Abstract
There are growing concerns about environmental degradation and economic expansions in West Africa. Although there are several growth-environmental studies in Africa, there is limited empirical research exploring West African countries’ potential of benefiting from the environmental Kuznets curve (EKC) hypothesis, with the few studies on this subject reporting diverse results based on selected West African countries. To fill this gap, this study explored the relationship between economic growth and environmental degradation within the EKC framework using 16 West African countries sub-grouped into low-income countries (LICs) and lower-middle-income countries (LMICs) between 1990 and 2018. This study implemented second-generation panel econometric estimators that are robust to cross-sectional dependent and parameter heterogeneity. The empirical results revealed that the data is cross-sectionally dependent, heterogeneous, integrated of order one, 1(1), and cointegrated. Controlling for other environmental determinants, panel estimates from the Augmented Meant Group and Common Correlated Effect Mean Group estimators revealed that economic growth accelerates environmental degradation in West African countries, with a greater impact on LMICs, followed by LICs in West Africa. The results also showed that West African countries especially LMICs could benefit from the EKC hypothesis. On the other hand, growth-environmental degradation among LICs in West Africa shows a monotonous increasing relationship. We found strong evidence to support for feedback hypothesis between economic growth and environmental degradation in LMICs, LICs, and West Africa as a whole. Based on the findings, policy recommendations that consider both LMICs and LICs and West Africa as a whole were offered to policymakers.
Graphical abstract

Similar content being viewed by others
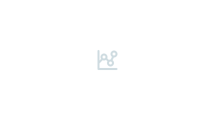
Avoid common mistakes on your manuscript.
Introduction
Growth and environmental consequences continue to be a major concern to researchers and policymakers at large. West Africa, a resource-dependent region (with each nation endowed with diverse resources) and one of the world’s fastest-growing regions, is receiving growth-environmental attention (Bazilian et al. 2013; USAID 2021). With the increasing economic growth (GDP) in West Africa, there are concerns regarding environmental degradation (ED). According to the World Bank Data, GDP in West Africa increased from US$ 211.30 billion in 1990 to US$ 700.93 billion (constant 2010 US$) in 2019 (World Bank 2021) representing a 331.73% increase over a period of 30 years. Within the same period, the GDP of low-income countries (LICs) grew from US$ 21.84 to US$ 72.29 billion, while lower-middle-income countries (LMICs) grew from US$ 189.45 to US$ 628.64 billion. Similarly, carbon emissions (CO2) in West Africa increased from 81,730 kt in 1990 to 199,860 kt in 2018 (World Bank 2021) representing a 244.54% increase in a period of 29 years. Within the same period, the CO2 of LICs and LMICs in West Africa grew from 4730 kt and 77,000 kt to 20,780 kt and 179,080 kt, respectively. Although worldwide carbon emissions decreased during the novel coronavirus 2019 disease (COVID-19) pandemic (Wang et al. 2022a, b; Wang and Zhang 2021), this was described as a temporary effect owing to the economic slowdown caused by the lockdown/shutdown (Hu et al. 2022; Ray et al. 2021). This draws more attention to post-pandemic economic activities and carbon emission connections, a major concern to policymakers and academics working towards environmental sustainability in West Africa.
The general notion that an increase in GDP increases CO2 (Kahia et al. 2019; Rahman and Vu 2020; Mensah et al. 2021) implies that economic expansion in West Africa will continually escalate CO2 in the region. However, the environmental Kuznets curve (EKC) hypothesis provides a framework for examining growth-environmental relationships through three channels: (1) scale effect (increased GDP adversely affects the environment), composition effect (structural changes could worsen or improve the environment), and technique effect (economic advancement promote environment by shifting toward more environmentally friendly technologies and regulations) (Mitić et al. 2019). Thus, EKC is one of the most well-known hypotheses that explain the relationship between GDP and ED (Apergis 2016; Dogan and Inglesi-Lotz 2017; Mitić et al. 2019; Ntarmah et al. 2022). It represents an important model that enables policymakers to deliver quality information–based decisions. EKC theory argues that the growth-environmental relationship is an inverted U-shaped, such that economic development initially leads to ED until a certain threshold where further economic development reduces ED through either composition or technical effects. This implies that growth-environmental relationships in West Africa should not be viewed solely from an adverse relationship perspective since there could be a potential for West African countries to benefit from EKC theoretical arguments.
Although there are many growth-environmental studies across the world, the results are diverse. Some scholars established a linear/monotonic relationship (Akadiri et al. 2019; Destek and Sinha 2020; Khan et al. 2021; Dimnwobi et al. 2021; Mensah et al. 2021), but no significant relationship (Omri et al. 2015; Bekhet et al. 2017; Charfeddine and Kahia 2019), U-shaped (Xu et al. 2020), inverted U-Shaped–EKC (Al-Mulali et al. 2016; Halliru et al. 2020; Ntarmah et al. 2021), N, or inverted N shape (Mitić et al. 2019; Sarkodie and Strezov 2019). From the perspective of selected countries in West Africa, Halliru et al. (2020) found a U-shaped relationship, while Omojolaibi (2010) found an inverted-U shaped with OLS but FE regression revealed no significant relationships between GDP and environment. The mixed results found in the literature are largely attributed to the different sample, periods, methodological frameworks, and analytical approaches. Interestingly, only a few studies (Omojolaibi 2010; Adu and Denkyirah 2017; Halliru et al. 2020) have examined growth-environmental relationships in West Africa within the EKC framework. Many scholars focus on SSA or Africa as a whole with West Africa treated as a sub-region in their studies. As such, the sub-regional analysis conducted by these scholars (Wasiu and Alasinrin 2015; Coulibaly 2016; Halliru et al. 2020) only focuses on West Africa as a whole ignoring heterogeneity (LICs and LMICs) among the West African countries. Considering the income heterogeneity in West Africa, the whole sample analysis may mislead policy directions since the outcomes suffer from heterogeneity problems (Apergis 2016; Musah et al. 2020).
To address this gap in the literature, this study seeks to examine the relationship between GDP and ED in West Africa within the EKC framework. The objective is divided into four specific objectives. First, it examines the growth-environmental relationships of West African countries as a whole. Second, it analyzes growth-environmental relationships from the perspectives of LICs and LMICs in West Africa. Third, it tests the possibility of the EKC hypothesis in West Africa from the perspectives of LICs, LMICs, and all sampled countries. Finally, it investigates feedback mechanisms between GDP and ED.
This study contributes to EKC theoretical argument and environmental studies in many ways. First, limited studies have empirically examined the EKC hypothesis in West Africa with a few studies relying on selected West African countries to investigate the topic, which has yielded mixed results. We address this gap by drawing data from all the 16 West African countries to explore possible EKC existence to aid policy decisions in the region. Second, among the limited studies that have attempted to examine EKC in West Africa, the main focus is on whole sample analysis, ignoring heterogeneity in the region. Therefore, we decompose the 16 West African countries into their respective income levels (LICs and LMICs) based on World Bank categorization to address heterogeneity problems found in earlier studies. This may clarify the mixed results of the EKC literature in West Africa. Finally, in contrast to Keho (2015), Ogundipe et al. (2015), Wasiu and Alasinrin (2015), and Omojolaibi (2010) who used “first-generational” econometric approaches (to examine the topic in West Africa), which cannot perform well in heterogeneous and cross-sectional dependence, we use “second-generational” econometric techniques, which are robust to cross-sectional dependence and slope heterogeneity and provide reliable results.
The remainder of the paper was set out as follows: “Literature” focused on the literature review. “Methodology” dealt with methodology, while “Results” presented the results and discussion. Finally, “Conclusions” presented the conclusions.
Literature
The quest for economic advancement and reducing global warming and climate change continues to attract attention. Djellouli et al. (2022) explored the relationship between renewable energy consumption (REC), non-REC, GDP, and foreign direct investment (FDI) on CO2 using 20 selected African countries from 2000 to 2015. The authors employed pooled mean group (PMG), dynamic fixed effect (DFE), and mean group (MG) estimators to investigate the topic. GDP, non-REC, and FDI had a significant positive influence on CO2, while REC had a negative influence on CO2 among the selected African countries. Their findings were consistent with Akadiri et al. (2019), Mensah et al. (2019), Destek and Sinha (2020), Khan et al. (2021), and Dimnwobi et al. (2021) findings that found a positive and monotonic relationship between GDP and CO2. A recent study by Xue et al. (2022) also found that GDP increases CO2 in France. It is important to note that while these studies are consistent in their findings, they fail to explore the possible existence of the EKC hypothesis in their data. While GDP is expected to increase CO2 at some point, further economic expansion may reduce CO2 due to changes in lifestyle and the quest for improvement in the environment (Li and Li 2021). Thus, the EKC hypothesis cannot be ignored in current environmental studies.
