Abstract
Introduction
This study aimed to quantify the potential epidemiological and health economic benefits of implementing preoperative anaemia measures (PAMs) in clinical practice in the German healthcare system.
Methods
An evidence-based health economic model was developed to assess the possible impact of implementing PAMs, the first pillar of patient blood management (PBM), in a German population. The analysis of two risk factors—iron-deficiency anaemia (IDA) and receipt of a red blood cell concentrate (RBC) transfusion during elective surgery—allowed the estimation of relative risks (RRs), average cost per patient, average length of hospital stay, and avoided hospital deaths after the implementation of PAMs.
Results
A total of 4,591,060 patients who had undergone elective surgery during 2015 were identified, of which 29,170 (0.64%) were diagnosed with preoperative IDA. These patients had an increased RR of receiving a RBC transfusion during surgery (RR 5.031; 95% confidence interval [CI] 4.928, 5.136) and increased mortality risk (RR 3.630; 95% CI 3.401, 3.874) versus patients without IDA. Patients who received a RBC transfusion during surgery had a 24.6-times higher risk of death than those who did not (RR 24.593; 95% CI 24.121, 25.075). Average cost of treatment was €7883 in patients with IDA, €21,744 in patients with IDA and RBC transfusion, and €4560 in patients without risk factors. The model identified 29,714 patients (0.65%) who had received a RBC transfusion and who potentially had IDA but remained undiagnosed before surgery. Hypothetical implementation of PAMs would have resulted in an estimated annual net hospital direct cost saving of €1029 million (1.58%) of total hospital direct costs of the German healthcare system and 3036 hospital deaths (0.07%) avoided.
Conclusions
This model estimated the impact of implementing PAMs for patients with IDA undergoing elective surgery. A significant number of deaths, costly treatments, and hospital days could have been avoided by the introduction of PAMs in routine clinical practice in Germany.
Similar content being viewed by others
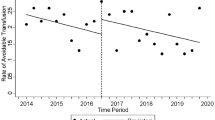
Avoid common mistakes on your manuscript.
Iron-deficiency anaemia in patients having elective surgery is associated with spending longer in hospital, an increase in the rate of blood transfusions, and higher mortality. |
In addition, receiving a red blood cell concentrate transfusion is associated with increased rates of illness, death, and length of stay in hospital. |
Using the patient blood management health economic model developed in this study, it was estimated that the implementation of preoperative anaemia measures in patients with iron-deficiency anaemia, undergoing elective surgery, would reduce the risk of death, length of hospital stay, and hospital costs. |
Introduction
Anaemia is defined as a reduced haemoglobin content of the blood or a lack of erythrocytes. According to the World Health Organization (WHO), anaemia, the thresholds for which were published in 1968 [1], can be triggered by the following events: increased loss of erythrocytes (blood loss); reduced production of erythrocytes; increased destruction of erythrocytes; or an increased physiological need for erythrocytes [2]. Anaemia is common in patients undergoing elective surgery; preoperative anaemia is detected in up to 40% of patients, with varying prevalence depending on the type of disease to be treated [3,4,5,6,7]. Iron-deficiency anaemia (IDA) is the most common type of preoperative anaemia, accounting for nearly two-thirds of cases globally [8]. Preoperative anaemia is associated with patients experiencing an increased length of hospital stay, a more complicated course of hospital treatment, an increase in the rate of blood transfusions, and higher mortality [3, 9, 10]. A growing number of studies have shown that receiving a red blood cell concentrate (RBC) transfusion, often used to treat anaemia, is associated in a dose-dependent relationship with increased morbidity, mortality, and length of hospital stay [11,12,13,14,15,16,17].
However, despite the known risks of anaemia and RBC transfusion, preoperative anaemia is still frequently ignored, with indiscriminate transfusion used as a ‘quick fix’. Munoz et al. identified ten misconceptions that constitute serious barriers to the wider implementation of preoperative anaemia measures (PAMs) [18]. Among these misconceptions were the following: preoperative anaemia may be caused by many conditions, some of which could be ameliorated or cured by the proposed surgery; preoperative anaemia poses no risk to patients, so scheduled procedures should not be delayed because of its presence; and the implementation of PAMs negatively impacts hospital personnel workload and is not cost-effective [18]. It was concluded that these misconceptions should be immediately abandoned by healthcare providers and replaced by evidence-based strategies such as detection, diagnosis, and proper treatment of preoperative anaemia [18].
The aim of this study was to estimate the potential clinical and health economic benefits of patient blood management (PBM) for patients undergoing elective surgery. A health economic model was developed to assess the impact of the implementation of one specific aspect of PBM, PAMs, in a large German population.
Patient Blood Management
PBM is a medical multidisciplinary approach to manage anaemia and is described in detail elsewhere [19]. Briefly, PBM is a set of measures designed to minimise risk in the pre-, intra-, and post-operative setting. PBM seeks to achieve better patient outcomes by relying on the patient’s own blood rather than on donor blood via transfusion; it achieves this by focussing on optimising erythrocyte mass, minimising blood loss and bleeding, and optimising physiological reserves to improve quality of care [16]. PBM is of critical importance for patients with anaemia or iron deficiency who are undergoing elective surgery, especially when they commonly exhibit a high probability of significant blood loss or transfusion [4, 20]. PBM measures directly focus on reducing risk factors associated with anaemia, as these may have a negative effect on treatment outcomes. For patients undergoing elective surgery, this means that both morbidity and mortality risk can increase. A higher morbidity risk could mean, for example, exacerbation of a chronic disease and an overall longer hospital stay as a consequence. A higher risk of mortality may be the result of complications.
Preoperative Anaemia Measures
The benefits of PBM, including the implementation of PAMs (principally iron therapy), would be experienced by many stakeholders, most notably patients, hospitals, the outpatient sector, healthcare funding providers, and health policymakers. International and national guidelines recommend preoperative treatment of IDA and iron deficiency with iron as the standard of care [4, 21,22,23,24,25,26,27]. PBM, including the use of PAMs and post-operative anaemia measures, have been shown to (1) shorten the length of hospital stay for patients undergoing emergency and elective surgery [28], in patients having cardiac surgery [29], major abdominal surgery [30], elective surgery [31], and hip and knee arthroplasty [32]; (2) lower the rate of surgical complications [28, 29, 31, 33]; (3) reduce mortality [28]; (4) reduce post-operative readmissions [32]; and (5) reduce the number of blood transfusions [28, 30,31,32,33,34,35]. Moreover, the economic benefits of PBM, including the use of PAMs, have been shown to (1) significantly reduce direct hospital costs of RBC transfusions per patient [36, 37]; (2) reduce overall hospital costs for patients undergoing hip and knee replacement surgery [32, 38,39,40] and major abdominal surgery [41]; (3) reduce costs to the healthcare system [42, 43]; and (4) improve patient safety by reducing surgical complications and mortality [28].
