Abstract
With disease-modifying approaches under evaluation in ataxia-telangiectasia and other ataxias, there is a need for objective and reliable biomarkers of free-living motor function. In this study, we test the hypothesis that metrics derived from a single wrist sensor worn at home provide accurate, reliable, and interpretable information about neurological disease severity in children with A-T.
A total of 15 children with A-T and 15 age- and sex-matched controls wore a sensor with a triaxial accelerometer on their dominant wrist for 1 week at home. Activity intensity measures, derived from the sensor data, were compared with in-person neurological evaluation on the Brief Ataxia Rating Scale (BARS) and performance on a validated computer mouse task.
Children with A-T were inactive the same proportion of each day as controls but produced more low intensity movements (p < 0.01; Cohen’s d = 1.48) and fewer high intensity movements (p < 0.001; Cohen’s d = 1.71). The range of activity intensities was markedly reduced in A-T compared to controls (p < 0.0001; Cohen’s d = 2.72). The activity metrics correlated strongly with arm, gait, and total clinical severity (r: 0.71–0.87; p < 0.0001), correlated with specific computer task motor features (r: 0.67–0.92; p < 0.01), demonstrated high reliability (r: 0.86–0.93; p < 0.00001), and were not significantly influenced by age in the healthy control group.
Motor activity metrics from a single, inexpensive wrist sensor during free-living behavior provide accurate and reliable information about diagnosis, neurological disease severity, and motor performance. These low-burden measurements are applicable independent of ambulatory status and are potential digital behavioral biomarkers in A-T.
Similar content being viewed by others
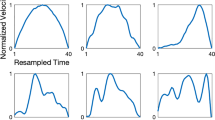
Avoid common mistakes on your manuscript.
Introduction
Ataxia-telangiectasia (A-T) is a rare autosomal recessive neurodegenerative disorder that affects one in every 40,000 to 100,000 children [1]. The neurological manifestation is characterized by progressive cerebellar atrophy and ataxia, peripheral neuropathy, and extrapyramidal features such as tremor, chorea, dystonia, and myoclonus[2, 3]. Heterogeneity in phenotype, day-to-day variability, and variable compliance with the neurological examination add to the challenge of accurately and precisely measuring disease severity in clinical practice and interventional trials. Tools are needed to objectively, frequently, and holistically monitor children with A-T outside the clinic, as they go through their usual daily routines and without requiring precise performance of specific tasks (i.e., during free-living conditions). Such tools have the potential to capture more ecologically valid information about the patient’s state and produce measures that better reflect how the disease impacts everyday function. Furthermore, more frequent or continuous sampling could reduce variance of disease assessments and enable more sensitive detection of changes in motor and cognitive behavior[4,5,6,7]. Detection of longitudinal, disease-related changes is important in initial diagnosis/determination of disease onset, monitoring for events that may require clinical intervention, and for determining efficacy in interventional trials. Wrist sensors with triaxial accelerometers are a promising tool for providing continuous movement information that is relatively low-burden and has the potential to be used in clinical practice and in clinical trials.
The use of wearable sensors for passive measurement of motor activity in real-world settings has yet to be investigated in A-T; however, it has shown potential in other neurodegenerative diseases including Parkinson’s disease[8], multiple sclerosis[9], and Huntington’s disease [10]. Assessments of balance[11, 12], gai [13], functional decline [14], and sleep quality [15] have been demonstrated, including applications of a paired sensor and vibro-tactile feedback system in neurorehabilitation [16]. Prior work in A-T has employed wearable sensors[17, 18] and computer mice[19] to measure limb motor activity during in-person administered tasks[20]. Recent work in other ataxias has focused on gait analysis from wearable sensor data in spinocerebellar ataxia in real-life settings[21] as well as in Friedreich’s Ataxia[22], ataxia from spinocerebellar degeneration[23], and SCA-6 in clinic settings[24]. Gait is a functionally and clinically important disease component in A-T; however, wheelchair use typically begins early in the second decade of life[25] thereby limiting the applicability of gait assessment in the second half of the disease.
Here we compared measures from a wrist-worn sensor to the physician-administered Brief Ataxia Rating Scale (BARS)[26, 27] and a validated computer mouse assessment of ataxia and parkinsonism[19] to assess free-living activity metrics as biomarkers in A-T.