Since Grossman and Krueger (1991) expounded the EKC hypothesis to explain that the relationship between income and environment exhibits an inverted U-shaped curve, many scholars have attempted to explore this argument further (Adu and Denkyirah 2017; Ahmad, et al. 2020; Liang and Yang 2019; Wang et al. 2021; Li et al. 2022). Using panel data from 30 provinces in China from 2006 to 2015, Liang and Yang (2019) established an inverted U-shaped relationship between GDP and environmental pollution. Although Liang and Yang (2019) established that EKC exists in China, their sample period is too short (lacks a time element), which is consequential to policy decisions. Hence, the results may change using a relatively large sample period, which is usually recommended. Using a long panel data (1980–2014) of 24 Organisation for Economic Co-operation and Development (OECD) countries, Destek and Sinha (2020) established a U-shaped relationship between GDP and EF, thus rejecting the EKC hypothesis. To understand the contradictions reported by Liang and Yang (2019) and Destek and Sinha (2020), Li et al. (2022) explored the EKC hypothesis among 89 Belt and Road Initiative (BRI) countries from 1995 to 2017. The study found that BRI collectively exhibits an inverse U-shaped relationship (EKC) between GDP and ED. However, in the region-specific results, EKC was valid in Asia, Pacific, and Latin American countries but invalid in MENA and SSA countries. Using a global sample, Wang et al. (2022a, b) applied a threshold regression model on panel data from 134 countries from 1996 to 2015 to study the effect of URB, natural resource rents, population aging, REC, trade openness, and GDP on environmental quality. The study found that whereas trade openness and natural resource rents worsen environmental quality, population aging and REC improve it. Furthermore, URB strengthens the positive link between GDP, CO2, and EF. GDP had a more beneficial impact on CO2 and EF. Accounting for heterogeneity in their study, an inverted U-shape was established in LMICs, while a U-shaped relationship was established in high-income countries. Additionally, Li et al. (2021a, b) investigated the impact of structural changes on CO2 emissions from the economy, trade, energy, and society using cointegration regressions on a panel data set of 147 nations from 1990 to 2015. The findings revealed that on a worldwide scale, GDP and economic structure had positive and negative effects on CO2. At the income group level, consistent with the global results, GDP and energy intensity increased escalated CO2, whereas REC reduced it. These divergent results suggest that EKC should be best viewed from regional perspectives.
From a single-country perspective, Alshehry and Belloumi (2017) reported a monotonically increasing relationship between GDP and ED in Saudi Arabia. In Indonesia, Saboori et al. (2012) used the ARDL model using annual data from 1971 to 2007 to study the dynamic link between CO2, GDP, EC, and foreign trade in Indonesia. The study, contrary to the EKC theory, discovers a U-shaped association between GDP and ED. Twerefou et al. (2016) studied the link between GDP and CO2 in Ghana using the autoregressive distributed lag (ARDL) bound testing technique using yearly data from 1970 to 2010. The authors discovered a U-shaped association between per capita GDP and CO2, showing that the EKC hypothesis for CO2 does not exist in Ghana. Minlah and Zhang (2021) used vector autoregressive models to test the presence of the EKC hypothesis for CO2 in Ghana. The empirical data demonstrated an upward sloping link between GDP and CO2 in Ghana. Aiyetan and Olomola (2017) looked at the link between CO2, GDP, population growth, and energy consumption (EC) in Nigeria. The authors applied the ARDL bounds testing technique to a time series data set spanning 1980 to 2012. The empirical data demonstrated that in the long term, EC and population growth increased CO2 emissions, but the EKC hypothesis was not validated. In contrast, Bekun, Agboola, and Joshua (2020) used yearly data from 1971 to 2015 to investigate the trade-off between GDP and CO2 in Nigeria. According to the ARDL regression, EC and GDP increase CO2 emissions in Nigeria. The current analysis verifies the EKC hypothesis in the Nigerian situation. Sarkodie and Ozturk (2020) examined the validity of EKC for CO2 in Kenya from 1971 to 2013 using the ARDL, statistically inspired modification of partial least squares (SIMPL) regression, and U test method confirmed EKC in Kenya. In a recent study, Liu et al. (2022) investigated the EKC hypothesis for ecological footprint and tourism-led-growth in Pakistan. Using time-series data from 1980 to 2017 and the ARDL bound model, the empirical analysis revealed an inverted U-shaped relationship supporting the EKC hypothesis for ecological footprint. The results from the ARDL model and the U test estimation validated the EKC hypothesis for CO2 in Kenya. Destek and Sarkodie (2019) also validated the EKC hypothesis in Singapore.
In West Africa, the few studies that have investigated the existence of the EKC hypothesis have been inconclusive. Halliru et al. (2020) used annual data from 1970 to 2017 to explore the relationship between GDP and CO2. The quantile regression results revealed a U-shaped relationship between GDP and CO2, rejecting EKC among selected West African countries. Similarly, Keho (2015) and Ogundipe et al. (2015) revealed that EKC is not valid in West Africa. On the contrary, Wasiu and Alasinrin (2015) supported the EKC hypothesis, while Omojolaibi (2010) found evidence of the EKC hypothesis from pooled OLS regression but not FE regression among selected West African countries. Similarly, Leonard and David (2016) used fixed effect and rand effect models to investigate the relationship between GDP and the environment for the period 1980–2012. The study provided evidence to support the EKC hypothesis in the region. Additionally, Oshin and Ogundipe (2014) found the validity of the EKC theory in West Africa for the period 1980–2012. The empirical results from the random effect model supported the theory of EKC theory in West Africa. The diversity of EKC results in West Africa could be due to different methodological frameworks such as selective sample, period, econometric modeling, and failure to account for heterogeneous growth patterns in West Africa. For example, the FE and RE models do not account for stationarity and cointegration that are present in growth-environmental studies. As a result, the validity and reliability of the FE and RE findings without considering stationarity and order of integration are not guaranteed. Another important weakness found in these studies is that West Africa consists of heterogeneous countries — LMICs and LICs. However, studies testing EKC in West Africa overlook these two heterogeneous groups, thus resulting in a heterogeneity problem in earlier studies. To address this gap, our study considers all the 16 West African countries while accounting for the heterogeneous groups in the region. In addition, our study uses up-to-date data on the variables to provide fresh insight into the topic in the West African region.
Globally, research outcomes on growth-environmental relationships can be categorized into a monotonic relationship (Bekhet et al. 2017; Mensah et al. 2021; Xue et al. 2022), U-shaped relationship (Destek and Sinha 2020; Xu et al. 2020), inverted U-shaped relationship (Jebli et al. 2016; Dogan and Inglesi-Lotz 2017; Sarkodie et al. 2020), N or inverted N-shaped relationship (Halkos and Polemis 2017; Zeraibi et al. 2021), bi-directional relationship (Gorus and Aydin 2019; Gyamfi et al. 2021), and mixed outcomes in studies where the samples are decomposed into sub-samples (Ntarmah et al. 2022). Table 1 summarizes growth-environmental studies in terms of sample/study area, period, methods, variables, and key findings. The literature summary in Table 1 brings to light why there is growing interest in growth-environmental studies.
Methodology
Theoretical rationale and model construction
Before setting out the econometric framework for exploring the relationship between GDP and ED, we provide a theoretical rationale guiding the selection of the variables of the study. ED which is the outcome variable is widely measured using CO2 (Akadiri et al. 2019; Ntarmah et al. 2020; Kong et al. 2020; Aslam et al. 2021; Shabani et al. 2021; Ntarmah et al. 2021). GDP is selected for the study due to the theoretical proposition of EKC that put forward three channels (scale, composition, and technique effects) through which GDP affects ED (Kurznets 1955; Mitić et al. 2019; Halliru et al. 2020). The scale effect indicates that GDP increases ED due to higher production volumes that require greater exploitation of natural resources and harmful emissions (Owusu and Asumadu-Sarkodie 2016; Sarkodie and Strezov 2019).). The composition effect implies that GDP could improve or worsen the environment, subject to the structural changes in the economy (Mitić et al. 2019). As a country’s income rises, a structural shift in the economy from agricultural to industrial activity raises ED, whereas a shift from energy-intensive industries to services and knowledge-based innovations and technologies lowers ED. Finally, the technique effect suggests that GDP reduces ED because higher income levels lead to more investment in research and development, which results in new and environmentally friendly technologies that replace old and highly polluting technologies, as well as strict environmental regulations and industry standards (Komen et al. 1997). The empirical findings of Dogan and Inglesi-Lotz (2017), Jebli et al. (2016), and Sarkodie et al. (2020) support the EKC theoretical position.
