Abstract
Background
The costs of performing research are an important input in value of information (VOI) analyses but are difficult to assess.
Objective
The aim of this study was to investigate the costs of research, serving two purposes: (1) estimating research costs for use in VOI analyses; and (2) developing a costing tool to support reviewers of grant proposals in assessing whether the proposed budget is realistic.
Methods
For granted study proposals from the Netherlands Organization for Health Research and Development (ZonMw), type of study, potential cost drivers, proposed budget, and general characteristics were extracted. Regression analysis was conducted in an attempt to generate a ‘predicted budget’ for certain combinations of cost drivers, for implementation in the costing tool.
Results
Of 133 drug-related research grant proposals, 74 were included for complete data extraction. Because an association between cost drivers and budgets was not confirmed, we could not generate a predicted budget based on regression analysis, but only historic reference budgets given certain study characteristics. The costing tool was designed accordingly, i.e. with given selection criteria the tool returns the range of budgets in comparable studies. This range can be used in VOI analysis to estimate whether the expected net benefit of sampling will be positive to decide upon the net value of future research.
Conclusion
The absence of association between study characteristics and budgets may indicate inconsistencies in the budgeting or granting process. Nonetheless, the tool generates useful information on historical budgets, and the option to formally relate VOI to budgets. To our knowledge, this is the first attempt at creating such a tool, which can be complemented with new studies being granted, enlarging the underlying database and keeping estimates up to date.
Similar content being viewed by others
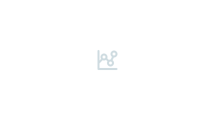
Avoid common mistakes on your manuscript.
Predicting budgets from study characteristics proves to be difficult. |
We designed a costing tool that, given certain details for a study, provides a range of historical budgets for comparable studies. |
This estimate can be used for VOI analysis, as well as for appraising budgets in grant proposals. |
1 Introduction
Evidence on cost effectiveness is increasingly required and is used for decisions to adopt or reimburse new health technologies. The analytical framework that is set up to produce this evidence also facilitates value of information (VOI) analysis, which aims to assess whether further research is worthwhile [1]. Moreover, VOI may nowadays be required with a new drugs submission, as, for example, in The Netherlands [2]. If the expected value of perfect information (EVPI) is non-negligible, an important step is the estimation of the expected net benefit of sampling (ENBS): the comparison of the costs of research to its added value. For this comparison, an accurate estimate of the costs of research is essential; however, currently there is no information available that can serve as a starting point for such estimates. From a consistency viewpoint, it would be desirable that these cost estimates were standardized within each decision-making jurisdiction. Additionally, for many grant programs, such as from the Netherlands Organization for Health Research and Development (ZonMw), reviewers need to assess the reasonableness of the proposed budget. Insight into the costs of research helps reviewers in this task. Finally, for both purposes, it would be valuable to enable easy access to the information by way of an electronic costing tool.
While some studies investigated the costs of randomized controlled trials (RCTs) [3,4,5,6,7,8] (often in pharmaceutical companies’ settings), little research has been done into the costs of other types of medical or epidemiological studies, such as cohort studies or literature reviews. Furthermore, existing studies used expert opinions or estimated costs of hypothetical projects, rather than actual observational data, and were aimed at, for instance, justifying the fee per patient received by the physician by demonstrating that treatment of participants in a trial was more expensive than treatment of non-participants. In addition, studies focusing on RCTs in companies’ settings might differ considerably from costs in an academic setting. Furthermore, studies concerning VOI analysis usually made rather simplistic assumptions about costs of research [9]. In contrast, recent publications on the use of VOI in practice do not even mention the issue of costing research, focusing more on the technicalities of VOI [10,11,12]. This shows that costing of various types of research is a neglected issue in VOI analysis, while it is indispensable to actual calculations of the Expected Value of Sampling Information and ENBS.