German Inpatient Healthcare System
In the system described in this study, the implementation of PBM incorporating PAMs would be organized and remunerated via the German Diagnosis-Related Groups (DRG) flat-rate system. The amount of DRG reimbursement depends on the type of illness (diagnosis), and the type and severity of operation required. Consequently, for patients with minor illnesses a smaller amount of remuneration is provided than for patients with serious illnesses who require more extensive treatment. The reimbursement per patient is calculated on the basis of an estimated range of length of hospital stay. Within this range, the same flat rate is paid regardless of the length of stay. For patients with a significantly longer or shorter length of stay, surcharges or discounts are paid on proof of medical necessity.
Preoperative treatment of a patient with iron deficiency (PAMs) prior to elective surgery is performed either at an outpatient practice (which refers the patient to hospital) or in a hospital outpatient clinic. In both cases, remuneration of PAMs is currently not regulated by the legislator in Germany, which suggests that there may be underuse of therapy in this area.
Methods
Identification of Population of Interest
Representative secondary data from the German database DRG Statistic for 2015 (DRG-Statistic 2015), made available for research purposes by the German Federal Statistical Office, was used to identify the population of interest for development of the PBM footprint model (RDC of the Federal Statistical Offices of the Länder, DRG-Statistic 2015; own calculations [44]). The DRG-Statistic 2015 is an official and representative data set of the German DRG-based hospital statistics for the year 2015. Information from the database includes sociodemographic and clinical characteristics (e.g. International Classification of Disease [ICD] and operation and procedure key [OPS; Operationen- und Prozedurenschlüssel]) of patients plus reimbursement prices based on DRGs. Patients included in the database are aged 0 to 99+ years, and the data were restricted to 2015 only.
The DRG-Statistic 2015 included a complete survey of all DRG hospitals and thus all inpatients in Germany who were billed according to the DRG system. It did not include hospitals that did not bill according to DRG (e.g. purely private hospitals, accounting for approximately 550,000 patients).
Of the 18 million patients included in the DRG-Statistic database, a random 10% sample of data from approximately 1.87 million patients from the complete survey was extracted. More specifically, in this assessment IDA was defined as ICD-10 German modification codes D50.8 (other IDAs) and D50.9 (IDA unspecified). Patients were identified as having received a RBC transfusion if recorded in the database as OPS code 8-800.c (a German modification of the International Classification of Procedures in Medicine coding for medical procedures) [45]. Using extrapolations of our own data and comparisons with other published results from the DRG-Statistic 2015, we can conclude that the 10% sample is highly representative (with a minimal deviation of generally less than 5%).
Compliance with Ethics Guidelines
As the data are secondary data, ‘Guideline 1: Ethics’ according to the recommendations of the ‘AGENS’ working group is applied for the analysis: “The recommendation to consult with an ethics committee need not apply to secondary data analyses, if all the data protection provisions on pseudo-anonymization of all personal data are fulfilled (see also Guideline 8) and no link to primary data is intended” [46]. The fulfilment of data protection provisions on pseudo-anonymization of all personal data is controlled by the German Federal Statistical Office. See ‘General terms of use’ [47] and ‘Regulations on the analysis of microdata’ [48]. The German database DRG-Statistic 2015 is available for research purposes from the German Federal Statistical Office.
Health Economic Model
Simulations are widely used in economic research to analyse the characteristics and behaviour of an economy or its subsystems, for instance, the healthcare system [49]. Economic simulation is intended to show the effects of health and economic policies by modelling socio-economic processes. In order to achieve this, the institutional and other conditions of the system to be analysed are changed. Microsimulation models have the benefit of having a large number of individual-level data that can be aggregated. If selected diagnoses, blood products used, outcomes, resulting effects, and correlations are to be analysed, it is essential to use individual level data. Large samples (n > 1 million patients) can ultimately only be analysed by using microsimulation models, if the different individual characteristics are to be fully considered. Data from DRG-Statistic 2015 were consequently analysed using microsimulation methods in order to quantify the epidemiological and economic effects of PBM and the implementation of PAMs. The model described here is the output of a comprehensive microsimulation model known as the ‘PBM footprint’ model.
Rationale for Estimating Epidemiological and Economic Impact
Methodology for Estimation of Relative Risks
To calculate the epidemiological and economic impact, patients were divided into subgroups, e.g. subgroups with no, one or two risk factors (IDA and RBC transfusion) or subgroups with no or one risk factor and no or a (negative) endpoint (mortality). From this, 2 × 2 tables with subgroup patient numbers were quantified (frequencies), which were then used to calculate the relative risk (RR).
The following RRs were calculated: (1) the RR of a patient with IDA receiving a RBC transfusion; (2) the RR of mortality in those patients with IDA; and (3) the RR of mortality in patients who received a RBC transfusion.
To estimate the association between IDA and risk of receiving a RBC transfusion, the following equation was used:
when:
Risk factor 2 | ||
---|---|---|
RBC transfusion | No RBC transfusion | |
Risk factor 1 | ||
IDA | a | b |
No IDA | c | d |
To calculate the association between mortality and each of the risk factors (IDA and receiving a RBC transfusion), the following equation was used, where a, b, c, and d relate to the number of patients for the population:
When:
Deceased | Not deceased | |
---|---|---|
Risk factor | a | b |
No risk factor | c | d |
In addition, the average cost per patient and the average days in hospital were calculated for each subgroup (a, b, c, d) of patients, and subsequently used as key parameters to calculate the cost impact of implementing PAMs.
Estimating Size of Population with Undiagnosed IDA
IDA can be challenging to diagnose, and it can often be asymptomatic in presentation without the presence of anaemia [50]. Misconceptions about IDA and barriers to testing within healthcare practice mean that the number of patients diagnosed with preoperative IDA in the study sample was considered to be lower than the actual prevalence in the population.
To establish the proportion of patients who had undiagnosed preoperative IDA, a series of calculations were made according to evidence-based assumptions from the literature.
-
1.
It is known that 20–40% of patients having elective surgery have preoperative anaemia [5,6,7, 10, 23, 51, 52]; a figure of 30% was selected because this represents the median percentage in the range.
-
2.
Kassebaum et al. stated that 63% of preoperative anaemia cases are attributable to iron deficiency [8], whereas other studies report that approximately 50% of anaemia cases are attributable to iron deficiency [53, 54]. In order to avoid overestimation, the more conservative figure of 50% was selected to be used in the population estimation calculations.
-
3.
Generally, more than 90% of patients with preoperative IDA are not treated for the condition [55, 56].
On the basis of these assumptions, an estimated 13.5% of patients undergoing elective surgery are thought to be undiagnosed and untreated for preoperative IDA; Fig. 1 illustrates the calculation rationale.
