Abstract
Background
Palliative Performance Scale (PPS) has been frequently used to estimate the survival time of palliative care patients. The objective was to determine the associations between the PPS and survival time among cancer and non-cancer patients in Thailand.
Methods
This is a retrospective cohort study. All in-patient adults who received a palliative care consultation at Chiang Mai University Hospital between 1 July 2018 to 31 July 2019 were included in the study and were followed-up until 26 June 2020. The Palliative Performance Scale was assessed using the validated Thai-Palliative Performance Scale for Adults. Survival analysis was used to determine the association between the Palliative Performance Scale and survival time among cancer and non-cancer patients.
Results
Out of 407 patients, 220 were male (54.1%). There were 307 cancer patients (75.4%) and 100 non-cancer patients (24.6%). The PPS and survival time in cancer patients were significantly correlated. Cancer patients with PPS 10, 20, 30, 40–60, and 70–80% had a median survival time of 2, 6, 13, 39, and 95 days, respectively. Non-cancer patients with PPS 10, 20, and 30% had a median survival time of 8, 6, and 24 days, respectively.
Conclusions
While useful for estimating survival time for cancer patients, other factors should be taken into account in estimating the survival time for non-cancer patients.
Similar content being viewed by others
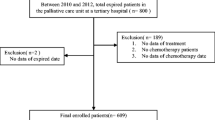
Introduction
The World Health Organization (WHO) estimates that more than 56.8 million people require palliative care globally every year, with 25.7 million people being near their last days of life. The number of people in need of palliative care is increasing, particularly in low and middle-income countries, where access to palliative care is scarce [1]. When caring for palliative care patients, the diseases that patients face are advanced, making prognostication crucial for coordinating a treatment plan between patients, families, and medical teams [2, 3].
However, prognostication in palliative care is extremely challenging. The stage of cancer and progression of the disease do correlate with survival time in general, although they are not the only factors influencing survival [4]. Different types of diseases manifest in different disease trajectories at the final stages. For advanced cancer patients, patients may function relatively well for a long time and then rapidly decline until death. For non-cancer patients, the trajectories may vary. Patients with congestive heart failure or end-stage renal disease usually exhibit a gradual functional decline with frequent acute exacerbations, where every episode can potentially result in death. By contrast, demented or frail elderly patients usually exhibit a slow steady functional decline over a long period of time [5, 6]. Each distinct trajectory results in different utilization of palliative care and other medical care at the end of life [7]. It is necessary for doctors to accurately estimate the survival time promptly for both cancer and non-cancer patients to ensure that the appropriate treatment planning could be made for each individual, harm and discomfort can be avoided, and patients’ autonomy can be enhanced [2,3,4]. In Thai and other similar Asian cultures, where diagnoses of serious illnesses are sometimes withheld from the patients by their doctors and caregivers, the estimation of short survival time also serves as a factor affecting whether the patients are allowed to know about their diagnoses [8].
Many prognostic tools to predict survival in palliative care exist [9, 10]. The PPS, one of the most studied prognostic tools in palliative care. is commonly used in Thailand [11]. The PPS was developed from the Karnofsky Performance Scale to measure physical functional performance in palliative care patients. It assesses five functional parameters, namely degree of ambulation, ability to do activities and extent of disease, ability to do self-care, food and fluid intake, and state of consciousness. PPS are in 10% decrements from 100% (fully ambulatory and healthy) to 0% (death). It is simple and practical for palliative care patients [12].
Most studies have examined the performance of the PPS for cancer patients with far fewer studies examining the performance of PPS for non-cancer patients [13]. A couple of studies have shown that PPS is associated with survival time in both cancer and non-cancer patients at the end of life, [13, 14] however, it may be less predictable in non-cancer patients [15]. Hence it has been recommended that the correlation between the PPS and survival time should be based on a local cohort where feasible [16].
This study aimed to examine the usefulness of PPS in estimating the survival time of patients with advanced cancer and non-cancer diagnoses in Chiang Mai, Thailand.
Methods
Participants and setting
Using a retrospective cohort design, we included all adult inpatients 18 years old or over receiving a consultation by the Palliative Care Unit at Chiang Mai University Hospital between 1 July 2018 and 31 July 2019. If a patient had more than one visit, we included information only from the first visit and excluded information for any readmission visits. Chiang Mai University Hospital is the largest university hospital in Northern Thailand and is a referral center for patients in Chiang Mai and 16 other provinces in the northern region of Thailand [17]. The institution’s consultation criteria include; negotiating an advance care plan, assisting in end-of-life care, managing socioeconomic or psychological problems, skill training for a caregiver to provide home care, and lending medical equipment for home care.