Methods
Participants
Thirty-six age- and sex-matched children with and without A-T were enrolled in the study. All participants were identified in partnership with the Ataxia-Telangiectasia Children’s Project (A-TCP). Written informed consent and assent were obtained from all participants prior to participation and the study. Control participants were siblings of A-T participants. Exclusion from enrollment in this study included as follows: younger than 4 years old, inability to tolerate wearing a wrist sensor for 1 week, inability to perform the computer mouse task, and the presence of another neurological disorder or other condition that affects arm function or mobility. Inclusion in data analysis required wearing a sensor on the wrist of the dominant arm continuously for 1 week and undergoing an in-person neurological examination. Three participants were excluded from analysis for non-compliance: two participants removed the device during the data collection period, while another participant was unable to wear the device for more than 24 h due to unrelated life circumstances. One participant’s device ran out of battery after one day of data collection and was excluded from the study. Two additional A-T participants were excluded because a BARS score could not be performed: one was due to escalation of the COVID-19 pandemic, and another child was enrolled too late in the day to perform the full neurological exam. Of the 30 children who successfully completed the study, 15 had A-T and 15 were controls. All children with A-T were genetically confirmed to have the disease and were phenotypically characterized with the classic subtype upon clinical examination[1] (see Table 1). The median age of A-T and control participants was 10 and 11 years old, respectively. Participants with A-T spanned a range of ambulatory status from being able to walk without assistance (BARS gait subscore ≤ 4) to requiring a wheelchair for mobility (BARS gait subscore ≥ 7, see Table 1).
Wearable Sensor Data Collection
The study used the GENEActiv Original actigraphy device (ActivInsights Ltd., Cambridge, UK), which measures tri-axial acceleration with an MEMS sensor (range: ± 8 g; res: 12bit), light level with a silicon photodiode (res: 5 Lux typical), and temperature with a linear active thermister (res: 0.25 °C). Participants were asked to wear the device on their dominant wrist 24 h per day for 1 week: which is the battery-life and data storage capacity of the device when set to record at a sampling frequency of 100 Hz.
Wearable devices were charged before being distributed to families either in-person or via mail. Study staff directed families through device placement and device activation. After 1 week of continuous wear, devices were mailed back and participant data were downloaded off of each device by study staff. Following data collection, study staff engaged in a guided interview with children and parents to discuss their experience with the wearable sensor. In four (13.3%) out of the 30 children who wore the device for 1 week, data were obtained for fewer than 7 days (3–6 days) due to battery charging issues.
Computer Mouse Task Data Collection
A browser-based task (Hevelius) hosted by Labinthewild.org[28] was in this study. Hevelius quantifies arm function by extracting 32 age-normalized features from continuous, target-driven computer mouse trajectories. These features reflect the duration, speed, smoothness, and shape of the mouse movements and were previously shown to be informative for classifying and quantifying the severity of individuals with ataxia and parkinsonism in a clinic setting [19].
Participants were asked to complete the Hevelius computer mouse task once a week for up to 12 weeks at home. Individuals also completed the task once during the in-person clinical assessment. In total, 27 of the 30 participants (14 A-T, 13 controls) completed the task at least once and these 27 individuals performed the task on average 8.4 times with a range of 5.25–11 times (25th–75th percentile). Hevelius features were computed from each session and the median feature values across each individual’s sessions were used in analysis.
Clinical Assessment
All A-T participants completed a detailed, in-person neurological exam and were scored on the Brief Ataxia Rating Scale (BARS), which evaluates gait, speech, oculomotor function, the finger-nose-finger task, and the heel-to-shin task[26]. Participants wore the wearable sensor on their dominant wrist as they completed these clinical tasks. Twelve clinical assessments were performed within 1 month of the start of wearable sensor data collection and all assessments were performed within 4 months of data collection.
Total BARS scores and subscores assigned to each A-T participant are shown in Table 1. For the two individuals for whom it was challenging to clinically assess speech and the one individual who was not properly participating in the right leg assessment, the subscore was assigned by taking the normalized average score of the other components of their BARS score. All control participants were assigned BARS scores of 0.
In addition to BARS ataxia scores, the presence or absence of hyperkinetic movements (i.e., tics, chorea, myoclonus, ballismus, and arrhythmic adventitious movements) and tremor/rhythmic movements, as defined in the A-T Neuro Examination Scale Toolkit (A-T NEST)[29], were retrospectively assessed from videos of the neurological examination (Table 1).
Wearable Sensor Data Processing and Feature Extraction
Each participant’s raw wearable sensor data were partitioned into day and night segments based on clear changes in each child’s daily activity level represented in the accelerometer data (see Fig. 1A). To account for differences in the time of day that sensor recording began across participants, day/night segmentation was started at the onset of the first full night of recording. This produced a maximum of 6 consecutive 24-h periods of recording from a full week of data collection. Data analysis focused on daytime activity given that the majority of night-time was determined to be spent in an inactive state (91.9 ± 4.5% for children with A-T and 92.1 ± 3.1% for controls) as measured by Activity Index (see below).