Theoretically, growth-environmental studies require certain key determinants to be controlled to minimize model uncertainties. According to the literature (Ntarmah et al. 2021; Ullah et al. 2021), ED decreases when people shift away from consumption of traditional energy sources to renewable energy sources such as wind and solar power, biomass, and hydropower. This is because the production of these energy sources creates little or no greenhouse gas emissions. According to the International Renewable Energy Agency (IRENA 2022), West Africa possesses sufficient renewable energy reserves to assist the region’s transition to a low-carbon growth path. As a result, there is a gradual shift from traditional energies, which are highly pollutant to renewable energies. Among many renewable energy initiatives, the Economic Community of West African States (ECOWAS) enacted the ECOWAS Renewable Energy Policy (EREP) in 2012, to increase the region’s entire electricity mix’s contribution to renewable energy to 35% by 2020 and 48% by 2030 (IRENA 2022; Karin 2014). In addition, there is a strong collaboration between ECOWAS’s Centre for Renewable Energy and Energy Efficiency (ECREEE), the ECOWAS Regional Electricity Regulatory Authority (ERERA), and the West African Power Pool (WAPP) to promote the development and integration of utility-scale renewable power into West African power systems (IRENA 2022). In keeping with West Africa’s clean energy corridor and the global need for alternative energy sources rather than traditional fossil-based ones, we chose REC as a determinant of ED. Scholars such as Destek and Sinha (2020), Rahman and Vu (2020), and Ntarmah et al. (2022) controlled REC in their studies. Besides, the conversion of Earth’s land surface to urban usage harms the environment. The environment of urban areas is polluted through anthropogenic activities such as the flying of a large number of automobiles, farming, and industrial activities that emit pollutants such as carbon dioxide, hydrocarbons, and toxic metals (Seto et al. 2011; Rai 2017; Zeeshan et al. 2022). As a result, we control for urbanization (URB) in this study. Scholars including Khan et al. (2021), Dimnwobi et al. (2021), and Xue et al. (2022) support this theoretical argument for selecting URB as an ED determinant. Similarly, industrialization (IND) intensifies ED by industrial pollutants. Highly polluting companies include thermal power plants, coal mines, cement, and steel emit smoke, gases, dust, and hazardous gas emissions. Although the level of IND in West Africa is low compared to many advanced countries, IND is a focus area in West Africa with many countries putting in place policies to boost IND in the region. For instance, Ghana has one district one factory (IDIF) initiative, which is boosting the industrial sector. The Nigerian Industrial Revolution Plan (NIRP) drives the Nigerian industrial sector. Again, the “Industry for Liberia’s Future” is the Liberian government’s policy framework for accelerating the development of a vibrant and competitive industrial sector in Liberia to produce jobs and long-term development for the nation. At the regional level, the West Africa Common Industrial Policy (WACIP) designed and adopted in 2010 aims at facilitating the industrialization and the promotion of quality standards in the region. Thus, IND becomes a key variable in determining ED in West Africa. In line with this, scholars including Wang and Su (2019) and Majeed and Tauqir (2020) who controlled for IND in growth-environmental studies have supported this position. As a result, in our model, we controlled IND. The factors (IND, URB, and REC) jointly controlled in this work were derived from academics such as Wang et al. (2018), Dong et al. (2019), Wang and Su (2019), Majeed and Tauqir (2020), Rahman and Vu (2020), Ntarmah et al. (2021), and Xue et al (2022).
Based on the theoretical arguments put forward, we proposed a function to estimate ED in a multivariate framework as:
where ED (environmental degradation) is the outcome variable and GDP (economic growth), REC (renewable energy consumption), URB (urbanization), and IND (industrialization) are the explanatory variables. Eq. 1 can be represented in a linear and panel data time series form as:
where \(\beta_1,\beta_2,\beta_3,and\;\beta_4\) are the coefficients of GDPit, RECit, URBit, and INDit, respectively. \({\alpha }_{i}\) denotes the time-invariant country-specific impact; \({\mu }_{it}\) is the stochastic white noise error term; i and t represent individual countries and periods respectively. We can log-linearize Eq. 2 as:
where \(\ln\;ED_{it},\;\ln\;GDP_{it},\ln\;REC_{it},\ln\;URB_{it},and\;\ln\;IND_{it}\) represent the log transformation of the variables of this study. Based on the theoretical argument presented by the EKC hypothesis, we can modify Eq. 3 to reflect the EKC hypothesis as follows:
The EKC model is represented by Eq. 4, where lnGDP2 is the quadratic form of GDP. For EKC to be validated, the coefficients \({\beta }_{1}\) and \({\beta }_{2}\) must be significantly positive and negative, respectively.
Variables, data source, and descriptive statistics
This study used annual data from 16 West African countries (comprising nine LICs and seven LMICs) for all the variables available from 1990 to 2018. The study sourced data (see Table 2) from World Bank through World Development Indicators (WDI).
Econometric approaches
Since the dataset for this study across the panel exhibits long panel data properties (time, T, is greater than the number of countries, N, in each dataset), a long panel data estimation procedure is recommended for estimating the results. This panel estimation procedure consists of the following six steps: (1) cross-sectional dependence (CD) testing, (2) slope homogeneity testing, (3) panel unit root estimation, (4) panel cointegration testing, (5) panel regression estimates, and (6) Granger causality test (Le and Sarkodie 2020; Ntarmah et al. 2021).
Cross-sectional dependence and slope homogeneity testing
Studies (Sarkodie et al. 2020; Mensah et al. 2021) have shown that there is a strong possibility that data from different countries will exhibit cross-sectional interdependence due to socioeconomic activities among them. Therefore, testing for CD and parameter heterogeneity serves as a reference for selecting appropriate panel econometric tests for estimating the findings. Ignoring cross-sectional associations may result in inaccurate calculations and conclusions (Li et al. 2021a, b). As a result, the study used CD among the Pesaran (2004) CD tests to identify the presence or absence of dependencies in the panels. Second, because the lack of knowledge about heterogeneity might lead to inaccurate estimations and extrapolations (Musah et al. 2021), the researchers examined the heterogeneity assumption using the Pesaran-Yamagata method (2008).
Panel unit root testing
This study utilized the Pesaran (2007) CIPS and CADF unit root test to test for the presence of unit roots in the data. The CIPS and CADF are second-generation unit root tests for heterogeneous panels with cross-section dependence (Le and Sarkodie 2020). Due to their appropriateness, the tests have been widely accepted and used to determine unit roots in several panel data studies (Ssali et al. 2019; Musah et al. 2020).
Panel cointegration testing
In this study, we used two-panel cointegration tests to test for cointegration. The first test is Persyn and Westerlund (2008) cointegration test. The test implements Westerlund’s (2007) four error correction-based tests. The second test is the Durbin-Hausman Panel Cointegration test by Westerlund (2008). This test implements two different tests — Durbin-Hausman panel (DHp) and Durbin-Hausman group (DHg) (Westerlund 2008). Both Westerlund’s (2007; 2008) tests were considered appropriate due to their suitability in handling CD and slope heterogeneity. In addition, the tests have proven to be reliable in panel cointegration estimation (Persyn and Westerlund 2008; Ntarmah et al. 2021).
Panel model estimations
To provide valid and reliable estimates, it is appropriate we consider panel data estimators that are robust to cross-sectional dependence, slope heterogeneity, unit roots, and cointegration in the data. Therefore, this study employed the Augmented Mean Group (AMG) and Common Correlated Effect Mean Group (CCEMG) estimators. The AMG and CCEMG estimators were chosen for this study due to the ability to produce efficient, robust, and reliable results in the presence of slope heterogeneity and cross-sectional dependence (Eberhardt and Bond 2009; Pesaran 2006; Eberhardt and Teal 2010; Apergis 2016). Scholars such as Li et al. (2020), Mensah et al. (2021), and Musah et al. (2021) have validated the appropriateness of AMG and CCEMG in the presence of cross-sectional dependence, slope heterogeneity, and cointegration in panel studies.
The CCEMG was developed by Pesaran (2006) and advanced by Kapetanios et al. (2011). Pesaran (2006) developed the CCEMG estimator based on the common correlated effect (CCE) function to be estimated then becomes:
where the dependent and independent variables are represented as \({y}_{it}\) and \({x}_{it}\), respectively. \({\beta }_{i}\) and \({\alpha }_{i}\) represent the slope and the heterogeneous fixed effects of each unit, respectively. \({f}_{t}\) and \({\varphi }_{i}\) represent the unobserved common effects and the heterogeneous factor loadings, respectively. The error term is represented as \({\varepsilon }_{it}\). Because our desire is in \({\beta }_{i}\), the estimates of coefficients, Pesaran and Smith (1995) and Pesaran (2006) indicated that the CCEMG estimator is the mean of the CCE estimator \({\widehat{\beta }}_{CCE,i}\) which can be expressed as:
where \({\widehat{\beta }}_{i}\) is the cross-sectional (or individual) coefficient computed from Eq. 5.
