Abstract
In educational research, the process of socioemotional adaptation is often examined using panel studies with repeated assessments across months or years. Intensive longitudinal designs (e.g., daily diaries or experience sampling methods) allow to target this process on a shorter time scale (e.g., from day to day or week to week). In this contribution, we demonstrate that these two approaches yield similar but not interchangeable information about the process of socioemotional adaptation in university students. Results of an intensive longitudinal study with 250 university students revealed (a) mean level differences in reported study satisfaction depending on the time frame of the assessment, (b) differential change in study satisfaction for daily vs. weekly ratings across seven weeks in one semester, and (c) the prognostic utility of repeated weekly assessments of study satisfaction for retrospective assessments of study satisfaction. Findings illustrate the benefit of combining panel studies with intensive longitudinal studies. Implications for the assessment of experiences, memories of experiences, and global self-beliefs are discussed.
Zusammenfassung
In der Bildungsforschung wird der Prozess sozioemotionaler Anpassung häufig über Panel-Studien mit wiederholten Messungen über Monate und Jahre untersucht. Intensive Längsschnittdesigns wie Tagebuchstudien oder experience sampling Studien erlauben, diesen Prozess auf einer zeitlich kürzeren Ebene abzubilden. In diesem Beitrag zeigen wir anhand eines empirischen Beispiels, dass diese beiden Ansätze ähnliche, aber nicht redundante Informationen zum Prozess sozioemotionaler Anpassung bei Studierenden liefern. Ergebnisse aus einer intensiv-längsschnittlichen Studie mit 250 Studierenden zeigten (a) Mittelwertsunterschiede in berichteter Studienzufriedenheit in Abhängigkeit der zeitlichen Auflösung der Erfassung, (b) differenzielle Veränderung in Studienzufriedenheit über sieben Wochen in einem Semester, und (c) die prognostische Validität von wiederholten, wöchentlichen Messungen der Studienzufriedenheit bei der Vorhersage von retrospektiver Studienzufriedenheit. Die Ergebnisse zeigen den Nutzen der Kombination von Panel-Studien mit intensiven Längsschnittdesigns auf. Implikationen für die Erfassung von momentanem Erleben, erinnertem Erleben und Selbstüberzeugungen werden diskutiert.
Similar content being viewed by others
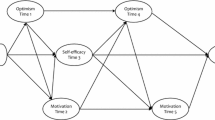
Avoid common mistakes on your manuscript.
1 Background
1.1 Socioemotional adaptation
Educational contexts are not only relevant for promoting academic development but they are also important contexts for socioemotional development across the life span. Adaptive and successful socioemotional development requires psychological adaptation to the educational context. Psychological adaptation (both inside and outside of educational contexts) occurs on multiple time scales, ranging from very brief, moment-to-moment fluctuations to long-term changes across months or years. Large panel studies such as the German National Educational Panel Study (NEPS; Blossfeld and Roßbach 2019) or the German Socio-Economic Panel Study (SOEP; Goebel et al. 2019) often spanning several years of assessment are excellently suited to capture long-term changes in adaptation processes and to illuminate consequences of better or worse socioemotional adjustment for outcomes such as educational attainment. In these studies, participants are typically asked about global retrospective assessments of, for instance, their study satisfaction and drop-out intentions (e.g., Scheunemann et al. 2021) or the extent to which they feel they belong to their educational setting (e.g., Menkor et al. 2021).
When it comes to understanding the mechanism of psychological adaptation, however, one particularly important aspect is to better understand the processes leading to higher or lower levels of socioemotional and academic adjustment. These processes are likely to occur in individuals’ daily lives and they can be expected to accumulate over weeks, months, or years before they manifest in global evaluations of socioemotional and academic adjustment. Intensive longitudinal studies (e.g., ecological momentary assessments or daily diary studies) allow to examine these processes in individuals’ everyday lives (Bolger et al. 2003; Mehl and Conner 2012; Trull and Ebner-Priemer 2013). In addition to examining average effects, intensive longitudinal data offer unique insights into between-person differences in psychological adaptation processes. Using modern data-analytic approaches such as multilevel modeling opens up possibilities to target the extent to which individuals differ in their within-person dynamics (Bolger et al. 2019; Bolger and Laurenceau 2013; Neubauer and Schmiedek 2020). For instance, while fulfillment of basic psychological needs on average is associated with higher levels of well-being, people differ in the size of this association (Neubauer et al. 2018, 2020a; Schmidt et al. 2020). Similarly, in an experience sampling study of university students, Ketonen et al. (2019) reported between-person heterogeneity in the within-person association between task value and affective well-being. This implies that the degree to which the perceived relevance of tasks impacts students’ well-being varies from student to student.
So far, panel studies and intensive longitudinal studies in educational contexts have often been implemented separately, one reason being that these designs often pursue different research goals: Panel studies focus on longitudinal change across longer time spans and do not target more short-term, within-person fluctuations in constructs, which would be regarded as noise. In contrast, intensive longitudinal studies treat the obtained data as snapshots of individuals’ daily lives and focus specifically on short-term, within-person fluctuations in a construct. In these studies, longitudinal change is typically not considered as the core focus. More than that, many data-analytic approaches for intensive longitudinal studies assume that these data are stationary, meaning that mean levels and other statistical moments are constant across time. Therefore, longitudinal change is not specifically targeted by intensive longitudinal studies, and if it is, it is treated as nuisance that needs to be statistically controlled for to satisfy the assumption of stationarity.
The combination of panel studies and intensive longitudinal studies into measurement burst designs is however a very powerful extension. In measurement burst designs, several bouts of intensive longitudinal assessment are repeated with the same individuals across weeks, months, or years (Sliwinski 2008). These designs allow not only to examine questions from both approaches in one design, but they also create synergies that are helpful in elucidating how everyday experiences (collected in intensive longitudinal designs) are transformed into global retrospective evaluations (assessed in panel studies) and how within-person dynamics might shape long-term developmental change (Neubauer et al. 2022).
