Abstract
Background
Previous studies have shown inconsistent or even contradictory results for some risk factors associated with HIV infection among drug users, and these may be partially explained by geographical variations.
Methods
Data were collected from 11 methadone clinics in the Liangshan Yi Autonomous Prefecture from 2004 to 2012. A non-spatial logistical regression model and a geographically weighted logistic regression model were fitted to analyze the association between HIV infection and specific factors at the individual level.
Results
This study enrolled 6,458 patients. The prevalence of HIV infection was 25.1 %. The non-spatial model indicated that being divorced was positively associated with HIV infection. The spatial model also showed that being divorced was positively associated with HIV infection, but only for 49.4 % of individuals residing in some northern counties. The non-spatial model suggested that service sector work was negatively associated with HIV infection. However, the spatial model indicated that service work was associated with HIV infection, but only for 23.0 % of patients living in some western counties. The non-spatial model did not show that being married was associated with HIV infection in our study field, but the spatial model indicated that being married was negatively associated with HIV infection for 12.0 % of individuals living in some western counties. For other factors, the non-spatial and spatial models showed similar results.
Conclusion
The spatial model may be useful for improving understanding of geographical heterogeneity in the relationship between HIV infection and individual factors. Spatial heterogeneity may be useful for tailoring intervention strategies for local regions, which can consequently result in a more efficient allocation of limited resources toward the control of HIV transmission.
Similar content being viewed by others
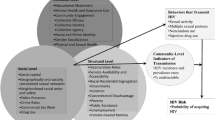
Multilingual abstracts
Please see Additional file 1 for translations of the abstract into the six official working languages of the United Nations.
Background
In recent decades, illicit drug abuse has become a serious social and public health problem worldwide. It directly cost the world 20.0 million disability-adjusted life years (DALYs) and accounted for 0.8 % of all-cause DALYs in 2010 [1]. There are about 2.4 million injecting drug users in China [2].
Human immunodeficiency virus (HIV), the causative pathogen of acquired immune deficiency syndrome (AIDS), is associated with intravenous drug use (IDU) and sharing of syringes. Approximately 34 million people are living with HIV worldwide [3]. In China, there were an estimated 780,000 individuals living with HIV, including 154,000 with AIDS in 2011, with 28.4 % of these infected from IDU [4]. By the end of January 2015, there were 508,864 reported existing live HIV infected patients, including 206,366 with AIDS, with 160,288 patients dying of AIDS in China [5]. The prevalence of HIV infection varies geographically [4, 6–8]. Study results showing associations of HIV infection with demographic, socioeconomic, and drug-related behavioral factors have not always been consistent across regions [7, 9–11], and sometimes were contradictory, which might have resulted from geographical heterogeneity. There are significant global and local spatial autocorrelations for HIV infection [4, 12]. Spatial autocorrelations could cause biases for association estimates and their standard errors if they are not properly accounted for [13, 14]. In the current study, we used a geographically weighted logistic regression (GWLR) model to determine the associations between demographic, socioeconomic, and drug use behavioral characteristics and HIV infection among drug users in the Liangshan Yi Autonomous Prefecture in Southwest China, taking geographical variations into consideration. The Yi people, with a population of approximately eight million, are the seventh largest ethnic group of the 55 ethnic minority groups in China. They live primarily in rural areas (usually in mountainous regions) of Sichuan, Yunnan, Guizhou, and Guangxi provinces. For the Yi ethnic group, rigid rules are stipulated for marriages within the same rank. The current analysis is important for developing more effective HIV prevention programs that are tailored to the needs of diverse groups and consequently increasing access to health services for those who need it most.
