Abstract
This paper is concerned with global robust exponential stability for a class of delayed cellular neural networks with time-varying delays. Some new sufficient conditions are presented for the uniqueness of equilibrium point and the global stability of cellular neural networks with time varying delay by constructing Lyapunov functional and using linear matrix inequality and the integral inequality approach. Numerical examples are illustrated to show the effectiveness of the proposed method. From the simulation results, significant improvement over the recent results can be observed.


Similar content being viewed by others
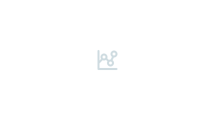
References
Arik S (2002) An analysis of global asymptotic stability of delayed cellular neural networks. IEEE Trans Neural Netw 13(5):1239–1242
Boyd SL, Feron GE, Balakrishnan V (1994) Linear matrix inequalities in system and control theory. SIAM, Philadelphia
Chandrasekar A, Murugesu R, Rakkiyappan R (2016) Exponential stability for Markovian jumping stochastic BAM neural networks with mode-dependent probabilistic time-varying delays and impulse control. Complexity 21(5):59–72
Chua LO, Yang L (1988) Cellular neural networks: theory. IEEE Trans Circuits Syst 35(10):1257–1272
Chua LO, Roska T (2002) Cellular neural networks and visual computing. Cambridge University Press, Cambridge
Gahinet P, Nemirovski A, Laub A, Chilali M (1995) LMI control toolbox user’s guide. The Mathworks, Massachusetts
Lakshmanan S, Balasubramaniam P (2011) New results of robust stability analysis for neutral-type neural networks with time-varying delays and Markovian jumping parameters. Can J Phys 89(8):827–840
Lakshmanan S, Park JH, Jung HY, Balasubramaniam P (2012) Design of state estimator for neural networks with leakage, discrete and distributed delays. Appl Math Comput 218(22):11297–11310
Lee TH, Lakshmanan S, Park JH, Balasubramaniam P (2013) State estimation for genetic regulatory networks with mode-dependent leakage delays, time-varying delays, and Markovian jumping parameters. IEEE Trans Nanobiosci 12(4):363–375
Liu PL (2003) Exponential stability for linear time-delay systems with delay dependence. J Frankl Inst 340:481–488
Liu PL (2003) Robust exponential stabilization for uncertain systems with state and control delay. Int J Syst Sci 34(12–13):675–682
Liu PL (2009) Robust exponential stability for uncertain time-varying delay systems with delay dependence. J Frankl Inst 346(10):958–968
Liu PL (2012) Global asymptotic stability for neural networks systems with discrete and distributed delay. ICIC Express Lett Part B Appl 1(2):233–240
Liu PL (2012) Further results on the exponential stability criteria for time delay singular systems with delay-dependence. Int J Innov Comput Inf Control 8(6):4015–4024
Liu PL (2013) Improved delay-dependent robust stability criteria for recurrent neural networks with time-varying delays. ISA Trans 52(1):30–35
Liu PL (2013) Delay-dependent global exponential robust stability for delayed cellular neural networks with time-varying delay. ISA Trans 52(6):711–716
Park JH (2006) Global exponential stability of cellular neural networks with variable delays. Appl Math Comput 183(2):1214–1219
Park JH (2007) Further note on global exponential stability of uncertain cellular neural networks with variable delays. Appl Math Comput 188(1):850–854
Rakkiyappan R, Chandrasekar A, Lakshmanan S, Park JH (2015) Exponential stability for Markovian jumping stochastic BAM neural networks with mode-dependent probabilistic time-varying delays and impulse control. Complexity 20(3):39–65
Rakkiyappan R, Chandrasekar A, Lakshmanan S (2016) Stochastic sampled data robust stabilisation of T-S fuzzy neutral systems with randomly occurring uncertainties and time-varying delays. Int J Syst Sci 47(10):2247–2263
Roska T, Chua LO (1992) Cellular neural networks with nonlinear and delay-type template. Int J Circuit Theory Appl 20(4):469–481
Xie JC, Chen CP, Liu PL, Jeng YC (2014) Robust exponential stability analysis for delayed neural networks with time-varying delay. Adv Differ Equ 2014:131–147
Yu GJ, Lu CY, Tsai JSH, Su TJ, Liu BD (2003) Stability of cellular neural networks with time-varying delay. IEEE Trans Circuits Syst 50(5):677–679
Zhang Q, Wei X, Xu J (2005) Delay-dependent exponential stability of cellular neural networks with time-varying delays. Chaos Solitons Fractals 23(4):1363–1369
Zhang Q, Wei X, Xu J (2005) On global exponential stability of delayed cellular neural networks with time-varying delays. Appl Math Comput 162(2):679–686
Zhu QX, Cao J (2010) Robust exponential stability of Markovian jump impulsive stochastic Cohen–Grossberg neural networks with mixed time delays. IEEE Trans Neural Netw 21(8):1314–1325
Zhu QX, Li X, Yang XS (2011) Exponential stability for stochastic reaction-diffusion BAM neural networks with time-varying and distributed delays. Appl Math Comput 217(13):6078–6091
Zhu QX, Cao J (2011) Exponential stability of stochastic neural networks with both Markovian jump parameters and mixed time delays. IEEE Trans Syst Man Cybern B Cybern 41(2):341–353
Zhu QX, Cao J (2012) Stability of Markovian jump neural networks with impulse control and time varying delays. Nonlinear Anal Real World Appl 13(5):2259–2270
Zhu QX, Rakkiyappan R, Chandrasekar A (2014) Stochastic stability of Markovian jump BAM neural networks with leakage delays and impulse control. Neurocomputing 136:136–151
Zhu QX, Cao J, Rakkiyappan R (2015) Exponential input-to-state stability of stochastic Cohen–Grossberg neural networks with mixed delays. Nonlinear Dyn 79(2):1085–1098
Author information
Authors and Affiliations
Corresponding author
Appendix A: Proof of Lemma 1
Appendix A: Proof of Lemma 1
For any positive semi-definite matrices
the following integral inequality holds
Proof
The New-Leibniz formula and integral inequality approach are used to derive the lemma; they are stated below:
\(\square \)
Rights and permissions
About this article
Cite this article
Liu, PL. Further Improvement on Delay-Dependent Global Robust Exponential Stability for Delayed Cellular Neural Networks with Time-Varying Delays. Neural Process Lett 47, 1177–1195 (2018). https://doi.org/10.1007/s11063-017-9683-6
Published:
Issue Date:
DOI: https://doi.org/10.1007/s11063-017-9683-6