These designs are ideally suited to examine central questions of human development and to examine how changes in short-term variation and long-term developmental change are interwoven (Nesselroade 1991). They can also contribute to trying to understand convergences and differences between repeatedly collected information in an intensive longitudinal design and retrospective/global evaluations of the same construct across a longer time frame (as is typically done in panel studies). For instance, previous research has begun to illuminate this question in the context of the assessment of well-being (Ganzach and Yaor 2019; Junghaenel et al. 2021; Neubauer et al. 2020b) and pain (e.g., Stone et al. 2010). Overall, these previous findings suggest that global retrospective ratings show some degree of divergence from aggregated momentary ratings collected via intensive longitudinal designs over the same time span. For instance, retrospective reports of negative affect are typically higher than aggregated momentary reports collected across the same time frame from the same individuals (Junghaenel et al. 2021; Neubauer et al. 2020b)—a phenomenon that has been termed the memory-experience gap (Miron-Shatz et al. 2009). For positive affect, findings are mixed but some studies also report a memory-experience gap for positive affective states (Lay et al. 2017; Neubauer et al. 2020b). Prior research on the memory-experience gap suggested various mechanisms that may account for the discrepancy between retrospective assessments and aggregated momentary assessments. One proposed explanation are peak-and-end effects (Fredrickson 2000; Redelmeier et al. 2003): According to this account, the maximum of affective experiences and the most recent affective experience are particularly salient and therefore bias retrospective evaluations away from the mean of the momentary experiences towards peak and end experiences. Emotion regulation processes, in particular reappraisal, have been suggested as an additional explanation for why momentary experiences are to some extent de-coupled from recollection of these experiences (Neubauer et al. 2020b).
The extent to which such divergences are also observed for ratings of study satisfaction is unknown so far. Better understanding how retrospective accounts of study satisfaction (which are typically assessed in panel studies such as NEPS) are related to students’ everyday experiences is, however, highly relevant because such knowledge can serve to better understand to what extent satisfaction ratings obtained in panel studies can be useful to predict students’ future behavior (such as successful degree completion or study drop out) and to identify potential targets of interventions improving study satisfaction.
1.2 The present study
In the present work, we target the extent to which ratings of one central dimension of psychological adaptation in tertiary education—study satisfaction (Westermann et al. 1996)—differ depending on the way they are assessed. To that end, we use data from a longitudinal study that targeted the transition into tertiary education during the Covid-19 pandemic. This study included both a panel design element (with measurements repeated every three months) and two intensive longitudinal elements: weekly retrospective assessments at the end of each week in the semester and an experience sampling part with six daily assessments for a total duration of 14 days across the semester. The present work addresses three central questions regarding the convergence between different assessment types of study satisfaction: First, are there mean level differences between aggregated daily assessments, short-term retrospective evaluations (across one week), and more global retrospective evaluations? Second, does study satisfaction change across seven weeks in one semester and does the rate of change differ for retrospective evaluations and aggregated daily assessments? And third, do weekly retrospective evaluations and aggregated daily assessments both uniquely predict more global retrospective evaluations at the end of the semester?
Prior work suggests that divergences may be larger for negative experiences and we approach these questions using both indicators of positive experiences (satisfaction with study content), and negative experiences (perceived study demands). We did not test specific a‑priori hypotheses and treated these questions as more exploratory in nature.
2 Method
The present analyses use data from a study examining the transition to tertiary education during the Covid-19 pandemic in Germany. This study consisted of three parts: Part 1 was a longitudinal panel design in which participants completed five online questionnaires between March/April 2021 and March/April 2022. Part 2 was an intensive longitudinal study with 15 weekly assessments in the summer semester 2021 (April—July 2021) and 15 weekly assessments in the winter semester 2021/2022 (October 2021—February 2022). Part 3 was an experience sampling study with six daily assessments across 14 days in the summer semester 2021.
In this contribution, we use data from two waves of data collection in study part 1, the weekly assessments in the summer semester in part 2, and the experience sampling data from part 3. Only the relevant information for these analyses is reported below. All data from this project are publicly available (https://osf.io/bhq3p/; Neubauer et al. 2021) and were accessed from this repository for the present analyses. In the OSF repository accompanying the present work (https://osf.io/84kdr/), we further provide the subset of the publicly available data used for analyses and the analysis code necessary to reproduce the results reported in this work.
2.1 Participants and procedure
Participants were recruited by contacting university administrations and student bodies at different universities across Germany. The study was advertised for first year students who had started tertiary education in fall 2020 or spring 2021 and who had obtained their university entrance qualification in 2019 or 2020. Recruitment started in March 2021 and participants could sign up for the study by April 11. Upon registration, participants could choose to take part in study parts 1 and 2 only, or sign up for all three study parts. Overall, 374 young adults (at least partially) completed the baseline questionnaire (see study protocol for details: https://osf.io/2q4wz; Neubauer and Kramer 2022). For the present analyses, we included only those participants who had completed at least two weekly assessments (study part 2) and who provided data at the third measurement occasion in study part 1 (i.e., in the September assessment). This yielded a final sample size of N = 250 (Mage = 19.70, SDage = 2.06; 76% female); most participants (94.4%) were first-year students.
All data were collected via online questionnaires, implemented in SoSci Survey (Leiner 2019). The study started with a baseline questionnaire in study part 1, which could be completed by April 11. In this baseline questionnaire, various sociodemographic variables were assessed as was information on participants’ current university, financial situation, and various psychological trait measures. Participants received the third questionnaire in study part 1 on September 27. This questionnaire could be completed by October 20, and contained similar scales to the baseline assessment but also retrospective evaluations of participants’ study satisfaction. For the present analyses, only the study satisfaction measures collected in the September assessment are relevant.
In study part 2, all participants received the links to a weekly questionnaire for 15 weeks across the summer semester. These links were e‑mailed to all participants on Friday, 3 pm and could be completed by Sunday, 11:45 pm. Study part 2 started on April 17 2021 and ran for 15 consecutive weeks. In the weekly questionnaires, information on the past week regarding for instance workload, types of classes visited, and well-being were assessed. Relevant for the present analyses are only the retrospective evaluations of study satisfaction with respect to the past week.