Methods
Study area and population
The Liangshan Yi Autonomous Prefecture is home to one of the largest illicit drug production and distribution centers in China. It lies on the border of Sichuan and Yunnan provinces in Southwest China, where HIV/AIDS is prevalent. It has a population of 4.9 million people and the largest population of ethnic Yi in China. This prefecture consists of 618 townships, 16 counties (B-J, M-S counties), and one city (A city) (Fig. 1). Five of the counties have more than 75 % of ethnic Yi, who are mostly located in the northeastern part of the prefecture and have the highest risk of HIV infection [4, 13]. In order to control the dual epidemics of HIV/AIDS and drug use, a methadone clinic was first established in the prefecture in 2004. As of late 2012, there were 10 fixed national methadone clinics and one mobile national methadone clinic in the prefecture. All patients who attended these 11 clinics from 2004 to 2012 were included in the present study and were examined for HIV infection, i.e., all patients were encouraged to have a test for HIV one month after the first visit to the methadone clinics. First, HIV antibody was tested for, then, positive test results were further confirmed using the Western blot analysis. All participants were also asked to take part in a questionnaire survey. The questionnaires were administered face-to-face in a private room by a same-sex interviewer trained in how to administer questionnaires. Information collected included data on demographic and socioeconomic factors, drug use behavioral factors, residency of drug users, etc. The participants’ residency information was taken from their identification cards. Detailed information about the infrastructure and procedures of these clinics, and how they related to the participants has been provided elsewhere [4, 13, 15–17]. Ethical approval for methadone maintenance treatment was provided by the institutional review board of the National Center for AIDS/STD Control and Prevention, China Center for Disease Control. Each participant gave his/her written informed consent.
Data collection
The current study was approved by the Center for Disease Prevention and Control of the Liangshan Yi Autonomous Prefecture. Data were extracted from the national methadone database, and included information on HIV infection, demographic and socioeconomic factors, drug use behavioral factors, and residency of all drug users who were treated at the 11 clinics between 2004 and 2012 [4, 15]. A digitized polygon map of the prefecture at a scale of 1:250,000 was obtained from the Shanghai Digital Bitmap Information Science Co., Ltd; the township is used as the basic geographical unit in this analysis. The latitude and longitude of the center of each township were extracted from this map. The location of each individual was defined as the geographical coordinates (i.e., latitude/longitude) of the center of the township in which he/she lived. All data were entered into Microsoft Excel version 2010 (Microsoft Corp., Redmond, WA, USA).
Analytic strategy
A GWLR model was used to examine the associations between HIV infection and individual factors. The GWLR model is expressed as follows [18]:
Here, y i , x ij , (u i ,v i ), and β ij signify the HIV infection for each individual i, a set of independent variables (j = 1, …, k) for individual i, the x-y coordinates of individual i, and the estimated effect of independent variable j for individual i, respectively.
Current explanatory variables grasped three domains of interest. The first domain was related to drug use behaviors that embody IDU, sharing of syringes, and drug rehabilitation. The second domain was related to demographic characteristics, including age (<30, 30–39, 40–49, and ≥50 years), gender (male, female), ethnicity (Yi, Han, other), and marital status (single, married, divorced, widowed). Three dummy variables were created for age before entering into the models; they were 30–39 (1 = yes, 0 = no), 40–49 (1 = yes, 0 = no), and ≥50 years (1 = yes, 0 = no) respectively, and the <30 years group served as the reference group. Gender was a dummy variable with female used as the reference group. Ethnicity was categorized into Yi, Han (reference group), and others using two dummy variables. Marital status was classified as single (reference), married, divorced, and widowed using three dummy variables. The third domain was related to socioeconomic factors such as occupation and education. Occupation was categorized as farmer, service sector worker, unemployed, and others (reference), with this category including factory worker, government employee, etc. (three dummy variables). Education status was categorized into four sub-categories (three dummy variables): no schooling (reference), elementary school, junior high school, and senior high school or above.
Non-spatial logistical regression analysis was carried out using the SPSS Statistical Package for Social Sciences (SPSS Inc., Chicago, IL, USA, 2007). The GWLR model was used with the iteratively reweighted least squares method in GWR 4.0 software (https://geodacenter.asu.edu/software/downloads/gwr_downloads). The kernel type and function for geographical weighting to estimate local (i.e., in a space rather than the space as a whole) coefficients and their bandwidth size was adaptive bisquare. The bandwidth was the number of nearest neighbors being included in the bisquare kernel. The golden section search method was used to automatically determine the best bandwidth size based on the Akaike information criterion (AICc) (small sample-bias-corrected AIC), with the bandwidth with the lowest AICc used in the analysis. A distance-based weighting scheme was used to assign weights in the GWLR model with the bisquare kernel and bandwidth. Geographical variability for each varying coefficient was tested by model comparison [19]. The coefficient of an explanatory variable in the GWLR model was the change in the log odds of the response given a unit change in that variable. Then, the exponentiation of the coefficient can be used to find the odds ratio (OR) corresponding to a unit change in the variable.