Data collection
As part of the routine clinical assessment at the time of the first consultation, the Thai PPS was assessed by medical residents or palliative care nurses. The Thai PPS, translated from the Palliative Performance Scale version 2 (PPSv2), has been validated and is one of the most commonly used tools for palliative care assessment in Thailand. The tool shows good inter- and intra-rater reliability. The intraclass correlation coefficient (ICC) for absolute agreement is 0.911 (95% CI 0.86–0.96) and for consistency is 0.92 (95% CI 0.87–0.96). Cohen’s kappa score is 0.55 [11].
As part of routine care, patients who have been discharged after palliative care consultations should be followed up weekly (if the initial PPS ≤ 30%) or monthly (if the initial PPS > 30%). The assessment was carried out via telephone to assess patient symptoms and to give a consultation when necessary. Based on this routine follow-up, data on mortality for this study was collected up to 26 June 2020. All patients who had not died during the follow-up period were contacted on 26 June 2020.
Additional demographic information such as age, diagnoses, and co-morbidities was extracted from the hospital’s electronic medical record database. Principal diagnosis from the patient’s discharge summary was considered as the primary diagnosis while diagnoses other than the principal diagnosis were classified as co-morbidities. Uncommon co-morbidities were not recorded. Data was entered using Research Electronic Data Capture (REDCap) (https://redcap.med.cmu.ac.th), which allows data checks to minimize transcription errors (or where necessary collected on paper and later double-entered into the electronic form) and post-entry checks for extreme values. All extreme values were double-checked with the source document in the electronic medical database.
Data analysis
Demographic characteristics and clinical data were shown as numbers and percentages for categorical variables and as mean and standard deviation for continuous variables. Baseline comparison between cancer and non-cancer patients was done using chi-square for categorical variables and t-test for continuous variables. Mann–Whitney U test was used for comparing the median number of co-morbidities between groups. The PPS at first consultation was categorized into five groups: 10%, 20%, 30%, 40–60%, and 70–80%. Survival time among cancer and non-cancer patients was demonstrated using a median, percentile, and Kaplan–Meier survival curves for all the PPS groups and between paired groups. A log-rank test was used to examine the association between the PPS and survival among cancer and non-cancer patients for all the PPS groups and between paired groups. A p-value of < 0.05 was considered statistically significant. All statistical analyses were performed using STATA version 15.
Results
Patient characteristics
Table 1 shows patient characteristics at the beginning of the research. A total of 407 patients were included in the study, 307 were cancer patients (75.4%) and 100 were non-cancer patients (24.6%). Both groups had similar proportions of males and females (45.0% of cancer patients and 49.0% of non-cancer patients, were men). The mean age of cancer patients was significantly lower than non-cancer patients, 60.6 (SD 14.9) and 70.4 (SD 18.3) years old, respectively. Almost all patients had health coverage (96.4% of cancer patients and 94% of non-cancer patients).
The three most common cancers in patients consulted by the palliative care unit were gastrointestinal, lung, and genitourinary cancers. As for non-cancer patients, the three most prevalent diagnoses were neurological disorders (including 26 strokes and 12 other neurological disorders, heart disease, and renal failure. Other diagnoses consisted of a wide range of diseases such as pneumonia, COPD (chronic obstructive pulmonary disease), HIV/AIDs (Human Immunodeficiency Virus/ Acquired immunodeficiency syndrome), and intertrochanteric fracture, none had a prevalence of over 6%. The most common co-morbidity in both cancer and non-cancer patients was hypertension. Non-cancer patients had significantly more comorbidities than cancer patients (Table 1). The top three reasons for a palliative care consultation in both groups were to negotiate an advance care plan (87.6% of cancer patients and 66% of non-cancer patients), to assist in end-of-life care (36.5% of cancer patients and 49% of non-cancer patients) and to manage socioeconomic or psychological problems (20.2% of cancer patients and 17% of non-cancer patients). Most patients had a PPS of \(\le\) 30% (58.3% for cancer patients and 89% for non-cancer patients). The distribution of PPS groups between cancer and non-cancer patients differed significantly (p-value< 0.001) with a higher PPS found among cancer patients than non-cancer patients, as only cancer patients received palliative care consultation at PPS 70–80%. The proportion of patients with PPS 40–60% were 39.1% in cancer patients and 11% in non-cancer patients (Table 2).