Study part 3 was an experience sampling part in which participants received the links to short online questionnaires six times per day via text messages sent to their smartphones. The first five assessments per day were sent out at semi-randomized time intervals between 9 am and 7 pm. For the present analyses, only the last assessments of each day (end-of-day assessment) are relevant. The link to this questionnaire was distributed at 9 pm. Participants were instructed to complete this questionnaire before going to bed; the questionnaire was deactivated at 5 am. Participants received these six daily questionnaires in study part 3 for seven consecutive days, and then again for seven consecutive days after a break of six weeks. The design of this study part can therefore be understood as a measurement burst design (Sliwinski 2008) with two measurement bursts of seven consecutive days of experience sampling (see Fig. 1). The weeks at which the assessments were scheduled were randomized. After signing up for study part 3, participants were randomized into one of seven groups which differed only in the schedule of this study part: Group 1 started the ambulatory assessment phase in the first week (April 12—April 18) and started their second burst 7 weeks later (May 31—June 6). Group 2 started one week later (Burst 1: April 19—April 25; Burst 2: June 7—June 13), group 3 again one week later (Burst 1: April 26—May 2; Burst 2: June 14—June 20) and so on (see study protocol for further details). Owing to this procedure, study part 3 was distributed across 14 weeks of the summer semester. Hence, daily data were obtained for almost the complete time frame of one semester. By randomizing participants into different study weeks, participant burden was kept lower compared to an alternative design in which each participant would need to complete ratings for all 14 weeks. By having participants complete one assessment in the first part (weeks 1–7) and one assessment in the second part (weeks 8–14), data were available for each participant in the first half of the semester and in the second half of the semester respectively. Moreover, the two measurement bursts were seven weeks apart for all participants, and therefore, the timespan between the two measurement bursts was constant across participants. At each of the two bursts, we removed data from participants who provided fewer than two (out of seven) end-of-day assessments.
Overview of the Study Procedure. Blue: Study part 1 (Online Questionnaire). Ocher: Study part 2 (Weekly Online Questionnaire). Red: Study part 3 (6 Assessments per day for 7 consecutive days). Figure reproduced from Neubauer and Kramer (2022). Only assessments until September were used in the present research
2.2 Measures
For the present analyses, only the assessment of study satisfaction is relevant. Study satisfaction was measured using the two subscales “Satisfaction with Study Content” (3 items, e.g., “I really enjoy the subject I’m studying.”) and “Study Demands” (3 items, e.g., “My course of study is wearing me down.”) as published in Westermann et al. (2018). These two scales were assessed in all three study parts, and rated on a scale from 1 (“not at all true”) to 7 (“completely true”). Items assessing study demands were not recoded before aggregation, therefore high scores on the subscale study demands indicate low study satisfaction.
2.2.1 Study part 1
In the September assessment, participants were asked to rate each of the six items with respect to the past semester (“Looking back, how would you rate your studies during the past summer semester? Please indicate to what extent the following statements apply.”). Items were aggregated into a measure of satisfaction with study content (ω = 0.89), and a measure of study demands (ω = 0.79).
2.2.2 Study part 2
Each week, participants were asked to rate the six items with respect to their past week (“How would you rate your current studies? Please indicate to what extent the following statements apply. Think about the past week”). Reference to the past week was added to each item (e.g., “In the past week, I really enjoyed the subject I’m studying.”). For each week and each participant the items were aggregated into weekly satisfaction with study content and weekly study demands respectively. Internal consistencies were estimated as multilevel McDonald’s ω (Geldhof et al. 2014) and suggested adequate internal consistencies for satisfaction with study content (ωwithin = 0.82; ωbetween = 0.98) and study demands (ωwithin = 0.75; ωbetween = 0.90) respectively. There were a total of 2715 weekly data points available, corresponding to a compliance rate of 2715 / (250 * 15) = 72.4%.
2.2.3 Study part 3
In each end-of-day assessment, participants were presented with six items and asked to rate these items with respect to the current day (“How would you rate your current studies? Please indicate to what extent the following statements apply today.”). Items were aggregated per day and participant into a measure of daily satisfaction with study content (ωwithin = 0.83; ωbetween = 0.98) and a measure of daily study demands (ωwithin = 0.69; ωbetween = 0.88). Given the 250 participants in the full sample, 225 had signed up for study part 3 and nine of these participants were excluded from the analysis of the Burst 1 data because they provided fewer than two out of seven end-of-day assessments. The average compliance of the remaining 216 participants was 75.4%. For Burst 2, 53 participants were removed due to too few data points, and the compliance in the remaining sample amounted to 66.7%.
2.3 Data preparation and analyses
We first merged the data from the three study parts and created information on the relative timing of the assessments: Daily assessments were scheduled from Monday through Sunday and therefore also partially overlapped with the respective weekly assessments. We therefore created a timing variable for each daily assessment, representing whether this assessment occurred before or after the assessment in part 2 of the same week.
2.3.1 Mean level differences between aggregated daily assessments, aggregated weekly assessments, and global retrospective evaluations
In our first analyses, we compared mean levels of study satisfaction between the three assessment time points (daily, weekly, September). To that end, we first aggregated weekly and daily measures for each person j and time point t and then compared mean levels using multilevel models with the three repeated assessments (daily, weekly, September) nested within participants. Two dummy variables (weekly, sept) were included as fixed effects in this model (daily ratings were hence the reference category; see Eqs. 1 and 2).
The regression coefficient β1 captures the difference in means between weekly ratings and daily ratings, β2 represents the difference in means between ratings from the September assessment and the aggregated daily ratings. Pairwise comparisons of all three rating types are reported with p-values adjusted for multiple testing using the Tukey method (Kramer 1956; Tukey 1953).
