Abstract
Purpose
The aim of this single-center retrospective study is to assess whether contrast-enhanced computed tomography (CECT) radiomics analysis is predictive of gastroenteropancreatic neuroendocrine neoplasms (GEP-NENs) grade based on the 2019 World Health Organization (WHO) classification and to establish a tumor grade (G) prediction model.
Material and methods
Preoperative CECT images of 78 patients with GEP-NENs were retrospectively reviewed and divided in two groups (G1–G2 in class 0, G3-NEC in class 1). A total of 107 radiomics features were extracted from each neoplasm ROI in CT arterial and venous phases acquisitions with 3DSlicer. Mann–Whitney test and LASSO regression method were performed in R for feature selection and feature reduction, in order to build the radiomic-based predictive model. The model was developed for a training cohort (75% of the total) and validated on the independent validation cohort (25%). ROC curves and AUC values were generated on training and validation cohorts.
Results
40 and 24 features, for arterial phase and venous phase, respectively, were found to be significant in class distinction. From the LASSO regression 3 and 2 features, for arterial phase and venous phase, respectively, were identified as suitable for groups classification and used to build the tumor grade radiomic-based prediction model. The prediction of the arterial model resulted in AUC values of 0.84 (95% CI 0.72–0.97) and 0.82 (95% CI 0.62–1) for the training cohort and validation cohort, respectively, while the prediction of the venous model yielded AUC values of 0.7877 (95% CI 0.6416–0.9338) and 0.6813 (95% CI 0.3933–0.9693) for the training cohort and validation cohort, respectively.
Conclusions
CT-radiomics analysis may aid in differentiating the histological grade for GEP-NENs.






Similar content being viewed by others
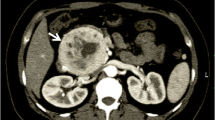
References
Bilimoria KY, Tomlinson JS, Merkow RP, Stewart AK, Ko CY, Talamonti MS et al (2007) Clinicopathologic features and treatment trends of pancreatic neuroendocrine tumors: analysis of 9,821 patients. J Gastrointest Surg 11:1460–1469. https://doi.org/10.1007/S11605-007-0263-3
Ito T, Igarashi H, Nakamura K, Sasano H, Okusaka T, Takano K et al (2015) Epidemiological trends of pancreatic and gastrointestinal neuroendocrine tumors in Japan: a nationwide survey analysis. J Gastroenterol 50:58–64. https://doi.org/10.1007/S00535-014-0934-2
Granata V, Coppola F, Grassi R, Fusco R, Tafuto S, Izzo F et al (2021) Structured reporting of computed tomography in the staging of neuroendocrine neoplasms: a Delphi consensus proposal. Front Endocrinol (Lausanne). https://doi.org/10.3389/FENDO.2021.748944
Fang JM, Shi J (2019) A clinicopathologic and molecular update of pancreatic neuroendocrine neoplasms with a focus on the new World Health Organization Classification. Arch Pathol Lab Med 143:1317–1326. https://doi.org/10.5858/ARPA.2019-0338-RA
Bicci E, Cozzi D, Ferrari R, Grazzini G, Pradella S, Miele V (2020) Pancreatic neuroendocrine tumours: spectrum of imaging findings. Gland Surg. 9:2215–24. https://doi.org/10.21037/GS-20-537
Chiti G, Grazzini G, Cozzi D, Danti G, Matteuzzi B, Granata V et al (2021) Imaging of pancreatic neuroendocrine neoplasms. Int J Environ Res Public Health. https://doi.org/10.3390/IJERPH18178895
Danti G, Flammia F, Matteuzzi B, Cozzi D, Berti V, Grazzini G et al (2021) Gastrointestinal neuroendocrine neoplasms (GI-NENs): hot topics in morphological, functional, and prognostic imaging. Radiol Med 126:1497–1507. https://doi.org/10.1007/S11547-021-01408-X