This estimated figure was subsequently applied to the patient group who had not been diagnosed with IDA and had received one or more RBC transfusions. This was based on the rationale that this patient group, comprising those undiagnosed and untreated for preoperative IDA, is exposed to the greatest risks when undergoing elective surgery and receiving RBC transfusions.
Reallocation of Patients After Implementation of PAMs
The aim of this part of the model was to establish the reallocation of patients based on the understanding that a proportion of patients would no longer be considered to have IDA following treatment with PAMs; in effect, PAMs had resolved their IDA. To inform these calculations, the findings of Froessler et al. [30] were taken into consideration; Froessler reported that 87.5% of a given population would no longer require a RBC transfusion after the implementation of PAMs. Figure 2 illustrates the calculations used in the model to reallocate the population after implementing PAMs (step 2).
Schematic representation of the potential redistribution of patients after the implementation of PAMs in the representative sample population of patients in the Diagnosis-Related Groups Statistic for 2015 who had undergone elective surgery (N = 4,591,060). Source: RDC of the Federal Statistical Offices of the Länder, DRG-Statistic 2015; own calculations [44]. aThe proportion of 13.5% is based on calculations shown in Fig. 1. bThe proportion of 87.5% is based on the findings of Froessler et al. [30] IDA iron-deficiency anaemia, PAMs preoperative anaemia measures, RBC red blood cell concentrate
Estimating Impact of PAMs in Germany
The economic impact of the implementation of PAMs on the target population in Germany was estimated, via the health economic model, by the calculation of total hospital cost savings minus the PAM therapy costs attributable to the treated patients. The estimation of total hospital cost savings is derived from the following two sources:
-
1.
The new distribution of patients among the four groups according to risk factors. In short, the implementation of PAMs modifies the original distribution such that there is a relatively low number of the costliest patients—those with the two risk factors (IDA and RBC transfusion). The estimated average cost per patient for each group and the comparison of the distribution of patients between the two scenarios (i.e. before and after implementing PAMs) give rise to potential hospital cost savings.
-
2.
The estimated average number of days in hospital, for each one of the four groups of patients, and the comparison between the original and final distribution of patients yield the number of avoided hospital days after implementing PAMs. The translation of potentially avoided hospital days into cost savings is made by using the average cost of an elective surgery hospital stay in Germany.
IDA Treatment Costs
For direct treatment costs necessary to perform PAMs, i.e. to treat patients with IDA prior to elective surgery in such a way that they enter surgery without IDA risks, the costs shown in Table 1 can be assumed [57, 58].
Avoided Direct Hospital Costs
The estimation of direct hospital costs was determined as follows: firstly, the average costs per patient, for each group of patients according to risk factors, were derived. These average costs were multiplied by the patient distributions of the two scenarios (i.e. before and after the implementation of PAMs) according to Fig. 2, resulting in a new cost structure. The cost savings were then calculated by comparing the total costs of the new scenario with the original total costs.
Avoided Hospital Days and Additional Cost Savings
The epidemiological impact of implementing PAMs was assessed via the PBM health economic model, by comparing the total number of hospital days before and after the implementation of PAMs. The average number of hospital days for the original population (allocation groups a, b, c, d) was derived from DRG-Statistic 2015. The new patient allocation based on the implementation of PAMs was then multiplied by this average number of hospital days to provide the projected total number of avoided hospital days for each of the four groups. The following table shows the average number of hospital days per person for each group before the implementation of PAMs, which was used to calculate the final results:
RBC transfusion | No RBC transfusion | |
---|---|---|
IDA | (a) 27.83 | (b) 11.45 |
No IDA | (c) 23.63 | (d) 5.46 |
The total avoided hospital days was calculated using the following formula:

The translation of potentially avoided hospital days into cost savings is made by using the average cost of an elective surgery hospital stay in Germany. The average cost of a hospital stay for a patient having elective surgery in Germany in 2015 was €844 per day (RDC of the Federal Statistical Offices of the Länder, DRG-Statistic 2015; own calculations [44]).
Avoided Hospital Deaths
The epidemiological impact of implementing PAMs was also assessed by comparing the total number of avoided hospital deaths before and after the implementation of PAMs.
The 4,591,060 elective patients were categorised in DRG-Statistic 2015 according to their reason for hospital discharge. The number of patients in each of the four groups (a, b, c, d) who were ‘discharged due to death’ was calculated, within groups, as a percentage of the total number of discharges for all reasons; this is known as the percentage mortality for each of the four groups. These percentages were then used to project the number of avoided deaths in the new allocation of patients based on the implementation of PAMs. The percentage mortalities used for each of the four groups are shown here:
RBC transfusion | No RBC transfusion | |
---|---|---|
IDA | (a) 10.31% | (b) 0.77% |
No IDA | (c) 9.79% | (d) 0.40% |
For calculation of the avoided hospital deaths, the following two formulas were used:
Formula 1:

Formula 2:

Univariate Sensitivity Analysis
Since some uncertainty remains regarding the parameters used to inform the evidence-based assumptions used in Fig. 1 (to estimate the proportion of patients who had undiagnosed preoperative IDA), assumptions about the parameter bandwidths were set up in order to perform a univariate sensitivity analysis with regard to the final results of costs and hospital days.
In addition, a sensitivity analysis was conducted for the assumption used in Fig. 2 to inform the calculation of reallocation of patients with IDA who no longer need a RBC transfusion after receiving PAMs.
The percentage mortality for each of the four groups (a, b, c, d) was then used to project the number of avoided deaths in the new allocation of patients based on the implementation of PAMs and based on the scenarios in the sensitivity analysis (minimum, base case, and maximum scenario).
Results
Total Population
In total, 18.555 million patients were treated in German hospitals; of these, 4.591 million patients underwent elective surgery. The average cost of all patients was €3516 per patient and €5399 for the target population. The average hospital stay was 6.29 days for all patients and 6.40 days for the target population. A total of 3.151 million blood bags were given as RBC transfusion in 2015 (all patients). Of these, 1.026 million RBC blood bags were given to the target population. See Table 2 for full results for the ‘all patients’ and ‘elective surgery’ populations.
Target Population
A total of 4,591,060 patients who had undergone elective surgery during 2015 were identified in DRG-Statistic 2015 for inclusion in the study. Of these, 308,190 patients were identified as having a diagnosis of anaemia (ICD D50.0, D50.8, D50.9, D62, and E61.1). In addition, 227,390 patients (both IDA and non-IDA) aged between 0 and 99+ years who underwent elective surgery and had received a RBC transfusion as part of the procedure were identified from the database.
The target population of interest comprised two groups: (1) 29,170 patients (patients with IDA: ICD D50.8 and D50.9) who had undergone elective surgery and were diagnosed as preoperatively iron deficient, who had either received (24%) or not received (76%) a RBC transfusion during their elective surgery procedure (a + b in the original allocation); (2) the expanded target population within the health economic model, which consists of the identified, undiagnosed 29,714 patients with IDA for whom a PAM was hypothetically implemented (Fig. 2).
