Abstract
What determines the differences in economic performance across European regions? In addressing this question, this paper takes inspiration from two different approaches. One approach highlights the role of capability-building, of a technological or social nature, while another perspective emphasizes the potential advantages of proximity and, hence, a relatively diversified economic structure, for regional economic performance. The paper argues that the impacts of capability-building and diversification on regional economic development need to be assessed jointly. Using information for 261 regions at NUTS2 level in 27 European countries in the 2000s, novel data sources are exploited to construct measures of technological and social capabilities, which are combined with indicators of related and unrelated variety in the analysis of regional economic dynamics. The results suggest that capability-building play a key role in regional economic development while the results for diversification are more mixed.




Similar content being viewed by others
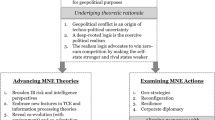
Notes
For an overview of the literature on social capital, see Portes 1998.
See Boschma (2005) for an overview and discussion of the role of proximity for innovation.
However, as pointed out by one of the referees to this paper, according to the economist John Sutton’s “bounds approach” to the study of technology and market structure (Sutton, 1998), low related variety may be expected to go together with high concentration in R&D-intensive industries, suggesting the possibility of high innovation and growth, i.e., counteracting the effect emphasized by Boschma (2005) and others.
Another hypothesis suggested by Frenken et al. (2007), and which was supported by their data, was that “related variety” would be positively correlated with increases in employment.
According to the number of patent applications to the European Patent Office (EPO) by priority year in Regional science and technology statistics from Eurostat (2020).
That is, in Cortinovis and van Oort (2015)’s sample, there is a sufficient number of employees in most regions to compute reliable indices.
If appropriate the indicators are adjusted by the size of the region (i.e. per capita or as % of GDP etc.).
Note that, due to data availability, observations sometimes are from different years. For example, the European Value Study was carried out in different years in different countries. However, this problem should not be exaggerated. In fact, for the indicators associated with the technological capability measure, all observations are from a very narrow time span (the years 2004–2006). The same holds for the indicators associated with social capability (the years 2008–2010) with the exception of one single indicator (which is from the years 2004–2006). See Table A.1 in the appendix to this paper for more details.
See also the correlation table in appendix (Table A4).
See Fagerberg et al. 2010 for a more detailed discussion. In addition, the regional CIS indicators are available at NUTS1 level only.
As shown by Barro and Sala-i-Martin (2003, pp. 274–275), the inclusion of the GDP per capita variable may be consistent both with Solow’s traditional neoclassical growth model (in which case the level of GDP per capita is assumed to reflect the capital intensity of the economy) and a Schumpeterian perspective (with a low GDP per capita indicating a high potential for diffusion).
This is a well-known problem in the empirical literature on economic growth, see, e.g., the discussion in Durlauf et al. (2005). One remedy that is often recommended is the use of instrumental variables, i.e., exogenous variables that do not belong to the model but that are nevertheless correlated with the (endogenous) explanatory factors, provided that such instruments can be found. However, scarcity of data, and the fact we have already exhausted most relevant data sources in our search for indicators, prevent us from pursuing this line of analysis here.
Cook’s distance with the conventional cut-off point at 4 / number of observations was used to exclude the outliers.
Hence, the estimated coefficients refer to the impact of changing an independent variable by one standard deviation.
A backward stepwise search for the best model specification was conducted using a criterion of 20% statistical significance level for exclusion and 10% statistical significance level for re-inclusion of a variable in the model.
Note that the impact of related variety for Eastern European regions (regression 3 in Table 4) is the sum of the general effect (common to all regions), which is positive, and the specific effect for Eastern regions, which is negative. Hence, these two effects tend to cancel each other, since the absolute value of the estimate is about the same in the two cases, with the result that the total effect becomes negligible.