Nagtegaal ID, Odze RD, Klimstra D, Paradis V, Rugge M, Schirmacher P et al (2020) The 2019 WHO classification of tumours of the digestive system. Histopathology 76:182–188. https://doi.org/10.1111/HIS.13975
Garcia-Carbonero R, Sorbye H, Baudin E, Raymond E, Wiedenmann B, Niederle B et al (2016) ENETS consensus guidelines for high-grade gastroenteropancreatic neuroendocrine tumors and neuroendocrine carcinomas. Neuroendocrinology 103:186–194. https://doi.org/10.1159/000443172
Busico A, Maisonneuve P, Prinzi N, Pusceddu S, Centonze G, Garzone G et al (2020) Gastroenteropancreatic high-grade neuroendocrine neoplasms: histology and molecular analysis, two sides of the same coin. Neuroendocrinology 110:616–629. https://doi.org/10.1159/000503722
Dasari A, Shen C, Halperin D, Zhao B, Zhou S, Xu Y et al (2017) Trends in the incidence, prevalence, and survival outcomes in patients with neuroendocrine tumors in the United States. JAMA Oncol 3:1335–1342. https://doi.org/10.1001/JAMAONCOL.2017.0589
Grazzini G, Danti G, Cozzi D, Lanzetta MM, Addeo G, Falchini M et al (2019) Diagnostic imaging of gastrointestinal neuroendocrine tumours (GI-NETs): relationship between MDCT features and 2010 WHO classification. Radiol Med 124:94–102. https://doi.org/10.1007/S11547-018-0946-8
Sorbye H, Welin S, Langer SW, Vestermark LW, Holt N, Osterlund P et al (2013) Predictive and prognostic factors for treatment and survival in 305 patients with advanced gastrointestinal neuroendocrine carcinoma (WHO G3): the NORDIC NEC study. Ann Oncol 24:152–160. https://doi.org/10.1093/ANNONC/MDS276
Milione M, Maisonneuve P, Spada F, Pellegrinelli A, Spaggiari P, Albarello L et al (2017) The clinicopathologic heterogeneity of grade 3 gastroenteropancreatic neuroendocrine neoplasms: morphological differentiation and proliferation identify different prognostic categories. Neuroendocrinology 104:85–93. https://doi.org/10.1159/000445165
Sorbye H, Baudin E, Borbath I, Caplin M, Chen J, Cwikla JB et al (2019) Unmet needs in high-grade gastroenteropancreatic neuroendocrine neoplasms (WHO G3). Neuroendocrinology 108:54–62. https://doi.org/10.1159/000493318
Sorbye H, Kong G, Grozinsky-Glasberg S (2020) PRRT in high-grade gastroenteropancreatic neuroendocrine neoplasms (WHO G3). Endocr Relat Cancer 27:R67-77. https://doi.org/10.1530/ERC-19-0400
Grassi R, Miele V, Giovagnoni A (2019) Artificial intelligence: a challenge for third millennium radiologist. Radiol Med 124:241–242. https://doi.org/10.1007/S11547-019-00990-5
Hassani C, Saremi F, Varghese BA, Duddalwar V (2020) Myocardial radiomics in cardiac MRI. AJR Am J Roentgenol 214:536–545. https://doi.org/10.2214/AJR.19.21986
Scapicchio C, Gabelloni M, Barucci A, Cioni D, Saba L, Neri E (2021) A deep look into radiomics. Radiol Med 126:1296–1311. https://doi.org/10.1007/S11547-021-01389-X
Coppola F, Faggioni L, Regge D, Giovagnoni A, Golfieri R, Bibbolino C et al (2021) Artificial intelligence: radiologists’ expectations and opinions gleaned from a nationwide online survey. Radiol Med 126:63–71. https://doi.org/10.1007/S11547-020-01205-Y
Shiradkar R, Ghose S, Jambor I, Taimen P, Ettala O, Purysko AS et al (2018) Radiomic features from pretreatment biparametric MRI predict prostate cancer biochemical recurrence: preliminary findings. J Magn Reson Imaging 48:1626–1636. https://doi.org/10.1002/JMRI.26178
Kumar V, Gu Y, Basu S, Berglund A, Eschrich SA, Schabath MB et al (2012) Radiomics: the process and the challenges. Magn Reson Imaging 30:1234–1248. https://doi.org/10.1016/J.MRI.2012.06.010
Canellas R, Burk KS, Parakh A, Sahani DV (2018) Prediction of pancreatic neuroendocrine tumor grade based on CT features and texture analysis. Am Roentgenol Ray Soc. 210:341–6. https://doi.org/10.2214/AJR.17.18417