Estimation of Key Epidemiological and Economic Parameters Using Database Information
Association Between IDA and Mortality: If a patient with IDA underwent elective surgery, their risk of death was calculated to have been 3.6-times higher than that of a patient without IDA (RR 3.630; 95% CI 3.401, 3.874). Table 3 details the patient numbers and full RR and odds ratio (OR) results.
Association Between RBC Transfusion and Mortality: If a patient received a RBC transfusion during a hospital stay after elective surgery, the risk of death was 24.6-times higher than in a non-transfused patient (RR 24.593; 95% CI 24.121, 25.075) (Table 3).
Association Between IDA and RBC Transfusion: If a patient with IDA underwent elective surgery, the risk of receiving a RBC transfusion was 5.0-times higher than that of a patient without IDA (RR 5.031; 95% CI 4.928, 5.136) (Table 3).
Average Cost per Patient: The average cost of treatment was €7883 in patients with IDA (without RBC transfusion) compared with €4560 for a patient without IDA; this equates to a 173% higher cost for patients with IDA (Table 4). The average cost of treating a patient with the two risk factors (IDA and RBC transfusion) was €21,744, compared with €4560 for a patient without either risk factor; this equates to a 477% higher cost for patients with both risk factors (Table 4).
Average Number of Days in Hospital per Patient: The average number of days in hospital for a patient with IDA (without RBC transfusion) was 11.45 days, compared with 5.46 days for a patient without risk factors (Table 4). The average number of hospital days as an inpatient for an individual with IDA and RBC transfusion was 27.83 days, compared with 5.46 days for a patient without risk factors (507%) (Table 4).
Obtaining New Allocation of Patients After Implementation of PAMs
The impact of implementing PAMs is shown in Fig. 2 and the final figures are shown in Table 5.
Step 1 Using the estimation that 13.5% of patients are undiagnosed with IDA, in Table 5 13.5% of the 220,100 patients who had received a RBC transfusion but were not treated for IDA would transfer to cell 'a' as diagnosed with IDA; 29,714 patients were reallocated to the IDA population row and were added to the existing 7080 patients (total of 36,794 patients).
Step 2 From the findings of Froessler et al. [30], a value of 87.5% of the 36,794 patients was considered to be the population that would no longer require a RBC transfusion after the implementation of PAMs. Consequently, from cell ‘a’ 32,194 patients were reallocated to cell ‘d’ (Table 5).
These estimations suggest that the implementation of PAMs would reduce the number of patients with IDA receiving a RBC transfusion to 4599, and the total number of patients receiving a RBC transfusion would reduce from 227,180 to 194,986 after the implementation of PAMs (Table 5). The undiagnosed and allocated patients were included in these model results.
Economic Impact of Implementing PAMs in Germany
As previously indicated, the estimation of potential hospital direct cost savings was derived from two sources (average cost per patient and average number of hospital days) and combined to give a total hospital cost saving of implementing PAMs.
Estimation of Hospital Cost Savings Using Mean Cost per Patient
The average costs per patient for elective surgery treatment both with and without a RBC transfusion in patients with and without IDA are shown in Table 6; this also details the total hospital direct costs of treatment of patients with and without IDA before and after the implementation of PAMs. The total hospital costs for 2015 were €65,247 million [59]. It was estimated that the implementation of PAMs in the German population studied would have resulted in an annual hospital direct cost saving of €536 million.
Estimation of Hospital Cost Savings Using Average Number of Hospital Days
The average cost of a hospital stay for a patient having elective surgery in Germany in 2015 was €844 per day (RDC of the Federal Statistical Offices of the Länder, DRG-Statistic 2015; own calculations [44]). The estimated number of annual hospital days for patients having elective surgery was projected to be reduced by 596,070 days after the implementation of PAMs (Table 7). This equates to an estimated annual additional cost saving of €503 million for 2015 if PAMs had been implemented. These additional cost savings result from a lower utilization/occupancy rate of hospital beds. The cost savings from a lower occupancy rate can then be used to improve efficiency. There is no double counting of costs, as the avoidable direct hospital costs refer to reduced treatment costs (e.g. fewer complications) and the avoidable costs relate to reduced inpatient infrastructure use.
Estimation of Total Hospital Cost Savings of Implementing PAMs
The aggregated impact on hospital cost savings accounts for €1039 million (€536 million + €503 million). It was estimated there were 58,884 undiagnosed and existing patients with IDA in the German population during 2015. The average cost per patient of IDA therapies in Germany during 2016 was €176.68 (with no differences in costs reported between 2015 and 2016, see Table 1) [57, 58]. The annual cost of implementing PAMs in Germany was estimated to be €10.4 million. Therefore, the net hospital direct cost saving of implementing PAMs in Germany for 2015 would have been €1029 million. This accounts for approximately 1.58% of total hospital direct costs of the German healthcare system.
The annual cost of implementing PAMs in Germany is estimated to be €10.4 million, whereby 58,884 patients with IDA would receive IDA therapy preoperatively. If, instead of a 500 mg IV iron dose, 1000 mg was used throughout (see footnote to Table 1), the cost would be €17.3 million.
Epidemiological Impact of Implementing PAMs in Germany
On the basis of the new patient allocation after implementation of PAMs, it was projected that a total of 3036 hospital deaths would have been avoided if PAMs were implemented in Germany in 2015 (Table 8).
Univariate Sensitivity Analysis
Estimation of Patients Undiagnosed/Untreated for Preoperative IDA
A univariate sensitivity analysis was conducted to assess the robustness of the results regarding the evidence-based data used to inform the estimate of patients having elective surgery in 2015 who were undiagnosed/untreated for preoperative IDA. In order to be able to estimate the sensitivity, ranges were defined for the individual assumptions. The entry into and exit from the bandwidth are always 10% below and 10% above the known value, respectively. The following ranges were formulated for the three evidence-based assumptions used in Fig. 1:
-
Range for assumption 1 [0.10; 0.50], step size [0.05]
-
Range for assumption 2 [0.40; 0.70], step size [0.05]
-
Range for assumption 3 [0.80; 1.00], step size [0.05]
If the lowest and highest values of the ranges of assumptions 1–3 are multiplied by each other (lowest range: 0.10 × 0.40 × 0.80; highest range: 0.50 × 0.70 × 1.00), a common range is obtained: [0.032; 0.350]. The following values are assumed for the univariate sensitivity analysis for calculation: [0.032; 0.135; 0.350].
For these parameter values, results were then derived from the new distribution of patients after the implementation of PAMs. This means that the patient numbers were recalculated with the values 0.032–0.350. According to the patient redistribution model, the patients with undiagnosed IDA were redistributed from population ‘c’ to population ‘a’. The results are presented in Table 9.