The AMG estimator was developed by Eberhardt and Bond (2009). Although both CCEMG and AMG are similar, the AMG estimator uses a 2-step calculation method. First, the AMG estimator introduces (1) the time dummies (D) and (2) the unobserved common factor in the first-difference OLS equation. This is expressed as:
where D and ∆ are the time dummies and first difference operator respectively. \(\gamma\) represents the coefficients of the time dummies. Second, the AMG estimates the slopes in each unit following the CCEMG mean estimation procedure outlined in Eq. 6, which is expressed as:
where \({\stackrel{\sim }{\beta }}_{i}\) represent the estimates \({\beta }_{i}\) in Eq. 7. While CCEMG and AMG estimators are both robust to cross-sectional dependent and parameter heterogeneity, Bond and Eberhardt (2013) demonstrate that AMG estimator is unbiased and efficient for various combinations of cross-section and time dimensions (Le and Sarkodie 2020).
Granger causality test
We employed the Dumitrescu and Hurlins (2012) (D-H) test to estimate the directional causal link between CO2, GDP, REC, URB, and IND across the panels. The D-H causality test was employed because it performs very well in the presence of slope heterogeneity and cross-sectional reliance (Dumitrescu and Hurlins 2012; Gyamfi et al. 2021). Using bootstrap options, the suggested D-H test is appropriate for assessing causalities in panel data. The test is based on the null hypothesis of no causation. The D-H panel Granger causality test’s underlying regression is expressed as:
where \({y}_{i,t}\) and \({x}_{i,t}\) are inter-related variables. The coefficients (\({\gamma }_{ik}\) and \({\beta }_{ik}\)) vary across individual observations under the time-invariant assumption. The lag order is denoted as K.
Taking into consideration the income heterogeneity among West African countries and the need to provide valid and reliable results, we used the following approaches to minimize heterogeneity problems in our study. First, we transformed the data into a natural log to decrease heteroscedasticity and improve statistical accuracy. Second, we sub-grouped the countries into their respective income levels — LICs and LMICs according to the World Bank categorization of countries into income levels. This does not only allow us to perform in-depth analysis but also conditions countries into their respective income levels to produce valuable results. Third, we implemented two second-generation panel data estimators that are verified to be reliable and suit the characteristics of the data as identified by the preliminary results. Thus, consistency between the two estimators proves the reliability of our results. Finally, we performed diagnostic statistics — the Wald chi2 and the RMSE checked the validity of the estimates.
Results
Descriptive statistics
Table 3 shows the descriptive statistics for the entire sample as well as the sub-samples. Table 3 reveals that there were 464 observations (from 16 countries) in West Africa, with 203 observations for LMICs and 261 observations for LICs. As such, studies that fail to account for this heterogeneity may provide unreliable results and mislead policies in the region. In terms of the variables, LMICs averagely has a higher GDP (M = 7.260, SD = 0.353), REC (M = 4.979, SD = 0.392), URB (M = 3.786, SD = 0.159), IND (M = 3.843, SD = 0.283), and ED (M = − 0.835, SD = 0.528) than GDP (M = 6.328, SD = 0.237), REC (M = 4.390, SD = 0.138), URB (M = 3.474, SD = 0.374), IND (M = 3.684, SD = 0.652), and ED (M = − 1.928, SD = 0.521) of LICs in West Africa. This shows that LMICs in West Africa are relatively at an advanced stage in terms of GDP, REC, URB, and IND than LICs in West Africa. Similarly, it is clear from the descriptive statistics that LMICs highly pollute the West African environment than LICs. These descriptive depict that growth-environmental outcomes in West Africa vary among LMICs and LICs. Consequently, it is important when studying this topic, authors consider the respective income levels in West African countries to avoid misleading results.
Concerning skewness, GDP is positively skewed in LMICs and the whole sample, while it is negatively skewed in LICs. ED and REC are negatively skewed across the samples. While URB and IND are negatively skewed in LICs and the whole sample, it is positively skewed in LMICs. Regarding kurtosis, the results show that the data is leptokurtic (kurtosis value greater than 3) except ED, which is platykurtic (kurtosis value less than 3) for all samples. The descriptive results generally show that the data is not normally distributed. This is further confirmed by the Jacque-Bera and probability results. Hence, heterogeneous panel data models will be appropriate for estimating the results (Li et al. 2021a, b).
Multicollinearity results
In addition to the descriptive statistics, this study performed multicollinearity analysis to detect possible multicollinearity problems. Table 4 presents the multicollinearity results. According to Table 4, the variables moderately correlated with each other suggesting associations among them. This provides an initial justification for studying the influence of the explanatory variables on the outcome variables. Furthermore, the collinearity statistics-tolerance (Tol) values greater than 0.2 and variance inflation factor (VIF) values less than 5 across the samples indicate that multicollinearity is not a problem in this study.
Cross-sectional dependency and slope homogeneity testing
Under the null hypothesis of no CD, Table 5 shows that all variables have significant, suggesting CD across West African countries as well as LICs and LMICs in the region.
In addition, the Pesaran and Yamaga (2008) test results in Table 5 reject the null hypothesis of homogeneity in slope coefficient for all countries, LMICs, and LICs in West Africa. Based on the results, econometric methods implemented in this study must be robust to cross-sectional dependence and slope heterogeneity to account for any potential distortions in the series (Musah et al. 2020; Mensah et al. 2021).
Panel unit root results
In this study, we used CIPS and CADF, which are robust to the series’ established CD. According to the CIPS and CADF results in Table 6, the variables are not stationary at a level across the countries and income levels. However, they are stationary at first differences in all unit root test specifications. This indicates that all of the variables utilized in the estimation are of order one integration, I(1).
Panel cointegration results
Table 7 presents Westerlund’s (2007; 2008) cointegration results of the relationship for all countries, LMICs, and LICs in West Africa. Westerlund (2007; 2008) test the null hypothesis of no cointegration. Table 7 shows that the null hypothesis is rejected, indicating that cointegration exists throughout the panels. The findings in Table 7 further support the use of panel econometric models that account for cointegration.
AMG and CCEMG results
For consistency of the estimates, the AMG and CCEMG estimators were used to estimate the results. In Table 8, the AMG and CCEMG results show that GDP had a significant and positive impact on ED among West African countries. Similarly, GDP had positive and significant effects on the ED of LICs and LMICs in West Africa. This implies that GDP worsens ED in the region. This positive effect of GDP on ED implies that initial economic expansion causes more environmental harm in West Africa by emitting high CO2 into the environment. This finding contradicts the findings of Ntarmah et al. (2021) who found GDP improves environmental quality in Central Africa and Bekhet et al. (2017) who revealed economic expansion in GCC decreases ED. On the other hand, this study provides evidence to support the studies of Akadiri et al. (2019), Destek and Sinha (2020), Khan et al. (2021), and Dimnwobi et al. (2021) who revealed higher GDP dampens the environment. This usually happens when the scale effect is in place such that increasing GDP requires higher volumes of natural resource extraction associated with high pollution, which damages the quality of the environment (Gill et al. 2018; Mensah et al. 2019; Ntarmah et al. 2021). According to the results, regardless of the country’s income status (i.e., LMIC or LIC) in West Africa, the increase in production and use of goods, as well as changes in peoples’ consumption (e.g., Non-renewable energy sources), is associated with high pollution (Sarkodie and Strezov 2019).
On the other hand, REC had a significant negative effect on ED for all West African countries as a whole, as well as LICs and LMICs in the region. This finding indicates that REC decreases ED in West Africa by reducing CO2 in the region. This is consistent with Destek and Sinha (2020) and Ullah et al. (2021) who found REC improves the environment. Thus, the quality of the West African environment improves as people shift toward renewable energy sources such as wind power, solar power, biomass, and hydropower, which generate little or no greenhouse gas emissions as against traditional energy sources that are highly pollutants (Ehigiamusoe and Dogan 2022). As a result, growth-environmental improvement through emissions reduction involves a paradigm shift from non-renewable energy to renewable energy technologies such as renewables, carbon capture, and improving energy efficiency, among other things (Owusu and Asumadu-Sarkodie 2016). According to Liobikienė and Butkus (2018), one of the most significant climate policy potentials for mitigating ED is to promote renewable and improved energy efficiency. Furthermore, URB and IND had significant positive controlling effects on ED across the panels, suggesting URB and IND expansions are detrimental to the West African environment. The study provides evidence to support Wang and Su (2019) and Wang et al. (2018) who found IND and URB upsurges in ED in China and India. Thus, pollution rises rapidly through industrialization and urbanization that focus on increasing material production and conversing of the earth's surface into urban places with little to its effects on the environment (Dasgupta et al. 2002; Xue et al. 2022). In terms of diagnostic statistics, the Wald chi2 values are significant at the 1% level, suggesting that the distribution of the variables fits well with the CO2 model. Similarly, the RMSE values ranging from 0.026 to 0.049 are far lower than the recommended 0.08 (Hair et al. 2017). Thus, the RMSE results show that the models have high predictive power.