Sample of raw acceleration data and Activity Index (AI) histogram for A-T participants and controls. A–B Tri-axial acceleration and light measurements from a sample participant over 24 h. Dashed lines separate nighttime and daytime segments. C Histograms show the proportion of daytime participants spent at each AI (group medians shown). X-axis plotted in cubic scale. Dashed lines denote the upper bound of AI categories defined as inactive (AI < 0.0045), low (0.0045–8.63), moderate (8.63–44.8), and high (44.8–336)
Activity Index Feature Extraction
Relative scale Activity Index (AI) is a non-proprietary measure of activity intensity derived from tri-axial accelerometry data that has been shown to correlate with energy expenditure and differentiate between activity types[30]. Instead of measuring the magnitude of raw acceleration, the AI metric is based on the variance of acceleration in each of the three axes of motion. AI was computed for each 1-s period of sensor recording during the free-living recording. Visualizing the histogram of daytime AI values for A-T and control participants on a cubically scaled axis (Fig. 1C) demonstrated a large, discontinuous peak in the first bin representing very small AI values (AI < 0.0045). In the night-time AI histogram of both A-T and control participants (not shown), this first bin contained > 90% of all AI values. Thus, AI values less than 0.0045 were categorized as “inactive.” Percent of daytime spent inactive was used as a sensor-derived metric in analysis. When computing statistics of the daytime AI distribution for each individual, periods of inactivity were excluded. Two sensor-derived metrics, mean AI and AI entropy, were extracted from this resultant AI distribution. AI entropy is highest when time is spent equally over all possible activity indices and lowest when all time is spent at a single Activity Index value.
Based on prior work, AI values were grouped into three categories: low intensity (0.0045 < AI < 8.63; e.g., watching a DVD while sitting quietly), moderate intensity (8.63 < AI < 44.8; e.g., doing laundry while standing), and high intensity (44.8 < AI < 336; e.g., treadmill walking at 2–2.5 miles per hour)[30]. These categories were used to determine the percentage of daytime each individual spent performing low, moderate, and high intensity activities.
Activity Index During In-person Clinical Assessments
To verify these categories in the A-T population and with a wrist sensor, AI was computed from recordings obtained during performance of the standard neurological examination. For each clinical task performed by an A-T participant, the 90th percentile AI value was used as a representation of the task activity intensity value during that specific task. 90th percentile was used to account for periods surrounding performance of the task when individuals were not yet engaged in the task (see Table 2).
Total Power Feature Extraction
In order to calculate the metric of total power, we first computed the magnitude of the tri-axial acceleration data for each time point during daytime. Data were filtered using a 6th-order Butterworth bandpass filter with a 0.1-Hz lower cutoff frequency and a 20-Hz higher cutoff frequency. The discrete Fourier transform was subsequently performed on each participant’s data using a fast Fourier transform algorithm. Power spectra were computed for the frequency range of 0.1–5 Hz since this band had previously been found to be informative in cerebellar ataxia[31]. Results were qualitatively unchanged when selecting the broader frequency band 0.1–20 Hz. Total power was computed by taking the sum of power across the frequency band 0.1–5 Hz for all days of data collection and dividing by the number of 24-h data collection periods. The total power metric captures the proportion of acceleration signal power that falls in the 0.1–5-Hz frequency band.
Statistical Analyses
All statistical analyses were completed in MATLAB. The Mann–Whitney U-test and Cohen’s d effect size were used for group comparisons between A-T and control groups. Pearson correlation coefficients and p-values were employed to evaluate the relationship between wearable sensor measures and age, clinical scores, and computer task measures. Pearson correlation coefficients and p-values were also used to evaluate the reliability of the wearable sensor measures over the recording period (i.e., comparing data from days 1–3 versus days 4–6). P-values less than 0.05 were considered significant.
Results
Free-Living Measures Distinguish A-T vs Control Participants
Activity Index (AI) values were computed for each 1-s interval over all days of data collection for children with A-T and were compared with age- and sex-matched controls. Visual inspection of the AI histograms (Fig. 1C) suggested that children with A-T spent more time performing lower intensity behaviors (histogram shifted to left) and that there was a reduced range of activity intensity (histogram more peaked).
To further quantify activity differences between A-T and controls, AI was divided into four categories based on prior work relating AI values to specific behaviors[30] (see “Methods” section): inactive, low intensity, moderate intensity, and high intensity. Children with A-T spent 17.3 ± 11.1% (mean ± 1 standard deviation) of their day inactive, 50.8 ± 8.1% of their day performing low intensity behaviors, 29.2 ± 11.8% time performing moderate intensity behaviors, and 2.6 ± 2.0% time performing high intensity behaviors (Fig. 2).