Estimation of Reallocation of Patients with IDA Who No Longer Need RBC Transfusion After Receiving PAMs
A sensitivity analysis was also conducted on the data supporting the assumption used to estimate the reallocation of patients with IDA who no longer need a RBC transfusion after receiving PAMs (Fig. 2). The following range was formulated for this:
-
Range for assumption used in Fig. 2 [0.80; 0.95], step size [0.05]
The following values are assumed for the univariate sensitivity analysis: [0.80; 0.85; 0.875; 0.90; 0.95]. The minimum scenario (0.80), the base case scenario (0.875), and the maximum scenario (0.95) were determined. The results for these three scenarios are presented in Table 9.
The results of the sensitivity analysis (minimum, base case, and maximum scenario) for IDA treatment costs, avoidable direct hospital costs, avoidable hospital days (additional cost savings), total cost savings, and total cost savings as a percentage of the total national hospital budget for Germany are presented in Table 10.
Avoidable Deaths Following Implementation of PAMs
A sensitivity analysis was conducted to establish the minimum, base case, and maximum scenario for the number of deaths which could have potentially been avoided if PAMs had been implemented in Germany in 2015. As described earlier, the following ranges were formulated for this:
The minimum scenario (0.032 and 0.80), the base case scenario (0.135 and 0.875), and the maximum scenario (0.350 and 0.95) were determined. The percentage mortality for each of the groups was then used to project the number of avoided deaths in the new allocation of patients based on the implementation of PAMs. In the minimum scenario, 1083 deaths were avoidable, in the base case scenario the number of avoidable deaths was 3036, and in the maximum scenario it is estimated that 7518 lives could have been saved with the implementation of PAMs.
Discussion
This study demonstrates the epidemiological impact and economic costs to the German healthcare system of untreated preoperative IDA for patients undergoing elective surgery. Preoperative IDA is known to be associated with a range of risk factors, including increased likelihood of receiving a RBC transfusion [4, 20]. Calculations based on representative hospital data suggest that a patient with IDA had a five-times higher risk of receiving a RBC transfusion during elective surgery than a patient without IDA. In addition, preoperative IDA and receiving a RBC transfusion were both associated with increased mortality and hospital costs.
The knowledge of the risks associated with preoperative IDA led to the exploration of PBM, and specifically PAMs, as a clinical approach to the management of preoperative anaemia in patients having elective surgery. To our knowledge, this is the first study to carry out a comprehensive model-based secondary data analysis with representative hospital data (DRGs) for an entire country to demonstrate the beneficial impact of the implementation of PAMs for patients with preoperative IDA, both in routine clinical practice and at a wider healthcare system level. It should be noted that the study by Froessler et al. [30] already shows similar positive effects; however, that randomized clinical trial was based on a far smaller cohort and without specific DRGs.
The cost modelling used in this study shows that, for the population studied, the implementation of PAMs would help to ameliorate the impact of IDA for patients undergoing elective surgery. The positive benefits were most marked for the patient population with IDA and receiving a RBC transfusion, likely because these patients face the full array of heightened risks associated with both preoperative IDA and RBC transfusion. Because this population is the costliest to treat, the associated healthcare represents a substantial burden to the healthcare system; therefore, the introduction of a clinical management approach that would reduce this burden offers benefits to both the healthcare system and the patient. Other PBM measures to reduce patient risk, such as avoiding unnecessary blood loss during and after surgery and an economical RBC transfusion regimen, were not modelled when quantifying the cost savings. However, it is anticipated that the additional cost and benefit effects that could be achieved with those measures would be similarly impactful.
The results of this analysis demonstrate that the overall cost saving for the German healthcare system would be significant after the implementation of PAMs for patients undergoing elective surgery. For 2015, it was calculated that a net cost saving of €1029 million could have been made; equivalent to almost 1.58% of the total national hospital budget for Germany. This is the base case scenario; results of the sensitivity analysis suggest that when a maximum scenario is considered the saving could be as much as €2547 million (3.90% of hospital budget). The net cost saving includes lower direct and indirect costs. Lower direct costs from complication-free patient care result in lower direct treatment costs, such as lower personnel costs and less material used, e.g. RBC blood bags. Additional cost savings through saved treatment days and hospital capacity result in a reduction of the bed occupancy rate. This leads to a reallocation of hospital beds; fewer hospital beds can relieve burdens on health insurance funds and hospital staffing. It could therefore be considered that the implementation of PBM could provide reduced hospital direct treatment and indirect costs, while at the same time improving patient care. The analysis shows that direct and indirect cost savings are somewhat balanced (direct cost saving of €536 million, indirect cost saving of €503 million).
The findings of this study support the adoption of the best practice guidelines published by Munoz et al. [4]. These statements include a diagnostic approach for anaemia and iron deficiency in surgical patients; identification of patients appropriate for treatment; and advice on practical management and follow-up. When other specific population-based studies in the literature are considered, the findings from this study are in alignment. Calvet et al. conducted a study of 282 patients with colorectal cancer and anaemia, in which the cost impacts of three iron replacement strategies for reducing the use of RBC transfusion were assessed using cost-minimisation analyses. Intravenous ferric carboxymaltose therapy reduced hospital stay by 2.3 days compared with iron sucrose and by 2.6 days compared with oral iron therapy, resulting in cost savings of €485 and €274 per patient, respectively [60]. In a comparative cost assessment of intravenous iron supplementation options (including blood transfusion) to the healthcare economy in the UK, it was concluded that blood, as a source of iron, is expensive and is the least attractive option from a cost perspective [61]. Moreover, in a recent assessment of the cost benefits of PAMs in a German population, Froessler et al. reported that perioperative administration of PAMs resulted in hospital cost savings (€786 per case) based on reduced blood transfusions and length of stay after elective abdominal surgery [62].
There are, however, some potential limitations to this study. First, it should be noted that the ICD coding of IDA is not optimised throughout the entire healthcare provision, starting in the doctor's surgery, and continuing in the hospital. In Germany, this is partly because laboratory tests for IDA are state-regulated in terms of quantity and price, and consequently a service provider may be exposed to negative economic incentives when conducting these tests. This is likely to lead to an underreporting of IDA in clinical practice and an observed event rate for IDA that is lower than that previously reported in the literature. In this study, the data are derived from a single country population; however, because the German healthcare system shares many commonalities with healthcare systems of other developed nations, the findings could be considered relevant beyond Germany. The results presented relate to the German healthcare system in 2015; more up-to-date data sets are now available, and it is conceivable that the existing analysis could also be applied to the German DRG Statistics 2016 and DRG Statistics 2017 to explore whether comparable results can be generated. The development of the model was based on a series of evidence-based assumptions derived from the literature; because these numerical assumptions were based on previous studies and somewhat limited data, it is possible that this could introduce a risk of inaccuracy in the subsequent calculations. In order to limit this risk, a univariate sensitivity analysis was conducted. With regard to confounding, on the basis of the data and analysis structure used, we were only able to depict any confounding, if there was any, through the selection of subgroups and grouping logics, but not through regression analyses or similar. Future analyses could incorporate this. It would be possible to carry out further analysis of the data set by age group; however, this was not conducted as such a reduction in the number of patients per group would result in an undesirable increase in the coefficients of variation.