This paper aims to provide a pragmatic overview of the costs of several types of research. This overview enables two distinct purposes: (1) to provide an estimate of research costs of various study types for use in VOI; and (2) to develop an electronic costing tool that can be used to support reviewers of grant proposals in the task of evaluating the budget of the proposed project. Of note, results from our tool form part of what is required for a VOI analysis. Other elements of costs, such as opportunity costs falling on individuals enrolled in the less optimal treatment arm while performing the research, should be added separately.
2 Methods
2.1 Dataset
Grant proposals regarding drug-related health services research were obtained mainly from the ‘Goed Gebruik Geneesmiddelen’ (GGG) program of ZonMw. The GGG program is aimed at a more rational use of pharmaceuticals in clinical practice. Although we also received proposals from other ZonMw programs, all proposed projects in the dataset were drug-related. Only projects that were granted over the period 2007–2014, and that had also actually started, were selected. The latter was done to ensure the quality of the included projects and budgets, since unrealistic budgeting may be a reason for rejection or early cancellation.
2.2 Classifying the Studies
For all project proposals in the initial selection, the type of study was assessed, alongside some general characteristics, such as title and date of submission. Existing classifications [13, 14] were adapted to our specific setting, leaving seven possible study types (see Fig. 1): qualitative, observational cross-sectional, randomized controlled, non-randomized controlled, observational longitudinal, literature review, and data synthesis. Note that as we considered the two types of observational cross-sectional studies (descriptive and analytical) to be comparable with respect to costs, we merged these into one study type. The classification focused on designs using empirical data (either primary data gathering or reanalysis of secondary data) since that is the type of study considered when ‘additional research’ is at stake in a VOI analysis. Initially, for a subset of 21 proposals, study type was assessed by two reviewers (any combination of TF, MJ, MA, MP, PV, IL-L, BR, ICR and TA), and cases of disagreement were discussed until consensus was reached. This process aimed to create uniformity in interpretation of the heterogeneous study designs. Subsequently, the remainder of the proposals was assessed by a single reviewer (PV, ICR, TA, IL-L or BR). Proposals could consist of more than one study type.
2.3 Data Extraction
For each study type, an exhaustive list of possible cost drivers was drafted. Cost drivers were defined as those variables that, according to the authors, could possibly contribute to the costs of performing the proposed research. Although many cost drivers were quite generic for all study types, there were also cost drivers thought to be unique for a certain study type; therefore, the lists differed between study types.
The resulting sets of potential cost drivers were sent to an expert panel (n = 5) for feedback and adjusted accordingly. Three of the experts (all professors with a background in pharmaceutical sciences) were members of the review committee of the ZonMw GGG program, and, in that capacity, they regularly need to evaluate research proposals, including the accompanying budgets. The remaining two experts were a pharmacoeconomics advisor from the National Health Care Institute (Zorginstituut Nederland; ZiN) and a program manager from ZonMw. The outcomes were also presented at two ZonMw committee meetings (i.e. GGG and DO—Healthcare Efficiency) and commented on by experts present at these meetings. Members had been sent the study results in advance of the meeting.
Next to potential cost drivers and study type, study costs were extracted from the proposal budgets. An important distinction here was between budgets requested for granting by ZonMw and any co-financing incorporated. When part of the budget was obtained elsewhere, which is a requirement in some of the ZonMw programs, this part is said to be paid by co-financing. Co-financing can take many forms, e.g. sponsoring of study medication by industry, time contribution of staff from the research institute, or grants from other sources. However, even though this part of study costs was not paid for by ZonMw, it is still part of the actual costs of performing the research, which is what we were interested in. Therefore, study costs were considered to consist of the total sum of grants requested plus co-financing.
Data on all possible cost drivers were extracted by PV, ICR, TA, IL-L and BR, for a subset of the proposals. Consistency was ensured by performing double extraction for proposals that were identified as complex, and calculating the percentage of agreement for each variable, where agreement would be either 0 (for different values) or 1 (for identical values). All differences between reviewers in these proposals were investigated and, when necessary, revisions were made to the variables. For instance, to express the fact that an item was not present, one reviewer used ‘0’, while another reviewer used ‘no’. This would show up as a disagreement, and therefore we would revise all ‘no’ to ‘0’. When variables, even after revision, showed poor agreement (< 50%), they were excluded from further analyses. See appendix A for a detailed overview of the agreement per variable. Where necessary, costs were adjusted to the price level of January 1st 2014, using the general price index as suggested by the Dutch costing manual [15].