PPS and survival time
Kaplan–Meier survival curves and log-rank tests comparing survival time between both groups of patients showed that non-cancer patients had a significantly longer survival time than cancer patients (p-value 0.03). Cox-regression showed hazard ratio of 0.76 with 95% confidence interval of 0.59 to 0.97, p-value 0.03 (Fig. 1). The PPS was associated with survival time among cancer (p-value< 0.001) and non-cancer patients (p-value< 0.001). (Figs. 2 and 3) Log-rank test between PPS 10–30% and 40–60% showed p-value< 0.001 in cancer patients (Fig. 2).
By contrast, the median survival time based on PPS differed between cancer and non-cancer patients. For cancer patients, there was an association between PPS and median survival time. The median survival times [percentile 25, percentile 75] for those with PPS of 10, 20, 30 were 2 days [1, 4], 6 days [3, 19] and 13 days [7, 31], respectively. In addition, those with PPS of 40–60 and 70–80 were 39 days [12, 106] and 95 days [35], respectively (Table 3). Most pairwise comparisons in cancer patients between PPS 10 vs. 20% (p-value < 0.001), PPS 30 vs. 40–60% (p-value < 0.01) and PPS 40–60 vs. 70–80% (p-value 0.05) showed significant differences except for PPS 20 vs. 30% (p-value 0.29).
For non-cancer patients, the logical association between PPS and median survival time was not observed. The median survival time [percentile 25, percentile 75] for those with PPS of 10, 20, and 30% were 8 days [3, 105], 6 days [3, 14], and 24 days [8, 176] respectively. The sample size for non-cancer patients with PPS 40–60% was small (n = 11) and the median survival time was not reached (Table 3). Similarly, pairwise comparisons in non-cancer patients between PPS 20 vs. 30%; and PPS 30 vs. 40–60% showed significant differences (p-value 0.02 and 0.004 respectively) but did not show significant differences between PPS 10 vs. 20% (p-value 0.38). Significant difference between survival time in non-cancer patients with PPS 10–30% and 40–60% were seen (p-value < 0.001).
Discussion
This study suggests that a low PPS (\(\le\) 30%) can serve as a predictor of the last months of life in both cancer and non-cancer patients. The study helps confirm that the PPS is a reliable prognosticator in estimating survival time for cancer patients but due to unexpected findings of PPS 10 and 20% in non-cancer patients, further studies are required to conclude the reliability of PPS in predicting survival time in non-cancer patients.
The median survival times for cancer patients with a PPS of 10, 20, 30, 40–60, and 70–80% were 2, 6, 13, 39, and 95 days, respectively. This is in line with previous studies for cancer patients where death was expected within: days if the PPS were 10%; a week if the PPS was 20%, weeks if the PPS was 30% and within months if PPS was over 40% [13]. However, there are still slight variations potentially due to some differences in standards of care in different settings. A study in a home-based hospice and palliative care program showed a median survival of 3, 5, 10, 19, 29.5, 35 days for PPS 10 to 60%, respectively [18]. One study in patients admitted to a palliative care unit in South Korea showed initial PPS after palliative care consultation of 10–20%, 30–50%, and more than 60% to have a median survival time of 14, 19, and 38 days [19]. Another study, in a Southeast Asian population who were admitted to a palliative care unit in a tertiary hospital found the mean survival time to be 19 and 43 days in patients at PPS of 10–30% and 40–60% [20].
In non-cancer patients, patients with a PPS of 10–30% had a significantly lower survival time compared to a PPS of 40–60%. The association for survival was not logical among the non-cancer patients with very low-performance status (PPS 10–20%). Many of the non-cancer patients with PPS 40–60% and even some of the patients with PPS ≤ 30% may have prolonged survival, despite the need for palliative care consultation. This cohort also had a wider variation in survival time than cancer patients. A large proportion of non-cancer patients in our study had a diagnosis of cerebrovascular disease (stroke), common in South-East Asian countries [21]. Stroke may lead to low-performance status but may still be associated with a relatively long survival. Thus, low PPS in stroke patients is not necessarily associated with short survival time [22]. Another explanation why the theoretical gradient relationship between lower PPS and shorter survival time was not demonstrated is likely due to the other forms of disease trajectories [22, 23]. For example, a PPS at a consultation for patients with severe end-organ failures presenting with an acute exacerbation may not fully represent actual physical functional performance. This results in an initially low PPS but a longer than expected survival time.
