Abstract
In this paper we establish the complete moment convergence for sequences of coordinatewise negatively associated random vectors in Hilbert spaces. The result extends the complete moment convergence in (Ko in J. Inequal. Appl. 2016:131, 2016) to Hilbert spaces as well as generalizes the Baum-Katz type theorem in (Huan et al. in Acta Math. Hung. 144(1):132-149, 2014) to the complete moment convergence.
Similar content being viewed by others
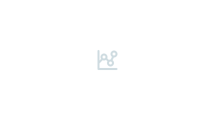
1 Introduction
Ko et al. [3] introduced the concept of negative association (NA) for \(\mathbb{R}^{d}\)-valued random vectors. A finite family of \(\mathbb{R}^{d}\)-valued random vectors \(\{X_{i}, 1\leq i \leq n\}\) is said to be negatively associated (NA) if for every pair of disjoint nonempty subsets A and B of \(\{1,2,\ldots, n\}\) and any real coordinatewise nondecreasing functions f on \(\mathbb{R}^{\vert A\vert d}\), g on \(\mathbb{R}^{\vert B\vert d}\),
whenever the covariance exists. Here and in the sequel, \(\vert A\vert \) denotes the cardinality of A. An infinite family of \(\mathbb{R}^{d}\)-valued random vectors is NA if every finite subfamily is NA.
In the case \(d=1\), the concept of negative association had already been introduced by Alam and Saxena [4] and carefully studied by Joag-Dev and Proschan [5].
A number of well-known multivariate distributions, such as a multinomial distribution, multivariate hypergeometric distribution, negatively correlated normal distribution and joint distribution of ranks, possess the NA property.
Let H be a real separable Hilbert space with the norm \(\Vert \cdot \Vert \) generated by an inner product \(\langle \cdot,\cdot\rangle \) and \(\{e_{j}, j\geq1 \}\) be an orthonormal basis in H. Let X be an H-valued random vector and \(\langle X, e_{j}\rangle \) be denoted by \(X^{(j)}\).
Ko et al. [3] extended the concept of negative association in \(\mathbb{R}^{d}\) to a Hilbert space as follows. A sequence \(\{X_{n}, n \geq1\}\) of H-valued random vectors is said to be NA if for some orthonormal basis \(\{e_{k}, k\geq1\}\) of H and for any \(d\geq1\), the d-dimensional sequence \(\{(X_{n}^{(1)}, X_{n}^{(2)},\ldots, X_{n} ^{(d)}), n\geq1\}\) of \(\mathbb{R}^{d}\)-valued random vectors is NA.
Ko et al. [3] proved almost sure convergence for H-valued NA random vectors and Thanh [6] proved almost sure convergence for H-valued NA random vectors and provided extensions of the results in Ko et al. [3]. Miao [7] showed Hajeck-Renyi inequality for NA random vectors in a Hilbert space.
Huan et al. [2] presented another concept of negative association for H-valued random vectors which is more general than the concept of H-valued NA random vectors introduced by Ko et al. [3] as follows.
A sequence \(\{X_{n}, n\geq1\}\) of H-valued random vectors is said to be coordinatewise negatively associated (CNA) if, for each \(j\geq1\), the sequence \(\{X_{n}^{(j)}, n\geq1\}\) of random variables is NA, where \(X_{n}^{(j)}=\langle X_{n}, e_{j}\rangle \).
Obviously, if a sequence of H-valued random vectors is NA, then it is CNA. However, the reverse is not true in general (see Example 1.4 of Huan et al. [2]).
Recently Huan et al. [2] showed Baum-Katz type theorems for CNA random vectors in Hilbert spaces and Huan [8] obtained the complete convergence for H-valued CNA random vectors with the kth partial sum. Hien and Thanh [9] investigated the weak laws of large numbers for sums of CNA random vectors in Hilbert spaces.