EKC results
Table 9 presents the EKC results from the AMG and CCEMG estimators. Using the West African countries as a whole, the results in Table 9 show that the coefficient of the linear term of GDP is positive and significant, while the coefficient of the quadratic term of GDP (GDP2) is negative and significant. This indicates an inverted U-shaped relationship between GDP and ED, validating the EKC hypothesis in West Africa. Similarly, the results from the perspective of LMICs in West Africa show that the coefficient of GDP is significant and positive, while GDP2 is significant and negative. Thus, the EKC hypothesis is validated among LMICs in West Africa. While this finding contradicts the outcomes of Keho (2015) and Ogundipe et al. (2015) who could not reveal evidence to support EKC’s existence in West Africa, it supports Wasiu and Alasinrin (2015) and Omojolaibi (2010) that supported EKC in West Africa. The evidence of the EKC hypothesis among West African countries especially LMICs implies that ED caused by economic expansion through scale effect can be corrected in later stages of economic expansion by composition and technique effect (Adu and Denkyirah 2017; Halliru et al. 2020; Ntarmah et al. 2021). Thus, air pollution initially increases with a country’s income level until a certain point where further increase in income level leads to strict environmental regulations, industry standards, and investment in research and development resulting in new, cleaner, and environmentally friendly technologies to replace old technologies that emit large quantities of pollution. Although GDP in West Africa is at its early stages of growth, West African countries have introduced several policies in line with global efforts in achieving environmental sustainability and carbon neutrality. Sorgho et al. (2020), for example, discovered that 15 countries have at least one national policy document on climate change and that 19 policy documents cover seven key sectors (health, agriculture, infrastructure, water resources, forestry, energy, and education) and eight thematic areas (research and development, community resilience, industry development, disaster risk management, institutional development, policymaking, and education). At this point, integrating environmental awareness in economic expansion and demand for a quality and sustainable environment increases (Beckerman 1992; Komen et al. 1997; Liang and Yang 2019; Li et al. 2022) is a major concern to LMICs in West Africa.
In contrast, the coefficients of GDP and GDP2 in LICs in West Africa are both positive, suggesting a monotonic positive relationship. Thus, there is a monotonic increasing curve established in LICs. The monotonic relationship between GDP and ED among LICs implies that further economic expansion causes more environmental harm for LICs, sometimes at a decreasing rate (Mitić et al. 2019). The EKC hypothesis may not apply to the LICs in West Africa since their early stages of economic growth were defined by the dominance of agriculture and labor-intensive techniques of production, which is associated with lower ED (Halliru et al. 2020) as compared to developed nations. However, these nations are increasingly becoming more urbanized and industrialized, signaling a shift toward the second stage of economic expansion and its consequence on ED. This shows that continuously increasing ED is associated with increasing income, suggesting that new sources of ED will keep emerging along with economic development creating pressure on the environment (Stern 2004). Countries at these stages of economic advancement are too poor to invest in improving the environment and neglect the consequences GDP has on the environment (Sarkodie and Strezov 2019). Generally, the findings of this study support He et al. (2021), Ntarmah et al. (2022), and Tachega et al. (2021) who revealed mixed results in terms of EKC existence. Particularly, the results support Li and Li’s (2021) findings that established EKC in high-income countries but not LICs. The likeness of AMG and CCEMG shows that the results are reliable. The Wald chi2 and RMSE values suggest that the distribution of the variables fits well with the CO2 model and has high predictive power.
Causality results
As indicated earlier, the D-H Granger causality test was conducted to explore the causalities among the variables. The study explored causalities for the whole sample and the respective income groups. Table 10 presents the results of the Granger causality tests.
The D-H causality results in Table 10 show that there are bidirectional causal links between GDP and ED in all countries, LMICs, and LICs in West Africa. This implies that while GDP influences ED depending on the economic structure and stages of economic development, increased pollution could bring down productivity, reducing crop yield and influencing biodiversity and ecosystems health. The findings support the Gorus and Aydin (2019), Gyamfi et al. (2021), and Rahman and Vu (2020) studies that established a bidirectional relationship between GDP and environmental variables. Similarly, there are bidirectional causal links between ED and IND for all countries, LMICs, and LICs in West Africa, suggesting that negative externalities such as pollution from IND have an impact on the future growth of industries in West Africa. On the other hand, a unidirectional causal link from REC to ED and URB to ED exists among all countries, LMICs, and LICs in West Africa. Thus, renewable energy sources such as wind power, solar power, biomass, and hydropower decrease ED (Destek and Sinha 2020), while the conversion of the Earth’s surface into urban forest damages the environment (Dimnwobi et al. 2021; Xue et al. 2022). However, the feedback from ED to URB and REC was inconsequential. Generally, the findings of this study support Ntarmah et al. (2022), Mensah et al. (2021), and Musah et al. (2020) who found mixed causal links between environmental variables and their determinants.
Furthermore, bidirectional causal links exist between REC and GDP in all countries, LMICs, and LICs in West Africa. Accordingly, REC is a significant driver of GDP, however, an increase in GDP inspires higher REC from renewable sources. Similarly, bidirectional causal links between GDP and URB exist across all the samples. While bidirectional causal links exist between IND and GDP among the whole West African countries and LMICs in West Africa, a unidirectional causal link from IND to GDP exists in LICs. Generally, the result implies that IND increases GDP through production activities. In turn, high GDP provides opportunities for industrial growth. There is a unidirectional causal link from URB to REC in LICs, LICs, and West African countries as a whole. Therefore, URB could increase the consumption of REC because of the increasing urban population. Logically, the growing urban population could increase household consumption of REC, especially where many societies are now advocating for a shift from traditional energy sources to renewable energy sources (Zeeshan et al. 2022). There is a unidirectional causal link from REC to IND for all countries, LMICs, and LICs in West Africa. This suggests that renewable energy sources such as wind and sun, which may be captured utilizing technologies are becoming useful in industrial growth strategies in West Africa. Although bidirectional causal links between URB and IND exist among LICs and West African countries as a whole, the feedback from IND to URB is significant at 10% significance levels. This means that the feedback mechanism is weak in statistical power. IND promotes URB by generating economic development and job possibilities that entice people to live in cities. The process of URB often begins when a factory or numerous factories are created within a region, producing a high need for manufacturing workers. Generally, the causality results support the results of Wang et al. (2018), Dong et al. (2019), Wang and Su (2019), Majeed and Tauqir (2020), Musah et al. (2020), Rahman and Vu (2020), Ntarmah et al. (2021), and Xue et al (2022) who established causalities among URB, REC, GDP, IND, and environmental indexes. Respectively, Figs. 1, 2, and 3 show graphical illustrations of the causalities for all West African countries, LMICs, and LICs in West Africa.
Conclusions
The global concern about the relationship between growth and the environment, as well as the need to understand country growth models and their consequences on the environment, continues to interest scholars and policymakers. In West Africa, a few studies have examined the potential of the countries benefiting from the EKC proposition, but the results have been mixed due to different methodological frameworks such as selective sample, time span, econometric modeling, and failure to account for heterogeneous growth patterns in West Africa. Addressing this gap, this study investigates the relationship between GDP and ED and establishes whether West African countries could benefit from the theoretical argument put forward by the EKC hypothesis. REC, IND, and URB are controlled in this study to understand their roles in environmental degradation in West Africa as well as minimizing their impacts on the growth-environmental studies. We selected panel data from all the 16 West African countries for the period 1990–2018. We applied heterogeneous panel data approaches and second-generational econometric techniques (AMG and CCEMG estimators) that are robust to cross-sectional dependence and slope heterogeneity. We established that the data from the West African countries are cross-sectionally dependent, heterogeneous, integrated of order one, I(1), and cointegrated.
Our empirical results from AMG and CCEMG revealed the following. First, GDP upsurges ED in West Africa. These positive effects of GDP on ED exist in both LICs and LMICs in West Africa. However, the impact is greater in LMICs than LICs suggesting a stronger connection between GDP and ED in LMICs than LICs in West Africa. Second, the EKC hypothesis is valid in West Africa implying that West African countries collectively could benefit from EKC arguments. By comparison, EKC is valid only in LMICs of West Africa but not LICs in West Africa. Rather, an adverse monotonic relationship between GDP and ED in LICs in West Africa. This comparison is very useful to guide policy directions for the two income groups in West Africa. Third, REC reduces ED in West Africa as well as LICs and LMICs in the region, supporting the global shift from non-renewable energy to renewable energy as a means to curb ED. Fourthly, both URB and IND had detrimental effects on ED across the panels. Finally, there are bidirectional relationships between GDP and ED. This feedback mechanism exists in both LICs, and LMICs in West Africa supporting the view that GDP and the West African environment are interconnected. We can conclude that West African countries could collectively benefit from the EKC proposition when the region’s growth model integrates environmental mitigation approaches and eco-friendly initiatives.
Based on the findings, the following recommendations focusing on improving the relationships between the environment and its determinants are offered.