Differentiating A-T versus controls based on daytime activity. A–D Proportion of daytime spent in each Activity Index (AI) category; E mean AI; F entropy of AI; and G total power (0.1–5 Hz) in A-T versus control groups. Levels of significance are indicated: p < 0.05(*); p < 0.01(**); p < 0.001(***); p < 0.0001(****)
Compared with controls, children with A-T spent more daytime performing low intensity behaviors (Mann–Whitney U-test, p < 0.01; Cohen’s d = 1.48) and less time performing high intensity behaviors (p < 0.001, Cohen’s d = 1.71). Mean AI was decreased in children with A-T versus controls (p < 0.001, Cohen’s d = 1.88) and total power in the 0.1–5-Hz range was also decreased in children with A-T (p < 0.0001, Cohen’s d = 2.02). There were no significant differences between children with A-T and controls for amount of time inactive and amount of time performing moderate intensity behaviors (Fig. 2).
To quantify the range of activity intensity in children with A-T, the entropy of the Activity Index histogram was computed for each participant. Entropy was significantly reduced in children with A-T compared with controls, with this metric carrying the highest significance level and effect size (p < 0.0001, Cohen’s d = 2.72, Fig. 2). Thus, individuals with A-T produced a reduced range of activity indices compared with controls.
The remainder of the analyses investigated the properties of the four metrics with the largest difference between children with A-T and controls: percent high intensity, mean AI, entropy of AI, and total power.
Correlation with Neurological Severity
All four metrics were compared with physician scores on the Brief Ataxia Rating Scale (BARS). As shown in Fig. 3, the four metrics correlated strongly and significantly with total BARS score, the BARS gait subscore, and the BARS score of the dominant arm, with Pearson correlations ranging in magnitude 0.64–0.78 for A-T participants only (p < 0.01) and increasing to 0.71–0.87 when considering A-T and controls together (Fig. 3). As anticipated, there were no significant correlations between the four metrics and speech, oculomotor, and leg subscores on BARS (data not shown). AI entropy demonstrated the highest correlations with BARS scores (absolute Pearson r of 0.85–0.87).
Reliability of Wearable Sensor Measures
Metric reliability was evaluated by splitting the data for each participant with 6 days of data (N = 26) into two periods (days 1–3 and days 4–6) and computing each of the four metrics separately for the two periods. The Pearson correlation for each metric across the two periods was highly significant (p < 0.00001) and ranged from 0.86 to 0.92, demonstrating high reliability (Fig. 4).
Reliability of wearable sensor metrics. A–D The 4 wearable sensor measures were computed from the first half (days 1–3) and the second half (days 4–6) of the data collection period per participant (A-T: red; controls: green) and then plotted against each other. The black dashed line represents the y = x line. Pearson correlation coefficients (r) and p-values are shown
Influence of Age on Wearable Sensor Measures
As there was a relationship between neurological disease severity (as measured by BARS) and age (Fig. 5E), it was anticipated that there would be a relationship between the wearable sensor metrics and age for the A-T population and, indeed, the Pearson r between each metric and age ranged from − 0.59 to − 0.72. In order to determine if the four wearable sensor measures were related to age without the confounding factor of disease progression, relationship with age was examined separately in the control population. Percent high intensity and mean AI were significantly correlated with age amongst controls (Pearson r = − 0.60 and − 0.59, respectively, p < 0.05), but entropy of AI and total power were not significantly correlated with age in control participants (Fig. 5).
Relationship between wearable sensor metrics and age. A–D The four activity metrics of interest were plotted against participant age with A-T (red) and control (green) participants shown separately. Linear regression line through each group is shown and listed Pearson correlation coefficients (r) and p-values are based on control group data only. E Total BARS score in the A-T group is plotted with respect to age and the corresponding regression line is shown
Relationship with Task-Based Digital Measures
To contextualize the four measures based on passively recorded movement from a wrist-worn sensor, we compared the measures with movement features on the Hevelius computer mouse task[19]. A consistent set of Hevelius features (duration of longest pause, number of pauses, movement time, and click duration) correlated most strongly (Pearson r 0.67–0.92) and significantly (p < 0.01) with the four wearable sensor measures (Fig. 6). These four Hevelius features were all previously found to be significantly increased in individuals with ataxia, and movement time and click duration were important features for estimating ataxia severity[19]. On the other hand, Hevelius features including fraction of the distance to the target center covered in the main submovement and click slip, which were previously determined to be informative for estimating ataxia severity, were not significantly correlated with the wearable sensor measures (data not shown).