Let \(\{X_{n}, n\geq1\}\) be a sequence of random variables. Let \(\{a_{n}, n\geq1\}\) and \(\{b_{n}, n\geq1\}\) be sequences of positive numbers and \(q>0\). The concept of complete moment convergence is introduced as follows. If \(\sum_{n=1}^{\infty}a_{n} E\{ b_{n}^{-1}\vert X _{n}\vert -\epsilon\}_{+}^{q}<\infty\) for all \(\epsilon>0\), then \(\{X_{n}, n\geq1\}\) is called the complete moment convergence.
Chow [10] first showed the complete moment convergence for a sequence of i.i.d. random variables by generalizing the result of Baum and Katz [11].
Since then, many complete moment convergences for various kinds of random variables in \(\mathbb{R}^{1}\) have been established. For more details, we refer the readers to Liang and Li [12], Guo and Zhu [13], Wang and Hu [14], Wu et al. [15], Shen et al. [16], Wu and Jiang [17], and Ko [1] among others.
Let \(\{X, X_{n}, n\geq1\}\) be a sequence of H-valued random vectors. We consider the following inequalities:
where \(X_{n}^{(j)}=\langle X_{n}, e_{j}\rangle \) and \(X^{(j)}=\langle X, e_{j}\rangle \) for all \(j\geq1\).
If there exists a positive constant \(C_{1}\) (\(C_{2}\)) such that the left-hand side (right-hand side) of (1.1) is satisfied for all \(j\geq1\), \(n\geq1\) and \(t\geq0\), then the sequence \(\{X_{n}, n\geq1 \}\) is said to be coordinatewise weakly lower (upper) bounded by X. The sequence \(\{X_{n}, n\geq1\}\) is said to be coordinatewise weakly bounded by X if it is both coordinatewise weakly lower and upper bounded by X.
In this paper we show the complete moment convergence for CNA random vectors in Hilbert spaces. The result extends the complete moment convergence for NA random variables in \(\mathbb{R}^{1}\) (the main result in Ko [1]) to a Hilbert space as well as generalizes the Baum-Katz type theorem (Theorem 2.1 in Huan et al. [2]) for CNA random vectors in a Hilbert space to the complete moment convergence in a Hilbert space.
2 Preliminaries
The key tool for proving our results is the following maximal inequality.
Lemma 2.1
(Huan et al. [2])
Let \(\{X_{n}, n\geq1\}\) be a sequence of H-valued CNA random vectors with \(EX_{n}=0\) and \(E\Vert X_{n}\Vert ^{2}<\infty\) for all \(n\geq1\). Then we have
Taking \(p=2\) in Lemma 3.1 of Huan et al. [2], we obtain the following lemma.
Lemma 2.2
Let r and α be positive real numbers such that \(1\leq r<2\) and \(\alpha r>1\), and let X be an H-valued random vector with
where \(X^{(j)}=\langle X, e_{j}\rangle \). Then we have
Remark
Let X be an H-valued random vector, where H is finite dimensional. If \(E\Vert X\Vert ^{r}<\infty\), then
holds.
Lemma 2.3
(Kuczmaszewska [18])
Let \(\{X_{n}, n\geq1\}\) be a sequence of random variables weakly upper bounded by a random variable X. Let \(r>0\) and, for some \(A>0\),
and
Then, for some constant \(C>0\),
-
(i)
if \(E\vert X\vert ^{r}<\infty\), then \((n^{-1})\sum_{i=1}^{n} E\vert X_{i}\vert ^{r} \leq CE\vert X\vert ^{r}\),
-
(ii)
\((n^{-1})\sum_{i=1}^{n} E\vert X_{i}^{\prime} \vert ^{r}\leq C(E\vert X^{\prime} \vert ^{r}+A ^{r} P(\vert X\vert >A))\) for all \(A>0\),
-
(iii)
\((n^{-1})\sum_{i=1}^{n} E\vert X_{i}^{\prime\prime} \vert ^{r}\leq CE\vert X^{\prime\prime} \vert ^{r}\) for all \(A>0\),
-
(iv)
\((n^{-1})\sum_{i=1}^{n} E\vert \tilde{X_{i}}\vert ^{r}\leq CE\vert \tilde{X}\vert ^{r}\) for all \(A>0\).