-
To start with GDP and ED, GDP was a substantial positive predictor of ED in West Africa. This conclusion implies that the nations’ economic activities have contributed to increasing ED. As a result, if governments do not work to deploy alternative energy sources and energy-saving technology, their goal of achieving both economic development and an environmentally friendly economy would be thwarted. This study recommends ECOWAS and country-level policymakers in the region that increasing GDP should be backed by environmentally friendly policies to mitigate against ED associated with economic expansion. While LMICs are encouraged to further strengthen environmentally friendly policies within the growth-environmental framework, LICs are encouraged to engage in restructuring economic expansion towards more environmentally friendly initiatives to put them on EKC pathways to the growth-environmental outcome. This will ensure that the feedback mechanism between GDP and the environment will be mutually beneficial.
-
REC reduces ED, implying that a shift from traditional energy sources to renewable energy sources will improve the environment of West Africa. This finding throw more support to the West African countries policy shifts from traditional energy to renewable energy as a means to improve the environment. We urge that governments in West Africa increase their investments in renewable energy sources. The investment could be infrastructure investment via green job creation for the clean production process and a shift from electricity consumption to hydropower, wind energy, and solar energy leading to the transition to renewable energy. Uniform policies to promote REC should be formulated in the region and regulated by ECOWAS.
-
URB had a positive influence on ED. This implies that URB spanning rural–urban migration and anthropogenic activities such as the flying of a large number of automobiles, the conversion of Earth’s land surface to urban usage, and industrial activities increase ED in West Africa. This study recommends that policymakers should focus on redeveloping rural areas to curb rural–urban migration. The concept of green and sustainable urbanization could help minimize the adverse influence of urbanization on the environment. A sustainable urban concept that incorporates eco-friendly practices, green spaces, and supporting technology into the urban environment to reduce ED and enhance air quality.
-
The positive influence of IND on ED implies that the activities of highly polluting companies such as steel emit smoke, thermal power plants, gases, and dust destroying the West African environment. However, this should not suggest that industrialization should be stopped. The industry could restructure its production processes to minimize ED by focusing on energy efficiency, fuel switching, combined heat and power, renewables, and more efficient use and recycling of materials. Reuse materials, such as production scrap or shop towels, rather than putting them into the waste stream. Governments should encourage industry standards and have an environmental compliance plan that promotes environmentally-friendly industrial practices. Alternatively, the introduction of the Carbon Tax and other environmental taxes such as “polluter pay tax” to deter highly environmentally damaging businesses could contribute to reducing ED.
Although the findings are robust and consistent, this study has some limitations. First, while inferences may be derived from this study to other jurisdictions, it is vital to note that the findings of this study are only applicable to West African countries because the sample only included West African countries. Second, it is important to recognize that the sub-sample analyses considered LMICs and LICs in West Africa; they did not consider the individual countries in the region. Therefore, policy decisions based on the study should take into account the aggregate growth of West African countries as well as specific income groups, rather than individual countries. Finally, the analysis failed to establish the turning point for West African countries concerning the EKC concept. As a result, future studies should seek to define thresholds at which GDP begins to improve the West African environment and compare it with other economic regions in Sub-Saharan Africa.
Data availability
The datasets generated and/or analyzed during the current study are available in the World Development Indicators repository, https://data.worldbank.org/
References
Adu DT, Denkyirah EK (2017) Economic growth and environmental pollution in West Africa: testing the environmental Kuznets curve hypothesis. Kasetsart J Soc Sci 1-8. https://doi.org/10.1016/j.kjss.2017.12.008
Ahmad M, Jiang P, Majeed A, Umar M, Khan Z, Muhammad S (2020) The dynamic impact of natural resources, technological innovations and economic growth on ecological footprint: an advanced panel data estimation. Resour Policy 69:101817
Akadiri SS, Bekun FV, Sarkodie SA (2019) Contemporaneous interaction between energy consumption, economic growth and environmental sustainability in South Africa: what drives what? Sci Total Environ 686:468–475
Aiyetan IR, Olomola PA (2017) Environmental degradation, energy consumption, population growth and economic growth: does environmental Kuznets curve matter for Nigeria? Econ Pol Rev 16
Alshehry AS, Belloumi M (2017) Study of the environmental Kuznets curve for transport carbon dioxide emissions in Saudi Arabia. Renew Sustain Energ Rev 75:1339–1347
Al-Mulali U, Solarin SA, Ozturk I (2016) Investigating the presence of the environmental Kuznets curve (EKC) hypothesis in Kenya: an autoregressive distributed lag (ARDL) approach. Nat Hazards 80(3):1729–1747
Apergis N (2016) Environmental Kuznets Curves: new evidence on both panel and country level CO2 emissions. Energy Econ 54:263–271
Aslam B, Hu J, Ali S, AlGarni TS, Abdullah MA (2021) Malaysia’s economic growth, consumption of oil, industry and CO2 emissions: evidence from the ARDL model. Int J Environ Sci Technol 1–2. https://doi.org/10.1007/s13762-021-03279-1
Bazilian M, Onyeji I, Aqrawi PK, Sovacool BK, Ofori E, Kammen DM, Van de Graaf T (2013) Oil, energy poverty and resource dependence in West Africa. J Energy Nat Resour Law 31(1):33–53
Beckerman W (1992) Economic growth and the environment: whose growth? Whose environment? World Dev 20(4):481–496
Bekhet HA, Matar A, Yasmin T (2017) CO2 emissions, energy consumption, economic growth, and financial development in GCC countries: Dynamic simultaneous equation models. Renew Sustain Energy Rev 70:117–132
Bekun FV, Agboola MO, Joshua U (2020) Fresh insight into the EKC hypothesis in Nigeria: accounting for total natural resources rent. In: Shahbaz M, Balsalobre-Lorente D (eds) Econometrics of green energy handbook. Springer, Cham. https://doi.org/10.1007/978-3-030-46847-7_11
Bond S, Eberhardt M (2013) Accounting for unobserved heterogeneity in panel time series models. University of Oxford, pp 1–11
Charfeddine L, Kahia M (2019) Impact of renewable energy consumption and financial development on CO2 emissions and economic growth in the MENA region: a panel vector autoregressive (PVAR) analysis. Renew Energy. https://doi.org/10.1016/j.renene.2019.01.010
Coulibaly SN (2016) Do West African economic and monetary union member countries have as unique environmental Kuznets curve (EKC)? J Sustain Dev Afr 18(4):20–38
Dasgupta S, Laplante B, Wang H, Wheeler D (2002) Confronting the environmental Kuznets curve. J Econ Perspect 16(1):147–168
Destek MA, Sarkodie SA (2019) Investigation of environmental Kuznets curve for ecological footprint: the role of energy and financial development. Sci Total Environ 650:2483–2489
Destek MA, Sinha A (2020) Renewable, non-renewable energy consumption, economic growth, trade openness and ecological footprint: evidence from organisation for economic Co-operation and development countries. J Clean Prod 242:118537
Dimnwobi SK, Ekesiobi C, Madichie CV, Asongu SA (2021) Population dynamics and environmental quality in Africa. Sci Total Environ 797:149172
Djellouli N, Abdelli L, Elheddad M, Ahmed R, Mahmood H (2022) The effects of non-renewable energy, renewable energy, economic growth, and foreign direct investment on the sustainability of African countries. Renew Energy 183:676–686
Dogan E, Inglesi-Lotz R (2017) Analyzing the effects of real income and biomass energy consumption on carbon dioxide (CO2) emissions: empirical evidence from the panel of biomass-consuming countries. Energy 138:721–727
Dong F, Wang Y, Su B, Hua Y, Zhang Y (2019) The process of peak CO2 emissions in developed economies: a perspective of industrialization and urbanization. Resour Conserv Recycl 141:61–75
Dumitrescu EI, Hurlin C (2012) Testing for Granger non-causality in heterogeneous panels. Econ Model 29:1450–1460
Eberhardt M, Bond S (2009) Cross-section dependence in nonstationary panel models: a novel estimator," MPRA Paper 17692. University Library of Munich, Germany