The ten regions that according to the estimates (based on regression 3 in Table 4) receive the highest impetus to growth from related variety are Veneto, Lombardia and Emilia-Romagna in Northern Italy; Schwaben in Southern Germany; Cataluña, Comunidad Valenciana, Castilla-la Mancha and Aragón in Spain; and, finally, two Portuguese regions (Centro & Norte). Among the next ten, there are five more regions from Spain, two Southern German regions, and one Italian region, illustrating the strong geographical concentration of the phenomenon.
See, e.g., https://ec.europa.eu/info/strategy/priorities-2019-2024/european-green-deal_en, accessed on 07/06/2021.
References
Abramovitz, M. (1986). Catching up, forging ahead, and falling behind. Journal of Economic History, 46, 386–406
Abramovitz, M. (1994a). ‘The origins of the postwar catch-up and convergence boom’. In J. Fagerberg, B. Verspagen, & N. von Tunzelmann (Eds.), The dynamics of technology, trade and growth (pp. 21–52). Aldershot, UK and Brookfield, VT, USA: Edward Elgar
Abramovitz, M. (1994b). ‘Catch-up and convergence in the postwar growth boom and after’. In W. J. Baumol, R. R. Nelson, & E. N. Wolf (Eds.), Convergence of productivity—cross-national studies and historical evidence (pp. 86–125). Oxford, UK and New York, NY, USA: Oxford University Press
Annoni, P., & Kozovska, K. (2010). EU regional competitiveness index 2010. Luxembourg: European Commissionhttps://ec.europa.eu/regional_policy/en/information/publications/working-papers/2011/a-new-regional-competitiveness-index-theory-methods-and-findings
Archibugi, D., & Coco, A. (2005). ‘Measuring technological capabilities at the country level: A survey and a menu for choice’. Research Policy, 34(2), 175–194
Barro, R. J. (1991). Economic growth in a cross section of countries. Quarterly Journal of Economics, 106, 407–443
Barro, R. J., & Sala-i-Martin, X. (2003). Economic growth (2nd ed.). The MIT Press Cambridge, MA
Bell, M., & Pavitt, K. (1993). ‘Technological accumulation and industrial growth: Contrasts between developed and developing countries’. Industrial Corporate Change, 2(1), 157–210
Boschma, R. A. (2005). ‘Proximity and innovation: A critical assessment. Regional Studies, 39(1), 61–74
Boschma, R. A., & Iammarino, S. (2009). ‘Related variety, trade linkages and regional growth’. Economic Geography, 85(3), 289–311
Braczyk, H. J., Cooke, P., & Heidenreich, M. (Eds.). (1998). Regional innovation systems: The role of governances in a globalized world. London, UK: Routledge
Castaldi, C., Frenken, K., & Los, B. (2015). Related variety, unrelated variety and technological breakthroughs: An analysis of US state-level patenting. Regional Studies, 49(11), 1938–1940
Charron, N., Dijkstra, L., & Lapuente, V. (2015). Mapping the regional divide in Europe: A measure for assessing quality of government in 206 European Regions. Social Indicators Research, 122, 315–346
Cohen, W. (1995). “Empirical studies of innovative activity”. In P. Stoneman (Ed.), Handbook of the economics of innovation and technological change (pp. 182–264). Oxford: Blackwell
Corden, W. M. (1984). The normative theory of international trade. In P. B. Kenen, & R.W. Jones (Eds.), Handbook of international economics (vol. 1, pp. 63–130). Elsevier
Cortinovis, N., & van Oort, F. (2015). ‘Variety, economic growth and knowledge intensity of European regions: A spatial panel analysis’. Annals of regional science, forthcoming. https://doi.org/10.1007/s00168-015-0680-2
Cortinovis, N., Xiao, J., Boschma, R., & van Oort, F. G. (2017). Quality of government and social capital as drivers of regional diversification in Europe. Journal of Economic Geography, 17(6), 1179–1208
Crescenzi, R., Rodríguez-Pose, A., & Storper, M. (2007). The territorial dynamics of innovation: A Europe-United States comparison. Journal of Economic Geography, 7, 673–709