The following result corresponds to Lemma 2.3 of Ko [1].
Lemma 2.4
(Huan et al. [2])
Let r and α be positive real numbers such that \(1\leq r<2\) and \(\alpha r>1\), and let \(\{X_{n}\}\) be a sequence of H-valued CNA random vectors with zero means. If \(\{X_{n}, n\geq1\}\) is coordinatewise weakly upper bounded by a random vector X satisfying (2.2), then
The following lemma corresponds to Lemma 2.4 of Ko [1].
Lemma 2.5
Let r and α be positive real numbers such that \(1\leq r<2\) and \(\alpha r>1\), and let \(\{X_{n}, n\geq1\}\) be a sequence of H-valued CNA random vectors with zero means. If \(\{X_{n}, n\geq1\}\) is coordinatewise weakly upper bounded by a random vector X, then (2.2) implies
Proof
For all \(u>0\) and \(j\geq1\), set
According to the proof of Theorem 2.1 in Huan et al. [2], we have
For \(I_{1}\), by the Markov inequality, (1.1), (2.2) and the fact that \(E\vert Y\vert ^{p}=p\int_{0}^{\infty}y^{p-1}P(\vert Y\vert >y)\,dy\), we obtain
For \(I_{2}\), we estimate that
Since \(\{Y_{ni}^{(j)}, i\geq1\}\) is NA for all \(j\geq1\), and so \(\{Y_{ni}, i\geq1\}\) is CNA. Hence, by the Markov inequality, Lemma 2.1 and Lemma 2.3(ii), we have
The last inequality above is obtained by Lemma 2.3(ii).
For \(I_{211}\), by (2.7) we have that \(I_{211}< \infty\).
For \(I_{212}\), by a standard calculation we observe that
It remains to prove \(I_{22}<\infty\). From (1.1), (2.2), (2.6) and the fact that \(EX_{i}^{(j)}=0\), for all \(i\geq1\) and \(j\geq1\), we obtain
By (2.7) we have that \(I_{221}<\infty\).
For \(I_{222}\), by a standard calculation as in (2.9), we obtain
which yields \(I_{22}<\infty\), together with \(I_{221}<\infty\). Hence, the proof is completed. □
The following lemma shows that Lemmas 2.4 and 2.5 still hold under a sequence of identically distributed H-valued CNA random vectors with zero means.
Lemma 2.6
Let r and α be positive real numbers such that \(1\leq r<2\) and \(\alpha r > 1\), and let \(\{X_{n}, n\geq1\}\) be a sequence of H-valued CNA random vectors with zero means. If \(\{X_{n}, n\geq1\}\) are identically distributed random vectors with
where \(X_{1}^{(j)}=\langle X_{1}, e_{j}\rangle \), then (2.4) and (2.5) hold.
Proof
The proofs are similar to those of Lemma 2.4 and Lemma 2.5, respectively. □
Lemma 2.7
(Huan et al. [2])
Let r and α be positive real numbers such that \(\alpha r\geq1\), and let \(\{X_{n}, n \geq1\}\) be a sequence of H-valued CNA random vectors with zero means. Suppose that \(\{X_{n}, n\geq1\}\) is coordinatewise weakly bounded by a random vector X with
If
then (2.2) holds.
Proof
See the proof of Theorem 2.6 in Huan et al. [2]. □
The following section will show that the complete moment convergence for NA random variables in Ko [1] can be extended to a Hilbert space.
3 Main results
The proofs of main results can be obtained by using the methods of the proofs as in the main results of Ko [1].