2.4 Data Analysis
Descriptive statistics were explored and univariate regression analyses were performed based on the dataset of extracted studies, relating costs to relevant predictors from the list of cost drivers. The predictors investigated were study type, number of substudies, sample size, expensive testing, multicenter versus monocenter, study duration, year of submission, Health Technology Assessment (HTA) included, and whether a study was part of the GGG program or not. This was done for all study types in combination, and for RCTs separately, since RCTs usually follow a rather standard design and were therefore thought to show a more straightforward relation between cost drivers and budget. Furthermore, additional information regarding program-specific restrictions, most importantly maximum budgets, was obtained and was used to find out whether these restrictions, rather than costs drivers, could explain actual budgets. Multivariate analyses were planned to be performed using statistically significant cost predictors from the univariate analyses.
2.5 Costing Tool
Based on the information obtained in the previous steps, an electronic costing tool was developed.
The tool was intended to link costs to type and size of study, and distinguish fixed costs (only dependent on the type of study) and variable costs (dependent on several size parameters).
3 Results
3.1 Study Types
From the 133 original proposals obtained from ZonMw, four were excluded, because for one proposal, the proposal document was missing, and three proposals were duplicates. Table 1 presents the number of studies per study type. A single project could contain multiple substudies; the proposal may, for instance, plan a literature review followed by an RCT, and, in addition, a qualitative study; therefore, the numbers do add up to more than 129. In total, 46 proposals contained two or more study types, while 83 proposals contained a single study type. Due to budget constraints, we were unable to extract data from all studies. Thus, since some of the study types were overrepresented in the initial selection of proposals, the subset for data extraction consisted of a subset of the RCT and longitudinal observational projects, plus the complete set of the other study types (see Table 1, third column). Selection of RCTs and longitudinal observational studies was performed randomly, apart from RCTs and longitudinal studies that contained multiple substudies, since these would be kept in the sample anyway because removing a qualitative study, for instance, was not an option. Final sets of cost drivers as used in the data extraction phase are available in Appendix B.
Average study duration was 34 months (standard deviation [SD] 9 months), and mean sample size for those studies that included subjects (n = 63) was 642 (SD 830).
3.2 Study Costs
Table 2 summarizes the main results regarding study costs. Total study duration was, on average, 34 months, and budgeted costs were, on average, €475,000 (including an average co-financing of €109,000), with a median value of €431,000. Costs ranged from €63,000 to €1.5 million. Figure 2 shows the distribution of costs in a histogram.
Costs per study type were analyzed in four categories, i.e. controlled, cross-sectional, longitudinal, and other (see Appendix C). Univariate regression analysis showed that at the 5% statistical significance level, only the longitudinal observational study type added significantly to total budget, while proposals including a controlled study (either randomized or non-randomized) were significantly more expensive at the 10% level. Median costs for proposals including an RCT (n = 35) were €482,000, compared with €447,000 for proposals including a longitudinal observational study (n = 24), and €343,000 for all other proposals (i.e. all proposals not including either of these, n = 15).
3.3 Relation Between Costs and Cost Drivers
Predictor variables (cost drivers) were not statistically significant (at the 5% level) in most univariate regression analyses (see Appendix D and Figs. 1–5 in Appendix E). Only study duration and GGG showed a significant relation to costs (see Fig. 3). In the case of study duration, the relation was positive, i.e. the longer the study duration, the higher the costs. For GGG, the coefficient was negative, and since yes/no was coded 1/0, this implies that GGG studies in general have lower budgets. Multivariate regression analysis (see Appendix C and D) confirmed the relation between study duration and total budget in the complete sample, but not in the sample with RCTs only. As for study types, longitudinal designs seem to be the most expensive, but this was not reproduced in the larger multivariate analysis including a wider range of covariates. No other significant associations were found.