Cusumano D, Meijer G, Lenkowicz J, Chiloiro G, Boldrini L, Masciocchi C et al (2021) A field strength independent MR radiomics model to predict pathological complete response in locally advanced rectal cancer. Radiol Med 126:421–429. https://doi.org/10.1007/S11547-020-01266-Z
Santone A, Brunese MC, Donnarumma F, Guerriero P, Mercaldo F, Reginelli A et al (2021) Radiomic features for prostate cancer grade detection through formal verification. Radiol Med 126:688–697. https://doi.org/10.1007/S11547-020-01314-8
Chiloiro G, Cusumano D, de Franco P, Lenkowicz J, Boldrini L, Carano D et al (2022) Does restaging MRI radiomics analysis improve pathological complete response prediction in rectal cancer patients? A prognostic model development. Radiol Med 127:11–20. https://doi.org/10.1007/S11547-021-01421-0
Lambin P, Rios-Velazquez E, Leijenaar R, Carvalho S, van Stiphout RGPM, Granton P et al (2012) Radiomics: extracting more information from medical images using advanced feature analysis. Eur J Cancer 48:441–446. https://doi.org/10.1016/J.EJCA.2011.11.036
Gillies RJ, Kinahan PE, Hricak H (2016) Radiomics: images are more than pictures, they are data. Radiology 278:563–577. https://doi.org/10.1148/RADIOL.2015151169
Palatresi D, Fedeli F, Danti G, Pasqualini E, Castiglione F, Messerini L et al (2022) Correlation of CT radiomic features for GISTs with pathological classification and molecular subtypes: preliminary and monocentric experience. Radiol Med 127:117–128. https://doi.org/10.1007/S11547-021-01446-5
Karmazanovsky G, Gruzdev I, Tikhonova V, Kondratyev E, Revishvili A (2021) Computed tomography-based radiomics approach in pancreatic tumors characterization. Radiol Med 126:1388–1395. https://doi.org/10.1007/S11547-021-01405-0
Sahani DV, Bonaffini PA, Fernández Del Castillo C, Blake MA (2013) Gastroenteropancreatic neuroendocrine tumors: role of imaging in diagnosis and management. Radiology. 266:38–61. https://doi.org/10.1148/RADIOL.12112512
Gregucci F, Fiorentino A, Mazzola R, Ricchetti F, Bonaparte I, Surgo A et al (2022) Radiomic analysis to predict local response in locally advanced pancreatic cancer treated with stereotactic body radiation therapy. Radiol Med 127:100–107. https://doi.org/10.1007/S11547-021-01422-Z
Neri E, Coppola F, Miele V, Bibbolino C, Grassi R (2020) Artificial intelligence: who is responsible for the diagnosis? Radiol Med 125:517–521. https://doi.org/10.1007/S11547-020-01135-9
Chen PT, Chang D, Yen H, Liu KL, Huang SY, Roth H et al (2021) Radiomic features at CT can distinguish pancreatic cancer from noncancerous pancreas. Radiol Imaging Cancer. https://doi.org/10.1148/RYCAN.2021210010
del Re M, Cucchiara F, Rofi E, Fontanelli L, Petrini I, Gri N et al (2021) A multiparametric approach to improve the prediction of response to immunotherapy in patients with metastatic NSCLC. Cancer Immunol Immunother 70:1667–1678. https://doi.org/10.1007/S00262-020-02810-6
Asselin MC, O’Connor JPB, Boellaard R, Thacker NA, Jackson A (2012) Quantifying heterogeneity in human tumours using MRI and PET. Eur J Cancer 48:447–455. https://doi.org/10.1016/J.EJCA.2011.12.025
Hu H, Shan Q, Chen S, Li B, Feng S, Xu E et al (2020) CT-based radiomics for preoperative prediction of early recurrent hepatocellular carcinoma: technical reproducibility of acquisition and scanners. Radiol Med. 125:697–705. https://doi.org/10.1007/S11547-020-01174-2
Srisajjakul S, Prapaisilp P, Bangchokdee S (2020) CT and MR features that can help to differentiate between focal chronic pancreatitis and pancreatic cancer. Radiol Med 125:356–364. https://doi.org/10.1007/S11547-019-01132-7