Dixit, A., & Norman, V. (1980). Theory of international trade: A dual, general equilibrium approach (Cambridge Economic Handbooks). Cambridge: Cambridge University Press
Dowrick, S. (1997). Trade and growth: A survey. In J. Fagerberg et al. (Ed.), Technology and international trade (pp. 107–126). Elgar
Durlauf, S. N., Johnson, P. A., & Temple, J. R. W. (2005). Growth Econometrics. In P. Aghion, & S. N. Durlauf (Eds.), Handbook of Growth Economics (pp. 556–677). Elsevier
European Commission. (2020). Annual regional database of the European Commission’s Directorate General for regional and Urban Policy (ARDECO). Release: 2020.02, https://knowledge4policy.ec.europa.eu/territorial/ardeco-database_en
Eurostat. (2020). Regional statistics by NUTS classification. https://ec.europa.eu/eurostat/web/regions/data/database, download of 23. 9. 2020
EVS. (2016). European values study 2008: Integrated dataset (EVS 2008). GESIS data archive, Cologne. ZA4800 data file version 4.0.0, https://doi.org/10.4232/1.12458
Fagerberg, J., & Srholec, M. (2008). ‘National innovation systems, capabilities and economic development’. Research Policy, 37(9), 1417–1435
Fagerberg, J., & Srholec, M. (2017). Explaining regional economic performance: The role of competitiveness, specialization and capabilities. In R. Huggins, & P. Thompson (Eds.), Handbook of regions and competitiveness: Contemporary theories and perspectives on economic development (pp. 117–135). Edward Elgar Publishing
Fagerberg, J., & Srholec, M. (2020). Capabilities, competitiveness, nations. In Jeong-Dong Lee, Keun Lee, Slavo Radosevic, Dirk Meissner, Nicholas S. Vonortas (Eds.), “The challenges of technology and economic catch-Up in emerging economies” (p. 37–66). Oxford University Press
Fagerberg, J., Srholec, M., & Verspagen, B. (2010). Innovation and economic development. In B. Hall, & N. Rosenberg (Eds.), Handbook of the economics of innovation (pp. 833–872, Vol. II.). Amsterdam: North-Holland
Fagerberg, J., Feldman, M., & Srholec, M. (2014). ‘Technological dynamics and social capability: U.S. states and European nations’. Journal of Economic Geography, 14(2), 313–337
Frenken, K., Van Oort, F., & Verburg, T. (2007). ‘Related variety, unrelated variety and regional economic growth’. Regional Studies, 41(5), 685–697
Gerschenkron, A. (1962). Economic backwardness in historical perspective. Cambridge, MA, USA: The Belknap Press
Griliches, Z. (1990). Patent statistics as economic indicators: A survey. Journal of Economic Literature, 28(4), 1661–1707
Hidalgo, C. A., & Hausmann, R. (2009). The building blocks of economic complexity. Proceedings of the national academy of sciences, 106(26), 10570–10575
Hidalgo, C. A., Klinger, B., Barabási, A. L., & Hausmann, R. (2007). The product space conditions the development of nations. Science, 317(5837), 482–487
Jacquemin, A. P., & Berry, C. H. (1979). ‘Entropy measure of diversification and corporate growth’. Journal of Industrial Economics, 27(4), 359–369
Kim, L. (1980). ‘Stages of development of industrial technology in a developing country: A model’. Research Policy, 9(3), 254–277
Kim, L. (1997). Imitation to innovation: The dynamics of Korea’s technological learning. Boston, MA, USA: Harvard Business School Press
Lall, S. (1992). ‘Technological capabilities and industrialization’. World Development, 20(2), 165–186
Li, G. (1985). Robust regression. In D. C. Hoaglin, F. Mosteller, & J. W. Tukey (Eds.), Exploring data tables, trends, and shapes (pp. 281–340). New York: Wiley
Lundvall, B. A. (Ed.). (1992). National systems of innovation: Towards a theory of innovation and interactive learning. London, UK: Pinter Publishers
McCann, P, & Ortega-Argilés, R. (2015). ‘Smart specialization, regional growth and applications to European Union cohesion policy’. Regional Studies, 49(8), 1291–302