Relationship between wearable sensor measures and Hevelius computer task features. Activity measures were compared with computer task features A–D longest pause, E–H number of pauses, I–L movement time, and M–P click duration. Feature values are medians across A-T participant weekly sessions. Line of best fit, Pearson r, and p-values are shown
Activity Measures During Clinical Tasks
To further contextualize the activity intensity values computed during free-living behavior, we computed AI from the wrist sensor data as the same participants with A-T performed several in-person neurological assessments. The median, 10th, and 90th percentile of maximum activity intensity across A-T participants for each clinical task are shown in Table 1. Clinical tasks involving speech and eye movements fell into the low intensity category (0.0045–8.63), tasks involving maintaining a posture or reaching fell into the moderate intensity category (8.63–44.8), and tasks involving fast repetitive arm movements fell into the high intensity category (44.8–336). Walking fell into the moderate intensity category for some individuals and the high intensity category for others since many children with A-T required assistance during the walking task.
Discussion
This study tested the hypothesis that motor activity metrics derived from 1 week of real-life wrist sensor data could accurately represent neurological disease state and severity in ataxia-telangiectasia. We found that children with A-T were inactive the same proportion of each day as healthy controls but were more likely to produce low intensity movements and less likely to perform high intensity movements compared to controls. In particular, the entropy of Activity Index values and total power were reduced in A-T and had properties supporting their potential as a biomarker: they distinguished children with A-T from controls with very large effect size, correlated strongly with clinical neurological severity, demonstrated high reliability, and were not significantly influenced by age in the healthy control population aged 4–15.
This study builds on prior work demonstrating that a wrist sensor can characterize hyperkinetic movements during specific motor tasks in A-T[17], can classify children with ataxia from controls during a reaching task[20], and that a three sensor array (feet and lumbar placement) can capture specific gait characteristics that correlate with severity during real-life ambulation in adult degenerative ataxias[21]. The current study differs from prior work in several ways with the goal of evaluating the potential for low-burden clinical application in A-T: (1) a single wrist-worn sensor was used, (2) natural (task-free) behavior was measured, (3) there were no functional restrictions on participation, such as requiring individuals to be able to ambulate without aids, and (4) key properties supporting the metrics’ use as a biomarker were evaluated. This work extends our knowledge of the disease information captured by a single wrist sensor during natural behavior and provides support for its use in measuring neurological severity in A-T clinical trials.
One challenge with passively collected data in real-world settings where context is unknown and uncontrolled is finding measures that are both informative and interpretable. Activity Index was used to represent activity intensity in each 1-s epoch of behavior as prior work established that this measure is sensitive for detecting sedentary, light, moderate, and vigorous physical activities and correlates strongly with energy expenditure, based on a sensor placed at the hip[30]. Furthermore, AI was computed as A-T participants performed standard neurological examination tasks while wearing the sensor on their dominant wrist. This enabled a mapping between activity intensities produced in the free-living setting with motor tasks performed during standard neurological assessments.
The daily distribution of AI measures is likely driven by several factors including the specific real-life behaviors performed by the individual, the intensity with which the behaviors were performed, and in A-T, the individual’s hyperkinetic and hypokinetic movement features. The A-T phenotype is known to include arrhythmic hyperkinetic movements (e.g., chorea, myoclonus, dystonia), rhythmic hyperkinetic movements (i.e., tremor), and bradykinesia[1]. Despite the presence of arrhythmic hyperkinetic movements in the limbs of all but one A-T participant, the A-T group produced more low intensity movements compared with the control group. This observation suggests that arrhythmic hyperkinetic movements have a relatively small impact on the daily distribution of AI, potentially because they make up a relatively small proportion of overall daily activity and/or are outweighed by other factors (i.e., the choices of behaviors and how they are performed). Only two A-T participants (both in the 12–18-year-old group) had a rhythmic limb tremor. As shown in Fig. 5, all individuals with A-T in this older age group had markedly reduced mean AI; thus, rhythmic hyperkinetic movements, similar to arrhythmic movements, appear to be outweighed by the other factors that contribute to daily activity intensity.
Entropy of the distribution of activity intensities experienced over a period of time also offers interpretable data for how A-T affects motor function. The measure reflects how evenly distributed a participant’s activities are over the range of activity intensities. In individuals with A-T, entropy of this distribution was markedly decreased, indicating that activities were concentrated in a narrower range of intensity levels compared to controls. Decreased entropy is a consequence of neurological disease that has been observed across different diagnoses and data types[32,33,34,35].