Limitations
There are at least three limitations to this research. Firstly, the number of non-cancer patients was relatively small, we were not able to do a subgroup analysis of specific diseases in non-cancer patients. However, we believe that reporting a combined median survival time in non-cancer diagnoses still yields meaningful estimations for generalist palliative care providers. Secondly, data collection was done from one study site, Chiang Mai University Hospital. The patient consulted by the palliative care unit in a tertiary hospital, may have more complex diseases and/or more complex palliative care needs when compared to other settings. However, we believe that our results are still generalizable in the cancer population in Thailand, as the most common cancer types in this study resemble cancer prevalence in the country [24]. Lastly, palliative care consultations in our population occurred late in the course of disease partly due to the institution’s consultation criteria of palliative care being for end of life care only. Patients were predominantly at PPS of 10–50%, whereas PPS of 60% or more were relatively few. Thus, it was not possible to get reliable estimates for the survival time among patients with higher PPS, especially for non-cancer patients.
Conclusion
The PPS is associated with survival time among cancer and non-cancer patients in Thailand. While PPS was a reliable predictor of survival time in advanced cancer patients, in non-cancer patients with PPS 10–30%, other considerations were needed for the prediction of survival time such as disease diagnoses. Further study should have an emphasis on prognostication in non-cancer patients to develop a more reliable tool.
Availability of data and materials
The datasets generated during and analyzed during the current study are not publicly available as they were not part of the consent but are available from the corresponding author on reasonable request.
Abbreviations
- PPS:
-
Palliative Performance Scale
References
Worldwide Hospice Palliative Care Alliance (WHPCA). Global Atlas of Palliative Care. 2020. www.thewhpca.org. Accessed 15 Oct 2020.
Chan E-Y, Wu H-Y, Chan Y-H. Revisiting the Palliative Performance Scale: change in scores during disease trajectory predicts survival. Palliat Med. 2013;27:367–74.
Jansen WJJ, Buma S, Gootjes JRG, et al. The palliative performance scale applied in high-care residential hospice: a retrospective study. Palliat Med. 2015;18:67–70.
Maltoni M, Caraceni A, Brunelli C, et al. Prognostic factors in advanced cancer patients: evidence-based clinical recommendations--a study by the Steering Committee of the European Association for Palliative Care. J Clin Oncol 2005; 23: 6240–6248. 2005/09/02.
Boyd K and Murray SA. Recognising and managing key transitions in end of life care. BMJ 2010; 341.
Murray SA, Kendall M, Boyd K, et al. Illness trajectories and palliative care. BMJ (Clinical Research Ed). 2005;330:1007–11.
Quinn KL, Stukel T, Stall NM, et al. Association between palliative care and healthcare outcomes among adults with terminal non-cancer illness: population based matched cohort study. BMJ. 2020;370:m2257.
Pinyopornpanish K, Angkurawaranon C, Gomutbutra P, et al. Factors affecting family members’ decisions to reveal cancer diagnoses to patients: A qualitative study. J Med Assoc Thai. 2017;100:808–14.
Simmons CPL, McMillan DC, McWilliams K, et al. Prognostic tools in patients with advanced cancer: a systematic review. J Pain Symptom Manage. 2017;53:962–70.
Jang RW, Caraiscos VB, Swami N, et al. Simple prognostic model for patients with advanced cancer based on performance status. J Oncol Pract 2014; 10: e335–341. 2014/08/15. con
Chewaskulyong B, Sapinun L, Downing GM, et al. Reliability and validity of the Thai translation (Thai PPS Adult Suandok) of the Palliative Performance Scale (PPSv2). Palliat Med 2012; 26: 1034–1041. 2011/10/14.
Anderson F, Downing GM, Hill J, et al. Palliative performance scale (PPS): a new tool. J Palliat Care 1996; 12: 5–11. 1996/01/01.
Baik D, Russell D, Jordan L, et al. Using the palliative performance scale to estimate survival for patients at the end of life: a systematic review of the literature. Palliat Med. 2018;21:1651–61.
Lau F, Downing M, Lesperance M, et al. Using the Palliative Performance Scale to provide meaningful survival estimates. J Pain Symptom Manage. 2009;38:134–44.
Head B, Ritchie CS, Smoot TM. Prognostication in Hospice Care: Can the Palliative Performance Scale Help? Palliat Med. 2005;8:492–502.
Lau F, Maida V, Downing M, et al. Use of the Palliative Performance Scale (PPS) for end-of-life prognostication in a palliative medicine consultation service. J Pain Symptom Manage. 2009;37:965–72.