Miguelez, E., & Moreno, R. (2018). Relatedness, external linkages and regional innovation in Europe. Regional Studies, 52(5), 688–701
Portes, A. (1998). Social capital: Its origins and applications in modern sociology. Annual Review of Sociology, 24, 1–24
Sutton, J. (1998). Technology and market structure. Cambridge, MA: MIT Press
Whittle, A., & Kogler, D. F. (2020). Related to what? Reviewing the literature on technological relatedness: Where we are now and where can we go? Papers in Regional Science, 99(1), 97–113
Acknowledgements
Martin Srholec gratefully acknowledges funding from the Czech Science Foundation (GAČR) project no 17-09265S and the Czech Academy of Sciences for the R&D&I Analytical Centre (RaDIAC). Jan Fagerberg would for his part like to acknowledge support from INTRANSIT, Centre for Technology, Innovation and Culture, University of Oslo (project number 295021). We thank Nicola Cortinovis and Frank van Oort (Cortinovis & van Oort, 2015) and Ernest Miguelez and Rosina Moreno (Miguelez & Moreno, 2018) for providing access to data on the indices of related and unrelated variety in European regions, and the editors and referees of this journal for helpful advice. All usual caveats apply.
Author information
Authors and Affiliations
Corresponding author
Additional information
Publisher’s note
Springer Nature remains neutral with regard to jurisdictional claims in published maps and institutional affiliations.
Appendix (data & sources)
Appendix (data & sources)
An overview of definitions and sources of the data is given in Table A1. Sample size and composition and reference periods were determined by the availability of data. For the indicators of technological and social capabilities we searched for data from the middle of the 2000s. However, due to lack of availability, some of the indicators are from the end of the decade. This holds particularly for indicators associated with social capability. Although the selected indicators have broad coverage, in some cases there were missing values that had to be estimated using the impute procedure in Stata/MP 15.1 (see the Stata 15.1 Manual for details). We based the estimation on data for the other indicators of technological and social capabilities used to construct the capability measures. The number of observations estimated by the procedure is given in the last column of Table A1.
All regions of the 27 countries located in the mainland Europe are included in the analysis (only small overseas and/or dependent territories, including Guadeloupe, Martinique, Guyane, Réunion, Ceuta, Melilla, Acores and Madeira, have been excluded). The regions refer to the second level of the division of the Nomenclature of Territorial Units for Statistics (NUTS2). Due to data limitations a combination of NUTS1 and NUTS2 regions was used in some cases. For instance, EVS (2016) data is at NUTS1 level in large countries that are divided in many regions (i.e. Germany, France, Italy, Spain and the United Kingdom), because the number of respondents was too small to derive reliable indicators at more detailed disaggregation. Due to changes in the NUTS2 classification some regions had to be merged using population as weight in averaging of the indicators (Brandenburg—Nordost (DE41) and Brandenburg—Südwest (DE42) merged in Brandenburg (DE40); Helsinki-Uusimaa (FI1B) and Etelä-Suomi (FI1C) merged in Etelä-Suomi (FI18); Inner London—West (UKI3) and Inner London—East (UKI4) merged in Inner London (UKI1) etc.). The full list of regions included in the analysis is given in Table A2.
Rights and permissions
About this article
Cite this article
Fagerberg, J., Srholec, M. Capabilities, diversification & economic dynamics in European Regions. J Technol Transf 48, 623–644 (2023). https://doi.org/10.1007/s10961-022-09917-1
Received:
Revised:
Accepted:
Published:
Issue Date:
DOI: https://doi.org/10.1007/s10961-022-09917-1