2.3.2 Change in aggregated daily and weekly study satisfaction
Next, we compared change in study satisfaction across the two measurement bursts. To that end, we aggregated the daily assessments per person j and burst b, using only data that were collected the week before a particular participant completed the weekly assessment. This aggregation resulted in up to four repeated measures per participant: two measures at Burst 1 (aggregated daily ratings this week, retrospective rating this week) and two measures at Burst 2 (aggregated daily ratings this week, retrospective rating this week). A multilevel model was estimated, which included the main effects for burst (coded 0 = burst 1; 1 = burst 2), rating type (0 = daily; 1 = weekly), and starting week (variable “start”; continuous variable coding the study week at which participants’ first burst was scheduled, ranging from 0 = April 12—April 18 to 6 = May 24—May 30). Random effects for burst and rating type were estimated in this model. This model was used to evaluate whether study satisfaction changes across seven weeks in one semester (which would be captured by a main effect of the predictor “burst”), and whether aggregated daily ratings would on average differ from weekly ratings (which would be manifested in a main effect of the predictor “rating type”).
In the next model, the interactions among the three predictors burst, rating type, and starting week were added. We examined whether the two rating types (aggregated daily vs. weekly) would differ in their change across the two bursts (which would be captured by a burst × rating type interaction). Equations 3, 4, 5, 6 and 7 represent the model including the interactions:
2.3.3 Predicting retrospective study satisfaction from daily and weekly ratings
Finally, a multiple regression analysis was conducted in which the September rating was predicted by the aggregated weekly assessments and daily assessments. In the first set of models, we aggregated all daily and all weekly ratings obtained across the whole semester. In this approach, weekly ratings contain information about the complete semester, whereas daily ratings contain information only about two weeks for each participant. In the second set of models we therefore reduced the amount of data that was aggregated such that we used only those weekly ratings from the same weeks as the daily ratings, and only those daily ratings that were obtained before the weekly ratings.
3 Results
Table 1 depicts descriptive statistics of study satisfaction for all three assessment methods (daily and weekly data were aggregated across all measurement occasions; within-person correlations of daily and weekly ratings are reported in the lower diagonal). Results show high correlations among the three rating types, r ≥ 0.59 for all. Furthermore, satisfaction with study content was correlated negatively with study demands with r’s ranging from −0.36 to −0.48 for ratings obtained from the same assessment types. Intraclass correlations (ICCs) for the four time-varying variables ranged between 0.399 and 0.562, suggesting that between about 40% and 56% of the variance in daily (satisfaction with study content: ICC = 0.551; study demands: ICC = 0.426) and weekly ratings (satisfaction with study content: ICC = 0.562; study demands: ICC = 0.399) were attributable to between-person differences.
3.1 Mean level differences between aggregated daily assessments, aggregated weekly assessments, and global retrospective evaluations
For satisfaction with study content, daily ratings were lower compared to weekly ratings, b = −0.419, p < 0.001, dFootnote 1 = −0.33, and lower than retrospective assessments in the September assessment, b = −0.399, p < 0.001, d = −0.32. The average of the weekly ratings across the whole semester was not significantly different from the global retrospective ratings collected in September, b = 0.020, p = 0.943, d < 0.01.
For study demands, higher ratings were obtained for longer recall periods, with daily ratings lower than weekly ratings, b = −0.453, p < 0.001, d = −0.37, which in turn were lower than retrospective ratings obtained in September, b = −0.377, p < 0.001, d = −0.30. Daily ratings were also lower on average than September ratings, b = −0.829, p < 0.001, d = −0.66.
Overall, results revealed that the mean levels in aggregated daily assessments, aggregated weekly assessments, and global retrospective evaluations of study satisfaction differed. Aggregated daily ratings were on average lower compared to both weekly and retrospective ratings. For study demands (but not satisfaction with study content), the retrospective ratings collected in September were also higher than the average weekly ratings collected throughout the semester. Mean levels are depicted in Fig. 2.
3.2 Change in aggregated daily and weekly study satisfaction
Results of the multilevel models can be found in Table 2. For both dependent variables, there was a main effect for assessment type, indicating that participants reported higher weekly scores on satisfaction with study content, b = 0.382, p < 0.001, and study demands, b = 0.298, p < 0.001 than in their aggregated daily reports. Satisfaction with study content decreased, b = −0.254, p = 0.003, and perceived study demands increased, b = 0.305, p = 0.002, across the two bursts. There was no effect of when participants started with the assessments on average levels of either satisfaction with study content, b = −0.028, p = 0.496, or study demands, b = 0.051, p = 0.210.
When entering the interaction effects, there was only a very small increase in explained variance, ∆R2 < 1%. Consequently, the individual statistically significant regression coefficients need to be interpreted with caution. For satisfaction with study content, results suggest that participants who started assessments in the first week of the semester showed larger decreases in their weekly ratings compared to their daily ratings, b = −0.314, p = 0.047. For study demands, a similar pattern was obtained: participants who started assessments in the first week of the semester showed larger increases in their weekly ratings compared to their daily ratings, b = 0.437, p = 0.011. In addition, daily ratings of study demands increased more strongly across the two bursts for participants who started assessments later in the semester, b = 0.112, p = 0.032. Together, these patterns suggest that changes in weekly ratings are non-linear across the semester, with satisfaction with study content decreasing across the first half of the semester, and study demands increasing in the second half of the semester. Daily ratings remained relatively more stable across the whole semester, emphasizing that the two assessment types differ in their change dynamics (see also Fig. 3 for a visual representation of these results).
To further examine the difference between daily and weekly ratings, we conducted exploratory analyses in which end-of-week ratings were predicted by the averages of daily ratings of this week, as well as the maximum rating of all daily ratings of a week (i.e., the peak rating of a week). End of week ratings were predicted by average daily ratings (satisfaction with study content, b = 0.785, p < 0.001; study demands, b = 0.837, p < 0.001). Peak ratings in satisfaction with study content, b = 0.180, p = 0.023, but not study demands, b = 0.103, p = 0.174, predicted end-of-week ratings above and beyond mean ratings (see Online Supplement for further details).
Analyses further showed that peak ratings of satisfaction with study content b = −0.052, p < 0.001, but not study demands, b = 0.015, p = 0.393, changed across the semester.