Theorem 3.1
Let r and α be positive numbers such that \(1\leq r<2\) and \(\alpha r>1\). Let \(\{X_{n}, n\geq1\}\) be a sequence of H-valued CNA random vectors with zero means. If \(\{X_{n}, n\geq1\}\) is coordinatewise weakly upper bounded by a random vector X satisfying (2.2), then we obtain
where \(a^{+}=\max\{a,0\}\).
Proof
The proof can be obtained by a similar calculation in the proof of Theorem 3.1 of Ko [1]. From Lemmas 2.4 and 2.5 we obtain
□
Theorem 3.2
Let r and α be positive numbers such that \(1\leq r<2\), \(\alpha r> 1\) and \(\alpha>\frac{1}{2}\). Let \(\{X_{n}, n\geq1\}\) be a sequence of H-valued CNA random vectors with zero means. If \(\{X_{n}, n\geq1\}\) is coordinatewise weakly upper bounded by a random vector X, then (3.1) implies (2.4).
Proof
It follows from (3.2) that
Hence, (3.3) and (3.1) imply (2.4). The proof Theorem 3.2 is complete. □
Theorem 3.3
Let r and α be positive numbers such that \(1\leq r<2\), \(\alpha r> 1\) and \(\alpha>\frac{1}{2}\). Let \(\{X_{n}, n\geq1\}\) be a sequence of H-valued CNA random vectors with zero means. If \(\{X_{n}, n\geq1\}\) is coordinatewise weakly upper bounded by a random vector X, then (2.2) implies
Proof
(3.1) provides that
where \(\epsilon^{\prime}=\epsilon2^{-\alpha}\). Hence the proof (3.4) is completed. □
Corollary 3.4
Let r and α be positive real numbers such that \(1\leq r<2\), \(\alpha r> 1\) and \(\alpha>\frac{1}{2}\). Let \(\{X_{n}, n\geq1\}\) be a sequence of H-valued CNA random vectors with zero means. If \(\{X_{n}, n\geq1\}\) is coordinatewise weakly upper bounded by a random vector X, then (2.2) implies
Proof
Inspired by the proof of Theorem 12.1 of Gut [19], we can prove it and omit the proof. □
The following theorem shows that complete convergence and complete moment convergence still hold under a sequence of identically distributed H-valued CNA random vectors with zero means.
Theorem 3.5
Let r and α be positive real numbers such that \(1\leq r<2\) and \(\alpha r> 1\). Let \(\{X_{n}, n\geq1\}\) be a sequence of H-valued CNA random vectors with zero means. Assume that \(\{X_{n}, n\geq1\}\) are identically distributed random vectors with (2.2′) in Lemma 2.6. Then (3.1), (3.4) and (3.5) hold.
Proof
The proofs are similar to those of Theorem 3.1, Theorem 3.3 and Corollary 3.4, respectively. □
Theorem 3.6
Let r and α be positive real numbers such that \(\alpha r \geq1\) and \(\{X_{n}, n\geq1\}\) be a sequence of H-valued CNA random vectors with zero means. If \(\{X_{n}, n\geq1\}\) is coordinatewise weakly bounded by a random vector X satisfying (2.11) and (2.12), then (3.1) holds.
Proof
4 Conclusions
-
1.
In Section 3 we have obtained the complete moment convergence for a sequence of mean zero H-valued CNA random vectors which is coordinatewise weakly upper bonded by a random variable and the related results.
-
2.
Theorem 3.1 generalizes the complete convergence for a sequence of mean zero H-valued CNA random vectors in Huan et al. [2] to the complete moment convergence.