Entropy of AI and total power correlated strongly with total BARS score, BARS dominant arm finger-nose-finger task score, and BARS gait score, but not speech, oculomotor, or heel-to-shin subscores. These observations are consistent with the intuition that wrist-sensor based movement measures reflect gait and arm function. Additionally, strong correlations between the wearable sensor measures and certain Hevelius computer mouse task features related to task duration and pausing behavior suggest that the wearable sensor metrics reflect slowed and interrupted motor task performance in ataxia-telangiectasia. Thus, it is possible that the wearable sensor metrics described here may be useful in assessments in other neurological conditions such as Parkinson disease.
The data required to compute the wearable sensor measures were easy to collect and low-burden on children, as only a single lightweight wrist sensor was needed and individuals were not required to perform any specific tasks. The single-sensor system was well-tolerated for a full week’s use in the majority of the pediatric cohort based on feedback from parents and children (see “Methods” section). Importantly, the measures could be computed from individuals who could not ambulate independently or were wheelchair-bound, and remained reflective of disease severity.
The low-cost wearable sensor-derived biomarkers reported here offer clinicians and researchers a mechanism to collect real-life, disease-relevant information, without requiring trips to the office and precise performance of behavioral tasks, which can be a challenge to obtain in children. Continuous data collection enables the generation of more frequent severity estimates based on multiple days of data rather than a single visit, which may reduce variance in disease estimates and sample sizes in clinical trials[4]. Additionally, continuous data collection provides the opportunity to characterize diurnal and day-to-day fluctuations, as well as evaluate the effects of variables such as mood, fatigue, and sleep on motor activity in A-T.
These data demonstrated that children with A-T produced lower intensity movements; however, since the data were collected in the free-living context, the precise nature of the activities and behaviors remains unknown. Children with A-T could either be engaging in the same activities as healthy controls but less vigorously or they could be engaging more often in different activities that are less strenuous. The answer may be a combination of the two scenarios, but further investigation is needed for a more granular understanding of activities performed in the free-living context. In addition, AI and its related metrics and categories have thus far been developed in the context of adult behaviors not pediatric behaviors and based on a hip-worn rather than a wrist-worn sensor. Measuring AI from a wrist-sensor in children with A-T as they participated in neurological assessments (Table 2) provided additional support and intuition for evaluating AI in the free-living context; however, there is a need to further evaluate these activity metrics in a broader set of pediatric and adult populations. Furthermore, it will be important to examine clinical scoring scales other than the BARS, which specifically captures ataxia, in order to investigate the biomarker’s potential applicability to other neurological phenotypes present in A-T.
References
Rothblum-Oviatt C, Wright J, Lefton-Greif MA, McGrath-Morrow SA, Crawford TO, Lederman HM. Ataxia telangiectasia: a review. Orphanet J Rare Dis. 2016;11(1):159. https://doi.org/10.1186/s13023-016-0543-7.
Lavin MF, Gueven N, Bottle S, Gatti RA. Current and potential therapeutic strategies for the treatment of ataxia-telangiectasia. Br Med Bull. 2007;81–82(1):129–47. https://doi.org/10.1093/bmb/ldm012.
Nissenkorn A, Ben-Zeev B. Ataxia telangiectasia. In: Handbook of clinical neurology. Vol 132. Elsevier; 2015:199–214. https://doi.org/10.1016/B978-0-444-62702-5.00014-7.
Dodge HH, Zhu J, Mattek NC, Austin D, Kornfeld J, Kaye JA. Use of high-frequency in-home monitoring data may reduce sample sizes needed in clinical trials. PLoS ONE. 2015;10(9): e0138095. https://doi.org/10.1371/journal.pone.0138095.
Bove R, White CC, Giovannoni G, et al. Evaluating more naturalistic outcome measures: a 1-year smartphone study in multiple sclerosis. Neurol Neuroimmunol Neuroinflammation. 2015;2(6): e162. https://doi.org/10.1212/NXI.0000000000000162.
Berry JD, Paganoni S, Carlson K, et al. Design and results of a smartphone-based digital phenotyping study to quantify ALS progression. Ann Clin Transl Neurol. 2019;6(5):873–81. https://doi.org/10.1002/acn3.770.
Rutkove SB, Narayanaswami P, Berisha V, et al. Improved ALS clinical trials through frequent at-home self-assessment: a proof of concept study. Ann Clin Transl Neurol. 2020;7(7):1148–57. https://doi.org/10.1002/acn3.51096.
Erb MK, Karlin DR, Ho BK, et al. mHealth and wearable technology should replace motor diaries to track motor fluctuations in Parkinson’s disease. Npj Digit Med. 2020;3(1):6. https://doi.org/10.1038/s41746-019-0214-x.