Variables were selected as follows: First, all variables were included in the GWLR model. Then, the variable with the largest p-value was removed from the model until, and only, the variables with some of the local p-values less than 0.1 remained. Based on the geographical coordinates (i.e., latitude/longitude) of each individual, the local mean estimates (OR) and p-values for each individual were visualized on a map to demonstrate the geographical variations in the associations between HIV infection and individual factors using ArcGIS 10.0 software (Environmental Systems Research Institute, Inc., Redlands, CA, US). The GWLR model is relatively insensitive to the choice of kernel function (e.g., bisquare versus Gaussian) [18].
Results
Prevalence of HIV infection
Of the 6,458 drug users, 45.9 % had a history of IDU and 18.1 % shared syringes. The prevalence of HIV infection was 25.1 % (1,622/6,458), and was higher in males (26.4 %) than in females (17.2 %) (χ 2 = 36.2, p < 0.001). The prevalence decreased with increasing age (χ trend 2 = 29.3, p < 0.001) and increasing education level (χ trend 2 = 296.8, p < 0.001). The prevalence was higher in Yi people (28.4 %) than other ethnicities (χ 2 = 184.7, p < 0.001), and in farmers than in non-farmers (see Table 1).
Non-spatial logistical regression
Table 2 shows the factors that were significantly associated with HIV infection. Histories of IDU and syringe sharing were positively associated with HIV infection (p < 0.001). Being divorced or widowed was associated with a greater risk of HIV infection when compared with being single (p < 0.01). It was not so for being married (p > 0.05). Male drug users or ethnic Yi individuals had a greater risk of HIV infection than female drug users (p < 0.01) or ethnic Han individuals (p < 0.001). Service sector work and some school education were negatively associated with HIV infection (p < 0.001) (see Table 2).
Multivariate spatial logistical regression
In the present study, the optimal bandwidth size was 170 (persons). The value of AICc in the global regression was 6236.7 compared with 6218.1 in the local regression, indicating that the fitness of the local regression was better than the global regression. Table 3 shows the factors that were significantly and locally associated with HIV infection. Sharing syringes was found to be positively associated with HIV infection for all individuals, with adjusted OR values ranging from 2.36 to 9.07 (see Table 3 and Fig. 1). It was also found that a history of IDU was positively associated with HIV infection for 99.9 % of drug users. The remaining 0.1 % all resided in E county (see Table 3 and Fig. 1). For 99.9 % of the individuals in this study, drug rehabilitation was not found to be significantly associated with HIV infection (p > 0.05) (see Table 3).
In terms of the demographic factors, being married was found to be negatively associated with HIV infection for 12 % of all individuals living in western counties (C, O, P, and Q counties) and A city (p < 0.05) (see Table 3 and Fig. 2). Being divorced was found to be positively associated with HIV infection for 49.4 % of all patients living in northern counties (p < 0.05) (see Table 3 and Fig. 2). Being widowed was also found to be positively associated with HIV infection for 61.1 % of all patients residing in eastern counties (p < 0.05) (see Table 2 and Fig. 2). Male participants had a significantly higher risk of HIV infection (p < 0.05) (see Table 3 and Fig. 2). Ethnic Yi participants were more likely to be infected with HIV than ethnic Han participants (p < 0.05) (see Table 3 and Fig. 2).
In terms of the socioeconomic factors, service sector work was found to be negatively associated with HIV infection for 23.0 % of all drug users living in western counties compared to other occupations such as factory workers or government workers (p < 0.05) (see Table 3 and Fig. 3). Compared with no schooling, having primary school education or above was negatively associated with HIV infection for most of the population (p < 0.05); OR values decreased with an increasing level of education (see Table 2 and Fig. 3).