It is a strength of our study that data were derived from a comprehensive and detailed large database including elective surgery of all types, in patients of all ages. In addition, all numerical assumptions that formed the basis of the model were averaged and generally considered conservative (e.g. in the calculation, 30% was selected as the proportion of preoperative patients with anaemia, whereas the range from the literature in fact spanned 20–40%), suggesting that the impact of implementing PAMs could in fact be much greater than that reported here. Sensitivity analysis allowed the range of impact of PAMs to be examined in more detail; this showed that the influence of an implementation of PAMs could be smaller, but also significantly larger than the base case suggests.
This large database analysis has shown that the implementation of PAMs for patients of all ages undergoing elective surgery would provide a host of benefits in terms of health economics, epidemiology, and favourable patient outcomes. It is hoped that these results will inform recommendations in the future on the management of patients having elective surgery.
Conclusions
The model developed in this analysis provides a simple method for calculating the impact of addressing IDA for preoperative patients undergoing elective surgery and illustrates the epidemiological and economic potential for implementing PAMs in a large population.
The impact estimates can be assessed at both patient and healthcare system levels. The model estimates show that a significant number of deaths, hospital treatments, and costly hospital days could have been avoided by the implementation of PAMs in Germany. On the basis of the calculations in this study for 2015, the potential cost savings of implementing PAMs were substantial, accounting for approximately 1.58% of the total annual hospital costs in Germany. The model described here could readily be adapted to other healthcare systems. Not only would the implementation of PAMs offer economic benefits at a national healthcare level, but the real health benefits to patients of reduced risk of receiving a blood transfusion are also evident.
References
World Health Organization (WHO). Nutritional anaemias. Report of a WHO scientific group. Geneva, World Health Organization, 1968. WHO Technical Report Series, No 405. https://apps.who.int/iris/handle/10665/40707. Accessed June 2020.
World Health Organization (WHO). The clinical use of blood in general medicine, obstetrics, paediatrics, surgery and anaesthesia, trauma and burns. 2009. https://www.who.int/bloodsafety/clinical_use/en/Manual_EN.pdf?ua=1. Accessed June 2020.
Fowler AJ, Ahmad T, Phull MK, Allard S, Gillies MA, Pearse RM. Meta-analysis of the association between preoperative anaemia and mortality after surgery. Br J Surg. 2015;102(11):1314–24.
Munoz M, Acheson AG, Auerbach M, et al. International consensus statement on the peri-operative management of anaemia and iron deficiency. Anaesthesia. 2017;72(2):233–47.
Dunkelgrun M, Hoeks SE, Welten GM, et al. Anemia as an independent predictor of perioperative and long-term cardiovascular outcome in patients scheduled for elective vascular surgery. Am J Cardiol. 2008;101(8):1196–200.
Karkouti K, Wijeysundera DN, Beattie WS. Reducing bleeding in cardiac surgery I. Risk associated with preoperative anemia in cardiac surgery: a multicenter cohort study. Circulation. 2008;117(4):478–84.
Richards T, Musallam KM, Nassif J, et al. Impact of preoperative anaemia and blood transfusion on postoperative outcomes in gynaecological surgery. PLoS One. 2015;10(7):e0130861.
Kassebaum NJ, GBD 2013 Anemia Collaborators. The global burden of anemia. Hematol Oncol Clin North Am. 2016;30(2):247–308.
Beattie WS, Karkouti K, Wijeysundera DN, Tait G. Risk associated with preoperative anemia in noncardiac surgery: a single-center cohort study. Anesthesiology. 2009;110(3):574–81.
Musallam KM, Tamim HM, Richards T, et al. Preoperative anaemia and postoperative outcomes in non-cardiac surgery: a retrospective cohort study. Lancet. 2011;378(9800):1396–407.
Bernard AC, Davenport DL, Chang PK, Vaughan TB, Zwischenberger JB. Intraoperative transfusion of 1 U to 2 U packed red blood cells is associated with increased 30-day mortality, surgical-site infection, pneumonia, and sepsis in general surgery patients. J Am Coll Surg. 2009;208(5):931–7, 937.e1–2 (discussion 8–9).
Murphy GJ, Reeves BC, Rogers CA, Rizvi SI, Culliford L, Angelini GD. Increased mortality, postoperative morbidity, and cost after red blood cell transfusion in patients having cardiac surgery. Circulation. 2007;116(22):2544–52.
Koch CG, Li L, Duncan AI, Mihaljevic T, et al. Morbidity and mortality risk associated with red blood cell and blood-component transfusion in isolated coronary artery bypass grafting. Crit Care Med. 2006;34(6):1608–16.
Surgenor SD, Kramer RS, Olmstead EM, et al. The association of perioperative red blood cell transfusions and decreased long-term survival after cardiac surgery. Anesth Analg. 2009;108(6):1741–6.
Karkouti K, Wijeysundera DN, Yau TM, et al. Acute kidney injury after cardiac surgery: focus on modifiable risk factors. Circulation. 2009;119(4):495–502.
Hofmann A, Farmer S, Towler SC. Strategies to preempt and reduce the use of blood products: an Australian perspective. Curr Opin Anaesthesiol. 2012;25(1):66–73.
Goel R, Patel EU, Cushing MM, et al. Association of perioperative red blood cell transfusions with venous thromboembolism in a North American registry. JAMA Surg. 2018;153(9):826–33.
Munoz M, Gomez-Ramirez S, Kozek-Langeneker S, et al. 'Fit to fly': overcoming barriers to preoperative haemoglobin optimization in surgical patients. Br J Anaesth. 2015;115(1):15–24.
Gombotz H, Hofmann A. Patient blood management: three pillar strategy to improve outcome through avoidance of allogeneic blood products. Anaesthesist. 2013;62(7):519–27.
Meybohm P, Froessler B, Goodnough LT, et al. “Simplified International Recommendations for the Implementation of Patient Blood Management” (SIR4PBM). Perioper Med (Lond). 2017;6:5.
Kozek-Langenecker SA, Ahmed AB, Afshari A, et al. Management of severe perioperative bleeding: guidelines from the European Society of Anaesthesiology: first update 2016. Eur J Anaesthesiol. 2017;34(6):332–95.
European Board of Anaesthesiology (EBA). European Board of Anaesthesiology (EBA) recommendations for preoperative anaemia and patient blood management. 2020. http://www.eba-uems.eu/resources/PDFS/safety-guidelines/EBA--Preop-anaemia-recommend.pdf. Accessed June 2020.