Chitnis T, Glanz BI, Gonzalez C, et al. Quantifying neurologic disease using biosensor measurements in-clinic and in free-living settings in multiple sclerosis. Npj Digit Med. 2019;2(1):123. https://doi.org/10.1038/s41746-019-0197-7.
Gordon MF, Grachev ID, Mazeh I, et al. Quantification of motor function in Huntington disease patients using wearable sensor devices. Digit Biomark. 2019;3(3):103–15. https://doi.org/10.1159/000502136.
Zampogna A, Mileti I, Palermo E, et al. Fifteen years of wireless sensors for balance assessment in neurological disorders. Sensors. 2020;20(11). https://doi.org/10.3390/s20113247.
Hansen C, Beckbauer M, Romijnders R, et al. Reliability of IMU-derived static balance parameters in neurological diseases. Int J Environ Res Public Health. 2021;18(7). https://doi.org/10.3390/ijerph18073644.
Geronimo A, Martin AE, Simmons Z. Inertial sensing of step kinematics in ambulatory patients with ALS and related motor neuron diseases. J Med Eng Technol. 2021:1–8. https://doi.org/10.1080/03091902.2021.1922526.
Muurling M, de Boer C, Kozak R, et al. Remote monitoring technologies in Alzheimer’s disease: design of the RADAR-AD study. Alzheimers Res Ther. 2021;13(1):89. https://doi.org/10.1186/s13195-021-00825-4.
Au-Yeung W-TM, Miller L, Beattie Z, et al. Monitoring behaviors of patients with late-stage dementia using passive environmental sensing approaches: a case series. Am J Geriatr Psychiatry Off J Am Assoc Geriatr Psychiatry. 2021. https://doi.org/10.1016/j.jagp.2021.04.008.
Gonçalves HR, Rodrigues AM, Santos CP. Vibrotactile biofeedback devices in Parkinson’s disease: a narrative review. Med Biol Eng Comput. 2021. https://doi.org/10.1007/s11517-021-02365-3.
Shaikh AG, Zee DS, Mandir AS, Lederman HM, Crawford TO. Disorders of upper limb movements in ataxia-telangiectasia. Maurits NM, ed. PLoS One. 2013;8(6):e67042. https://doi.org/10.1371/journal.pone.0067042.
Oubre B, Daneault J-F, Whritenour K, et al. Decomposition of reaching movements enables detection and measurement of ataxia. The Cerebellum. 2021. https://doi.org/10.1007/s12311-021-01247-6.
Gajos KZ, Reinecke K, Donovan M, et al. Computer mouse use captures ataxia and parkinsonism, enabling accurate measurement and detection. Mov Disord. 2020;35(2):354–8. https://doi.org/10.1002/mds.27915.
Martinez-Manzanera O, Lawerman TF, Blok HJ, et al. Instrumented finger-to-nose test classification in children with ataxia or developmental coordination disorder and controls. Clin Biomech. 2018;60:51–9. https://doi.org/10.1016/j.clinbiomech.2018.10.007.
Ilg W, Seemann J, Giese M, et al. Real-life gait assessment in degenerative cerebellar ataxia: toward ecologically valid biomarkers. Neurology. 2020;95(9):e1199–210. https://doi.org/10.1212/WNL.0000000000010176.
LeMoyne R, Heerinckx F, Aranca T, De Jager R, Zesiewicz T, Saal HJ. Wearable body and wireless inertial sensors for machine learning classification of gait for people with Friedreich’s ataxia. In: 2016 IEEE 13th International Conference on Wearable and Implantable Body Sensor Networks (BSN). IEEE; 2016:147–151. https://doi.org/10.1109/BSN.2016.7516249.
Terayama K, Sakakibara R, Ogawa A. Wearable gait sensors to measure ataxia due to spinocerebellar degeneration. Neurol Clin Neurosci. 2018;6(1):9–12. https://doi.org/10.1111/ncn3.12174.
Hickey A, Gunn E, Alcock L, et al. Validity of a wearable accelerometer to quantify gait in spinocerebellar ataxia type 6. Physiol Meas. 2016;37(11):N105–17. https://doi.org/10.1088/0967-3334/37/11/N105.
Crawford TO. Ataxia telangiectasia. Semin Pediatr Neurol. 1998;5(4):287–94. https://doi.org/10.1016/s1071-9091(98)80007-7.
Schmahmann JD, Gardner R, MacMore J, Vangel MG. Development of a brief ataxia rating scale (BARS) based on a modified form of the ICARS: brief ataxia rating scale. Mov Disord. 2009;24(12):1820–8. https://doi.org/10.1002/mds.22681.