Discussion
The GWLR model showed geographical variations in the risk factors associated with HIV infection, something that was not indicated by the non-spatial logistic regression analysis. For example, the non-spatial analysis indicated that being divorced or widowed was associated with HIV infection, as a previous study also showed [20]. However, the GWLR model showed that being divorced was positively associated with HIV infection for only 49.4 % and being widowed was associated with HIV infection for only 61.1 % of all drug users in this study, respectively. Geographical variations might explain, at least in part, the inconsistent results for the associations between demographic factors and HIV infection, as also shown in previous studies [7, 9, 11]. The geographical variations in the association estimates in the current study might be related to different transmission patterns of HIV infection in the study area [4, 13]. For example, heterosexual intercourse was found to be a major route of transmission in some eastern counties (e.g., I, M) and IDU was a dominant route of transmission in some northwestern counties (e.g., A city) [4, 15]. The first case of HIV was found in the eastern M county in 1995, and some older AIDS patients had begun to die in some of the eastern counties in recent years (e.g., I and M). These might be plausible explanations for the association between being widowed and HIV infection in some eastern counties. However, this speculation needs confirmation.
For drug use behaviors, the GWLR and non-spatial logistic regression models showed similar results regarding injection history and sharing of syringes as global factors associated with HIV infection, which is in accordance with results from previous studies [7, 13]. Consistent results were also obtained for ethnicity. A previous ecological study showed that the relative number of ethnic Yi individuals among drug users was closely related to the prevalence of HIV infection in this prefecture [13]. Another study also showed that 82.9 % of the drug users in the prefecture were of Yi ethnicity, and the areas with the highest prevalence of HIV infection were mainly distributed in the I, M, R, and P counties, where the proportion of Yi ethnic individuals was over 50 % [4]. These findings all indicate that the Yi ethnic group is a high-risk population for HIV infection. The Liangshan Yi Autonomous Prefecture has experienced an alarming increase in HIV infection since the first HIV case was found in M county, where 97.1 % were Yi people in 1995. By the end of 2011, the cumulative number of cases with positive HIV infection was over 25,000 [4], with most of these in the M, R, and I counties, which has a vast majority of the Yi population.
Poverty, culture, and tradition might explain why the Yi people have a high risk of HIV infection. Previous studies have shown that poverty is associated with HIV infection, and some dimensions of being poor have been found to increase the risk and vulnerability to HIV [21–24]. Most Yi ethnic individuals reside in the poorest rural and minority areas (e.g., M, I, H, and R counties, which are all beneath the national poverty line). Arranged marriage between individuals of the same social status is common in the Yi community, and marriage within the social stratum may reinforce barriers to advancement and education. There is a custom of marrying the widow of one’s brother to maintain his line. For example, a man may marry his brother’s widow even if his brother, his sister-in-law, or both are known to have been HIV positive. There is also some acceptance of casual sex and condom use is infrequent [25].
Both the GWLR and non-spatial logistic regression models indicated that the odds of HIV infection were higher in male than female drug users, and the association tended to be stronger than previously estimated [7]. A previous township-level ecological study showed that a proportion of male drug users were positively associated with the prevalence of HIV infection in northwestern counties, but the opposite trend was observed in eastern counties [13]. Another study showed that the percentage of existing HIV infections that could be attributed to sexual transmission was 88 % in both I and M counties, 55 % in P county, 67 % in R county, and only 38 % in A city [4]. It is likely that there were different patterns of transmission of HIV infection in this area. Increasing the level of education might be helpful in preventing HIV infection in the Liangshan Autonomous Yi Prefecture, which is in accordance with some existing reports [21, 23, 26, 27].
Our findings may have some important implications: 1) Spatial autocorrelation and heterogeneity might exist for demographic and socioeconomic factors associated with HIV infection, which should not be ignored. 2) The role of geographical variations in factors might provide some guidance for tailoring site-specific intervention strategies to better prevent or control HIV transmission in areas with limited resources. For example, drug users who are widowed and reside in eastern counties, or who are divorced and live in northern counties should be prioritized for intervention. 3) It is important to increase the level of education for people living in the Liangshan Yi Autonomous Prefecture. Most ethnic Yi individuals in the prefecture are either illiterate or have acquired only primary school education.
The present study had several limitations. First, information regarding sexual behavior, which can include important risk factors for HIV transmission, was not available. The effects of sexual risk factors on HIV infection could not be estimated or controlled, however, homosexuality (especially gay men) was rarely found in the study population. Second, not all the drug users in the region were enrolled in the national methadone clinics, and we are not able to confirm that our study population is a representative sample of community-dwelling drug users. However, one study did report that the prevalence of HIV among patients at the methadone clinics was not significantly different from that among other drug users [28]. Third, the study took place over a long time period, and misclassification bias might occur due to the change in diagnosis tests of HIV, however, this misclassification might be a nonspecific misclassification that made ORs to be close to 1. Fourth, the GWLR model might have some limitations including risk of multicollinearity among local estimates [18].