Goodnough LT, Maniatis A, Earnshaw P, et al. Detection, evaluation, and management of preoperative anaemia in the elective orthopaedic surgical patient: NATA guidelines. Br J Anaesth. 2011;106(1):13–22.
National Institute for Health and Care Excellence. NICE guideline [NG24]. Blood transfusion. Published 18 November 2015. 2015. https://www.nice.org.uk/guidance/ng24. Accessed June 2020.
National Blood Authority Australia. Patient blood management guidelines: module 2 perioperative. 2012. https://www.blood.gov.au/system/files/documents/pbm-module-2.pdf. Accessed June 2020.
AWMF. Präoperative Anämie. Diagnostik und Therapie de Präoperativen Anämie. AWMF Registernummer 001–0024 Version 1.0. 2018. https://www.awmf.org/uploads/tx_szleitlinien/001-024l_S3_Praeoperative-Anaemie_2018-04.pdf. Accessed June 2020.
Wang Y, Wang X. Chinese experts consensus statement on patient blood management in patients undergoing cardiovascular surgery. Chin J Blood Transfus. 2018;31(4):321–5.
Leahy MF, Hofmann A, Towler S, et al. Improved outcomes and reduced costs associated with a health-system-wide patient blood management program: a retrospective observational study in four major adult tertiary-care hospitals. Transfusion. 2017;57(6):1347–58.
Gross I, Seifert B, Hofmann A, Spahn DR. Patient blood management in cardiac surgery results in fewer transfusions and better outcome. Transfusion. 2015;55(5):1075–81.
Froessler B, Palm P, Weber I, Hodyl NA, Singh R, Murphy EM. The important role for intravenous iron in perioperative patient blood management in major abdominal surgery: a randomized controlled trial. Ann Surg. 2016;264(1):41–6.
Khalafallah AA, Yan C, Al-Badri R, et al. Intravenous ferric carboxymaltose versus standard care in the management of postoperative anaemia: a prospective, open-label, randomised controlled trial. Lancet Haematol. 2016;3(9):e415–25.
Kotze A, Carter LA, Scally AJ. Effect of a patient blood management programme on preoperative anaemia, transfusion rate, and outcome after primary hip or knee arthroplasty: a quality improvement cycle. Br J Anaesth. 2012;108(6):943–52.
Meybohm P, Herrmann E, Steinbicker AU, et al. Patient blood management is associated with a substantial reduction of red blood cell utilization and safe for patient's outcome: a prospective, multicenter cohort study with a noninferiority design. Ann Surg. 2016;264(2):203–11.
Rineau E, Chaudet A, Chassier C, Bizot P, Lasocki S. Implementing a blood management protocol during the entire perioperative period allows a reduction in transfusion rate in major orthopedic surgery: a before-after study. Transfusion. 2016;56(3):673–81.
Shuvy M, Mewa J, Wolff R, et al. Preprocedure anemia management decreases transfusion rates in patients undergoing transcatheter aortic valve implantation. Can J Cardiol. 2016;32(6):732–8.
Mehra T, Seifert B, Bravo-Reiter S, et al. Implementation of a patient blood management monitoring and feedback program significantly reduces transfusions and costs. Transfusion. 2015;55(12):2807–15.
Frank SM, Thakkar RN, Podlasek SJ, et al. Implementing a health system-wide patient blood management program with a clinical community approach. Anesthesiology. 2017;127(5):754–64.
Spahn DR, Theusinger OM, Hofmann A. Patient blood management is a win-win: a wake-up call. Br J Anaesth. 2012;108(6):889–92.
Cuenca J, Garcia-Erce JA, Martinez AA, Solano VM, Molina J, Munoz M. Role of parenteral iron in the management of anaemia in the elderly patient undergoing displaced subcapital hip fracture repair: preliminary data. Arch Orthop Trauma Surg. 2005;125(5):342–7.
Luporsi E, Mahi L, Morre C, Wernli J, de Pouvourville G, Bugat R. Evaluation of cost savings with ferric carboxymaltose in anemia treatment through its impact on erythropoiesis-stimulating agents and blood transfusion: French healthcare payer perspective. J Med Econ. 2012;15(2):225–32.
Froessler B, Foerster D, Hardt T, Rueger A, Connolly M. Treatment cost effects of perioperative anaemia treatment with ferric carboxymaltose. Transfusion Medicine. 2017;27(Suppl. 1):22–71, P86.
Robalo Nunes A, Brilhante D, Fonseca C, et al. Economic value of a nationwide patient blood management implementation in Portugal. Transfus Med. 2017;27(Suppl 1):33.
Shander A, Van Aken H, Colomina MJ, et al. Patient blood management in Europe. Br J Anaesth. 2012;109(1):55–68.
Statistische Amter Des Bundes Und Der Lander Forschungsdatenzentren. Metadata. 2016. https://www.forschungsdatenzentrum.de/en/10-21242-23141-2015-00-00-1-2-0. Accessed June 2020.
The German Institute of Medical Documentation and Information (DIMDI). The Operation and Procedure Code (OPS). 2020. https://www.dimdi.de/dynamic/en/classifications/ops/. Accessed June 2020.
Working Group for the Survey and Utilization of Secondary Data (AGENS) with representatives from the German Society for Social Medicine and Prevention (DGSMP) and the German Society for Epidemiology (DGEpi) and Working Group for Epidemiological Methods with representatives from the German Society for Epidemiology (DGEpi), the German Society for Medical Informatics, Biometry and Epidemiology (GMDS) and the German Society for Social Medicine and Prevention (DGSMP). GPS—Good Practice in Secondary Data Analysis: Revision after Fundamental Reworking. Version 2. January 2008. 2020. https://www.dgepi.de/assets/Leitlinien-und-Empfehlungen/Practice-in-Secondary-Data-Analysis.pdf. Accessed June 2020.
Research Data Centres of the Federation and the federal states (RDC). Regulations on the analysis of microdata. Version: 1 September 2017. 2017. https://www.forschungsdatenzentrum.de/sites/default/files/rdc_regulations_short_0.pdf. Accessed June 2020.
Research Data Centres of the Federation and the federal states (RDC). General terms of use. Version: 19 February 2020. 2020. https://www.forschungsdatenzentrum.de/sites/default/files/rdc_general_terms_of_use.pdf. Accessed June 2020.
Orcutt GH. A new type of socio-economic system. Rev Econ Stat. 1957;39:116–23.
Soppi ET. Iron deficiency without anemia—a clinical challenge. Clin Case Rep. 2018;6(6):1082–6.
Fowler AJ, Ahmad T, Abbott TEF, et al. Association of preoperative anaemia with postoperative morbidity and mortality: an observational cohort study in low-, middle-, and high-income countries. Br J Anaesth. 2018;121(6):1227–35.
Baron DM, Hochrieser H, Posch M, et al. Preoperative anaemia is associated with poor clinical outcome in non-cardiac surgery patients. Br J Anaesth. 2014;113(3):416–23.