References
Ko, MH: On complete moment convergence for nonstationary negatively associated random variables. J. Inequal. Appl. 2016, 131 (2016)
Huan, NV, Quang, NV, Thuan, NT: Baum-Katz type theorems for coordinatewise negatively associated random vectors in Hilbert spaces. Acta Math. Hung. 144(1), 132-149 (2014)
Ko, MH, Kim, TS, Han, KH: A note on the almost sure convergence for dependent random variables in a Hilbert space. J. Theor. Probab. 22, 506-513 (2009)
Alam, K, Saxena, KML: Positive dependence in multivariate distributions. Commun. Stat., Theory Methods 10, 1183-1196 (1981)
Joag-Dev, K, Proschan, F: Negative association of random variables with applications. Ann. Stat. 11, 286-295 (1983)
Thanh, LV: On the almost sure convergence for dependent random vectors in Hilbert spaces. Acta Math. Hung. 139(3), 276-285 (2013)
Miao, Y: Hajeck-Renyi inequality for dependent random variables in Hilbert space and applications. Rev. Unión Mat. Argent. 53(1), 101-112 (2012)
Huan, NV: On complete convergence for sequences of random vectors in Hilbert spaces. Acta Math. Hung. 147(1), 205-219 (2015)
Hien, NTT, Thanh, LV: On the weak laws of large numbers for sums of negatively associated random vectors in Hilbert spaces. Stat. Probab. Lett. 107, 236-245 (2015)
Chow, YS: On the rate of moment complete convergence of sample sums and extremes. Bull. Inst. Math. Acad. Sin. 16, 177-201 (1988)
Baum, LE, Katz, M: Convergence rates in the law of large numbers. Trans. Am. Math. Soc. 120(1), 108-123 (1965)
Liang, HY, Li, DL: Complete moment and integral convergence for sums of negatively associated random variables. Acta Math. Sin. Engl. Ser. 26(3), 419-432 (2010)
Guo, ML, Zhu, DJ: Equivalent conditions of complete moment convergence of weighted sums for \(\rho^{*}\)-mixing sequence of random variables. Stat. Probab. Lett. 83, 13-20 (2013)
Wang, XJ, Hu, SH: Complete convergence and complete moment convergence for martingale difference sequence. Acta Math. Sin. Engl. Ser. 30, 119-132 (2014)
Wu, YF, Cabrea, MO, Volodin, A: Complete convergence and complete moment convergence for arrays of rowwise END random variables. Glas. Mat. 49(69), 449-468 (2014)
Shen, AT, Xue, MX, Volodin, A: Complete moment convergence for arrays of rowwise NSD random variables. Stochastics 88(4), 606-621 (2016)
Wu, Q, Jiang, Y: Complete convergence and complete moment convergence for negatively associated sequences of random variables. J. Inequal. Appl. 2016, 57 (2016)
Kuczmaszewska, A: On complete convergence in Marcinkiewicz-Zygmund type SLLN for negatively associated random variables. Acta Math. Hung. 128(1), 116-130 (2010)
Gut, A: Probability: A Graduate Course. Springer, New York (2005)
Acknowledgements
This paper was supported by Wonkwang University in 2017.
Author information
Authors and Affiliations
Contributions
All authors read and approved the final manuscript.
Corresponding author
Ethics declarations
Competing interests
The author declares that there is no conflict of interest regarding the publication of this article.
Additional information
Publisher’s Note
Springer Nature remains neutral with regard to jurisdictional claims in published maps and institutional affiliations.
Rights and permissions
Open Access This article is distributed under the terms of the Creative Commons Attribution 4.0 International License (http://creativecommons.org/licenses/by/4.0/), which permits unrestricted use, distribution, and reproduction in any medium, provided you give appropriate credit to the original author(s) and the source, provide a link to the Creative Commons license, and indicate if changes were made.
About this article
Cite this article
Ko, MH. The complete moment convergence for CNA random vectors in Hilbert spaces. J Inequal Appl 2017, 290 (2017). https://doi.org/10.1186/s13660-017-1566-x
Received:
Accepted:
Published:
DOI: https://doi.org/10.1186/s13660-017-1566-x