Conclusion
Studying geographical variations in risk factors associated with HIV infection might provide some guidance for tailoring site-specific intervention strategies to better control and prevent HIV transmission in areas with limited resources. Spatial autocorrelation and heterogeneity are important factors to consider in future studies of risk factors for HIV infection.
Abbreviations
- AICc:
-
Akaike information criterion
- AIDS:
-
Acquired immune deficiency syndrome
- DALY:
-
Disability-adjusted life year
- GWLR:
-
Geographically weighted logistic regression
- HIV:
-
Human immunodeficiency virus
- IDU:
-
Intravenous drug use
- OR:
-
Odds ratio
References
Degenhardt L, Whiteford HA, Ferrari AJ, Baxter AJ, Charlson FJ, Hall WD, et al. Global burden of disease attributable to illicit drug use and dependence: findings from the Global Burden of Disease Study 2010. Lancet. 2013;382:1564–74.
Zhou YH, Liu FL, Yao ZH, Duo L, Li H, Sun Y, et al. Comparison of HIV-, HBV-, HCV- and co-infection prevalence between Chinese and Burmese intravenous drug users of the China-Myanmar border region. PLoS One. 2011;6, e16349.
De Cock KM, Jaffe HW, Curran JW. The evolving epidemiology of HIV/AIDS. AIDS. 2012;26:1205–13.
Zhou YB, Liang S, Wang QX, Gong YH, Nie SJ, Nan L, et al. The geographic distribution patterns of HIV-, HCV- and co-infections among drug users in a national methadone maintenance treatment program in Southwest China. BMC Infect Dis. 2014;14:134.
NCAIDS, NCSTD, China CDC. Update on the AIDS/STD epidemic in China and main response in control and prevention in January, 2015. Chin J AIDS STD. 2015;21:175.
Cuadros DF, Awad SF, Abu-Raddad LJ. Mapping HIV clustering: a strategy for identifying populations at high risk of HIV infection in sub-Saharan Africa. Int J Health Geogr. 2013;12:28.
Zhuang X, Wang Y, Chow EP, Liang Y, Wilson DP, Zhang L. Risk factors associated with HIV/HCV infection among entrants in methadone maintenance treatment clinics in China: a systematic review and meta-analysis. Drug Alcohol Depend. 2012;126:286–95.
Kretzschmar M, Zhang W, Mikolajczyk RT, Wang L, Sun X, Kraemer A, et al. Regional differences in HIV prevalence among drug users in China: potential for future spread of HIV? BMC Infect Dis. 2008;8:108.
Des Jarlais DC, Boltaev A, Feelemyer J, Bramson H, Arasteh K, Phillips BW, et al. Gender disparities in HIV infection among persons who inject drugs in Central Asia: a systematic review and meta-analysis. Drug Alcohol Depend. 2013;132:S7–12.
Des Jarlais DC, Feelemyer JP, Modi SN, Arasteh K, Hagan H. Are females who inject drugs at higher risk for HIV infection than males who inject drugs: an international systematic review of high seroprevalence areas. Drug Alcohol Depend. 2012;124:95–107.
Des Jarlais DC, Bramson HA, Wong C, Gostnell K, Cepeda J, Arasteh K, et al. Racial/ethnic disparities in HIV infection among people who inject drugs: an international systematic review and meta-analysis. Addiction. 2012;107:2087–95.
Peng ZH, Cheng YJ, Reilly KH, Wang L, Qin QQ, Ding ZW, et al. Spatial distribution of HIV/AIDS in Yunnan Province, People's Republic of China. Geospat Health. 2011;5:177–82.
Zhou YB, Wang QX, Liang S, Gong YH, Yang MX, Nie SJ, et al. HIV-, HCV-, and Co-Infections and Associated Risk Factors among Drug Users in Southwestern China: A Township-Level Ecological Study Incorporating Spatial Regression. PLoS One. 2014;9, e93157.
Weidmann C, Schneider S, Litaker D, Weck E, Klüter H. A spatial regression analysis of German community characteristics associated with voluntary non-remunerated blood donor rates. Vox Sang. 2012;102:47–54.