3.4 Relation Between Cost and Program Restrictions
Figure 4 depicts the different programs and their restrictions regarding maximum budget and co-financing in relation to proposal budgets. Also in this case, a clear relation could not be found.
Total budget per ZonMw program (program name, percentage of co-financing required, maximum budget excluding co-financing). DO Doelmatigheids Onderzoek, HTA Health Technology Assessment methodology research, GGG Goed Gebruik Geneesmiddelen, DO FT Doelmatigheidsonderzoek Farmacotherapie, DO E&K Doelmatigheidsonderzoek Effecten & Kosten, DO VEMI Doelmatigheidsonderzoek Vroege Evaluatie Medische Interventies, max maximum
3.5 Choice of Costing Tool
Due to a lack of patterns in the observed data, a more modest aim of providing reference values was set for the tool and it was adjusted accordingly. Only items with sufficient agreement (i.e. ≥ 50%) were selected, as specified above. After discussion with the expert panel, items relating to Good Clinical Practice (CGP) requirements were dropped. Initially, studies that needed to comply with GCP requirements were expected to be expensive because of, for instance, the costs of monitoring. However, based on the project proposal, whether or not a study would be subject to GCP requirements turned out to be quite difficult to assess, and agreement on this variable was poor. Appendix F gives a screenshot of the input and output frames of the costing tool. For each item, the user can select to use it as a selection criterion and, if so, which value should be selected. Since the more selection criteria, the smaller the set of relevant studies, the user is warned once selection criteria are set too tight and less than five studies remain for comparison. Warnings also appear about the number of studies with missing values on an outcome. When there are sufficient studies for comparison, the tool returns the median total budget (as well as, for example, median budget for personnel and amount of co-financing) for comparable studies, based on the chosen criteria. The tool also provides minimum and maximum and 25th and 75th percentiles. In this way, the tool can be used to provide reference values for use in a VOI, and can also be used to support grant application reviewers.
4 Discussion
Costs for actual health services research, as subsidized by the national organization for health research and development in The Netherlands, ranged from €63,000 to more than €1,500,000, with a mean of €475,000. Study costs showed little relation to obvious explanatory variables such as sample size, expensive testing, or number of study locations. The only relation that was consistently statistically significant was for study duration
Overall study costs showed a wide variety, with seemingly similar studies being costed very differently. Various explanations can be offered. First, program restrictions may have been an incentive for researchers to apply for more costs than actually needed since they tend to try obtaining the highest possible funding, rather than what is needed. On the other hand, applicants may skimp on personnel and materials, for instance, of an otherwise expensive clinical trial, to fit the maximum allowed budget. While auditing precludes profits to be made on public funding, losses are in no way prohibited, and are known to occur in practice, and by scaling up the budget researchers try to avoid a negative financial result after the project. However, Fig. 4 did not convincingly show clustering of budgets towards the maximum budget of the programs. Moreover, the GGG program, which has no maximum budget restrictions, tended to result in lower budgets. Second, researchers may be poor budgeters, since their expertise is in other fields and, as discussed above, consequences of miscalculations are often found in overwork or co-financing from within the institute. Third, institutional rules regarding overhead and additional material costs may vary within The Netherlands.
Co-financing was another highly variable item. It was impossible to verify whether these budgeted amounts were actually obtained and used during the study. Finally, it was worrisome that many proposals showed different versions of the budget within the same proposal, which indicates this is not an issue that is reviewed very scrupulously in the current procedure.
Another striking finding was the large number of ‘not reported’ elements/items in our dataset (see Appendix A for an overview per variable). This implies that we were unable to extract information from the grant proposals on many items that we and our expert panel considered relevant for study costs a priori, such as hours or full-time equivalent of personnel needed, follow-up time, or total study duration. Since these items also seem quite relevant for a clear description of the study design in general, that is rather surprising.
The classification of the studies turned out to be feasible, and consistency between different researchers was satisfactory. It was however a complicating factor that many proposals (46 of 129) had more than one study type, and hence budgets could not be simply allocated to a single study design. For the application in VOI in particular, extension with more single studies would be desirable.