Liang W, Yang P, Huang R, Xu L, Wang J, Liu W et al (2019) A combined nomogram model to preoperatively predict histologic grade in pancreatic neuroendocrine tumors. Clin Cancer Res 25:584–594. https://doi.org/10.1158/1078-0432.CCR-18-1305
Bian Y, Jiang H, Ma C, Wang L, Zheng J, Jin G et al (2020) CT-based radiomics score for distinguishing between grade 1 and grade 2 nonfunctioning pancreatic neuroendocrine tumors. AJR Am J Roentgenol 215:852–863. https://doi.org/10.2214/AJR.19.22123
Gu D, Hu Y, Ding H, Wei J, Chen K, Liu H et al (2019) CT radiomics may predict the grade of pancreatic neuroendocrine tumors: a multicenter study. Eur Radiol 29:6880–6890. https://doi.org/10.1007/S00330-019-06176-X
Zhao Z, Bian Y, Jiang H, Fang X, Li J, Cao K et al (2020) CT-radiomic approach to predict G1/2 nonfunctional pancreatic neuroendocrine tumor. Acad Radiol 27:e272–e281. https://doi.org/10.1016/J.ACRA.2020.01.002
Zilli A, Arcidiacono PG, Conte D, Massironi S (2018) Clinical impact of endoscopic ultrasonography on the management of neuroendocrine tumors: lights and shadows. Dig Liver Dis 50:6–14. https://doi.org/10.1016/J.DLD.2017.10.007
Benedetti G, Mori M, Panzeri MM, Barbera M, Palumbo D, Sini C et al (2021) CT-derived radiomic features to discriminate histologic characteristics of pancreatic neuroendocrine tumors. Radiol Med 126:745–760. https://doi.org/10.1007/S11547-021-01333-Z
IARC Publications Website—WHO Classification of Tumours of the Digestive System [Internet]
Funding
The authors declare that no funds, grants, or other support were received during the preparation of this manuscript.
Author information
Authors and Affiliations
Contributions
Conceptualization was performed by GG and BM. Formal analysis was performed by GC, FF, PT and SB. Investigation was performed by GC and FF. Resources were collected by SB. Data curation was performed by GC, FF, EP and LM. GC, FF and GG were involved in writing—original draft preparation. GG was involved in writing—review and editing and project administration. Visualization was performed by GC, PT and SB. BM, VG, SP, DM and VM performed supervision. All authors have read and agreed to the published version of the manuscript.
Corresponding author
Ethics declarations
Conflict of interest
The authors declare that they have no conflict of interest related to the publication of this article.
Ethical standards
Institutional Review Board Statement number 13261_oss date of approval February 02, 2021. The study was conducted according to the guidelines of the Declaration of Helsinki.
Informed consent
Informed consent was obtained from all subjects involved in the study. All patients gave their informed consent for the CT examination with intravenous administration. Patients’ records were anonymized and de-identified prior to analysis.
Additional information
Publisher's Note
Springer Nature remains neutral with regard to jurisdictional claims in published maps and institutional affiliations.
Rights and permissions
Springer Nature or its licensor holds exclusive rights to this article under a publishing agreement with the author(s) or other rightsholder(s); author self-archiving of the accepted manuscript version of this article is solely governed by the terms of such publishing agreement and applicable law.
About this article
Cite this article
Chiti, G., Grazzini, G., Flammia, F. et al. Gastroenteropancreatic neuroendocrine neoplasms (GEP-NENs): a radiomic model to predict tumor grade. Radiol med 127, 928–938 (2022). https://doi.org/10.1007/s11547-022-01529-x
Received:
Accepted:
Published:
Issue Date:
DOI: https://doi.org/10.1007/s11547-022-01529-x