Perez‐Lloret S, van de Warrenburg B, Rossi M, et al. Assessment of ataxia rating scales and cerebellar functional tests: critique and recommendations. Mov Disord. 2020:mds.28313. https://doi.org/10.1002/mds.28313.
Reinecke K, Gajos KZ. LabintheWild: conducting large-scale online experiments with uncompensated samples. In: Proceedings of the 18th ACM Conference on Computer Supported Cooperative Work & Social Computing. CSCW ’15. Association for Computing Machinery; 2015:1364–1378. https://doi.org/10.1145/2675133.2675246.
Jackson TJ, Chow G, Suri M, Byrd P, Taylor MR, Whitehouse WP. Longitudinal analysis of the neurological features of ataxia-telangiectasia. Dev Med Child Neurol. 2016;58(7):690–7. https://doi.org/10.1111/dmcn.13052.
Bai J, Di C, Xiao L, et al. An Activity Index for raw accelerometry data and its comparison with other activity metrics. Harezlak J, ed. Plos One. 2016;11(8):e0160644. https://doi.org/10.1371/journal.pone.0160644.
Krishna R, Pathirana PN, Horne M, Power L, Szmulewicz DJ. Quantitative assessment of cerebellar ataxia, through automated limb functional tests. J NeuroEngineering Rehabil. 2019;16(1):31. https://doi.org/10.1186/s12984-019-0490-3.
Azami H, Arnold SE, Sanei S, et al. Multiscale fluctuation-based dispersion entropy and its applications to neurological diseases. IEEE Access. 2019;7:68718–33. https://doi.org/10.1109/ACCESS.2019.2918560.
Pasluosta C, Hannink J, Gaßner H, et al. Motor output complexity in Parkinson’s disease during quiet standing and walking: analysis of short-term correlations using the entropic half-life. Hum Mov Sci. 2018;58:185–94. https://doi.org/10.1016/j.humov.2018.02.005.
Flood MW, Jensen BR, Malling A-S, Lowery MM. Increased EMG intermuscular coherence and reduced signal complexity in Parkinson’s disease. Clin Neurophysiol. 2019;130(2):259–69. https://doi.org/10.1016/j.clinph.2018.10.023.
Zheng H, Onoda K, Nagai A, Yamaguchi S. Reduced dynamic complexity of BOLD signals differentiates mild cognitive impairment from normal aging. Front Aging Neurosci. 2020;12. https://doi.org/10.3389/fnagi.2020.00090.
Williams K, Thomson D, Seto I, et al. Standard 6: age groups for pediatric trials. Pediatrics. 2012;129(Supplement 3):S153–60. https://doi.org/10.1542/peds.2012-0055I.
Acknowledgements
The authors thank Jennifer Thornton and Sara Reiling of the A-T Children’s Project for support throughout the study, Jessey Ouillon and Winnie Ching for assistance with data collection, Karin Knudson for insights in data analysis, and Brandon Oubre and Sunghoon Ivan Lee for helpful conversations. The authors also thank the children and families who collaborated with us on this project.
Funding
VP is funded in part by Biogen Inc. KZG receives research funding from Biogen Inc. and Sony. ASG is a consultant for Biogen Inc., Triplet Therapeutics, and Remix Therapeutics and is funded by the Ataxia-Telangiectasia Children’s Project, Biogen Inc., the Massachusetts Life Sciences Center, and the University of Pennsylvania Orphan Disease Center. NCK has no disclosures to report.
Author information
Authors and Affiliations
Contributions
NCK and ASG conceptualized and designed the study. NCK, VP, and ASG contributed to the acquisition of data. NCK, VP, KZG, and ASG analyzed the data. NCK and ASG drafted the manuscript. NCK, VP, KZG, and ASG revised the manuscript for intellectual content.
Corresponding author
Ethics declarations
Ethics Approval
Written informed consent and assent were obtained from all participants prior to participation and the study was approved by the Partners Healthcare Research Committee Institutional Review Board (No. 2019P002752).
Conflict of Interest
The authors declare no competing interests.
Additional information
Publisher’s Note
Springer Nature remains neutral with regard to jurisdictional claims in published maps and institutional affiliations.
Rights and permissions
About this article
Cite this article
Khan, N.C., Pandey, V., Gajos, K.Z. et al. Free-Living Motor Activity Monitoring in Ataxia-Telangiectasia. Cerebellum 21, 368–379 (2022). https://doi.org/10.1007/s12311-021-01306-y
Accepted:
Published:
Issue Date:
DOI: https://doi.org/10.1007/s12311-021-01306-y