3.3 Predicting retrospective study satisfaction from daily and weekly ratings
Results of the multiple regression analyses are reported in Table 3. When using all available weekly and daily ratings of the semester, both weekly b = 0.742, p < 0.001 (satisfaction with content), b = 0.726, p < 0.001 (study demands), and daily ratings, b = 0.261, p = 0.023 (satisfaction with content), b = 0.190, p = 0.045 (study demands), were uniquely related to retrospective study satisfaction assessed in September. When using only weekly and daily ratings that were collected in the same time frames (i.e., only those weekly ratings collected in the week of the daily ratings), both predictors remained statistically significant (see Table 4). A comparison of these results to the results in Table 3 suggests that the unique effect of the weekly rating slightly decreased, whereas the effects of the daily ratings increased in size. Notably in Table 3, weekly ratings contain information across the whole semester, whereas in Table 4 they contain information only on the same time frames as the daily ratings. This suggests that even though weekly and daily ratings should contain the same information (they refer to the same time span), they show unique validity in predicting retrospective end-of-semester ratings of study satisfaction.
4 Discussion
Intensive longitudinal data offer a rich opportunity to better understand academic and socioemotional adjustment as it unfolds in students’ everyday lives by providing complimentary information compared to data obtained from panel designs. Our results suggest that study satisfaction as reported by university students on a daily or weekly basis provides similar, yet not identical information to global reports of study satisfaction assessed retrospectively at the end of the semester. Furthermore, differences between assessment types could also be observed on a shorter time scale: assessments collected at the end of a week differed to some extent from aggregated daily assessments collected across the week.
4.1 Divergence in levels
For perceived study demands, mean levels were higher with increasing recall period: reports on perceived study demands were lowest for aggregated daily ratings, followed by weekly ratings, and ratings obtained at the end of the semester. This pattern is in line with work by Charles et al. (2016) who compared negative affect ratings collected retrospectively for a day, for a week, or for a month. Here too, average ratings were higher with longer recall periods. In general, the pattern of results converges with prior work on the memory-experience gap, which has consistently reported differences between momentary ratings (Ganzach and Yaor 2019; Neubauer et al. 2020b) or end-of-day ratings (Junghaenel et al. 2021) compared to retrospective evaluations across a longer time frame. For the positive dimension of study satisfaction assessed in the present work—satisfaction with study content—a similar pattern emerged: mean levels increased from daily to weekly, yet, the mean of the most global study satisfaction rating collected (the rating that referred to the overall experience across the whole semester) was similar in size to the average of all weekly ratings.
This pattern of results suggests that the observed discrepancy between different rating types is not due to overly pessimistic retrospective biases because both negative and positive ratings of study satisfaction were larger in global ratings compared to retrospective ratings. Furthermore, different processes might give rise to the memory-experience gap for positive states (e.g., positive affect, satisfaction with study content) and negative states (e.g., negative affect and study demands). Future research is needed to further investigate the roles of peak-and-end effects (Fredrickson 2000), emotion regulation (Neubauer et al. 2020b), and within-person variation (Stone et al. 2005) for the emergence of the memory-experience gap in adjustment measures in educational settings and beyond.
4.2 Divergence in change
The measurement burst design allowed a novel and unique perspective on change in academic adjustment measures across time: By including both daily and weekly ratings, the present study could target not only the question if study satisfaction changes across seven weeks in one semester, but also whether the amount of change varies depending on the type of assessment (daily vs. weekly ratings). By spreading the experience sampling phase of the study (study part 3), information on the first half of the semester could be compared to information on the second half of the semester within participants, and potential non-linear changes could be examined by comparing the trajectories of participants who completed the assessments earlier vs. later in the semester. Results suggested that the increase in perceived study demands was stronger for participants who completed the assessments later in the semester. Closer proximity to exams might be one reason for this accelerated increase in perceived study demands across the semester.
Results further showed that study satisfaction decreased within participants from the first to the second half of the semester. Interestingly, the amount of change in study satisfaction was more pronounced for weekly ratings than for daily ratings: results suggested small differences in the rate of change for both perceived study demands and satisfaction with study content between daily ratings and weekly ratings. Depending on how the data were collected, one might therefore draw slightly different conclusions about the size of change in study satisfaction when using weekly assessments compared to daily assessments. Explanations for this differential change remain speculative, but potentially peak experiences might have biased weekly ratings in an upward direction. Results of exploratory analyses showed that for satisfaction with study contents (but not study demands) peak ratings predicted end of week ratings above and beyond the mean of all daily ratings of the week. That is, the average daily experiences indicate decrease in satisfaction with study contents and weekly recollections of the same time frames suggest even larger decreases across time. This larger decrease was potentially driven by a decrease in peak ratings of study satisfaction. End-of-week ratings in study satisfaction therefore capture not only the average study satisfaction students reported over a week, but they are slightly biased towards the most positive experience of the week.
4.3 Differential predictive utility for global assessments
When predicting retrospective study satisfaction after the semester, both daily and weekly ratings were uniquely associated with global assessments of study satisfaction. In our first modeling approach, we aggregated all available information of the weekly and daily ratings. This gave an advantage to weekly ratings because these contained experiences collected across up to 15 weeks whereas daily ratings contained information on two weeks only. It is all the more noteworthy that even given these conditions, aggregated daily ratings predicted end-of-semester ratings above and beyond weekly ratings. Overall, this pattern suggests that weekly and daily ratings do not contain completely redundant, interchangeable information but that they may each be relevant for predicting future outcomes.
At this point, the reasons for the divergence (and the unique effects of daily and weekly ratings of study satisfaction on retrospective study satisfaction) remain speculative. One explanation could be reduced measurement error of the aggregated daily ratings: By aggregating multiple daily ratings, measurement error can be expected to be reduced compared to single-shot, end-of week ratings of the same construct. By reducing measurement error, the regression coefficient would become disattenuated and their effects in the multiple regression would (compared to weekly ratings with ostensibly larger measurement error) increase. Furthermore, end-of-week ratings could be biased (e.g. in the direction of the peak experience, see Fredrickson 2000).