The one factor that consistently came out significantly affecting costs was study duration, or approximations thereof. In the regression on study designs, the longitudinal studies were shown to be more expensive; however, this may also be related to study duration. It should be kept in mind that a Dutch PhD lasts between 36 and 48 months, which probably affects average study duration.
The small number of significant cost predictors could be attributed to a lack of power. This will certainly be an explanation for many of the more study-type-specific cost items, such as type of post-processing for qualitative data, or type of model in the case of data synthesis. However, a number of cost items were extracted for almost all study types, e.g. sample size, number of study locations, whether or not expensive tests were involved, and whether or not a cost-effectiveness analysis was performed, and none of these showed significance either. For variables such as sample size, a clear relation would have been expected a priori, but our findings did not support this expectation. Given the current data, we do not expect that adding more studies would result in a significant relation between sample size and study costs. For good costing of drug studies that have to fulfill GCP requirements, other datasets would be needed, including more industry-supported studies. On the other hand, as the current sample size did not allow for investigation of interactions between sample size and, for instance, study type or study duration, we cannot rule out the existence of these interactions, and adding more data could contribute to their detection.
A drawback of a tool based on historical data may be that past mistakes will translate into future errors. If many researchers underbudgeted their studies in the past, this will imply a burden to future study proposals when committees apply the historic reference values. On the other hand, no clear reason exists why we would expect such underbudgeting, except for anecdotal evidence. To evaluate whether budgets were accurate, it would be useful to incorporate information on the financial results of the projects, and also on feasibility in retrospect, e.g. to what extent projects were successful in recruiting the number of patients aimed for, and whether the project finished on time or needed to apply for an extension. Although recruitment numbers and extensions could be traced from the final project report, the financial settlement will not reflect the true issues with the budget. For instance, when the budget turns out to be too low, researchers will solve this by adding funds from their own department, or by deploying unpaid labor. These rather common solutions for financial and organizational problems will rarely be recorded officially. Because of this, the accuracy of the budget for past projects is difficult to assess. Another limitation of this study was that as the tool was developed using only proposals on drug-related studies, results from the tool may not be generalizable to non-drug research.
5 Conclusions
Summarizing, we conclude that the current tool should be considered as a work in progress that gains in value once more studies are added to it. Of note, our tool is not predictive, but rather shows what actual budgeted costs were for various types of studies performed in a Dutch academic setting over the past period. We certainly do not advocate to have the tool replace proper budgeting and the thorough assessment of presented budgets. Nevertheless, our present findings can be helpful to ZonMw, as well as ZonMw committees, to evaluate their current reviewing process with regard to study costs. For use in VOI analyses, it would be desirable to add more single-type studies. For the time being, the data may at least provide a better estimate than the very simplistic assumptions that have been applied up to now.
References
Claxton K, Posnett J. An economic approach to clinical trial design and research priority-setting. Health Econ. 1996;5:513–24.
Versteegh M, Knies S, Brouwer W. From good to better: new Dutch guidelines for economic evaluations in healthcare. Pharmacoeconomics. 2016;34:1071–4.
Eisenstein EL, Lemons PW 2nd, Tardiff BE, Schulman KA, Jolly MK, Califf RM. Reducing the costs of phase III cardiovascular clinical trials. Am Heart J. 2005;149:482–8.
Bennett CL, Adams JR, Knox KS, Kelahan AM, Silver SM, Bailes JS. Clinical trials: are they a good buy? J Clin Oncol. 2001;19:4330–9.
Emanuel EJ, Schnipper LE, Kamin DY, Levinson J, Lichter AS. The costs of conducting clinical research. J Clin Oncol. 2003;21:4145–50.
Fireman BH, Fehrenbacher L, Gruskin EP, Ray GT. Cost of care for patients in cancer clinical trials. J Natl Cancer Inst. 2000;92:136–42.
