Abstract
In this study, Adaptive auto regressive-moving average (A-ARMA) analysis of EMG signals recorded on the ulnar nerve region of the right hand in resting position was performed. A-ARMA method, especially in the calculation of the spectrums of stationary signals, is used for frequency analysis of signals, which give frequency response as sharp peaks and valleys. In this study, as the result of A-ARMA method analysis of EMG signals frequency–time domain, frequency spectrum curves (histogram curves) were obtained. As the images belonging to these histograms were evaluated, fibrillation potential widths of the muscle fibers of the ulnar nerve region of the people (material of the study) were examined. According to the degeneration degrees of the motor nerves, 22 people had myopathy, 43 had neuropathy, and 28 were normal.





Similar content being viewed by others
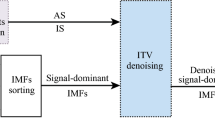
References
Deluca, C. J., Towards understanding the EMG signal ch 3 of muscles alive, 4th edn. Williams & Wilkonson: Baltimore, 1978.
Seroussi, R., The design and use of a microcomputerized real-time muscle fatigue monitor based on the median frequency shift in the electromyographic signal. IEEE Trans. Biomed. Eng. 36(2):284–286, 1989.
Hagg, G. M., Interpretation of EMG spectral alterations and alteration index at sustained contraction. J. Appl. Physiol. 73(4):1211–1217, 1992.
Bigland-Ritchie, B., Donovan, E. F., and Roussos, C. S., Conduction velocity and EMG power spectrum changes in fatigue of sustained maximal efforts. J. Appl. Physiol. 51(5):1300–1305, 1981.
Broman, H., Bilotto, G., and DeLuca, C. J., Myoelectric signal conduction velocity and spectral parameters influence of force and time. J. Appl. Physiol. 58(5):1428–1437, 1985.
Graupe, D., Stochastic analysis of myoelectric temporal signatures for multifunctional single-site activation of prostheses and orthoses. J. Biomed. Eng. 7:18–29, 1985.
Graupe, D., EMG pattern analysis for patient-responsive control of FES in paraplegics for walker-supported walking. IEEE. Trans. Biomed Eng. 36:711–719, 1989.
Saridis, G. N., and Gootee, T. P., EMG pattern analysis and classification for a prosthetic arm. IEEE. Trans. Biomed. Eng. 29:403–412, 1982.
Trio, R. J., Nash, D. H., and Moskowitz, G. D., The identification of time series models of lower extremity EMG for the control of prostheses using Box–Jenkins criteria. IEEE. Trans. Biomed. Eng. 35:584–594, 1988.
Kang, W. J., Shiu, J. R., Cheng, C. K., Lai, J. S., Tsao, H. W., and Kuo, T. S., The application of cepstral coefficients and maximum likelihood method in EMG pattern recognition. IEEE. Trans. Biomed. Eng. 42:777–785, 1995.
Mananas, M. A., Jane, R., Fiz, J. A., Morera, J., and Caminal, P., Influence of estimators of spectral density on the analysis of electromyographic and vibromyographic signals. Med. Biol. Eng. Comput. 40(1):90–98, 2002.
Korosec, D., Parametric estimation of the continuous non-stationary spectrum and its dynamics in surface EMG studies. Int. J. Med. Inf. 58–59:59–69, 2000.
Proakis, J. G., and Manolakis, D. G., Digital signal processing principles algorithms and applications. Prentice-Hall: Englewood Cliffs, NJ, 1996.
Akay, M., Time frequency and wavelets in biomedical signal processing. IEEE Press: New York, 1998.
Chen, J., Vandewalle, J., and Sansen, W., Adaptive method for cancellation of respiratory artifact in electrastris measurement. Med. Biol. Eng. Comput. 27:57–63, 1989.
Chen, J., Adaptive altering and its applications in echo cancellation and biomedical signal processing. Belgium: Ph.D. Thesis, Katholieke Universiteit Leuven, 1989.
Chen, J., Vandewalle, J., Sansen, W., Vantrappen, G., and Janssens J., Adaptive spectral analysis of coetaneous electrical signals using autoregressive moving average modeling. Med. Biol. Eng. Comput. 28:531–536, 1990.
Proakis, J. G., and Manolakis, D. G., Digital signal processing principles, algorithms, and applications. Prentice-Hall: Englewood Cliffs, NJ, 1996.
Kay, S. M., and Marple, S. L., Spectrum analysis—a modern perspective. Proc. IEEE. 69:1380–1419, 1981.
Stoica, P., and Moses, R., Introduction to spectral analysis. Prentice-Hall: Englewood Cliffs, NJ, 1997.
Kay, S. M., Modern spectral estimation: Theory and application. Prentice-Hall: Englewood Cliffs, NJ, 1988.
Akaike, H., A new look at the statistical model identification. IEEE Trans. Automat. Contr. AC-19:716–723, 1974.
Akay, M., Semmlow, J. L., Welkowitz, W., Bauer, D., and Kostis J. B., Noninvasive detection of coronary stenoses before and after angioplasty using eigenvector methods. IEEE Trans. Biomed. Eng. 37:1095–1104, 1990.
Semmlow, J. L., Akay, M., and Welkowitz, W., Noninvasive detection of coronary artery disease using parametric spectral analysis methods. IEEE Eng. Med. Biol. Mag. 9:33–36, 1990.
Wax, M., and Kailath, T., Detection of signals by information theoretic criteria. IEEE Trans. Acoust. Speech Signal Process. ASSP-33(2):387–392, 1985.
Author information
Authors and Affiliations
Corresponding author
Rights and permissions
About this article
Cite this article
Barişçi, N. The Adaptive ARMA Analysis of EMG Signals. J Med Syst 32, 43–50 (2008). https://doi.org/10.1007/s10916-007-9106-8
Received:
Accepted:
Published:
Issue Date:
DOI: https://doi.org/10.1007/s10916-007-9106-8