Eberhardt M, Teal F (2010) Productivity analysis in global manufacturing production. DEGIT Conference Papers c015_019, DEGIT, Dynamics, Economic Growth, and International Trade
Ehigiamusoe KU, Dogan E (2022) The role of interaction effect between renewable energy consumption and real income in carbon emissions: evidence from low-income countries. Renew Sustain Energy Rev 154:111883
Gill AR, Viswanathan KK, Hassan S (2018) The environmental Kuznets curve (EKC) and the environmental problem of the day. Renew Sustain Energy Rev 81:1636–1642
Gorus MS, Aydin M (2019) The relationship between energy consumption, economic growth, and CO2 emission in MENA countries: causality analysis in the frequency domain. Energy 168:815–822
Grossman GM, Krueger AB (1991) Environmental impacts of a North American free trade agreement. National Bureau of Economic Research Working Paper 3914, NBER, Cambridge
Gyamfi BA, Adedoyin FF, Bein MA, Bekun FV (2021) Environmental implications of N-shaped environmental Kuznets curve for E7 countries. Environ Sci Pollut Res: 1–11
Hair JF, Sarstedt M, Ringle CM, Gudergan SP (2017) Advanced issues in partial least squares structural equation modeling. SAGE Publications, CA
Halkos GE, Polemis ML (2017) Does financial development affect environmental degradation? Evidence from the OECD countries. Bus Strat Environ 26(8):1162–1180
Halliru AM, Loganathan N, Hassan AAG, Mardani A, Kamyab H (2020) Re-examining the environmental Kuznets curve hypothesis in the economic community of West African states: a panel quantile regression approach. J Clean Prod 276:124247
He L, Zhang X, Yan Y (2021) Heterogeneity of the environmental Kuznets curve across Chinese cities: how to dance with ‘shackles’? Ecol Ind 130:108128
Hu J, Chen, J, Zhu P, Hao S, Wang M, Li H, Liu N (2022) Difference and cluster analysis on the carbon dioxide emissions in China during COVID-19 lockdown via a complex network model. Front Psychol 12. https://doi.org/10.3389/fpsyg.2021.795142
International Renewable Energy Agency (2022) West Africa clean energy corridor. /Africa/West-Africa-Clean-Energy-Corridor. https://irena.org/africa/West-Africa-Clean-Energy-Corridor#:~:text=In%20July%202013%2C%20the%20Economic
Jamel L, Derbali A (2016) Do energy consumption and economic growth lead to environmental degradation? Evidence from Asian economies. Cogent Econ Finance 4(1):1–19
Jebli MB, Youssef SB, Ozturk I (2016) Testing environmental Kuznets curve hypothesis: the role of renewable and non-renewable energy consumption and trade in OECD countries. Ecol Ind 60:824–831
Kahia M, Ben Jebli M, Belloumi M (2019) Analysis of the impact of renewable energy consumption and economic growth on carbon dioxide emissions in 12 MENA countries. Clean Technol Environ Policy 21:871–885. https://doi.org/10.1007/s10098-019-01676-2
Kapetanios G, Pesaran MH, Yamagata T (2011) Panels with non-stationary multifactor error structures. J Econom 160(2):326–348
Karin R (2014) Developing renewable energy sectors and technologies in West Africa. United Nations. https://www.un.org/en/chronicle/article/developing-renewable-energy-sectors-and-technologies-west-africa
Keho Y (2015) An econometric study of the long-run determinants of CO2 emissions in Cote d’Ivoire. J Financ Econ 3(2):11–21
Khan I, Hou F, Le HP (2021) The impact of natural resources, energy consumption, and population growth on environmental quality: fresh evidence from the United States of America. Sci Total Environ 754:142222
Komen MH, Gerking S, Folmer H (1997) Income and environmental R&D: empirical evidence from OECD countries. Environ Dev Econ 2(4):505–515
Kong Y, Ntarmah AH, Cobbinah J, Menyah MV (2020) The impacts of banking system stability on sustainable development: conditional mean-based and parameter heterogeneity approaches. IJMAE 7(9):482–505
Le HP, Sarkodie SA (2020) Dynamic linkage between renewable and conventional energy use, environmental quality and economic growth: evidence from Emerging Market and Developing Economies. Energy Rep 6:965–973
Leonard AN, David U (2016) Economies of West Africa region and the environmental Kuznets curve: empirical assessment. Asian J Empirical Res 6(1):1–8
Li S, Shi J, Wu Q (2020) Environmental Kuznets curve: Empirical relationship between energy consumption and economic growth in upper-middle-income regions of China. Int J Environ Res Public Health 17(19):6971
Li S, Li R (2021) Revisiting the existence of EKC hypothesis under different degrees of population aging: empirical analysis of panel data from 140 countries. Int J Environ Res Public Health 18(23):12753. https://doi.org/10.3390/ijerph182312753
Li K, Hu E, Xu C, Musah M, Kong Y et al (2021a) A heterogeneous analysis of the nexus between energy consumption, economic growth and carbon emissions: evidence from the Group of Twenty (G20) countries. Energy Explor Exploit 39(3):815–837
Li R, Wang Q, Liu Y, Jiang R (2021b) Per-capita carbon emissions in 147 countries: the effect of economic, energy, social, and trade structural changes. Sustain Prod Consumption 27:1149–1164. https://doi.org/10.1016/j.spc.2021.02.031
Li W, Qiao Y, Li X, Wang Y (2022) Energy consumption, pollution haven hypothesis, and environmental Kuznets curve: examining the environment–economy link in belt and road initiative countries. Energy 239:122559
Liang W, Yang M (2019) Urbanization, economic growth and environmental pollution: evidence from China. Sustain Comput: Informatics Syst 21:1–9. https://doi.org/10.1016/j.suscom.2018.11.007
Liobikienė G, Butkus M (2018) The challenges and opportunities of climate change policy under different stages of economic development. Sci Total Environ 642:999–1007. https://doi.org/10.1016/j.scitotenv.2018.06.140
Liu HH, Ezekowitz MD, Columbo M, Khan O, Martin J, Spahr J, ... Kapelusznik L (2022) Testing the feasibility of operationalizing a prospective, randomized trial with remote cardiac safety EKG monitoring during a pandemic. J Interv Card Electrophy 63(2):345–356
Majeed MT, Tauqir A (2020) Effects of urbanization, industrialization, economic growth, energy consumption, financial development on carbon emissions: an extended STIRPAT model for heterogeneous income groups. Pakistan Journal of Commerce and Social Sciences (PJCSS) 14(3):652–681
Mensah IA, Sun M, Gao C, Omari-Sasu AY, Zhu D, Ampimah BC, Quarcoo A (2019) Analysis on the nexus of economic growth, fossil fuel energy consumption, CO2 emissions and oil price in Africa based on a PMG panel ARDL approach. J Clean Prod 228:161–174
Mensah IA, Sun M, Omari-Sasu AY, Gao C, Obobisa ES, Osinubi TT (2021) Potential economic indicators and environmental quality in African economies: new insight from cross-sectional autoregressive distributed lag approach. Environ Sci Pollut Res: 1–27
Minlah MK, Zhang X (2021) Testing for the existence of the environmental Kuznets curve (EKC) for CO2 emissions in Ghana: evidence from the bootstrap rolling window Granger causality test. Environ Sci Pollut Res 28:2119–2131. https://doi.org/10.1007/s11356-020-10600-x
Mitić P, Kresoja M, Minović J (2019) A literature survey of the environmental Kuznets curve. Econ Anal 52(1):109–127
Musah M, Kong Y, Mensah IA, Antwi SK, Donkor M (2020) The link between carbon emissions, renewable energy consumption, and economic growth: a heterogeneous panel evidence from West Africa. Environ Sci Pollut Res 27:28867–28889. https://doi.org/10.1007/s11356-020-08488-8
Musah M, Kong Y, Vo XV (2021) Predictors of carbon emissions: an empirical evidence from NAFTA countries. Environ Sci Pollut Res 28(9):11205–11223
Ntarmah AH, Kong Y, Gyan MK (2019) Banking system stability and economic sustainability: a panel data analysis of the effect of banking system stability on sustainability of some selected developing countries. Quantitative Finance and Economics 3(4):709–738
Ntarmah AH, Kong Y, Cobbinah E, Gyan MK, Manu EK (2020) Analysis of the responsiveness of environmental sustainability to non-performing loans in Africa. Appl Econ J 27(2):77–109
Ntarmah AH, Kong Y, Manu EK (2021) Investigating the dynamic relationships between credit supply, economic growth, and the environment: empirical evidence of sub-regional economies in Sub-Saharan Africa. Environ Sci Pollut Res 28(5):5786–5808
Ntarmah AH, Kong Y, Obeng AF, Gyedu S (2022) The role of bank financing in economic growth and environmental outcomes of sub-Saharan Africa: evidence from novel quantile regression and panel vector autoregressive models. Environ Sci Pollut Res: 1–39
Ogundipe AA, Olurinola OI, Odebiyi JT (2015) Examining the validity of EKC in Western Africa: different pollutants option. Environ Manag Sustain Dev 4(2):69–90
Omojolaibi JA (2010) Environmental quality and economic growth in some selected west african countries: a panel data assessment of the Environmental Kuznets Curve. J Sustain Dev Afr 12:35–48
Omri A, Daly S, Rault C, Chaibi A (2015) Financial development, environmental quality, trade and economic growth: what causes what in MENA countries. Energy Econ 48:242–325