4.4 Integrating daily, weekly and global assessments of study satisfaction
Results of the present study suggest that daily, weekly and global retrospective assessments of study satisfaction are related, yet the assessments are not mutually redundant. Taking the perspective of classical test theory, it would be expected that repeated assessments (daily, weekly ratings) converge to the true score of the construct they assess. Our results show however that these measures do not fully converge—a finding similar to previous work targeting the assessment of affective states (e.g., Ganzach and Yaor 2019; Junghaenel et al. 2021; Neubauer et al. 2020b). How, then, do these different assessment modi capture (slightly) different constructs? The accessibility model of emotional self-report (Robinson and Clore 2002) argues that longer recall periods (e.g., evaluations of the past semester vs. the past week) activate semantic memory systems and therefore tap into somewhat different concepts than assessments targeting short recall periods or momentary assessments. Similarly, Conner and Barrett (2012) suggested that different assessment types capture different “selves”: Trait assessments which assess self-beliefs (“How do you think you feel in general?”) are hypothesized to capture the believing self and to be a good predictor of deliberate future decisions. In contrast, more short-term retrospective assessments (e.g., “How did you feel in the past week?”) assess the remembering self, and momentary assessments (e.g., “How do you feel right now?”) are hypothesized to capture the experiencing self. This reasoning implies that when choosing a study design and an assessment type (global retrospective assessment vs. repeated daily or weekly assessments), the optimal choice critically depends on the goal of the research. For instance, global self-reports of study satisfaction might be better predictors of study drop-out than (aggregated) momentary, daily or weekly assessments. Consequently, in this case, a panel design might be better suited for research targeting predictors of study drop-out. In contrast, short-term retrospective or momentary assessments might be more sensitive to capture effects of interventions aiming to improve study satisfaction: It seems conceivable that intervention effects are captured faster by measures that target more short-term experiences. In this context, momentary experiences or daily retrospective reports might be more strongly related to transient events that occurred close to the time of assessment, whereas global self-reports are likely less impacted by short-term events. This example further highlights that panel designs and intensive longitudinal designs bear distinct strengths and weaknesses which need to be considered when deciding on an appropriate study design for specific research questions.
Notably, these previous elaborations primarily target the question why and to what extent momentary experiences, memories of these experiences, and self-beliefs are distinct. They are of course also interrelated. In the context of affective experiences, previous studies showed that retrospective assessments of momentary affective states are biased in the direction of self-beliefs: Higher degrees of depression (Zetsche et al. 2019) and neuroticism (Lay et al. 2017) have been associated with an overestimation of past negative affect; extraversion (Lay et al. 2017) has been linked to exaggerated recall of positive affect. This suggests that general beliefs about oneself (“I get easily irritated”) can distort retrospective assessments. In addition to these top-down processes (self-beliefs shape experiences and memories thereof), there are likely also bottom-up processes that link experiences to later self-beliefs. Our results showed that memories of study satisfaction (on the daily and weekly level) predict more global, end-of-semester assessments of study satisfaction: How students recalled individual days/weeks across the semester predicted how they retrospectively recalled the semester. Extrapolating these findings, we further speculate that in general, momentary experiences build up self-beliefs over time—but only if these experiences are retrospectively recalled. For instance, students who repeatedly experience moments of fairly low study satisfaction are expected to show a long-term decrease in global study satisfaction over time. However, not all “bad” moments are recalled, even at the end of the week (see for instance the non-perfect convergence of daily and weekly assessments). Those “bad” moments that are no longer recalled at the end of the week will likely also not impact students’ global self-beliefs later. Figure 4 illustrates this idea: self-beliefs can change over time, and these changes are related to how individuals remember their experiences. According to this model, experiences themselves only predict changes in self-beliefs via memories of experiences (there is no direct path from experiences to change in self-beliefs). We hope that the conceptual model in Fig. 4 is useful for future confirmatory research targeting the dynamic interplay of experiences, memories thereof and self-beliefs.
4.5 Limitations
A number of limitations have to be considered when interpreting the results of the present study. First, although this study utilized an experience sampling approach, we collected retrospective assessments of study satisfaction only. Retrospective end-of-day assessments probably do not contain the same information as aggregated momentary assessments collected across the day (Neubauer et al. 2020b). However, it might be difficult to assess momentary study satisfaction because questions about momentary study satisfaction might not be relevant in all daily situations. Future studies might therefore consider (a) using an event-contingent sampling scheme where participants are asked to report their momentary study satisfaction in contexts relevant to their study situation (e.g., in lectures) or (b) collecting momentary study satisfaction in these specific contexts. Second, we targeted specifically study satisfaction. Future research would need to determine whether the pattern of results is also generalizable to other relevant constructs in the context of tertiary education (e.g., study-related motivation, learning goals, perceived belonging, intention to drop out). Third, compliance rates in the experience sampling parts were lower than the average compliance rates reported in a recent meta-analysis on experience sampling studies (Wrzus and Neubauer 2022). This may have partially been due to no direct financial reimbursement of participants (retail vouchers were raffled after each week to retain compliance) and may restrict the generalizability of the reported results to some extent. Fourth, there is a wealth of parameters that can be extracted from intensive longitudinal data such as the intra-individual standard deviation (e.g., Eid and Diener 1999), autoregressive parameters (inertia; e.g., Kuppens et al. 2010), or within-person couplings among different time-varying variables (e.g., Neubauer and Schmiedek 2020). In the present analyses, we focused only on the means of individuals’ intensive longitudinal data given the focus on the question whether these converge with global retrospective evaluations. Future research might exploit this rich intensive longitudinal data further, targeting other process-related parameters of daily and/or weekly study satisfaction.
5 Conclusions
A profound understanding of psychological adaptation requires targeting this phenomenon on multiple temporal resolutions. Intensive longitudinal designs allow to capture momentary experiences or recollection of experiences with short recall periods, while panel designs allow to assess more global, retrospective evaluations across a longer recall period. Our findings suggest that the information gained from assessments of students’ study satisfaction differs between intensive longitudinal designs and panel designs. Therefore, combining both designs into measurement burst designs can be a very powerful means to learn more about the process underlying academic adaptation and their (medium- and long-term) consequences.