Goldman DP, Berry SH, McCabe MS, Kilgore ML, Potosky AL, Schoenbaum ML, et al. Incremental treatment costs in national cancer institute-sponsored clinical trials. JAMA. 2003;289:2970–7.
Du W, Reeves JH, Gadgeel S, Abrams J, Peters WP. Cost-effectiveness and lung cancer clinical trials. Cancer. 2003;98:1491–6.
Conti S, Claxton K. Dimensions of design space: a decision-theoretic approach to optimal research design. Med Decis Mak. 2009;29:643–60.
Tuffaha HW, Gordon LG, Scuffham PA. Value of information analysis in oncology: the value of evidence and evidence of value. J Oncol Pract. 2014;10:e55–62.
Steuten L, van de Wetering G, Groothuis-Oudshoorn K, Retel V. A systematic and critical review of the evolving methods and applications of value of information in academia and practice. Pharmacoeconomics. 2013;31:25–48.
Wilson WC. A practical guide to value of information analysis. Pharmacoeconomics. 2015;33(2):105–21.
Grimes DA, Schulz KF. An overview of clinical research: the lay of the land. Lancet. 2002;359:57–61.
Rohrig B, du Prel JB, Wachtlin D, Blettner M. Types of study in medical research: part 3 of a series on evaluation of scientific publications. DtschArztebl Int. 2009;106:262–8.
Guideline for economic evaluations in healthcare. Netherlands: Zorginstituut; 2015. https://english.zorginstituutnederland.nl/publications/reports/2016/06/16/guideline-for-economic-evaluations-in. Accessed 26 Apr 2017.
Acknowledgements
The authors thank Anne Paans for her contribution to this study, and we thank the experts involved for their useful feedback: Prof. dr. Y Hekster, who sadly passed away in 2015, Prof. dr. M Bouvy, Prof. dr. C. Knibbe, Dr. N Dragt, and Dr. B Vingerhoeds.
Author information
Authors and Affiliations
Contributions
TF supervised the project and, together with MJ, MA, and MP, conceptualized and designed the study. TA, BR, PV, IL, and ICR performed the data extraction. ICR performed the major part of the regression analyses, and PV designed the costing tool, supported by BR. TA and TF wrote the first draft of the manuscript. All authors contributed to subsequent drafts of the manuscript and responses to the peer reviewers.
Corresponding author
Ethics declarations
Funding
This research has been funded by an unrestricted ZonMw implementation (VIMP) grant, project number 80-82500-98-14005.
Conflicts of interest
Maarten Postma received grants and honoraria from various pharmaceutical companies, all unrelated to the content of the project underlying this paper. Talitha Feenstra is on the ZonMw committee for GGG and was on the ZonMw committee for expensive medicines; as such, she reviews and judges grant proposals and their budgets. Manuela Joore and Thea van Asselt are also members of the ZonMw committee for GGG. Maiwenn Al, Pepijn Vemer, Ivonne Lesman-Leegte, Bram Ramaekers, and Isaac Corro Ramos have no conflicts of interest to declare.
Data availability statement
The datasets generated and/or analyzed during the current study are not publicly available due to the nature of the data, which contains sensitive information on, for example, project groups, research plans, and detailed budgets. Granting of this project by ZonMw was under the strict condition of confidentiality with respect to the information made available to us.
Electronic supplementary material
Below is the link to the electronic supplementary material.
Rights and permissions
Open Access This article is distributed under the terms of the Creative Commons Attribution-NonCommercial 4.0 International License (http://creativecommons.org/licenses/by-nc/4.0/), which permits any noncommercial use, distribution, and reproduction in any medium, provided you give appropriate credit to the original author(s) and the source, provide a link to the Creative Commons license, and indicate if changes were made.
About this article
Cite this article
van Asselt, T., Ramaekers, B., Corro Ramos, I. et al. Research Costs Investigated: A Study Into the Budgets of Dutch Publicly Funded Drug-Related Research. PharmacoEconomics 36, 105–113 (2018). https://doi.org/10.1007/s40273-017-0572-7
Published:
Issue Date:
DOI: https://doi.org/10.1007/s40273-017-0572-7