Oshin S, Ogundipe A (2014) An empirical examination of environmental Kuznets curve (EKC) in West Africa. Euro-Asia Journal of Economics and Finance 3(1):1–16
Owusu PA, Asumadu-Sarkodie S (2016) A review of renewable energy sources, sustainability issues and climate change mitigation. Cogent Eng 3(1):1167990
Persyn D, Westerlund J (2008) Error-correction–based cointegration tests for panel data. Stand Genomic Sci 8(2):232–241
Pesaran MH (2004) General diagnostic tests for cross section dependence in panels; CESifo. Working Paper (No. 1229). Cambridge University, United Kingdom
Pesaran MH (2006) Estimation and inference in large heterogeneous panels with a multifactor error structure. Econometrica 74(4):967–1012
Pesaran MH (2007) A simple panel unit root test in the presence of cross-section dependence. J Appl Economet 22:265–312
Pesaran MH, Smith R (1995) Estimating long-run relationships from dynamic heterogeneous panels. J Econ 68(1):79–113
Pesaran MH, Yamaga T (2008) Testing slope homogeneity in large panels. J Econ 142:50–93
Rahman MM, Vu XB (2020) The nexus between renewable energy, economic growth, trade, urbanisation and environmental quality: a comparative study for Australia and Canada. Renew Energy 155:617–627
Rai MS (2017) Impact of urbanization on environment. Int J Emerg Technol 8(1):127–129
Ray RL, Singh VP, Singh SK, Acharya BS, He Y (2021) What is the impact of COVID-19 pandemic on global carbon emissions? Sci Total Environ 151503. https://doi.org/10.1016/j.scitotenv.2021.151503
Sarkodie SA, Strezov V (2019) A review on environmental Kuznets curve hypothesis using bibliometric and meta-analysis. Sci Total Environ 649:128–145
Sarkodie SA, Ozturk I (2020) Investigating the environmental Kuznets curve hypothesis in Kenya: a multivariate analysis. Renew Sustain Energ Rev 117:109481
Saboori B, Sulaiman JB, Mohd S (2012) An empirical analysis of the environmental Kuznets curve for CO2 emissions in Indonesia: the role of energy consumption and foreign trade. Int J Econ Financ 4:243–251
Sarkodie SA, Adams S, Owusu PA, Leirvik T, Ozturk I (2020) Mitigating degradation and emissions in China: the role of environmental sustainability, human capital and renewable energy. Sci Total Environ 719:137530
Seto KC, Fragkias M, Güneralp B, Reilly MK (2011) A meta-analysis of global urban land expansion. PLoS one 6(8):e23777
Shabani E, Hayati B, Pishbahar E, Ghorbani MA, Ghahremanzadeh M (2021) A novel approach to predict CO2 emission in the agriculture sector of Iran based inclusive multiple model. J Clean Prod 279:123708. https://doi.org/10.1016/j.jclepro.2020.123708
Sorgho R, Quiñonez CAM, Louis VR, Winkler V, Dambach P, Sauerborn R, Horstick O (2020) Climate change policies in 16 West African countries: a systematic review of adaptation with a focus on agriculture, food security, and nutrition. Int J Environ Res Public Health 17(23):8897. https://doi.org/10.3390/ijerph17238897
Ssali MW, Du J, Mensah IA, Hongo DO (2019) Investigating the nexus among environmental pollution, economic growth, energy use, and foreign direct investment in 6 selected sub-Saharan African countries. Environ Sci Pollut Res 26(11):11245–11260
Stern DI (2004) The rise and fall of the environmental Kuznets curve. World Dev 32(8):1419–1439
Tachega MA, Yao X, Liu Y, Ahmed D, Ackaah W, Gabir M, Gyimah J (2021) Income heterogeneity and the environmental Kuznets curve turning points: evidence from Africa. Sustainability 13(10):5634. https://doi.org/10.3390/su13105634
Twerefou D, Adusah-Poku F, Bekoe W (2016) An empirical examination of the environmental Kuznets curve hypothesis for carbon dioxide emissions in Ghana: an ARDL approach. Environ Socio-Econ Stud 4(4):1–12. https://doi.org/10.1515/environ-2016-0019
Ullah A, Ahmed M, Raza SA, Ali S (2021) A threshold approach to sustainable development: nonlinear relationship between renewable energy consumption, natural resource rent, and ecological footprint. J Environ Manage 295:113073
USAID (2021) West Africa Regional. https://www.usaid.gov/west-africa-regional
Vo XV, Zaman K (2020) Relationship between energy demand, financial development, and carbon emissions in a panel of 101 countries: “go the extra mile” for sustainable development. Environ Sci Pollut Res 27(18):23356–23363
Wang Q, Su M (2019) The effects of urbanization and industrialization on decoupling economic growth from carbon emission–a case study of China. Sustain Cities Soc 51:101758
Wang Q, Su M, Li R (2018) Toward to economic growth without emission growth: the role of urbanization and industrialization in China and India. J Clean Prod 205:499–511
Wang Q, Zhang F (2021) What does the China’s economic recovery after COVID-19 pandemic mean for the economic growth and energy consumption of other countries? J Clean Prod 295:126265. https://doi.org/10.1016/j.jclepro.2021.126265
Wang Q, Guo J, Li R (2021) Official development assistance and carbon emissions of recipient countries: a dynamic panel threshold analysis for low-income countries and lower-middle-income countries. Sustain Prod Consumption. https://doi.org/10.1016/j.spc.2021.09.015
Wang Q, Li S, Li R, Jiang F (2022a) Underestimated impact of the COVID-19 on carbon emission reduction in developing countries — a novel assessment based on scenario analysis. Environ Res 204:111990. https://doi.org/10.1016/j.envres.2021.111990
Wang Q, Wang X, Li R (2022b) Does urbanization redefine the environmental Kuznets curve? An empirical analysis of 134 countries. Sustain Cities Soc 76:103382. https://doi.org/10.1016/j.scs.2021.103382
Wasiu OI, Alasinrin BK (2015) Growth thresholds and environmental degradation in sub-Saharan African countries: an exploration of Kuznets hypothesis. Int J Manag Account Econ 2:858–871
Westerlund J (2007) Testing for panel cointegration with multiple structural breaks. Oxford Bull Econ Stat 68(1):101–132
Westerlund J (2008) Panel cointegration tests of the Fisher effect. J Appl Economet 23(2):193–233
World Bank (2021) World development indicators 2021. World Bank, Washington, DC
Xu Y, Fan X, Zhang Z, Zhang R (2020) Trade liberalisation and haze pollution: evidence from China. Ecol Ind 109:105825. https://doi.org/10.1016/j.ecolind.2019.105825
Xue C, Shahbaz M, Ahmed Z, Ahmad M, Sinha A (2022) Clean energy consumption, economic growth, and environmental sustainability: what is the role of economic policy uncertainty? Renewable Energy 184:899–907
Zeeshan M, Ullah I, Rehman A, Afridi FE (2022) Trade openness and urbanization impact on renewable and non-renewable energy consumption in China. Environ Sci Pollut Res: 1–16
Zeraibi A, Balsalobre-Lorente D, Shehzad K (2021) Testing the environmental Kuznets curve hypotheses in Chinese provinces: a nexus between regional government expenditures and environmental quality. Int J Environ Res Public Health 18(18):9667. https://doi.org/10.3390/ijerph18189667
Funding
We acknowledge the funding support of the National Natural Science Foundation (grant numbers 71973054; 71371087; 20BGL099).
Author information
Authors and Affiliations
Contributions
LJ contributed to all aspects of the project, including conceptualization, methodology, formal analysis, and writing. KC contributed to all aspects of the project, including conceptualization, methodology, formal analysis, and writing. ZJ contributed to conceptualization, leadership, and writing. YK was involved in conceptualization, leadership, and writing. AHN contributed to all aspects of the project, including conceptualization, data extraction, methodology, formal analysis, writing, and proofreading.
Corresponding author
Ethics declarations
Ethical approval
Not applicable.
Consent to participate
Not applicable
Consent for publication
Not applicable
Competing interests
The authors declare no competing interests.
Additional information
Responsible Editor: Ilhan Ozturk
Publisher's note
Springer Nature remains neutral with regard to jurisdictional claims in published maps and institutional affiliations.
Highlights
• The relationship between economic growth and environmental degradation in West Africa is explored within the EKC framework.
• Second-generation panel econometric estimators robust to cross-sectional dependent and income heterogeneity are implemented on panel data of 16 West African countries.
• Economic growth hastens environmental degradation in West African countries, with a greater impact in lower-middle-income countries (LMICs) than in low-income countries (LICs) in West Africa.
• EKC hypothesis is valid in West Africa, particularly LMICS, whereas a monotonous increasing relationship exists in LICs.
• Feedback mechanisms exist between economic growth and environmental degradation in West Africa across LMICs and LICs.
Rights and permissions
About this article
Cite this article
Jian, L., Chuimin, K., Jijian, Z. et al. The relationship between economic growth and environmental degradation: could West African countries benefit from EKC hypothesis?. Environ Sci Pollut Res 29, 73052–73070 (2022). https://doi.org/10.1007/s11356-022-21043-x
Received:
Accepted:
Published:
Issue Date:
DOI: https://doi.org/10.1007/s11356-022-21043-x