Notes
Effect sizes were computed as Cohen’s ds, that is, the variances of the three ratings were pooled and the mean differences were standardized relative to the square root of the pooled variance.
References
Blossfeld, H.-P., & Roßbach, H.-G. (Eds.). (2019). Education as a lifelong process: The German National Educational Panel Study (NEPS) (Edition ZfE). Wiesbaden: Springer.
Bolger, N., & Laurenceau, J.-P. (2013). Intensive longitudinal methods: An introduction to diary and experience sampling research. Methodology in the social sciences. New York: Guilford.
Bolger, N., Davis, A., & Rafaeli, E. (2003). Diary methods: Capturing life as it is lived. Annual Review of Psychology, 54(1), 579–616. https://doi.org/10.1146/annurev.psych.54.101601.145030.
Bolger, N., Zee, K. S., Rossignac-Milon, M., & Hassin, R. R. (2019). Causal processes in psychology are heterogeneous. Journal of Experimental Psychology: General, 148(4), 601–618. https://doi.org/10.1037/xge0000558.
Charles, S. T., Piazza, J. R., Mogle, J. A., Urban, E. J., Sliwinski, M. J., & Almeida, D. M. (2016). Age differences in emotional well-being vary by temporal recall. The Journals of Gerontology. Series B, Psychological Sciences and Social Sciences, 71(5), 798–807. https://doi.org/10.1093/geronb/gbv011.
Conner, T. S., & Barrett, L. F. (2012). Trends in ambulatory self-report: The role of momentary experience in psychosomatic medicine. Psychosomatic Medicine, 74(4), 327–337. https://doi.org/10.1097/PSY.0b013e3182546f18.
Eid, M., & Diener, E. (1999). Intraindividual variability in affect: Reliability, validity, and personality correlates. Journal of Personality and Social Psychology, 76, 662–676.
Fredrickson, B. L. (2000). Extracting meaning from past affective experiences: The importance of peaks, ends, and specific emotions. Cognition & Emotion, 14(4), 577–606. https://doi.org/10.1080/026999300402808.
Ganzach, Y., & Yaor, E. (2019). The retrospective evaluation of positive and negative affect. Personality & Social Psychology Bulletin, 45(1), 93–104. https://doi.org/10.1177/0146167218780695.
Geldhof, G. J., Preacher, K. J., & Zyphur, M. J. (2014). Reliability estimation in a multilevel confirmatory factor analysis framework. Psychological Methods, 19(1), 72–91. https://doi.org/10.1037/a0032138.
Goebel, J., Grabka, M. M., Liebig, S., Kroh, M., Richter, D., Schröder, C., & Schupp, J. (2019). The German Socio-Economic Panel (SOEP). Jahrbücher Für Nationalökonomie Und Statistik, 239(2), 345–360. https://doi.org/10.1515/jbnst-2018-0022.
Junghaenel, D. U., Broderick, J. E., Schneider, S., Wen, C. K. F., Mak, H. W., Goldstein, S., Mendez, M., & Stone, A. A. (2021). Explaining age differences in the memory-experience gap. Psychology and Aging, 36(6), 679–693. https://doi.org/10.1037/pag0000628.
Ketonen, E. E., Malmberg, L.-E., Salmela-Aro, K., Muukkonen, H., Tuominen, H., & Lonka, K. (2019). The role of study engagement in university students’ daily experiences: A multilevel test of moderation. Learning and Individual Differences, 69, 196–205. https://doi.org/10.1016/j.lindif.2018.11.001.
Kramer, C. Y. (1956). Extension of multiple range tests to group means with unequal numbers of replications. Biometrics, 12, 307–310.
Kuppens, P., Allen, N. B., & Sheeber, L. B. (2010). Emotional inertia and psychological maladjustment. Psychological Science, 21(7), 984–991. https://doi.org/10.1177/0956797610372634.
Lay, J. C., Gerstorf, D., Scott, S. B., Pauly, T., & Hoppmann, C. A. (2017). Neuroticism and extraversion magnify discrepancies between retrospective and concurrent affect reports. Journal of Personality, 85(6), 817–829. https://doi.org/10.1111/jopy.12290.
Leiner, D. J. (2019). SoSci Survey (Version 3.2.23). https://www.soscisurvey.de Computer software. Accessed: 12. March 2021.
Mehl, M. R., & Conner, T. S. (Eds.). (2012). Handbook of research methods for studying daily life. New York: Guilford.
Menkor, M., Nagengast, B., van Laar, C., & Sassenberg, K. (2021). The fit between dignity self-construal and independent university norms: Effects on university belonging, well-being, and academic success. European Journal of Social Psychology, 51(1), 100–112. https://doi.org/10.1002/ejsp.2717.
Miron-Shatz, T., Stone, A., & Kahneman, D. (2009). Memories of yesterday’s emotions: Does the valence of experience affect the memory-experience gap? Emotion (Washington, D.C.), 9(6), 885–891. https://doi.org/10.1037/a0017823.
Nesselroade, J. R. (1991). The warp and woof of the developmental fabric. In R. Downs, L. Liben & D. S. Palermo (Eds.), Visions of aesthetics, the environment, and development: The legacy of Joachim F. Wohwill (pp. 213–240). Mahwah: Lawrence Erlbaum.
Neubauer, A. B., & Kramer, A. C. (2022). STECCO—Starting tertiary education during the corona crisis: a challenge and an opportunity. Study protocol. https://doi.org/10.17605/OSF.IO/2q4wz.
Neubauer, A. B., & Schmiedek, F. (2020). Studying within-person variation and within-person couplings in intensive longitudinal data: lessons learned and to be learned. Gerontology, 66(4), 332–339. https://doi.org/10.1159/000507993.
Neubauer, A. B., Lerche, V., & Voss, A. (2018). Interindividual differences in the intraindividual association of competence and well-being: Combining experimental and intensive longitudinal designs. Journal of Personality, 86(4), 698–713. https://doi.org/10.1111/jopy.12351.