Yin W, Hao Y, Sun X, Gong X, Li F, Li J, et al. Scaling up the national methadone maintenance treatment program in China: achievements and challenges. Int J Epidemiol. 2010;39:ii29–37.
Du WJ, Xiang YT, Wang ZM, Chi Y, Zheng Y, Luo XN, et al. Socio-demographic and clinical characteristics of 3129 heroin users in the first methadone maintenance treatment clinic in China. Drug Alcohol Depend. 2008;94:158–64.
Pang L, Hao Y, Mi G, Wang C, Luo W, Rou K, et al. Effectiveness of first eight methadone maintenance treatment clinics in China. AIDS. 2007;21:S103–7.
Yang TC, Matthews SA. Understanding the non-stationary associations between distrust of the health care system, health conditions, and self-rated health in the elderly: a geographically weighted regression approach. Health Place. 2012;18:576–85.
Nakaya T, Fotheringham AS, Brunsdon C, Charlton M. Geographically weighted Poisson regression for disease association mapping. Stat Med. 2005;24:2695–717.
Davis WR, Johnson BD, Randolph D, Liberty HJ. Risks for HIV infection among users and sellers of crack, powder cocaine and heroin in central Harlem: implications for interventions. AIDS Care. 2006;18:158–65.
Li L, Guo Y. The social determinates of HIV/AIDS. Med Philos. 2012;33:29–30,33.
Masanjala W. The poverty-HIV/AIDS nexus in Africa: a livelihood approach. Soc Sci Med. 2007;64:1032–41.
Kalichman SC, Simbayi LC, Kagee A, Toefy Y, Jooste S, Cain D, et al. Associations of poverty, substance use, and HIV transmission risk behaviors in three South African communities. Soc Sci Med. 2006;62:1641–9.
Li XY, Tang LX. The relationship of AIDs and poverty. Chin Rural Surv. 2005;3:63–71 (in Chinese).
Yang Y, Luan RS, Liu P, Wu CL, Zhou Y, Chen W. Casual sex and concurrent sexual partnerships among young people from an Yi community with a high prevalence of HIV in China. Asian J Androl. 2012;14:758–65.
Birdthistle I, Floyd S, Nyagadza A, Mudziwapasi N, Gregson S, Glynn JR. Is education the link between orphanhood and HIV/HSV-2 risk among female adolescents in urban Zimbabwe? Soc Sci Med. 2009;68:1810–8.
Lakhanpal M, Ram R. Educational attainment and HIV/AIDS prevalence: A cross - country study. Econ Educ Rev. 2008;27:14–21.
Zhuang X, Liang Y, Chow EP, Wang Y, Wilson DP, Zhang L. HIV and HCV prevalence among entrants to methadone maintenance treatment clinics in China: a systematic review and meta-analysis. BMC Infect Dis. 2012;12:130.
Author information
Authors and Affiliations
Corresponding authors
Additional information
Competing interests
The authors declare that they have no competing interests.
Authors’ contributions
YBZ, QXW, YHG, SJN, MXY, LN, AHY, QL, and XXS contributed to the collection and management of the data. YBZ, MXY, SL, YC, YY, and QWJ contributed to the data analysis and wrote the paper. All authors contributed to the interpretation of the data and reviewed the paper.
Additional file
Additional file 1:
Multilingual abstracts in the six official working languages of the United Nations. (PDF 312 kb)
Rights and permissions
Open Access This article is distributed under the terms of the Creative Commons Attribution 4.0 International License (http://creativecommons.org/licenses/by/4.0/), which permits unrestricted use, distribution, and reproduction in any medium, provided you give appropriate credit to the original author(s) and the source, provide a link to the Creative Commons license, and indicate if changes were made. The Creative Commons Public Domain Dedication waiver (http://creativecommons.org/publicdomain/zero/1.0/) applies to the data made available in this article, unless otherwise stated.
About this article
Cite this article
Zhou, YB., Wang, QX., Liang, S. et al. Geographical variations in risk factors associated with HIV infection among drug users in a prefecture in Southwest China. Infect Dis Poverty 4, 38 (2015). https://doi.org/10.1186/s40249-015-0073-x
Received:
Accepted:
Published:
DOI: https://doi.org/10.1186/s40249-015-0073-x