Faculty of Medicine Chiang Mai University. Information of Maharaj Nakorn Chiang Mai Hospital, 2020. https://www.med.cmu.ac.th/en/service/suandok/. (Accessed date 13 Oct 2020).
Weng LC, Huang HL, Wilkie DJ, et al. Predicting survival with the Palliative Performance Scale in a minority-serving hospice and palliative care program. J Pain Symptom Manage 2009; 37: 642–648. 2008/10/01.
Yoon SJ, Choi SE, LeBlanc TW, et al. Palliative Performance Scale Score at 1 Week After Palliative Care Unit Admission is More Useful for Survival Prediction in Patients With Advanced Cancer in South Korea. Am J Hosp Palliat Care 2018; 35: 1168–1173. 2018/04/18.
Mei AH, Jin WL, Hwang MK, et al. Value of the Palliative Performance Scale in the prognostication of advanced cancer patients in a tertiary care setting. Palliat Med 2013; 16: 887–893. 2013/06/14.
Venketasubramanian N, Yoon BW, Pandian J, et al. Stroke Epidemiology in South, East, and South-East Asia: A Review. J Stroke 2017; 19: 286–294. 2017/09/29.
Cohen-Mansfield J, Skornick-Bouchbinder M, Brill S. Trajectories of End of Life: A Systematic Review. J Gerontol B Psychol Sci Soc Sci: Series B. 2018;73:564–72.
Bostwick D, Wolf S, Samsa G, et al. Comparing the palliative care needs of those with cancer to those with common non-cancer serious illness. J Pain Symptom Manage. 2017;53:1079–84.
Stevenson J, Abernethy AP, Miller C, et al. Managing comorbidities in patients at the end of life. BMJ. 2004;329:909–12.
Acknowledgements
The authors acknowledge the support of the Comprehensive Palliative Care Center in providing the data for this study and the Family Medicine Department Research Unit for statistical support.
Funding
The author(s) received no financial support for the research, authorship, and/or publication of this article.
Author information
Authors and Affiliations
Contributions
PP, ND, CP and CA contributed to the design of the study. PP, KP, and LC collected the data. All authors were involved in the analyses and the interpretation of the data. PP, ChanA, and ND drafted the manuscript. All authors critically revised the manuscript and approved the final version of the manuscript and agreed to be accountable in all aspects of the work. The corresponding author attests that all authors meet authorship criteria.
Corresponding author
Ethics declarations
Ethics approval and consent to participate
The research had been conducted in accordance with the Declaration of Helsinki. Ethical approval was granted from The Research Ethics Committee Faculty of Medicine, Chiang Mai University (Reference number 163/2019). The research was retrospective in nature, therefore, informed consent from research participants were not applicable. However, according to the routine clinical care in our palliative care unit, all participants (or their proxies, if the participants were unable to give consent) gave informed consent for telephone follow-up after hospital discharge.
Consent for publication
Not applicable.
Competing interests
The authors declare that they have no competing interests.
Additional information
Publisher's Note
Springer Nature remains neutral with regard to jurisdictional claims in published maps and institutional affiliations.
Supplementary Information
Rights and permissions
Open Access This article is licensed under a Creative Commons Attribution 4.0 International License, which permits use, sharing, adaptation, distribution and reproduction in any medium or format, as long as you give appropriate credit to the original author(s) and the source, provide a link to the Creative Commons licence, and indicate if changes were made. The images or other third party material in this article are included in the article's Creative Commons licence, unless indicated otherwise in a credit line to the material. If material is not included in the article's Creative Commons licence and your intended use is not permitted by statutory regulation or exceeds the permitted use, you will need to obtain permission directly from the copyright holder. To view a copy of this licence, visit http://creativecommons.org/licenses/by/4.0/. The Creative Commons Public Domain Dedication waiver (http://creativecommons.org/publicdomain/zero/1.0/) applies to the data made available in this article, unless otherwise stated in a credit line to the data.
About this article
Cite this article
Prompantakorn, P., Angkurawaranon, C., Pinyopornpanish, K. et al. Palliative Performance Scale and survival in patients with cancer and non-cancer diagnoses needing a palliative care consultation: a retrospective cohort study. BMC Palliat Care 20, 74 (2021). https://doi.org/10.1186/s12904-021-00773-8
Received:
Accepted:
Published:
DOI: https://doi.org/10.1186/s12904-021-00773-8