Neubauer, A. B., Lerche, V., Koehler, F., & Voss, A. (2020a). What do you (think you) need? Perceived vs. experienced effects of need fulfillment on well-being. Journal of Research in Personality, 86, 103938. https://doi.org/10.1016/j.jrp.2020.103938.
Neubauer, A. B., Scott, S. B., Sliwinski, M. J., & Smyth, J. M. (2020b). How was your day? Convergence of aggregated momentary and retrospective end-of-day affect ratings across the adult life span. Journal of Personality and Social Psychology, 119(1), 185–203. https://doi.org/10.1037/pspp0000248.
Neubauer, A. B., Kramer, A. C., & Soenens, B. (2021). STECCO—starting tertiary education during the corona crisis: a challenge and an opportunity. https://doi.org/10.17605/OSF.IO/BHQ3P.
Neubauer, A. B., Brose, A., & Schmiedek, F. (2022). How within-person effects shape between-person differences: A multilevel structural equation modeling perspective. Psychological Methods. https://doi.org/10.1037/met0000481.
Redelmeier, D. A., Katz, J., & Kahneman, D. (2003). Memories of colonoscopy: a randomized trial. Pain, 104(1), 187–194. https://doi.org/10.1016/S0304-3959(03)00003-4.
Rights, J. D., & Sterba, S. K. (2019). Quantifying explained variance in multilevel models: An integrative framework for defining R-squared measures. Psychological Methods, 24(3), 309–338. https://doi.org/10.1037/met0000184
Robinson, M. D., & Clore, G. L. (2002). Belief and feeling: evidence for an accessibility model of emotional self-report. Psychological Bulletin, 128(6), 934–960. https://doi.org/10.1037/0033-2909.128.6.934.
Scheunemann, A., Schnettler, T., Bobe, J., Fries, S., & Grunschel, C. (2021). A longitudinal analysis of the reciprocal relationship between academic procrastination, study satisfaction, and dropout intentions in higher education. European Journal of Psychology of Education. https://doi.org/10.1007/s10212-021-00571-z.
Schmidt, A., Neubauer, A. B., Dirk, J., & Schmiedek, F. (2020). The bright and the dark side of peer relationships: Differential effects of relatedness satisfaction and frustration at school on affective well-being in children’s daily lives. Developmental Psychology, 56(8), 1532–1546. https://doi.org/10.1037/dev0000997.
Sliwinski, M. J. (2008). Measurement-burst designs for social health research. Social and Personality Psychology Compass, 2(1), 245–261. https://doi.org/10.1111/j.1751-9004.2007.00043.x.
Stone, A. A., Schwartz, J. E., Broderick, J. E., & Shiffman, S. S. (2005). Variability of momentary pain predicts recall of weekly pain: A consequence of the peak (or salience) memory heuristic. Personality & Social Psychology Bulletin, 31(10), 1340–1346. https://doi.org/10.1177/0146167205275615.
Stone, A. A., Broderick, J. E., & Schwartz, J. E. (2010). Validity of average, minimum, and maximum end-of-day recall assessments of pain and fatigue. Contemporary Clinical Trials, 31(5), 483–490. https://doi.org/10.1016/j.cct.2010.06.004.
Trull, T. J., & Ebner-Priemer, U. (2013). Ambulatory assessment. Annual Review of Clinical Psychology, 9, 151–176. https://doi.org/10.1146/annurev-clinpsy-050212-185510.
Tukey, J. W. (1953). The problem of multiple comparisions. Princeton: Princeton University Press.
Westermann, R., Elke, H., Spies, K., & Trautwein, U. (1996). Identifikation und Erfassung von Komponenten der Studienzufriedenheit. Psychologie in Erziehung Und Unterricht, 43(1), 1–22.
Westermann, R., Heise, E., & Spies, K. (2018). FB-SZ‑K – Kurzfragebogen zur Erfassung der Studienzufriedenheit. https://doi.org/10.23668/PSYCHARCHIVES.4654.
Wrzus, C., & Neubauer, A. B. (2023). Ecological Momentary Assessment: A Meta-Analysis on Designs, Samples, and Compliance Across Research Fields. Assessment, 30(3), 825–846. https://doi.org/10.1177/10731911211067538.
Zetsche, U., Bürkner, P.-C., & Renneberg, B. (2019). Future expectations in clinical depression: Biased or realistic? Journal of Abnormal Psychology, 128(7), 678–688. https://doi.org/10.1037/abn0000452.
Acknowledgements
The authors thank Andrea Kramer and Anna-Maria Grimm for their invaluable support in conducting this research.
Funding
This research was supported by grants awarded to Andreas B. Neubauer by the Volkswagen Foundation (Grant number 99 728) and the German Research Foundation (DFG; Grant number NE 2480/1-1).
Funding
Open Access funding enabled and organized by Projekt DEAL.
Author information
Authors and Affiliations
Corresponding author
Ethics declarations
Conflict of interest
A.B. Neubauer and F. Schmiedek declare that they have no competing interests.
Rights and permissions
Open Access This article is licensed under a Creative Commons Attribution 4.0 International License, which permits use, sharing, adaptation, distribution and reproduction in any medium or format, as long as you give appropriate credit to the original author(s) and the source, provide a link to the Creative Commons licence, and indicate if changes were made. The images or other third party material in this article are included in the article’s Creative Commons licence, unless indicated otherwise in a credit line to the material. If material is not included in the article’s Creative Commons licence and your intended use is not permitted by statutory regulation or exceeds the permitted use, you will need to obtain permission directly from the copyright holder. To view a copy of this licence, visit http://creativecommons.org/licenses/by/4.0/.
About this article
Cite this article
Neubauer, A.B., Schmiedek, F. Approaching academic adjustment on multiple time scales. Z Erziehungswiss 27, 147–168 (2024). https://doi.org/10.1007/s11618-023-01182-8
Received:
Revised:
Accepted:
Published:
Issue Date:
DOI: https://doi.org/10.1007/s11618-023-01182-8