Meybohm P, Fischer D, Schnitzbauer A, et al. Patient blood management: current state of the literature. Chirurg. 2016;87(1):40–6.
Clevenger B, Gurusamy K, Klein AA, Murphy GJ, Anker SD, Richards T. Systematic review and meta-analysis of iron therapy in anaemic adults without chronic kidney disease: updated and abridged Cochrane review. Eur J Heart Fail. 2016;18(7):774–85.
Gombotz H, Hofman A, Rehak P, Kurz J. Patient blood management (part 2). Practice: the 3 pillars. Anasthesiol Intensivmed Notfallmed Schmerzther. 2011;46(7–8):466–74.
Gombotz H, Zacharowski K, Spahn DR. Patient blood management. Stuttgart: Thieme. 2015 (ISBN 978-3-13-241077-0), eISBN 9783132004511.
Kleineruschkamp AG, Zacharowski K, Ettwein C, et al. Cost analysis of patient blood management. Anaesthesist. 2016;65(6):438–48.
Kleineruschkamp A, Meybohm P, Straub N, Zacharowski K, Choorapoikayil S. A model-based cost-effectiveness analysis of patient blood management. Blood Transfus. 2019;17(1):16–26.
Federal Statistical Office. Federal Statistical Office, Special Series 12, Series 6.4. Fallpauschalenbezogene Krankenhausstatistik (DRG-Statistik) Diagnosen, Prozeduren, Fallpauschalen und Case Mix der vollstationären Patientinnen und Patienten in Krankenhäusern. 2015. https://www.statistischebibliothek.de/mir/servlets/MCRFileNodeServlet/DEHeft_derivate_00029136/2120640157004_korr19012017.pdf. Accessed June 2020.
Calvet X, Gene E, AngelRuiz M, et al. Cost-minimization analysis favours intravenous ferric carboxymaltose over ferric sucrose or oral iron as preoperative treatment in patients with colon cancer and iron deficiency anaemia. Technol Health Care. 2016;24(1):111–20.
Bhandari S. Update of a comparative analysis of cost minimization following the introduction of newly available intravenous iron therapies in hospital practice. Ther Clin Risk Manag. 2011;7:501–9.
Froessler B, Rueger AM, Connolly MP. Assessing the costs and benefits of perioperative iron deficiency anemia management with ferric carboxymaltose in Germany. Risk Manag Healthc Policy. 2018;11:77–82.
Acknowledgements
Funding
This study, the journals’ rapid service fee and open access fee was supported by Vifor Pharma.
Medical Writing and Editorial Assistance
Medical writing assistance was provided by Rebecca Hornby and Charlotte Cookson of Oxford PharmaGenesis Ltd, Oxford, UK, and was funded by Vifor Pharma in accordance with Good Publication Practice.
Authorship
All named authors meet the International Committee of Medical Journal Editors (ICMJE) criteria for authorship for this article, take responsibility for the integrity of the work as a whole, and have given their approval for this version to be published.
Disclosures
Thomas Drabinski is a consultant to Vifor Pharma. Kai Zacharowski and Patrick Meybohm received research grants from B. Braun Melsungen, CSL Behring, Fresenius Kabi, and Vifor Pharma for the implementation of Frankfurt's Patient Blood Management Program and prizes from “Aktionsbündnis Patientensicherheit (APS)”, Lohfert-Stiftung AG, Masimo—Patient Safety Foundation, and MSD-Gesundheitspreis. Alexandra Rüger and Antonio Ramirez de Arellano are employees of Vifor Pharma.
Compliance with Ethics Guidelines
As the data are secondary data, ‘Guideline 1: Ethics’ according to the recommendations of the ‘AGENS’ working group is applied for the analysis: “The recommendation to consult with an ethics committee need not apply to secondary data analyses, if all the data protection provisions on pseudo-anonymization of all personal data are fulfilled (see also Guideline 8) and no link to primary data is intended” [46]. The fulfilment of data protection provisions on pseudo-anonymization of all personal data is controlled by the German Federal Statistical Office. See ‘General terms of use’ [47] and ‘Regulations on the analysis of microdata’ [48]. The German database Diagnosis-Related Groups Statistic for 2015 (DRG-Statistic 2015) is available for research purposes by the German Federal Statistical Office.
Data Availability
The datasets generated during and/or analysed during the current study are not publicly accessible due to the German Federal Data Protection Act but are available from the corresponding author on reasonable request.
Prior Presentation
Previously interim data related to this work have been presented at the following meetings: (1) Congress for Health Networkers, “Patient Blood Management—Milestone for Patient Safety”. 03 April 2019, Berlin. (2) 20th Annual NATA Symposium, Poster presentation, “Calculation of risks associated with preoperative anaemia and the health-economic footprint of patient blood management (PBM)”. 04 April 2019, Berlin. (3) ISPOR Europe 2019 Conference, Poster presentation, “Estimating the Clinical and Economic Impact of Implementing Preoperative Anemia Measures in the German Healthcare System: The Health Economic Footprint of Patient Blood Management (PBM)”. 04 November 2019, Copenhagen. In addition, a booklet was published in German in 2018. This covers the initial approaches, questions, analyses, and interpretations of the blood market in Germany and the PBM model. It was Volume 30 in the IfMDA series of publications under the name “Health Economic Footprint Patient Blood Management (PBM footprint)”. The analysis presented in this paper goes far beyond the previous discussion of the topic, as the sensitivity analysis and the resulting impact represent a fundamental new research approach.
Author information
Authors and Affiliations
Corresponding author
Additional information
Digital Features
To view digital features for this article go to https://doi.org/10.6084/m9.figshare.12229250.
Rights and permissions
Open Access This article is licensed under a Creative Commons Attribution-NonCommercial 4.0 International License, which permits any non-commercial use, sharing, adaptation, distribution and reproduction in any medium or format, as long as you give appropriate credit to the original author(s) and the source, provide a link to the Creative Commons licence, and indicate if changes were made. The images or other third party material in this article are included in the article's Creative Commons licence, unless indicated otherwise in a credit line to the material. If material is not included in the article's Creative Commons licence and your intended use is not permitted by statutory regulation or exceeds the permitted use, you will need to obtain permission directly from the copyright holder. To view a copy of this licence, visit http://creativecommons.org/licenses/by-nc/4.0/.
About this article
Cite this article
Drabinski, T., Zacharowski, K., Meybohm, P. et al. Estimating the Epidemiological and Economic Impact of Implementing Preoperative Anaemia Measures in the German Healthcare System: The Health Economic Footprint of Patient Blood Management. Adv Ther 37, 3515–3536 (2020). https://doi.org/10.1007/s12325-020-01372-4
Received:
Published:
Issue Date:
DOI: https://doi.org/10.1007/s12325-020-01372-4