect response joint modeling of a continuous and a categorical clinical endpoint. J Pharmacokinet Pharmacodyn 41(4):335–349. https://doi.org/10.1007/s10928-014-9366-0
Hu C, Zhou H (2016) Improvement in latent variable indirect response joint modeling of a continuous and a categorical clinical endpoint in rheumatoid arthritis. J Pharmacokinet Pharmacodyn 43(1):45–54
Hu C, Xu Y, Zhuang Y, Hsu B, Sharma A, Xu Z, Zhang L, Zhou H (2018) Joint longitudinal model development: application to exposure-response modeling of ACR and DAS scores in rheumatoid arthritis patients treated with sirukumab. J Pharmacokinet Pharmacodyn 45(5):679–691. https://doi.org/10.1007/s10928-018-9598-5
Hu C, Zhou H, Sharma A (2020) Facilitating longitudinal exposure-response modeling of a composite endpoint using the joint modeling of sparsely and frequently collected subcomponents. AAPS J. https://doi.org/10.1208/s12248-020-00441-4
Hu C, Szapary PO, Yeilding N, Zhou H (2011) Informative dropout modeling of longitudinal ordered categorical data and model validation: application to exposure-response modeling of physician’s global assessment score for ustekinumab in patients with psoriasis. J Pharmacokinet Pharmacodyn 38(2):237–260
Feagan BG, Sandborn WJ, Gasink C, Jacobstein D, Lang Y, Friedman JR, Blank MA, Johanns J, Gao LL, Miao Y, Adedokun OJ, Sands BE, Hanauer SB, Vermeire S, Targan S, Ghosh S, de Villiers WJ, Colombel JF, Tulassay Z, Seidler U, Salzberg BA, Desreumaux P, Lee SD, Loftus EV Jr, Dieleman LA, Katz S, Rutgeerts P, Group U-I-US (2016) Ustekinumab as induction and maintenance therapy for Crohn’s disease. The N Engl J Med 375(20):1946–1960. https://doi.org/10.1056/NEJMoa1602773
Sandborn WJ, Gasink C, Gao LL, Blank MA, Johanns J, Guzzo C, Sands BE, Hanauer SB, Targan S, Rutgeerts P, Ghosh S, de Villiers WJ, Panaccione R, Greenberg G, Schreiber S, Lichtiger S, Feagan BG, Group CS (2012) Ustekinumab induction and maintenance therapy in refractory Crohn’s disease. N Engl J Med 367(16):1519–1528. https://doi.org/10.1056/NEJMoa1203572
Zhou H, Hu C, Zhu Y, Lu M, Liao S, Yeilding N, Davis HM (2010) Population-based exposure-efficacy modeling of ustekinumab in patients with moderate to severe plaque psoriasis. J Clin Pharmacol 50(3):257–267. https://doi.org/10.1177/0091270009343695
Hu C, Zhou H, Sharma A (2020) Application of beta-distribution and combined uniform and binomial methods in longitudinal modeling of bounded outcome score data. AAPS J 22(5):95. https://doi.org/10.1208/s12248-020-00478-5
Hu C, Zhou H (2008) An improved approach for confirmatory phase III population pharmacokinetic analysis. J Clin Pharmacol 48(7):812–822. https://doi.org/10.1177/0091270008318670
Hu C, Zhang J, Zhou H (2011) Confirmatory analysis for phase III population pharmacokinetics. Pharm Stat 10(1):14–26. https://doi.org/10.1002/pst.403
Zhu Y, Hu C, Lu M, Liao S, Marini JC, Yohrling J, Yeilding N, Davis HM, Zhou H (2009) Population pharmacokinetic modeling of ustekinumab, a human monoclonal antibody targeting IL-12/23p40, in patients with moderate to severe plaque psoriasis. J Clin Pharmacol 49(2):162–175
Xu Y, Hu C, Chen Y, Miao X, Adedokun OJ, Xu Z, Sharma A, Zhou H (2020) Population pharmacokinetics and exposure-response modeling analyses of ustekinumab in adults with moderately to severely active ulcerative colitis. J Clin Pharmacol. https://doi.org/10.1002/jcph.1582
Beal SL (2001) Ways to fit a PK model with some data below the quantification limit. J Pharmacokinet Pharmacodyn 28(5):481–504
Holford N (2015) Clinical pharmacology = disease progression + drug action. Br J Clin Pharmacol 79(1):18–27. https://doi.org/10.1111/bcp.12170
Ursino M, Gasparini M (2018) A new parsimonious model for ordinal longitudinal data with application to subjective evaluations of a gastrointestinal disease. Stat Methods Med Res 27(5):1376–1393. https://doi.org/10.1177/0962280216661370
Hu C, Zhou H, Sharma A (2020) Applying beta distribution in analyzing bounded outcome score data. AAPS J 22(3):61. https://doi.org/10.1208/s12248-020-00441-4
Zhang L, Beal SL, Sheiner LB (2003) Simultaneous vs sequential analysis for population PK/PD data I: best-case performance. J Pharmacokinet Pharmacodyn 30(6):387–404
Beal SL, Sheiner LB, Boeckmann A, Bauer RJ (2009) NONMEM user’s guides (1989–2009). Icon Development Solutions, Ellicott
Bauer RJ (2019) NONMEM tutorial part II: estimation methods and advanced examples. CPT. https://doi.org/10.1002/psp4.12422
Karlsson MO, Holford NHG (2008) A tutorial on visual predictive checks. www.page-meeting.org/?abstract=1434
Funding
This research was funded by Janssen Research and Development, LLC.
Author information
Authors and Affiliations
Corresponding author
Additional information
Publisher's Note
Springer Nature remains neutral with regard to jurisdictional claims in published maps and institutional affiliations.
Supplementary Information
Below is the link to the electronic supplementary material.
Rights and permissions
About this article
Cite this article
Hu, C., Zhou, H. Improving categorical endpoint longitudinal exposure–response modeling through the joint modeling with a related endpoint. J Pharmacokinet Pharmacodyn 49, 283–291 (2022). https://doi.org/10.1007/s10928-021-09796-3
Received:
Accepted:
Published:
Issue Date:
DOI: https://doi.org/10.1007/s10928-021-09796-3